- 1Department of Cardiology, The Fourth Affiliated Hospital of School of Medicine, and International School of Medicine, International Institutes of Medicine, Zhejiang University, Yiwu, China
- 2Liangzhu Laboratory, Zhejiang University Medical Center, Hangzhou, China
- 3Department of Gynecological Oncology, Fudan University Shanghai Cancer Center, Shanghai, China
Diabetic cardiomyopathy (DCM) is one of the most prevalent and severe complications associated with diabetes mellitus (DM). The onset of DCM is insidious, with the symptoms being obvious only in the late stage. Consequently, the early diagnosis of DCM is a formidable challenge which significantly influences the treatment and prognosis of DCM. Thus, it becomes imperative to uncover innovative approaches to facilitate the prompt identification and diagnosis of DCM. On the traditional clinical side, we tend to use serum biomarkers as well as imaging as the most common means of diagnosing diseases because of their convenience as well as affordability. As we delve deeper into the mechanisms of DCM, a wide variety of biomarkers are becoming competitive diagnostic indicators. Meanwhile, the application of multiple imaging techniques has also made efforts to promote the diagnosis of DCM. Besides, the spurt in sequencing technology has made it possible to give hints about disease diagnosis from the genome as well as the transcriptome, making diagnosis less difficult, more sensitive, and more predictive. Overall, sequencing technology is expected to be the superior choice of plasma biomarkers for detecting lesions at an earlier stage than imaging, and its judicious utilization combined with imaging technologies will lead to a more sensitive diagnosis of DCM in the future. Therefore, this review meticulously consolidates the progress and utilization of various biomarkers, imaging methods, and sequencing technologies in the realm of DCM diagnosis, with the aim of furnishing novel theoretical foundation and guide future research endeavors towards enhancing the diagnostic and therapeutic landscape of DCM.
1 Introduction
Diabetes mellitus (DM) is a common metabolic disease featuring organ dysfunction caused directly or indirectly by hyperglycemia. It ranks among the most prevalent and rapidly increasing chronic diseases globally (1). It was estimated that nearly 10.5% adults, ranging from 20–79 years, were suffering from managing DM worldwide, with type 1 diabetes mellitus (T1DM) and type 2 diabetes mellitus (T2DM) constituting the major types, and T2DM accounting for 90% of cases (2–4). According to the fluctuations in disease prevalence, it has been calculated that without intervention, the number of people with DM will reach 783 million globally by 2045, representing 12.2% of the population (5). At the same time, the prevalence of T2DM, the mainstay of DM, is set to reach 700 million (2). The escalation imposes a heavy healthcare expenses burden on global healthcare system. DM patients are vulnerable to a spectrum of complications, including diabetic retinopathy, diabetic nephropathy, diabetic cardiomyopathy (DCM), and neurological issues (1, 6). Importantly, the prevalence of heart failure in DM patients is four-times higher than in the general populations, severely affecting their quality of life (7).
DCM stands out as a primary cause of heart failure in diabetic patients, specifically defined as cardiomyopathy developing in the absence of traditional cardiovascular risk factors such as coronary artery disease, valvular disease, or hypertension (8). Research categorizes DCM into two distinct phenotypes based on the ejection fraction (EF): the restrictive phenotype with preserved EF and the dilated phenotype with reduced EF (9). Initial symptoms of DCM often present as subclinical myocardial fibrosis and cardiac remodeling, primarily manifesting as early diastolic dysfunction (10). Moreover, recent hypotheses propose that early-stage DCM is characterized by a phase of myocardial hypercontractility triggered by metabolic imbalances, which progresses into a subclinical phase without overt symptoms (11). As the condition worsens, patients may develop symptoms like fatigue, palpitations, exertional dyspnea, arrhythmias, and angina pectoris, ultimately culminating in left ventricular (LV) failure and potentially life-threatening heart failure, alongside other diabetes-related complications (12). Although conventional imaging techniques like echocardiography can detect diastolic dysfunction in 40%–75% of T1DM or T2DM (13, 14), the insidious onset and subtle early symptoms of DCM can lead to missed early diagnosis or treatment. The current gold standard for diagnosing DCM is endomyocardial biopsy (EMB), which enables the detection of specific histological and ultrastructural changes in early-stage DCM. Histological examinations often reveal increased collagen volume, sparse coronary microvasculature, and advanced glycation end-product accumulation in the coronary microvasculature (9). However, due to its invasiveness and risks, EMB is predominantly confined to laboratory researches. Despite growing scholarly interest in DCM, existing guidelines lack well-defined and concrete diagnostic criteria for early stage of DCM.
Numerous studies have proposed potential diagnostic strategies for DCM, focusing on biomarkers, imaging techniques, and sequencing technologies. As for biomarkers, research mainly revolves around inflammatory mediators, fibrosis markers, antioxidant markers, cardiac hypertrophy markers and myocardial injury markers, which play essential roles in the pathogenesis of DCM (15). Researchers have mainly employed various imaging techniques to evaluate early cardiac changes in DCM, such as myocardial fibrosis, myocardial hypertrophy, and structural alterations in the diabetic myocardium. These imaging methods offer significant advantages over the invasive EMB in terms of non-invasiveness, reliability and repeatability (16, 17). Additionally, advancements in sequencing technologies showed great promise for the early diagnosis of DCM. Some studies have even leveraged sequencing technology to investigate the genetic, transcriptional and epigenetic underpinnings of DCM, opening new avenues for the diagnosis of DCM (18–20). This review consolidates the research progress on biomarkers, imaging methods, and sequencing technologies for DCM diagnosis, aiming to provide a comprehensive reference for the development of accurate and efficient diagnostic strategies and models.
2 Potential biomarkers for diabetic cardiomyopathy
2.1 Mediators of inflammation
Persistent hyperglycemia stimulates inflammatory pathways, which are core mechanisms mediating myocardial dysfunction in DCM (21). It has been reported that cytokine and chemokine release induced by high blood glucose level would result in a common signaling pathway, known as nuclear factor kappa B (NF-κB) (22). Tumor necrosis factor alpha (TNF-α), interleukin (IL)-6, IL-1β and C-reactive protein (CRP) are all notable components of the downstream mediators, Figure 1 and Table 1. Elevated TNF-α levels have been observed in DCM patients (23, 24), which was particularly associated with LV diastolic dysfunction (25). IL-1β and IL-6 are also significantly elevated in both DCM animal models (27) and DCM patients (23) as compared to DM individuals, Figure 1 and Table 1. IL-6, in particular, has been significantly correlated with DCM incidence in long-term clinical studies (28, 29). Simultaneously, while CRP exacerbates LV dysfunction in DCM patients (31–36), its application as a diagnostic marker is hampered by its non-specificity and close association with infections or stressed states. Overall, inflammatory mediators are highly sensitive for the detection of DCM, especially for the assessment of DM populations at high pathogenic risk, but their specificity is susceptible to immune status.
