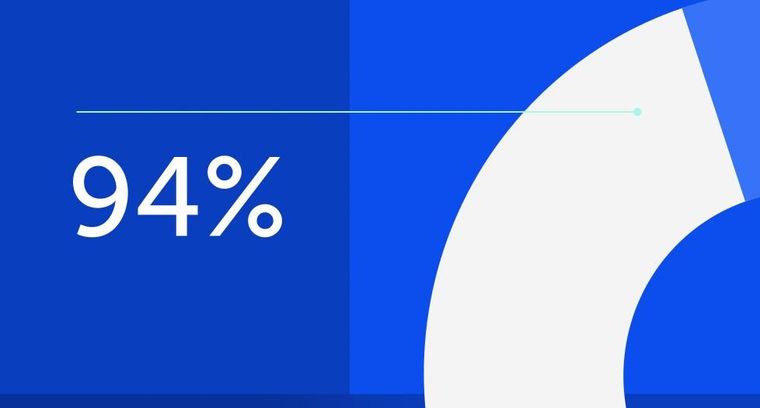
94% of researchers rate our articles as excellent or good
Learn more about the work of our research integrity team to safeguard the quality of each article we publish.
Find out more
ORIGINAL RESEARCH article
Front. Cardiovasc. Med., 03 April 2025
Sec. Heart Failure and Transplantation
Volume 12 - 2025 | https://doi.org/10.3389/fcvm.2025.1476815
Background: The role of the triglyceride-glucose (TyG) index in critically ill patients with congestive heart failure (CHF) and atrial fibrillation (AF), requiring intensive care unit (ICU) admission, remains unclear. This study aimed to investigate the association between the TyG index and the clinical prognosis of critically ill patients with CHF and AF.
Methods: This retrospective observational cohort study utilized data from the Medical Information Mart for Intensive Care-IV (MIMIC IV2.2) database. Participants were categorized into four groups based on TyG index level. The primary outcome was hospital all-cause mortality. Multivariable logistic proportional regression analysis and restricted cubic spline regression were employed to assess the TyG index's association with hospital mortality in patients with CHF and AF. Sensitivity analysis included determining the TyG index's feature importance through subgroup analysis in different subgroups.
Results: A total of 787 patients were included in the study, with hospital and ICU mortalities of 14.2% and 8.3%, respectively. Multivariate logistic regression analysis demonstrated that the TyG index was independently associated with an increased risk of hospital mortality (odds ratio (OR), 1.59 [95% confidence interval (CI) 1.15–2.19], P = 0.005) and ICU mortality [OR 1.9; (95% CI 1.28–2.83), P = 0.001] after adjusting for confounders. The restricted cubic spline regression model indicated a linear increase in the risks of in-hospital and ICU mortality with a higher TyG index. Sensitivity analysis revealed consistent effect sizes and directions in different subgroups, ensuring result stability.
Conclusions: The results of our study suggest a significant association between the TyG index and hospital and ICU all-cause mortality in critically ill patients with CHF and AF. This finding implies that the TyG index could potentially serve as a valuable tool for identifying patients with CHF and AF at an elevated risk of all-cause mortality.
Heart failure (HF) is defined as the heart's inability to maintain adequate cardiac output without resorting to maladaptive compensatory mechanisms (1). Annually, over one million patients with HF are admitted to hospitals in the United States and Europe (2). Based on the findings of multiple heart failure registries, the in-hospital mortality rate for patients with acute heart failure (AHF) is 2%–8%. Within 3 months after discharge, the mortality rate reaches 10%–15%, with a readmission rate of 20%–30%. One year after discharge, the mortality rate reaches 20%–30%, and the readmission rate soars to 30%–50% (3–5). Due to the presence of common risk factors and the intricate interaction between them, atrial fibrillation (AF) and HF are observed to coexist in a significant proportion of patients, reaching up to 50% in some cases.1 An insightful analysis of participants from the Framingham Heart Study, who developed either new-onset AF or HF, uncovered compelling findings: 32% of patients diagnosed with HF had a prior history of AF, with an additional 30% being diagnosed with AF thereafter. Similarly, amongst those with new-onset AF, 8% had a pre-existing diagnosis of HF, while a further 28% were diagnosed with HF at a later stage (6). However, patients may already have symptomatic structural or functional cardiac abnormalities preceding CHF development (7, 8). Identifying prognostic-related biomarkers is crucial for early detection of high-risk patients, enabling more aggressive treatment measures.
Although the precise pathogenesis of CHF and AF remains unclear, insulin resistance (IR) plays a significant role (9). The triglyceride (TG)-glucose (TyG) index, closely associated with the progression of metabolic disorders, has emerged as a reliable surrogate marker for assessing IR (10, 11). Several studies have linked the TyG index to various metabolic, cardiovascular, and cerebrovascular diseases (12–14). However, there is a lack of research in the literature examining the relationship between the TyG index and CHF with AF. This study aimed to investigate the association between the TyG index and the clinical outcome of patients with CHF and AF.
This study utilized data from the Medical Information Mart for Intensive Care-IV (MIMIC-IV) database, a repository of comprehensive medical records from patients admitted to the intensive care units (ICUs) at Beth Israel Deaconess Medical Center (15). The MIT Computational Physiology Laboratory managed this database. The author (Fuqiang Kan), authorized to access the dataset (ID: 12552525), completed the US National Institutes of Health's online course on protecting human research participants and oversaw data extraction. Adherence to STROBE guidelines for observational research was maintained.
Patients meeting the following criteria were included: (a) Diagnosed with CHF and AF based on the ninth revision of the International Classification of Diseases (ICD-9) code (code 4280 and code 42731); (b) Adults aged 18 and older; (c) First admission to ICUs. Participants lacking TGs and fasting blood glucose (FBG) data on their initial ICU admission day were excluded. A total of 787 patients were ultimately included and categorized into four groups based on their TyG index quartiles on the first ICU admission day (Figure 1).
Data extraction utilized PostgresSQL software (version 16) and Navicat Premium software (version 16.1.15) through Structured Query Language execution.
Variable extraction comprised four main groups: (a) Demographics—age, gender, and race; (b) Comorbidities—Hypertension, diabetes, and chronic kidney disease (CKD); (c) Laboratory indicators—white blood cells (WBCs), red blood cells, hemoglobin, platelets, serum sodium, serum potassium, serum calcium, anion gap, international normalized ratio (INR), urea nitrogen, serum creatinine, total cholesterol (TC), low-density lipoprotein cholesterol (LDL-C), high-density lipoprotein cholesterol (HDL-C), FBG, and TG; (d) Severity of illness scores at admission—Acute Physiology Score III (APSIII), simplified Acute Physiology Score II (SAPS-II), Oxford Acute Severity of Illness Score (OASIS), and Sepsis-related Organ Failure Assessment score (SOFA). It is worth noting that CKD is a well-known risk marker, and in this study, CKD was diagnosed according to the Ninth Revision of the ICD-9 codes (codes 5859). The TyG index was calculated using the formula (16). All variables and severity scores were extracted within the first 24 h of ICU admission.
The primary focus of this study was hospital mortality, with secondary attention to ICU mortality.
Continuous variables were portrayed as mean ± SD or median (interquartile range) based on data distribution, while categorical variables were presented as proportions. Differences in continuous variables were examined using the analysis of variance test or the rank sum test. For categorical variables, the Chi-square test or Fisher's exact test was employed to compare the characteristics of subjects in the result group.
Kaplan–Meier survival analysis assessed endpoint incidence rates among TyG index groups, with log-rank tests determining differences. Multivariable logistic regression models calculated odds ratios (ORs) and 95% confidence intervals (CIs) between the TyG index and endpoints, adjusting for various models. Confounding variables were selected based on a P value <0.05 in univariate analysis. Clinically relevant and prognosis-associated variables were included in the multivariate model: model 1 (unadjusted); model 2 (adjusted for age, gender, and race); model 3 (adjusted for age, gender, race, hypertension, diabetes, CKD, WBC, hemoglobin, serum potassium, serum calcium, anion gap, INR, urea nitrogen, serum creatinine, and LDL).
Additionally, a restricted cubic spline regression model analyzed the nonlinear relationship between the baseline TyG index and hospital and ICU mortality. The TyG index was incorporated into models as continuous or categorical variables, using the first quartile as the reference group. P values for trends were calculated based on quartile levels. A stratified analysis, considering age, gender, hypertension, diabetes, and CKD, determined the TyG index's consistency for primary outcomes. Interaction between the TyG index and stratification variables was assessed through the likelihood-ratio test.
This study employed a two-tailed test, considering a P-value <0.05 statistically significant. For missing data, a single imputation method based on an iterative imputer was used, employing a Bayesian Ridge model. All statistical analyses were conducted using R Statistical Software (http://www.R-project.org, The R Foundation) and the Free Statistics Analysis Platform (Beijing, China) (17).