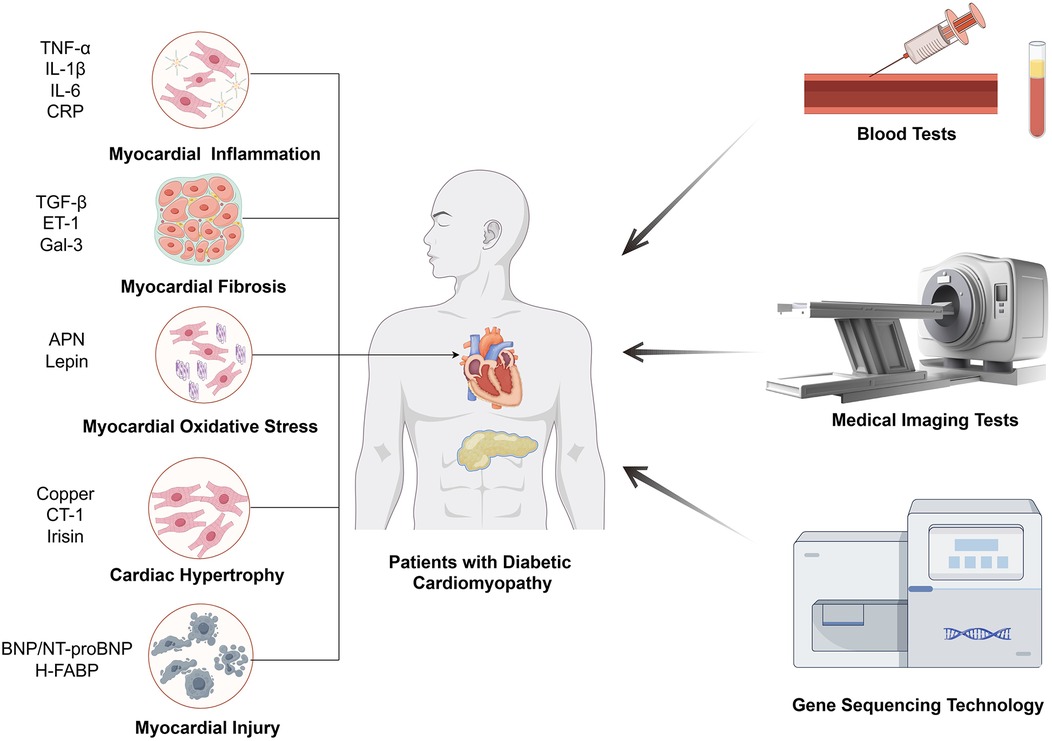
Figure 1. The main means of inspection for DCM. The biomarkers of DCM diagnosis through blood tests are related with five mechanisms, including myocardial inflammation, myocardial fibrosis, myocardial oxidative stress, cardiac hypertrophy, myocardial injury.
2.2 Fibrosis markers
Myocardial fibrosis is an essential stage in the process of progression of cardiomyocyte dysfunction, in which it is accompanied by the production of a number of specific factors, and the detection of changes in the levels of these factors can be applied as a basis for the diagnosis of myocardial fibrotic changes in DCM (63, 64). TGF-β is one of the most prominent profibrotic cytokines promoting extracellular matrix accumulation. Elevated plasma TGF-β levels have been observed in DCM patients, Figure 1 and Table 1 (23). And experiments have shown that high glucose could dysregulate TGF-β signaling through Smad3/Smad4 pathway in direct or indirect ways, which notably caused fibroblast activation and fibrosis in heart (64–66). In addition to TGF-β, there is a factor endothelin-1 (ET-1) that affects cardiomyocyte fibrosis independently of blood glucose. ET-1 is a profibrotic peptide produced by vascular endothelial cells in response to inflammation or oxidative stress. ET-1 could independently activate cardiac fibroblasts, promoting the development of pathologic myocardial fibrosis (43). Galectin-3 (Gal-3), a lectin family protein, is also linked to the fibrotic processes in cardiovascular diseases and is elevated in diabetic patients with mildly decreased EF and reduced global longitudinal strain (GLS), which was an early evidence of LV dysfunction for DCM, Figure 1 and Table 1 (44, 67, 68). Several studies have demonstrated that GLS was closely related to Gal-3, suggesting that Gal-3 combined with GLS served as predictors of early LV dysfunction in DCM (44, 45). In other diseases, Gal-3 expression was regulated by epigenetic mechanisms, and we look forward to further studies on the potential relationship between Gal-3 methylation levels and DCM (69, 70). Generally speaking, fibrosis markers are supposed to be combined with imaging techniques to obtain better sensitivity and specificity for DCM diagnosis.
2.3 Antioxidant markers
Studies have shown that DCM leads to alterations in the cardiomyocyte metabolome and related gene expression, resulting in hyperglycemia, cardiac lipid accumulation as well as oxidative stress (71, 72). Other research also linked mitochondrial dysfunction and oxidative stress to DCM (73). In these studies, oxidative stress is a direct influence on cardiac function that is ultimately shared by different pathways. Adiponectin (APN) and leptin are common substances that can alter oxidative stress by affecting metabolism, however, the former is a protective factor and the latter is harmful (71, 74). APN is an insulin-sensitizing hormone with levels inversely correlated to LV hypertrophy, Figure 1 and Table 1 (69). Zhao et al. noted that lower APN levels are linked with increased cardiovascular disease incidence (70). Studies have found that low plasma APN levels in DCM patients cause oxidative damage in cardiomyocytes (71). Similarly, Shaver et al. found that serum APN levels in DCM patients were lower than those in DM patients and markedly much lower than in healthy controls (12), indicating that clinical APN levels may serve as a monitoring tool for DCM patients. In terms of leptin, it is an adipose-derived hormone proportional to body fat, correlates with adverse cardiovascular outcomes, Figure 1 and Table 1 (47, 75). Higher serum leptin levels have been observed in DCM compared to DM controls (23), and were positively correlated with interventricular septum thickness in vivo (48). The mechanism could be explained by mitochondrial production of reactive oxygen species (ROS), which is the downstream effector of high leptin levels. And the relationship between development of diabetic vascular injury and leptin has been firmly strengthened through the pathogenic mechanism of oxidative stress (49). To summarize the clinical value of antioxidant markers, they can sensitively predict the development of cardiovascular outcomes, which are essential for the early prediction and diagnosis of DCM.
2.4 Cardiac hypertrophy markers
Cardiac hypertrophy is a common pathological change in many cardiac diseases such as atherosclerosis, heart failure and likewise for DCM. From pathophysiological aspect, cardiac hypertrophy is the result of an imbalance in energy metabolism with disruption of glucose or ionic homeostasis, which is one of the typical features of DCM (76). It has been proved that copper deficiency can induce cardiac hypertrophy and aggravate cardiomyopathy in DCM patients (52). And the defective excretion and uptake of copper are markedly altered throughout the body, where concentration of copper is elevated in plasma and extracellular myocardial cells but decreased in cardiac myocytes (53). Therefore, in combination with circulating copper levels as well as imaging findings, it can become possible to make a presumption as to whether DM patients are predisposed to cardiac hypertrophic lesions. In addition to copper, cardiotrophin-1 (CT-1), a member of the glycoprotein 130 family, is also a potent inducer of cardiac hypertrophy, Figure 1 and Table 1 (74). Several studies have demonstrated that CT-1 is highly expressed in DCM and other chronic heart disease, presenting a significant positive correlation between CT-1 plasma levels and LV mass index, which indicates its critical role in DCM pathogenesis through its involvement in myocardial remodeling (47, 48, 75). As for protective factors against cardiac hypertrophy, irisin is an important member with additional roles in regulating glucose metabolism. It mainly exerted protective effects through integrin αV/β5-AKT signaling and AMPK/mTOR signal pathway in DCM patients, Figure 1 and Table 1 (72, 73). For its rapid response to cardiac hypertrophy, irisin sheds light on early detection of DCM and pharmacological treatments. Taken together, the results of many studies have shown that cardiac hypertrophy markers reflect excellent sensitivity and specificity for the diagnosis of DCM, especially for early risk detection.