A total of 787 patients were included in this study, with an average age of 73.1 ± 13.6 years, and 419 (53.2%) being male. Among all participants, the average TyG index value was 8.9 ± 0.7. The rates of hospital mortality and ICU mortality were 14.2% and 8.3%, respectively.
Table 1 presents the baseline characteristics of critically ill patients with CHF and AF categorized by TyG index quartiles. Participants were distributed into four groups based on TyG index levels at admission (Quartiles: Q1, 7.213–8.456; Q2, 8.457–8.835; Q3, 8.841–9.300; and Q4, 9.301–13.493). The mean TyG index values for each quartile were 8.2 ± 0.2, 8.6 ± 0.1, 9.1 ± 0.1, and 9.9 ± 0.6, respectively. Patients in the highest TyG index quartile exhibited a higher prevalence of diabetes and CKD, elevated severity of illness scores at admission, increased WBC levels, TC, urea nitrogen, serum creatinine, and anion gap, as well as lower levels of HDL-C. Additionally, this group showed longer hospital and ICU stays, along with higher rates of hospital and ICU mortality.
Table 2 illustrates the differences in baseline characteristics between survivors and non-survivors during the hospital stay. Factors associated with hospital mortality (P < 0.05) included gender, age, WBCs, hemoglobin, LDL-C, urea nitrogen, serum creatinine, potassium, and calcium. The TyG index levels in the non-survivor group were significantly higher than those in the survivor group (9.2 ± 0.8 vs. 8.9 ± 0.7, P < 0.001).
The Kaplan–Meier survival analysis curves were employed to evaluate the incidence of primary outcomes among different groups, stratified by the quartiles of the TyG index. Figure 2 graphically represents the results. Patients with a higher TyG index exhibited an elevated risk of hospital and ICU mortality. However, no significant difference was observed during the short-term follow-ups of 28 days and 3 months (log-rank P = 0.085, 0.26, respectively).
Figure 2. Kaplan–Meier survival analysis curves for all-cause mortality. Kaplan–Meier curves showing cumulative probability of all-cause mortality according to groups at 28 days (a), and 3 months (b).
Table 3 outlines the results of the multivariable logistic regression analysis investigating the association between the TyG index and hospital mortality. The results indicated that the TyG index was a significant risk factor in the unadjusted model [OR, 1.54 (95% CI: 1.2–1.98) P = 0.001], partly adjusted model [OR, 1.75 (1.33–2.29) P < 0.001], and fully adjusted model [OR, 1.59 (1.15–2.19) P = 0.005] when considering the TyG index as a continuous variable. When the TyG index was treated as a nominal variable, patients in the higher quartile were significantly associated with a higher risk of hospital death in the three established multiple logistic regression models—unadjusted [OR, 2.53 (1.37–4.66) P = 0.003], partly adjusted [OR, 3.18 (1.68–5.99) P < 0.001], and fully adjusted models [OR, 2.67 (1.3–5.5) P = 0.008]—compared to subjects in the lowest quartile. This trend was consistent with an increase in the TyG index. Similar findings were observed in the multiple logistic regression analysis of the TyG index and ICU mortality.
The risk of hospital mortality and ICU mortality increased linearly with the growth of the TyG index (P for non-linearity = 0.107 and P for non-linearity = 0.165, respectively) (Figure 3).
Figure 3. Restricted cubic spline curve for the TyG index and all-cause mortality. (a) Restricted cubic spline for hospital mortality. (b) Restricted cubic spline for ICU mortality. OR, odds ratio; ICU, intensive care unit; TyG, triglyceride-glucose.
To further substantiate the relationship between the TyG index and hospital mortality, ICU mortality, stratified analyses were conducted based on age, gender, hypertension, diabetes, and CKD (see Figures 4, 5). The TyG index exhibited a significant association with a higher risk of hospital mortality in CHF and AF patient subgroups, including females [OR (95% CI) 2.02 (1.23–3.29)], those aged 65 years or older [OR (95% CI) 1.78 (1.22–2.59)], those without hypertension [OR (95% CI) 2.04 (1.28–3.26)], those without diabetes [OR (95% CI) 1.92 (1.28–2.88)], and those with CKD [OR (95% CI) 2.2 (1.07–4.51)]. Similarly, concerning stratified analyses of ICU mortality, the TyG index demonstrated a significant association with a higher risk of ICU mortality in subgroups, including females [OR (95% CI) 2.11 (1.16–3.82)], those aged <65 years [OR (95% CI) 2.45 (1.01–5.94)], those aged 65 years or older [OR (95% CI) 1.65 (1.01–2.67)], those without hypertension [OR (95% CI) 2.51 (1.49–4.23)], those with diabetes [OR (95% CI) 2.07 (1.01–4.25)], those without diabetes [OR (95% CI) 1.82 (1.1–3.01)], and those with CKD [OR (95% CI) 3.03 (1.34–6.88)]. No interactions were observed between the TyG index and age, gender, hypertension, diabetes, and CKD in subgroup analyses (all P values for interaction >0.05).
Figure 4. Fores plots of odds ratios for the hospital mortality in different subgroups. OR, odds ratio; CI, confidence interval; CKD, chronic kidney disease.
Figure 5. Fores plots of odds ratios for the ICU mortality in different subgroups. OR, odds ratio; CI, confidence interval; CKD, chronic kidney disease.
To the best of our knowledge, this study is the first to explore the relationship between the TyG index and all-cause mortality in patients with CHF and AF. Our findings revealed a significant association between an elevated TyG index and increased all-cause ICU and hospital mortality among patients with CHF and AF. Importantly, this association remained robust even after adjusting for multiple clinical and laboratory variables, suggesting that the TyG index could be a valuable decision-making tool for clinicians managing patients with CHF and AF.
In recent years, the TyG index has emerged as a potential indicator of metabolic disorders, type 2 diabetes mellitus, atherosclerotic disease, cerebrovascular disease, and cardiovascular disease (CVD) (12–14, 18, 19). Numerous clinical studies have explored the link between an elevated TyG index and higher morbidity and mortality in the general population or other patient cohorts (20, 21). According to Liu et al., an increased TyG index is associated with coronary artery disease, myocardial infarction, and CVD in the general population (22). Zheng's research indicated that a high cumulative TyG index is linked to a higher risk of HF (23). Yang et al. reported that the TyG index may serve as a predictive marker for adverse cardiovascular outcomes in patients with chronic coronary syndrome (24). Multiple meta-analyses suggest that the TyG index is associated with various coronary artery diseases (25–27). Chen et al. observed that an elevated TyG index is an independent risk factor for AF among non-diabetic hospitalized patients (28). And Alireza Azarboo et al. found in their research that the TyG index is an easy-to-measure surrogate marker of IR in patients with AF (29). Another study involving 1,226 patients suggested that an elevated TyG index was associated with an increased risk of stroke recurrence and death (30). Similar results are also observed in the studies of Yang et al. (31). A meta-analysis has identified a significant association between TyG levels and Peripheral artery disease (PAD) and its severity (32). Collectively, these studies underscore the potential of the TyG index in predicting clinical outcomes related to cerebrovascular diseases and CVDs.
Our findings suggest a significant association between a high TyG index and all-cause mortality in patients with CHF and AF. Upon considering and adjusting for various covariates, a stronger correlation is observed. However, the precise biological mechanism underlying the relationship between the TyG index, CHF, and AF remains uncertain. A potential mechanism could be associated with IR. Hyperinsulinemic-euglycemic Clamp is the gold standard for diagnosing insulin resistance (IR), but due to its limitations, it is difficult to apply in large-scale clinical studies. HOMA-IR is the most widely used surrogate marker; however, its calculation requires the measurement of fasting insulin concentrations (33). The TyG index serves as a simple and widely used method for determining IR (11). Previous studies have demonstrated that compared to the hyperinsulinemic-euglycemic Clamp technique, the TyG index exhibits good sensitivity and specificity in diagnosing IR (33). Extensive research has demonstrated the intimate association of IR with endothelial dysfunction, oxidative stress, immune deregulation, coagulation imbalance, and inflammatory responses (34–36). The state of IR can lead to a significant accumulation of fatty acids and TGs within cardiomyocytes, giving rise to “cardiac lipotoxicity.” This condition has the potential to trigger cellular dysfunction, cardiomyocyte apoptosis, and hinder myocardial metabolism, ultimately altering the function and structure of cardiomyocytes and elevating the risk of HF and arrhythmias (37–39). Our research revealed that the different TyG index quartiles reflect consistent variations of inflammatory and metabolic markers, such as WBC, hemoglobin, and creatinine. Consequently, we hypothesize that these parameters may also drive the results, albeit the underlying mechanisms are yet to be elucidated and require further exploration through fundamental research.