2.5 Myocardial injury markers
DCM is tightly linked to myocardial injury as well as heart failure, for chronic neurohormonal dysregulation and endothelial dysfunction create a milieu that predisposes individuals to more serious cardiac disease (77). For various DCM-associated disorders of the cardiovascular system, natriuretic peptides are an important family of biomarkers, especially brain natriuretic peptide (BNP) and its inactive precursor N-terminal pro-brain natriuretic peptide (NT-proBNP). BNP is primarily synthesized and secreted by the ventricles of the heart in response to overload volume or pressure, and exerts efforts in different target cells (78). The rich set of features include vasodilation, anti-inflammatory effects, anti-fibrotic effects, and antihypertrophic effects, which are supposed to relieve myocardial damage (79). Therefore, it mechanistically makes sense for BNP and NT-proBNP to contribute to the diagnosis of DCM. Researchers found that the combination of conventional echocardiography with BNP and NT-proBNP can effectively detect diastolic dysfunction (57, 59), which is often prior to symptomatic DCM and generally accompanied by subtle LV hypertrophy, Figure 1 and Table 1. However, normal natriuretic peptide levels can also be observed in some patients with abnormal diastolic function (57, 59), which limits the definitive diagnosis based solely on BNP levels. Apart from BNP or NT-proBNP, heat-fatty acid binding protein (H-FABP), a cytosolic lipoprotein that is abundant in myocardial tissues, also exhibits high specificity for myocardial injury, which is released early in response to cardiomyocyte damage. And serum H-FABP levels were significantly higher in DM patients compared to healthy controls (61), suggesting its promising role as a biomarker in asymptomatic DCM patients, Figure 1 and Table 1. Moreover, Shearer et al. established that reductions in plasma H-FABP may contribute to the correction of insulin resistance and glucose uptake in the heart (80), which underscores the necessity for further research into the relationship between H-FABP and DCM severity to enhance our understanding of its diagnostic role in early-stage DCM patients. Overall, myocardial injury markers were assessed to have good clinical value for myocardial injury and heart failure due to DCM, but the sensitivity and specificity for the early diagnosis of DCM remain to be supported by more evidence.
3 Diagnostic imaging methods for DCM
3.1 Echocardiography
Conventional echocardiography (CE) can be used to assess intermediate-term DCM, which is mainly characterized by decreased E/A ratio, increased LV myocardial mass, decreased left ventricular diameter (LVD), and prolonged deceleration time, Figure 2 (81). Studies also showed that CE can identify abnormalities associated with LV hypertrophy and impaired diastolic filling present in early DCM (81, 82). In clinical practice, CE is non-invasive, painless, safe, affordable and available from clinics to general hospitals, which is widely regarded as the preferred method to evaluate DCM cardiac structures and function. And among all available imaging technologies, CE is the most cost-effective imaging modality, which leads to high frequency of CE utilization in patients with DCM. However, CE has several limitations, including operator subjectivity, angular correlation, and operator-induced noise. Additionally, the technique is susceptible to variations in patient heart rate and cardiac volumetric status. Furthermore, the pseudo-normal LV filling pattern observed in DM patients may also lead to underestimation of early DCM (83). Currently, there are several novel imaging techniques in echocardiography that are more helpful in workup of patients with DCM, however with higher expense. Tissue doppler imaging (TDI) serves as a valuable diagnostic imaging technique primarily utilized in the assessment of cardiovascular diseases. In patients with intermediate-term DCM, TDI is predominantly characterized by an E/E' ratio exceeding 15 (81). It enables the evaluation of myocardial velocities across various myocardial segments and effectively detects longitudinal, circumferential, and radial contractions of myocardial fibers, thereby quantifying the degree of ischemia and fibrosis. Di Bonito et al. discovered that 50% of diabetic patients exhibiting no cardiac dysfunction on CE examinations had an E/A ratio greater than 1 when assessed by TDI (84), which underscored the enhanced sensitivity of TDI compared to CE. However, the application of TDI is heavily influenced by angle, which makes it quite dependent on the technical competence of the professionals (85). Speckle tracking echocardiography (STE) is a relatively novel imaging modality for assessing myocardial tissue function. It overcomes limitations of traditional echocardiography by minimizing interference from factors such as examiner subjectivity, angle dependency and noise interference (86). STE provides progressive diastolic and systolic function assessment by tracking explicit speckle patterns, produced by interferences of ultrasound beams with the myocardial tissue. As a result, it facilitates the detection of myocardial deformation across three axes: radial, circumferential, and longitudinal strains (87). In the evaluation of systolic function in DCM patients, GLS is the most widely used parameter, indicating the degree of impairment. Reports showed that reduced GLS was hypersensitive to early cardiac lesions in DM and there was a correlation with the severity of symptoms (88–90). Additionally, another new STE marker, peak systolic longitudinal rotation, was also found to be diminished in early stages of DCM (91). Therefore, the new parameters, which have been gradually mined along with the development of STE, have injected new vigor into the early imaging diagnosis and assessing cardiac functional changes of DCM. Nevertheless, STE does face certain challenges, its accuracy is influenced by ventricular wall thinning and abnormal ventricular remodeling.
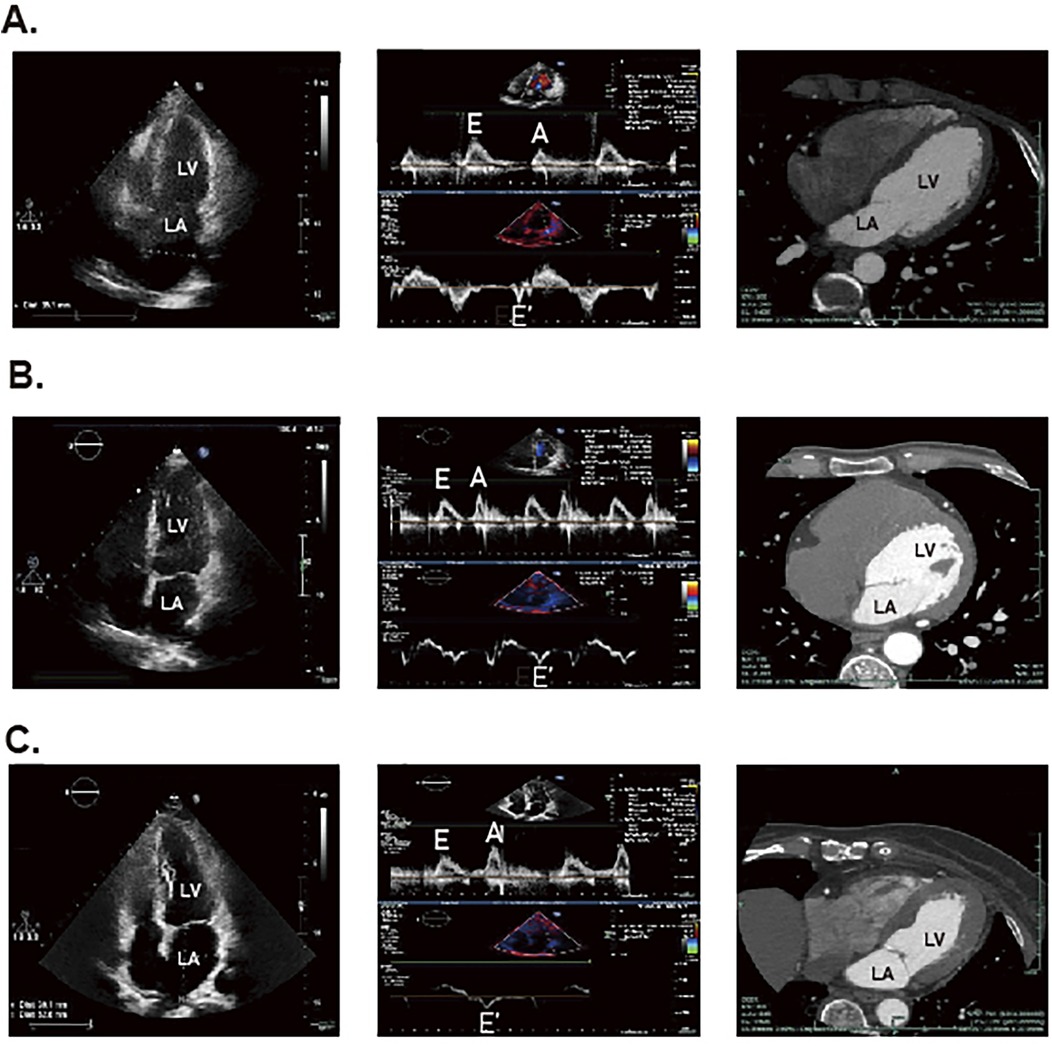
Figure 2. The STE and CT results for DCM patients, DM patients and healthy individuals. The panel (A) represents the result of healthy individuals. The panel (B) represents the result of patients with DM. The (C) panel represents the result of patients with DCM.