In sensitivity analysis, the consistent direction of all results indicates the stability and reliability of the core outcomes. Furthermore, our study found that the linear relationship between the TyG index and all-cause mortality in patients with CHF and AF remained steady in those without diabetes and non-hypertensive individuals. This result might be attributed to the higher likelihood of patients with these comorbidities adhering to appropriate treatment regimens and adopting healthier lifestyle habits (40). Additionally, our study revealed that the association between the TyG index and all-cause mortality seemed to be more pronounced in patients with CKD. CKD is a frequent comorbidity in HF, and worsening renal function often accompanies HF decompensation. Similarly, there is a bidirectional relationship between AF and CKD (41, 42). Patients with HF and AF usually require anticoagulation therapy, but severe renal dysfunction limits the use of such therapy (41). To some extent, this limitation may contribute to an increase in all-cause mortality. In summary, the TyG index emerges as a significant predictor of all-cause mortality in patients with CHF and AF.
However, our study comes with several limitations. Firstly, the results obtained may not be entirely representative as the enrolled patients were from an American population. The inherent limitations of public databases introduce the possibility of unmeasured variables, such as genetic factors, dietary patterns, and psychosocial factors. Secondly, our analysis only considered the baseline TyG index. To comprehensively understand its prognostic significance, observing the dynamics of the TyG index over time in patients with CHF and AF is imperative. This longitudinal approach would offer a more complete understanding of the index's predictive value. Thirdly, our study employed a retrospective analysis from an observational study, lacking direct evidence to firmly establish a causal relationship. Nevertheless, we applied a comprehensive array of rigorous statistical methods to ensure the robustness and credibility of our findings. Fourthly, as this study is a retrospective analysis, it is not feasible to differentiate patients with paroxysmal, persistent, or permanent AF, or those with or without AF upon admission. Additionally, the same limitation extends to differentiating HF with reduced EF (HFrEF), HF with mid-range EF (HFmrEF), and HF with preserved EF (HFpEF) among patients diagnosed with CHF. In conclusion, while our study demonstrated the prognostic significance of the TyG index in patients with CHF and AF, its practical clinical utility requires validation through larger-scale prospective studies.
In summary, our results suggest a strong association between an elevated TyG index and increased hospital mortality and ICU mortality in patients with CHF and AF. Monitoring the TyG index holds the potential to inform clinical decision-making and enhance disease management in clinical practice. However, further prospective studies are necessary to validate these findings.
The original contributions presented in the study are included in the article/Supplementary Material, further inquiries can be directed to the corresponding author.
Ethical approval was not required for the study involving humans in accordance with the local legislation and institutional requirements. Written informed consent to participate in this study was not required from the participants or the participants' legal guardians/next of kin in accordance with the national legislation and the institutional requirements.
FK: Data curation, Formal Analysis, Funding acquisition, Methodology, Writing – original draft, Writing – review & editing. ZY: Formal Analysis, Writing – original draft, Writing – review & editing. DB: Formal Analysis, Supervision, Visualization, Writing – review & editing. MT: Formal Analysis, Supervision, Visualization, Writing – review & editing. NJ: Conceptualization, Writing – review & editing.
The author(s) declare that financial support was received for the research and/or publication of this article. The research was funded by Jinhua Science and Technology Plan Project (2020-4-152), Yiwu Science and Technology Plan Project (19-3-132).
We thank Free Statistics team for providing technical assistance and valuable tools for data analysis and visualization. We also thank Dr Jie Liu of the Department of Vascular and Endovascular Surgery, Chinese people's liberation army general hospital for comments regarding the manuscript.
The authors declare that the research was conducted in the absence of any commercial or financial relationships that could be construed as a potential conflict of interest.
The author(s) declare that no Generative AI was used in the creation of this manuscript.
All claims expressed in this article are solely those of the authors and do not necessarily represent those of their affiliated organizations, or those of the publisher, the editors and the reviewers. Any product that may be evaluated in this article, or claim that may be made by its manufacturer, is not guaranteed or endorsed by the publisher.