3.2 Cardiovascular magnetic resonance
The early manifestation observed via cardiovascular magnetic resonance (CMR) in DCM patients is characterized by altered cardiac metabolism (81). And CMR can detect early interstitial fibrotic changes in DCM, which could uniquely be detected by CMR-derived T1 mapping at present, whereas STE was only able to predict the risk of subsequent development of heart failure and thus detect further progression of fibrosis (92). Compared to CE, CMR provides superior spatial and temporal resolution for evaluating ventricular size, left ventricular ejection fraction (LVEF) and myocardial mass distribution (81). Furthermore, CMR provides information about myocardial ischemia and precise tissue characterization that could not be detected with echocardiography (93). DCM can also be classified into three primary stages based on CMR findings: the early stage, featuring slight LV hypertrophy that may be accompanied by diastolic insufficiency; the intermediate stage, exhibiting progressive cardiomyocyte hypertrophy, increased myocardial mass and thickening of the ventricular wall, which leads to diastolic dysfunction and mild systolic dysfunction (EF < 50%); and the late stage, characterized by further increases in LV size, wall thickness and mass, accompanied by evident diastolic and systolic dysfunction (94). Fischer et al. demonstrated that tagging MRI provides high-precision assessments of myocardial motion, facilitating the measurement of deformation during the cardiac cycle and enabling the monitoring of early DCM changes (95). However, the cost of CMR devices and the requirement for high image quality limit its application scenarios (93). In terms of patient costs, CMR does not have a dominant position among several imaging technologies, while its accessibility is only surpassed by CE. Besides, CMR has other limitations including lengthy duration of examination, potential claustrophobia for some patients, relatively poor sensitivity to diastolic dysfunction and incompatibility with certain pacemakers or implantable defibrillators.
3.3 Multi-slice computed tomography
The multi-slice computed tomography (MSCT) examination of advanced DCM predominantly reveals myocardial calcification associated with ischemia, Figure 2 (81). Notably, Schuijf JD et al. have demonstrated that MSCT can reliably assess LV dysfunction in T2DM patients (96). Additionally, it provides secure and reliable methods for patients who cannot undergo invasive coronary angiography or CMR, as well as for those with suspicious echocardiographic outcomes (97). For patients, although MSCT is second only to CE concerning examination expense, its diagnostic value is much lower than CMR as well as echocardiography. Nonetheless, most clinic guidelines firmly suggest high-risk patients with T2DM are supposed to undergo invasive coronary examination, which restricts the extensive application of MSCT in DCM (97). Moreover, MSCT has other notable limitations, including radiation exposure and the use of toxic contrast agents, which impede its position as the preferred diagnostic imaging modality. Also, the maintenance and site requirements of MSCT are more stringent than those of CE, making its application in DCM not as convenient as the latter. Therefore, further research is essential to optimize the use of contrast agents in MSCT and enhance its utility in clinical practice.
3.4 Nuclear imaging technology
Nuclear imaging is mainly applied to detect myocardial metabolism in the early stages of DCM and consists of two main techniques, gated single-photon emission computed tomography (G-SPECT) and positron emission tomography (PET) (81). Di Carli et al. have demonstrated that G-SPECT provides comprehensive data on ventricular function, myocardial wall thickness, exercise capacity, and diastolic parameters through three-dimensional imaging utilizing labeled myocardial perfusion agents (98). Given that the metabolism of tracers is influenced by metabolic alterations in disease states, G-SPECT presents considerable promise for the early detection of DCM (99). Similarly, PET is the most broadly applied radionuclide method to detect myocardial metabolism and to perform molecular imaging, due to its flexibility in radiotracer design and inherent quantitative capabilities (100, 101). It is rather helpful in patients who are obese or exhibit advanced LV dysfunction and coronary artery disease, conditions that may not be detected by G-SPECT imaging (102). Van den Brom et al. noted in early-stage DCM rat models that PET using 18F-2-fluoro-2-deoxy-D-glucose (18F-FDG) as a tracer revealed decreased myocardial glucose utilization and increased fatty acid oxidation (103). Overall, nuclear imaging technology offers specific advantages over CE with respect to resolution, reproducibility, and sensitivity. Nevertheless, the primary physical drawback of PET is low spatial resolution. However, it can be alleviated through technologies combination like PET/CT and PET/MR, which guarantee more precise localization of radiotracers (104). Other limitations include the cost of the radiotracer and the hazards of radiation to patients and technicians, and the requirements for equipment and space, making nuclear imaging technology still far from clinical diagnostic DCM.
4 Sequencing and its application in DCM diagnosis
Since the introduction of the dideoxy chain-termination method for DNA sequencing by Sanger et al. in 1976, sequencing technology has undergone remarkable advancements over the decades. Sanger sequencing translates nucleotide variations at each position into fluorescent signals by employing dideoxy-nucleoside triphosphates (ddNTPs) as chain terminators (105). Although, the efficiency of this method was subsequently enhanced through the use of polymerase chain reaction (PCR) and capillary electrophoresis, challenges related to sequence length, throughput and cost persisted.
To overcome these limitations, next-generation sequencing (NGS) was developed. Rather than sequencing entire DNA molecules, NGS subdivides the total sequence into 150–200 base pairs (bp) small fragments, which are amplified using specialized PCR techniques, such as bridge PCR or rolling circle amplification (RCA), to improve the accuracy of basecalling (106, 107). Subsequently, the fragments are aligned to reconstruct a comprehensive genetic sequence, Figure 3 (108, 109). NGS significantly enhances sequencing throughput while lowering the barrier to the application of sequencing technologies, making it one of the most utilized methodologies in contemporary research and clinical settings (110). The resolution of both DNA and RNA through NGS has greatly advanced the understanding of human disease genomes and transcriptomes. In addition, technologies built upon NGS, including single-cell sequencing, high-throughput chromosome conformation capture (Hi-C), and assay for transposase-accessible chromatin using sequencing (ATAC-Seq), are being employed across a variety of diseases (111–113).
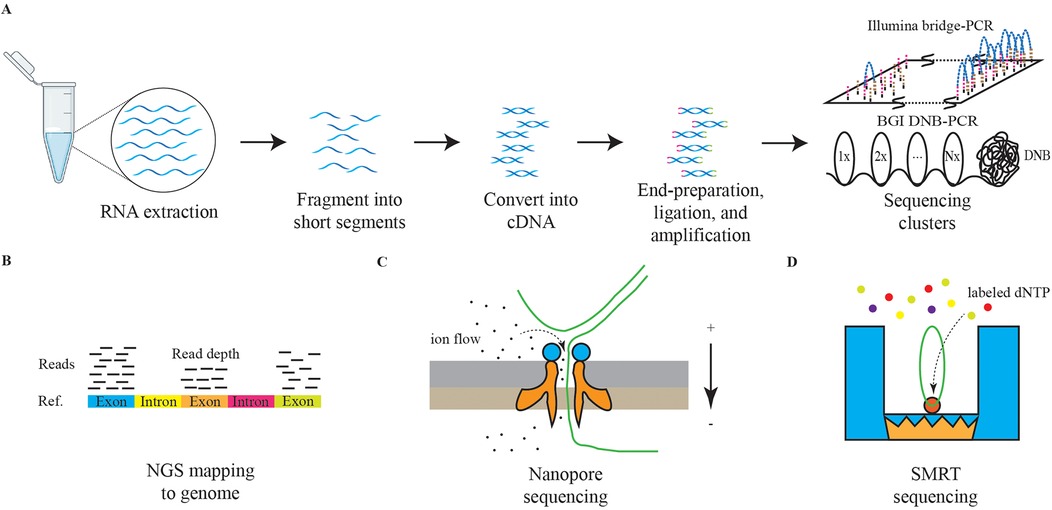
Figure 3. The schematic diagrams of NGS and TGS. The panel (A) represents the schematic diagram of NGS RNA sequencing. The graph (B) represents the schematic diagram of NGS reads mapping. The graph (C) represents the schematic diagram of nanopore sequencing. The graph (D) represents the schematic diagram of SMRT sequencing.
Currently, third-generation sequencing (TGS) technologies, characterized by long-read sequencing (LRS), are gaining traction. Methods such as nanopore sequencing and single-molecule real-time (SMRT) sequencing are providing deeper insights into the genetic transcription mechanisms underlying diseases, Figure 3 (114, 115). Furthermore, the advantages of TGS in interpreting methylation modifications are significantly enhancing our understanding of pathological processes (116).
Sequencing technology is increasingly utilized in DCM, Figure 4. Among the diagnostic approaches, NGS technology for RNA has predominantly focused on research related to DCM, while applications of DNA sequencing and TGS remain comparatively limited. RNA sequencing technology has been instrumental in identifying numerous novel long non-coding RNAs (lncRNAs) associated with glycemic cardiopathy, which may prove useful for both diagnosis and monitoring disease progression. For instance, Yu et al. demonstrated that lncRNA NONRATT007560.2 regulates oxidative stress and apoptosis in cardiomyocytes induced by high glucose levels (117). Additionally, Xie et al. reported that lncRNA ZNF593-AS mitigates DCM by suppressing the IRF3 signaling pathway (118). A transcriptomic screening study using a rat model of DCM also identified five significant lncRNAs implicated in the condition (119). The above study relies on NGS technology to screen underscore the potential of RNA sequencing in uncovering diagnostic targets and elucidating injury mechanisms in DCM, starting from the transcriptome level.
Moreover, NGS has revealed interesting targets among other non-coding RNAs. Mathur et al. first reported the differential expression of various microRNAs (miR-1249, miR-3596d, miR-3586-3p, miR-7b-3p, miR-191, miR-330-3p, miR-328a, let-7i-5p, miR-146-3p, miR-26a-3p) in diabetes-induced cardiac cells, suggesting their potential as minimally invasive diagnostic biomarkers (120). Dong et al. identified five circular RNAs (circRNAs) involved in the pathogenesis of DCM, which may serve as promising biomarkers and therapeutic targets for early-stage intervention (121).
In the realm of mRNA, sequencing technology has broad applications as well. For example, Xie et al. found a positive correlation between USP28 mRNA levels and Mfn2 mRNA levels in hearts of diabetic patients, indicating that USP28 may promote PPARα nuclear translocation to influence Mfn2 transcription in cardiomyocytes (122). Similarly, Meng et al. demonstrated that NPRC deletion attenuates cardiac fibrosis in a diabetic mouse model by activating PKA/PKG signaling and inhibiting TGF-β1/Smad pathways, which represents an essential consideration for clinical diagnosis and treatment (123). Furthermore, RNA sequencing has established a stronger connection between DCM and inflammation as well as cell death (124, 125). A series of studies leveraging RNA sequencing have led to a more profound understanding of both classical and non-classical pathways associated with DCM, facilitating the identification of novel diagnostic targets.
In addition to routine RNA sequencing, other NGS applications are being explored for DCM diagnosis. Ju et al. combined m6A-specific methylated RNA identification with NGS to demonstrate that m6A modification patterns are altered in DCM, suggesting clinical relevance for diagnosis and treatment (126). Su et al. took full advantage of single-cell technologies, including scRNA-seq and scATAC-seq, revealing the pivotal roles of fibroblasts and endothelial cells playing in driving DM myocardial fibrosis and contributing to cardiac dysfunction (127). Advances in genome sequencing studies contribute significantly to our understanding of DM as a primary contributor to DCM. Previous research has established a clear link between DCM and DM, with the progression of DM exacerbating DCM symptoms (128). DNA sequencing in diabetic patients and genome-wide association studies (GWAS) have identified multiple genes associated with DM development, including TCF7L2, CCND2, PAM, and PDX1 (129, 130). These findings further emphasize the potential value of genome sequencing technologies, such as whole-genome sequencing (WGS) and whole-exome sequencing (WES), in the context of DCM. Distinguished from NGS, TGS could demonstrate genomic variants at higher resolution, especially for >50 bp mutations and variants in repeated sequence regions (131, 132). In previous studies, it has been found that advanced glycation end products (AGEs) and the downstream receptor for advanced glycation end products (RAGE) can lead to increased oxidative stress and activation of pro-inflammatory pathways in diabetic complications, like DCM, promoting the damage to target organs (133, 134). Researchers from India obtained high accuracy polymorphisms for RAGE gene in DM patients, which include two novel ones in the intron and 3′UTR region respectively. Analysis of genotype distribution and allele frequencies combined with clinical manifestations circled several risky RAGE gene polymorphisms resulting in DM and its complications (135). Additionally, nanopore sequencing has significantly enhanced our ability to analyze genomic variants in patients with cardiomyopathy (136). Apart from the application of TGS in detecting DCM pathogenic gene polymorphisms, LRS also plays an excellent role in revealing different RNA isoforms. Researchers found that RNA binding fox-1 homolog 2 (RBFOX2) plays a key role in the pathogenesis of DCM by mediating alternative splicing (AS) (137). By virtue of nanopore sequencing technology, a novel sequence of full-length tropomyosin 1 (TPM1) isoforms has been revealed, which was closely associated with chronic heart disease, including DCM, under RBFOX2-mediated AS regulation (138).
Challenges persist in the translation of sequencing technology into clinical diagnostics, particularly regarding the validation of biomarkers across broader populations and the reduction of associated costs. Conducting large-scale studies and pilot sampling in healthcare settings can help establish standardized processes, thereby improving the early detection and treatment of DCM. Overall, ongoing advancements in the integration of traditional clinical practices with sequencing technologies hold significant potential to revolutionize the management of diseases at their early stages.
5 Concluding remarks
The elusive onset and atypical clinical manifestations of DCM pose significant challenges to early detection, resulting in the loss of opportunities for optimal therapeutic intervention. Traditional diagnostic methods such as EMB, is difficult to become a universal diagnostic method due to the invasiveness of the procedure, limiting their widespread applicability in early DCM patients. In recent years, with the deepening of research into the pathophysiological mechanisms of DCM, a range of novel biomarkers have emerged, showing promising potential in the diagnosis of this condition. Nevertheless, the clinical application of these biomarkers still confronts numerous challenges, including suboptimal diagnostic specificity and sensitivity due to various confounding factors. Consequently, there is an urgent and critical need for large-scale, systematic patient cohort studies to thoroughly explore the clinical utility and appropriate scope of these biomarkers.