1. Franca EL, Manno G, Ajello L, Gesaro GD, Minà C, Visconti C, et al. Physiopathology and diagnosis of congestive heart failure: consolidated certainties and new perspectives. Curr Probl Cardiol. (2020) 46(3):100691. doi: 10.1016/j.cpcardiol.2020.100691
2. Povsic TJ. Emerging therapies for congestive heart failure. Clin Pharmacol Ther. (2018) 103(1):77–87. doi: 10.1002/cpt.913
3. Atherton JJ, Hayward CS, Wan Ahmad WA, Kwok B, Jorge J, Hernandez AF, et al. Patient characteristics from a regional multicenter database of acute decompensated heart failure in Asia Pacific (ADHERE International-Asia Pacific). Card Fail. (2012) 18(1):82–8. doi: 10.1016/j.cardfail.2011.09.003
4. Chioncel O, Mebazaa A, Harjola V, Coats AJ, Piepoli MF, Crespo-Leiro MG, et al. Clinical phenotypes and outcome of patients hospitalized for acute heart failure: the ESC heart failure long-term registry. Eur J Heart Fail. (2017) 19(10):1242–54. doi: 10.1002/ejhf.890
5. Yaku H, Ozasa N, Morimoto T. Demographics, management, and in-hospital outcome of hospitalized acute heart failure syndrome patients in contemporary real clinical practice in Japan- observations from the prospective, multicenter Kyoto congestive heart failure (KCHF) registry. Circ J. (2018) 82 (11):2811–9. doi: 10.1253/circj.CJ-17-1386
6. Newman JD, O’Meara E, Bohm M, Savarese G, Kelly PR, Vardeny O, et al. Implications of atrial fibrillation for guideline-directed therapy in patients with heart failure: JACC state-of-the-art review. J Am Coll Cardiol. (2024) 83(9):932–50. doi: 10.1016/j.jacc.2023.12.033
7. Seferovic PM, Ponikowski P, Anker SD, Bauersachs J, Chioncel O, Cleland JGF, et al. Clinical practice update on heart failure 2019: pharmacotherapy, procedures, devices and patient management. An expert consensus meeting report of the heart failure association of the European Society of Cardiology. Eur J Heart Fail. (2019) 21(10):1169–86. doi: 10.1002/ejhf.1531
8. Cotter G, Metra M, Milo-Cotter O, Dittrich HC, Gheorghiade M. Fluid overload in acute heart failure–re-distribution and other mechanisms beyond fluid accumulation. Eur J Heart Fail. (2008) 10(2):165–9. doi: 10.1016/j.ejheart.2008.01.007
9. Khalaji A, Behnoush AH, Khanmohammadi S, Ghanbari Mardasi K, Sharifkashani S, Sahebkar A, et al. Triglyceride-glucose index and heart failure: a systematic review and meta-analysis. Cardiovasc Diabetol. (2023) 22(1):244. doi: 10.1186/s12933-023-01973-7
10. Hill MA, Yang Y, Zhang L, Sun Z, Jia G, Parrish AR, et al. Insulin resistance, cardiovascular stiffening and cardiovascular disease. Metab Clin Exp. (2021) 119:154766. doi: 10.1016/j.metabol.2021.154766
11. Tahapary DL, Pratisthita LB, Fitri NA, Marcella C, Wafa S, Kurniawan F, et al. Challenges in the diagnosis of insulin resistance: focusing on the role of HOMA-IR and tryglyceride/glucose index. Diabetes Metab Syndr. (2022) 16(8):102581. doi: 10.1016/j.dsx.2022.102581
12. Zhao Q, Cheng YJ, Xu YK, Zhao ZW, Liu C, Sun TN, et al. Comparison of various insulin resistance surrogates on prognostic prediction and stratification following percutaneous coronary intervention in patients with and without type 2 diabetes mellitus. Cardiovasc Diabetol. (2021) 20(1):190. doi: 10.1186/s12933-021-01383-7
13. Zhang R, Shi S, Chen W, Wang Y, Lin X, Zhao Y, et al. Independent effects of the triglyceride-glucose index on all-cause mortality in critically ill patients with coronary heart disease: analysis of the MIMIC-III database. Cardiovasc Diabetol. (2023) 22(1):10. doi: 10.1186/s12933-023-01737-3
14. Ma X, Han Y, Jiang L, Li M. Triglyceride-glucose index and the prognosis of patients with acute ischemic stroke: a meta-analysis. Horm Metab Res. (2022) 54(6):361–70. doi: 10.1055/a-1853-9889
15. Johnson AEW, Bulgarelli L, Shen L, Gayles A, Shammout A, Horng S, et al. MIMIC-IV, a freely accessible electronic health record dataset. Sci Data. (2023) 10(1):1. doi: 10.1038/s41597-022-01899-x
16. Cheng L, Zhang F, Xue W, Yu P, Wang X, Wang H, et al. Association of dynamic change of triglyceride-glucose index during hospital stay with all-cause mortality in critically ill patients: a retrospective cohort study from MIMIC IV2.0. Cardiovasc Diabetol. (2023) 22(1):142. doi: 10.1186/s12933-023-01874-9
17. Fu Z, Zhang X, Zhao X, Wang Q. U-shaped relationship of sodium-to-chloride ratio on admission and mortality in elderly patients with heart failure: a retrospective cohort study. Curr Probl Cardiol. (2023) 48(1):101419. doi: 10.1016/j.cpcardiol.2022.101419
18. Cai W, Xu J, Wu X, Chen Z, Zeng L, Song X, et al. Association between triglyceride-glucose index and all-cause mortality in critically ill patients with ischemic stroke: analysis of the MIMIC-IV database. Cardiovasc Diabetol. (2023) 22(1):138. doi: 10.1186/s12933-023-01864-x
19. Liu D, Yang K, Gu H, Li Z, Wang Y, Wang Y. Predictive effect of triglyceride-glucose index on clinical events in patients with acute ischemic stroke and type 2 diabetes mellitus. Cardiovasc Diabetol. (2022) 21(1):280. doi: 10.1186/s12933-022-01704-4
20. Liu XC, He GD, Lo K, Huang YQ, Feng YQ. The triglyceride-glucose index, an insulin resistance marker, was non-linear associated with all-cause and cardiovascular mortality in the general population. Front Cardiovasc Med. (2021) 7:628109. doi: 10.3389/fcvm.2020.628109
21. Zhou D, Liu XC, Kenneth L, Huang YQ, Feng YQ. A non-linear association of triglyceride glycemic index with cardiovascular and all-cause mortality among patients with hypertension. Front Cardiovasc Med. (2021) 8:778038. doi: 10.3389/fcvm.2021.778038
22. Liu X, Tan Z, Huang Y, Zhao H, Liu M, Yu P, et al. Relationship between the triglyceride-glucose index and risk of cardiovascular diseases and mortality in the general population: a systematic review and meta-analysis. Cardiovasc Diabetol. (2022) 21(1):124. doi: 10.1186/s12933-022-01546-0
23. Zheng H, Chen G, Wu K, Wu W, Huang Z, Wang X, et al. Relationship between cumulative exposure to triglyceride-glucose index and heart failure: a prospective cohort study. Cardiovasc Diabetol. (2023) 22(1):239. doi: 10.1186/s12933-023-01967-5
24. Erdoğan A, İnan D, Genç Ö, Yıldız U, Demirtola Aİ, Çetin İ, et al. The triglyceride-glucose index might be a better indicator for predicting poor cardiovascular outcomes in chronic coronary syndrome. J Clin Med. (2023) 12(19):6201. doi: 10.3390/jcm12196201
25. Liu F, Ling Q, Xie S, Xu Y, Liu M, Hu Q, et al. Association between triglyceride glucose index and arterial stiffness and coronary artery calcification: a systematic review and exposure-effect meta-analysis. Cardiovasc Diabetol. (2023) 22(1):111. doi: 10.1186/s12933-023-01819-2
26. Liu H, Wang L, Zhou X, Wang H, Hao X, Du Z, et al. Triglyceride-glucose index correlates with the occurrence and prognosis of acute myocardial infarction complicated by cardiogenic shock: data from two large cohorts. Cardiovasc Diabetol. (2024) 23(1):337. doi: 10.1186/s12933-024-02423-8
27. Liang S, Wang C, Zhang J, Liu Z, Bai Y, Chen Z, et al. Triglyceride-glucose index and coronary artery disease: a systematic review and meta-analysis of risk, severity, and prognosis. Cardiovasc Diabetol. (2023) 22(1):170. doi: 10.1186/s12933-023-01906-4
28. Chen S, Mei Q, Guo L, Yang X, Luo W, Qu X, et al. Association between triglyceride-glucose index and atrial fibrillation: a retrospective observational study. Front Endocrinol (Lausanne). (2022) 13:1047927. doi: 10.3389/fendo.2022.1047927
29. Azarboo A, Behnoush AH, Vaziri Z, Daneshvar MS, Taghvaei A, Jalali A, et al. Assessing the association between triglyceride-glucose index and atrial fibrillation: a systematic review and meta-analysis. Eur J Med Res. (2024) 29(1):118. doi: 10.1186/s40001-024-01716-8
30. Yang X, Wang G, Jing J, Wang A, Zhang X, Jia Q, et al. Association of triglyceride-glucose index and stroke recurrence among nondiabetic patients with acute ischemic stroke. BMC Neurol. (2022) 22(1):79. doi: 10.1186/s12883-022-02588-3
31. Yang Y, Huang X, Wang Y, Leng L, Xu J, Feng L, et al. The impact of triglyceride-glucose index on ischemic stroke: a systematic review and meta-analysis. Cardiovasc Diabetol. (2023) 22(1):2. doi: 10.1186/s12933-022-01732-0