Concurrently, emerging imaging modalities, exemplified by STE, CMR and PET have offered novel approaches for early DCM detection. These techniques enable precise capture of subtle signs of myocardial metabolic changes, providing robust imaging evidence for identifying early DCM patients. However, their high costs and the potential toxicity of contrast agents involved in some of these techniques remain as bottlenecks hindering their widespread adoption. In the future, with continuous technological advancements and effective cost control, these imaging approaches are expected to play an even more pivotal role in the early diagnosis of DCM.
Compared to the above more clinically relevant tests, sequencing technology is still some distance away from the application of DCM for clinical diagnosis. However, with the booming development of sequencing technology, its application field has gradually expanded from Mendelian genetic diseases to monogenic and polygenic genetic diseases. In terms of DCM, principally, NGS and TGS can not only detect DM and cardiomyopathy-related risk gene variants at the genomic level, but also dynamically monitor the process of cardiac damage, fibrosis, and cell death pathways at the transcriptomic level. This will enable us to achieve the aim of early prevention, diagnosis and treatment of DCM, significantly improving patient course. Currently, the translation of sequencing technology to DCM clinical diagnostics still needs to overcome the following urgent challenges, calling for more advances in relevant research directions. Firstly, although emerging DCM studies with different sequencing technology discover new massages from genomic or transcriptomic dimension, there is an urgent need for guidelines to clarify the scenarios in which sequencing can be used and the standard process for diagnosis. Secondly, the cost of sequencing is still expensive, especially for WGS, which limits it to the lab and makes it difficult to move into the clinic broadly like biomarkers or imaging technologies. There still exists room to develop new materials and technologies to achieve lower costs. Thirdly, we have witnessed various advanced algorithms created for analysis of sequencing data. However, there are certain programming thresholds for clinicians, such as, the ability of basic programming and the construction of dependent environments. We need some pipelines or software packages to break down barriers to sequence analysis.
Once these challenges are effectively addressed, the emerging application of sequencing will surely enhance human health protection and pave the road for a further boom in clinic research on DCM-like diseases. For an era of healthcare characterized by the development of precision medicine and personalized medicine, the joint advancement of traditional clinical examination and sequencing technology will open up new ways for prevention and diagnosis of multiple diseases.
Author contributions
QH: Conceptualization, Visualization, Writing – original draft, Writing – review & editing. ZL: Conceptualization, Visualization, Writing – original draft, Writing – review & editing. ZZ: Visualization, Writing – review & editing. BZ: Writing – review & editing. YS: Writing – review & editing. CF: Funding acquisition, Writing – review & editing.
Funding
The author(s) declare that financial support was received for the research, authorship, and/or publication of this article. This work was supported by grants from the Public Welfare Project of Zhejiang Province (LGC22H020001) and the Key Science & Technology Project of Medicine and Health, Zhejiang province (WKJ-ZJ-2009).
Conflict of interest
The authors declare that the research was conducted in the absence of any commercial or financial relationships that could be construed as a potential conflict of interest.
Generative AI statement
The authors declare that no Generative AI was used in the creation of this manuscript.
Publisher's note
All claims expressed in this article are solely those of the authors and do not necessarily represent those of their affiliated organizations, or those of the publisher, the editors and the reviewers. Any product that may be evaluated in this article, or claim that may be made by its manufacturer, is not guaranteed or endorsed by the publisher.
References
1. Cole JB, Florez JC. Genetics of diabetes mellitus and diabetes complications. Nat Rev Nephrol. (2020) 16(7):377–90. doi: 10.1038/s41581-020-0278-5
2. Magliano DJ, Boyko EJ, Committee IDFDAtes, Idf Diabetes Atlas. Idf Diabetes Atlas. Brussels: International Diabetes Federation © International Diabetes Federation (2021).
3. Hossain MJ, Al-Mamun M, Islam MR. Diabetes mellitus, the fastest growing global public health concern: early detection should be focused. Health Sci Rep. (2024) 7(3):e2004. doi: 10.1002/hsr2.2004
4. Wang J, Zhou L, Yin W, Hu C, Zuo X. Trends of the burden of type 2 diabetes mellitus attributable to high body mass index from 1990 to 2019 in China. Front Endocrinol. (2023) 14:1193884. doi: 10.3389/fendo.2023.1193884
5. Ong KL, Stafford LK, McLaughlin SA, Boyko EJ, Vollset SE, Smith AE, et al. Global, regional, and national burden of diabetes from 1990 to 2021, with projections of prevalence to 2050: a systematic analysis for the global burden of disease study 2021. Lancet. (2023) 402(10397):203–34. doi: 10.1016/s0140-6736(23)01301-6
6. Braunwald E. Diabetes, heart failure, and renal dysfunction: the vicious circles. Prog Cardiovasc Dis. (2019) 62(4):298–302. doi: 10.1016/j.pcad.2019.07.003
7. Rosano GM, Vitale C, Seferovic P. Heart failure in patients with diabetes mellitus. Card Fail Rev. (2017) 3(1):52–5. doi: 10.15420/cfr.2016:20:2
8. Heidenreich PA, Bozkurt B, Aguilar D, Allen LA, Byun JJ, Colvin MM, et al. 2022 Aha/Acc/Hfsa guideline for the management of heart failure: executive summary: a report of the American college of cardiology/American heart association joint committee on clinical practice guidelines. Circulation. (2022) 145(18):e876–e94. doi: 10.1161/cir.0000000000001062
9. Seferović PM, Paulus WJ. Clinical diabetic cardiomyopathy: a two-faced disease with restrictive and dilated phenotypes. Eur Heart J. (2015) 36(27):1718–27. doi: 10.1093/eurheartj/ehv134
10. Lee MMY, McMurray JJV, Lorenzo-Almorós A, Kristensen SL, Sattar N, Jhund PS, et al. Diabetic cardiomyopathy. Heart. (2019) 105(4):337–45. doi: 10.1136/heartjnl-2016-310342