32. Samavarchitehrani A, Cannavo A, Behnoush AH, Kazemi Abadi A, Shokri Varniab Z, Khalaji A. Investigating the association between the triglyceride-glucose index and peripheral artery disease: a systematic review and meta-analysis. Nutr Diabetes. (2024) 14(1):80. doi: 10.1038/s41387-024-00341-y
33. Hou XZ, Lv YF, Li YS, Wu Q, Lv QY, Yang YT, et al. Association between different insulin resistance surrogates and all-cause mortality in patients with coronary heart disease and hypertension: NHANES longitudinal cohort study. Cardiovasc Diabetol. (2024) 23(1):86. doi: 10.1186/s12933-024-02173-7
34. Ormazabal V, Nair S, Elfeky O, Aguayo C, Salomon C, Zuñiga FA. Association between insulin resistance and the development of cardiovascular disease. Cardiovasc Diabetol. (2018) 17(1):122. doi: 10.1186/s12933-018-0762-4
35. Markus M, Rospleszcz S, Ittermann T, Baumeister SE, Schipf S, Siewert-Markus U, et al. Glucose and insulin levels are associated with arterial stiffness and concentric remodeling of the heart. Cardiovasc Diabetol. (2019) 18(1):145. doi: 10.1186/s12933-019-0948-4
36. Laakso M, Kuusisto J. Insulin resistance and hyperglycaemia in cardiovascular disease development. Nat Rev Endocrinol. (2014) 10(5):293–302. doi: 10.1038/nrendo.2014.29
37. Toit EFD, Donner DG. Myocardial insulin resistance: an overview of its causes, effects, and potential therapy. In: Arora S, editor. Insulin Resistance. Rijeka: InTech (2012). p. 189–226. doi: 10.5772/50619
38. Alpert MA, Karthikeyan K, Abdullah O, Ghadban R. Obesity and cardiac remodeling in adults: mechanisms and clinical implications. Prog Cardiovasc Dis. (2018) 61(2):114–23. doi: 10.1016/j.pcad.2018.07.012
39. Wende AR, Symons JD, Abel ED. Mechanisms of lipotoxicity in the cardiovascular system. Curr Hypertens Rep. (2012) 14(6):517–31. doi: 10.1007/s11906-012-0307-2
40. Sánchez-Íñigo L, Navarro-González D, Fernández-Montero A, Pastrana-Delgado J, Martínez JA. The TyG index may predict the development of cardiovascular events. Eur J Clin Invest. (2016) 46(2):189–97. doi: 10.1111/eci.12583
41. Farmakis D, Chrysohoou C, Giamouzis G, Giannakoulas G, Hamilos M, Naka K, et al. The management of atrial fibrillation in heart failure: an expert panel consensus. Heart Fail Rev. (2021) 26(6):1345–58. doi: 10.1007/s10741-020-09978-0
Keywords: triglyceride-glucose index, all-cause mortality, congestive heart failure, atrial fibrillation, MIMIC-IV database
Citation: Kan F, Yang Z, Bao D, Tang M and Ji N (2025) Association between triglyceride-glucose index and all-cause mortality in patients with congestive heart failure and atrial fibrillation. Front. Cardiovasc. Med. 12:1476815. doi: 10.3389/fcvm.2025.1476815
Received: 6 August 2024; Accepted: 18 March 2025;
Published: 3 April 2025.
Edited by:
Francesco Gentile, Sant’Anna School of Advanced Studies, ItalyReviewed by:
Mario Matta, AOU Città della Salute e della Scienza, ItalyCopyright: © 2025 Kan, Yang, Bao, Tang and Ji. This is an open-access article distributed under the terms of the Creative Commons Attribution License (CC BY). The use, distribution or reproduction in other forums is permitted, provided the original author(s) and the copyright owner(s) are credited and that the original publication in this journal is cited, in accordance with accepted academic practice. No use, distribution or reproduction is permitted which does not comply with these terms.
*Correspondence: Ningning Ji, eWFuaG9uZzkzMEBTaW5hLmNvbQ==
†These authors have contributed equally to this work
Disclaimer: All claims expressed in this article are solely those of the authors and do not necessarily represent those of their affiliated organizations, or those of the publisher, the editors and the reviewers. Any product that may be evaluated in this article or claim that may be made by its manufacturer is not guaranteed or endorsed by the publisher.
Research integrity at Frontiers
Learn more about the work of our research integrity team to safeguard the quality of each article we publish.