11. Hensel KO. Non-Ischemic diabetic cardiomyopathy may initially exhibit a transient subclinical phase of hyperdynamic myocardial performance. Med Hypotheses. (2016) 94:7–10. doi: 10.1016/j.mehy.2016.06.002
12. Jia G, DeMarco VG, Sowers JR. Insulin resistance and hyperinsulinaemia in diabetic cardiomyopathy. Nat Rev Endocrinol. (2016) 12(3):144–53. doi: 10.1038/nrendo.2015.216
13. Boyer JK, Thanigaraj S, Schechtman KB, Pérez JE. Prevalence of ventricular diastolic dysfunction in asymptomatic, normotensive patients with diabetes mellitus. Am J Cardiol. (2004) 93(7):870–5. doi: 10.1016/j.amjcard.2003.12.026
14. Shivalkar B, Dhondt D, Goovaerts I, Van Gaal L, Bartunek J, Van Crombrugge P, et al. Flow mediated dilatation and cardiac function in type 1 diabetes mellitus. Am J Cardiol. (2006) 97(1):77–82. doi: 10.1016/j.amjcard.2005.07.111
15. Huo JL, Feng Q, Pan S, Fu WJ, Liu Z, Liu Z. Diabetic cardiomyopathy: early diagnostic biomarkers, pathogenetic mechanisms, and therapeutic interventions. Cell Death Discov. (2023) 9(1):256. doi: 10.1038/s41420-023-01553-4
16. Wamil M, Goncalves M, Rutherford A, Borlotti A, Pellikka PA. Multi-modality cardiac imaging in the management of diabetic heart disease. Front Cardiovasc Med. (2022) 9:1043711. doi: 10.3389/fcvm.2022.1043711
17. Marwick TH, Gimelli A, Plein S, Bax JJ, Charron P, Delgado V, et al. Multimodality imaging approach to left ventricular dysfunction in diabetes: an expert consensus document from the European association of cardiovascular imaging. Eur Heart J Cardiovasc Imaging. (2022) 23(2):e62–84. doi: 10.1093/ehjci/jeab220
18. Wang L, Bai Y, Cao Z, Guo Z, Lian Y, Liu P, et al. Histone deacetylases and inhibitors in diabetes mellitus and its complications. Biomed Pharmacother. (2024) 177:117010. doi: 10.1016/j.biopha.2024.117010
19. Yao X, Huang X, Chen J, Lin W, Tian J. Roles of non-coding Rna in diabetic cardiomyopathy. Cardiovasc Diabetol. (2024) 23(1):227. doi: 10.1186/s12933-024-02252-9
20. Rabinovich-Nikitin I, Kirshenbaum E, Kirshenbaum LA. Autophagy, clock genes, and cardiovascular disease. Can J Cardiol. (2023) 39(12):1772–80. doi: 10.1016/j.cjca.2023.08.022
21. Kakkar C, Sharma V, Mannan A, Gupta G, Singh S, Kumar P, et al. Diabetic cardiomyopathy: an update on emerging pathological mechanisms. Curr Cardiol Rev. (2024). doi: 10.2174/011573403X331870241025094307
22. Ramesh P, Yeo JL, Brady EM, McCann GP. Role of inflammation in diabetic cardiomyopathy. Ther Adv Endocrinol Metab. (2022) 13:20420188221083530. doi: 10.1177/20420188221083530
23. Shaver A, Nichols A, Thompson E, Mallick A, Payne K, Jones C, et al. Role of serum biomarkers in early detection of diabetic cardiomyopathy in the West Virginian population. Int J Med Sci. (2016) 13(3):161–8. doi: 10.7150/ijms.14141
24. Abukhalil MH, Althunibat OY, Aladaileh SH, Al-Amarat W, Obeidat HM, Al-Khawalde AAA, et al. Galangin attenuates diabetic cardiomyopathy through modulating oxidative stress, inflammation and apoptosis in rats. Biomed Pharmacother. (2021) 138:111410. doi: 10.1016/j.biopha.2021.111410
25. Dinh W, Füth R, Nickl W, Krahn T, Ellinghaus P, Scheffold T, et al. Elevated plasma levels of tnf-alpha and interleukin-6 in patients with diastolic dysfunction and glucose metabolism disorders. Cardiovasc Diabetol. (2009) 8:58. doi: 10.1186/1475-2840-8-58
26. Lorenzo O, Picatoste B, Ares-Carrasco S, Ramírez E, Egido J, Tuñón J. Potential role of nuclear factor Κb in diabetic cardiomyopathy. Mediators Inflamm. (2011) 2011:652097. doi: 10.1155/2011/652097
27. Luo B, Li B, Wang W, Liu X, Xia Y, Zhang C, et al. Nlrp3 gene silencing ameliorates diabetic cardiomyopathy in a type 2 diabetes rat model. PLoS One. (2014) 9(8):e104771. doi: 10.1371/journal.pone.0104771
28. Herder C, Schöttker B, Rothenbacher D, Roden M, Kolb H, Müller H, et al. Interleukin-6 in the prediction of primary cardiovascular events in diabetes patients: results from the esther study. Atherosclerosis. (2011) 216(1):244–7. doi: 10.1016/j.atherosclerosis.2011.01.041
29. Ofstad AP, Gullestad L, Orvik E, Aakhus S, Endresen K, Ueland T, et al. Interleukin-6 and activin a are independently associated with cardiovascular events and mortality in type 2 diabetes: the prospective Asker and Bærum cardiovascular diabetes (Abcd) cohort study. Cardiovasc Diabetol. (2013) 12:126. doi: 10.1186/1475-2840-12-126
30. Boengler K, Hilfiker-Kleiner D, Drexler H, Heusch G, Schulz R. The myocardial Jak/Stat pathway: from protection to failure. Pharmacol Ther. (2008) 120(2):172–85. doi: 10.1016/j.pharmthera.2008.08.002
31. Shah SJ, Marcus GM, Gerber IL, McKeown BH, Vessey JC, Jordan MV, et al. High-Sensitivity C-reactive protein and parameters of left ventricular dysfunction. J Card Fail. (2006) 12(1):61–5. doi: 10.1016/j.cardfail.2005.08.003
32. Anand IS, Latini R, Florea VG, Kuskowski MA, Rector T, Masson S, et al. C-Reactive protein in heart failure: prognostic value and the effect of valsartan. Circulation. (2005) 112(10):1428–34. doi: 10.1161/circulationaha.104.508465
33. Kardys I, Knetsch AM, Bleumink GS, Deckers JW, Hofman A, Stricker BH, et al. C-Reactive protein and risk of heart failure. The rotterdam study. Am Heart J. (2006) 152(3):514–20. doi: 10.1016/j.ahj.2006.02.023
34. Araújo JP, Lourenço P, Azevedo A, Friões F, Rocha-Gonçalves F, Ferreira A, et al. Prognostic value of high-sensitivity C-reactive protein in heart failure: a systematic review. J Card Fail. (2009) 15(3):256–66. doi: 10.1016/j.cardfail.2008.10.030
35. Windram JD, Loh PH, Rigby AS, Hanning I, Clark AL, Cleland JG. Relationship of high-sensitivity C-reactive protein to prognosis and other prognostic markers in outpatients with heart failure. Am Heart J. (2007) 153(6):1048–55. doi: 10.1016/j.ahj.2007.03.044
36. Nakatani D, Sakata Y, Mizuno H, Shimizu M, Suna S, Usami M, et al. Impact of diabetes mellitus on rehospitalization for heart failure among survivors of acute myocardial infarction in the percutaneous coronary intervention era. Circ J. (2009) 73(4):662–6. doi: 10.1253/circj.cj-08-0579
37. Miyazawa K, Miyazono K. Regulation of Tgf-Β family signaling by inhibitory smads. Cold Spring Harb Perspect Biol. (2017) 9(3). doi: 10.1101/cshperspect.a022095
38. Shi Y, Massagué J. Mechanisms of Tgf-Beta signaling from cell membrane to the nucleus. Cell. (2003) 113(6):685–700. doi: 10.1016/s0092-8674(03)00432-x
39. Lin X, Duan X, Liang YY, Su Y, Wrighton KH, Long J, et al. Ppm1a functions as a Smad phosphatase to terminate Tgfbeta signaling. Cell. (2006) 125(5):915–28. doi: 10.1016/j.cell.2006.03.044
40. Li ZL, Shi Y, Ding Y, Ran Y, Le G. Dietary oxidized tyrosine (O-Tyr) stimulates Tgf-Β1-induced extracellular matrix production via the Jnk/P38 signaling pathway in rat kidneys. Amino Acids. (2017) 49(2):241–60. doi: 10.1007/s00726-016-2353-6
41. Ferreira RR, de Souza EM, de Oliveira FL, Ferrão PM, Gomes LH, Mendonça-Lima L, et al. Proteins involved on Tgf-Β pathway are up-regulated during the acute phase of experimental chagas disease. Immunobiology. (2016) 221(5):587–94. doi: 10.1016/j.imbio.2016.01.009
42. Wojciechowski MC, Mahmutovic L, Shu DY, Lovicu FJ. Erk1/2 signaling is required for the initiation but not progression of Tgfβ-induced Lens epithelial to mesenchymal transition (Emt). Exp Eye Res. (2017) 159:98–113. doi: 10.1016/j.exer.2017.03.012
43. Russo I, Frangogiannis NG. Diabetes-Associated cardiac fibrosis: cellular effectors, molecular mechanisms and therapeutic opportunities. J Mol Cell Cardiol. (2016) 90:84–93. doi: 10.1016/j.yjmcc.2015.12.011
44. Flores-Ramírez R, Azpiri-López JR, González-González JG, Ordaz-Farías A, González-Carrillo LE, Carrizales-Sepúlveda EF, et al. Global longitudinal strain as a biomarker in diabetic cardiomyopathy. A comparative study with Gal-3 in patients with preserved ejection fraction. Arch Cardiol Mex. (2017) 87(4):278–85. doi: 10.1016/j.acmx.2016.06.002
45. Seropian IM, Cassaglia P, Miksztowicz V, González GE. Unraveling the role of galectin-3 in cardiac pathology and physiology. Front Physiol. (2023) 14:1304735. doi: 10.3389/fphys.2023.1304735
46. Xu GR, Zhang C, Yang HX, Sun JH, Zhang Y, Yao TT, et al. Modified citrus pectin ameliorates myocardial fibrosis and inflammation via suppressing galectin-3 and Tlr4/Myd88/Nf-Κb signaling pathway. Biomed Pharmacother. (2020) 126:110071. doi: 10.1016/j.biopha.2020.110071
47. Martínez-Martínez E, Jurado-López R, Cervantes-Escalera P, Cachofeiro V, Miana M. Leptin, a mediator of cardiac damage associated with obesity. Horm Mol Biol Clin Investig. (2014) 18(1):3–14. doi: 10.1515/hmbci-2013-0060
48. Paolisso G, Tagliamonte MR, Galderisi M, Zito GA, Petrocelli A, Carella C, et al. Plasma leptin level is associated with myocardial wall thickness in hypertensive insulin-resistant men. Hypertension. (1999) 34(5):1047–52. doi: 10.1161/01.hyp.34.5.1047
49. Li J, Shen X. Oxidative stress and adipokine levels were significantly correlated in diabetic patients with hyperglycemic crises. Diabetol Metab Syndr. (2019) 11:13. doi: 10.1186/s13098-019-0410-5
50. Zhang C, Yu H, Ye J, Tong H, Wang M, Sun G. Ginsenoside Rg3 protects against diabetic cardiomyopathy and promotes adiponectin signaling via activation of Ppar-Γ. Int J Mol Sci. (2023) 24(23):16736. doi: 10.3390/ijms242316736
51. Shibata R, Sato K, Pimentel DR, Takemura Y, Kihara S, Ohashi K, et al. Adiponectin protects against myocardial ischemia-reperfusion injury through Ampk- and Cox-2-dependent mechanisms. Nat Med. (2005) 11(10):1096–103. doi: 10.1038/nm1295
52. Cooper GJ, Young AA, Gamble GD, Occleshaw CJ, Dissanayake AM, Cowan BR, et al. A copper(ii)-selective chelator ameliorates left-ventricular hypertrophy in type 2 diabetic patients: a randomised placebo-controlled study. Diabetologia. (2009) 52(4):715–22. doi: 10.1007/s00125-009-1265-3
53. Zhang S, Liu H, Amarsingh GV, Cheung CC, Hogl S, Narayanan U, et al. Diabetic cardiomyopathy is associated with defective myocellular copper regulation and both defects are rectified by divalent copper chelation. Cardiovasc Diabetol. (2014) 13:100. doi: 10.1186/1475-2840-13-100
54. Ruiz-Hurtado G, Gómez-Hurtado N, Fernández-Velasco M, Calderón E, Smani T, Ordoñez A, et al. Cardiotrophin-1 induces sarcoplasmic reticulum Ca(2+) leak and arrhythmogenesis in adult rat ventricular myocytes. Cardiovasc Res. (2012) 96(1):81–9. doi: 10.1093/cvr/cvs234
55. Jougasaki M. Cardiotrophin-1 in cardiovascular regulation. Adv Clin Chem. (2010) 52:41–76. doi: 10.1016/s0065-2423(10)52002-x
56. Deng J, Zhang N, Chen F, Yang C, Ning H, Xiao C, et al. Irisin ameliorates high glucose-induced cardiomyocytes injury via Ampk/Mtor signal pathway. Cell Biol Int. (2020) 44(11):2315–25. doi: 10.1002/cbin.11441
57. Dahlström U. Can natriuretic peptides be used for the diagnosis of diastolic heart failure? Eur J Heart Fail. (2004) 6(3):281–7. doi: 10.1016/j.ejheart.2004.01.005
58. Chang P, Zhang X, Zhang J, Wang J, Wang X, Li M, et al. Bnp protects against diabetic cardiomyopathy by promoting Opa1-mediated mitochondrial fusion via activating the Pkg-Stat3 pathway. Redox Biol. (2023) 62:102702. doi: 10.1016/j.redox.2023.102702
59. Betti I, Castelli G, Barchielli A, Beligni C, Boscherini V, De Luca L, et al. The role of N-terminal pro-brain natriuretic peptide and echocardiography for screening asymptomatic left ventricular dysfunction in a population at high risk for heart failure. The Probe-Hf study. J Card Fail. (2009) 15(5):377–84. doi: 10.1016/j.cardfail.2008.12.002
60. Sun S, Dawuti A, Gong D, Wang R, Yuan T, Wang S, et al. Puerarin-V improve mitochondrial respiration and cardiac function in a rat model of diabetic cardiomyopathy via inhibiting pyroptosis pathway through P2x7 receptors. Int J Mol Sci. (2022) 23(21):13015. doi: 10.3390/ijms232113015
61. Akbal E, Özbek M, Güneş F, Akyürek Ö, Üreten K, Delibaşı T. Serum heart type fatty acid binding protein levels in metabolic syndrome. Endocrine. (2009) 36(3):433–7. doi: 10.1007/s12020-009-9243-6
62. Wang X, Yan K, Wen C, Wang J. Simvastatin combined with resistance training improves outcomes in patients with chronic heart failure by modulating mitochondrial membrane potential and the Janus kinase/signal transducer and activator of transcription 3 signaling pathways. Cardiovasc Ther. (2022) 2022:8430733. doi: 10.1155/2022/8430733
63. Shi B, Wang J, Zhang J, Li J, Hao Y, Lin X, et al. Dapagliflozin suppresses high glucose-induced proliferation, oxidative stress, and fibrosis by reducing Mettl3-induced M6a modification in Marcks Mrna. Cardiovasc Toxicol. (2024). doi: 10.1007/s12012-024-09945-3
64. Pan KL, Hsu YC, Chang ST, Chung CM, Lin CL. The role of cardiac fibrosis in diabetic cardiomyopathy: from pathophysiology to clinical diagnostic tools. Int J Mol Sci. (2023) 24(10):8604. doi: 10.3390/ijms24108604
65. Ziyadeh FN, Sharma K, Ericksen M, Wolf G. Stimulation of collagen gene expression and protein synthesis in murine mesangial cells by high glucose is mediated by autocrine activation of transforming growth factor-beta. J Clin Invest. (1994) 93(2):536–42. doi: 10.1172/jci117004
66. Toblli JE, Cao G, DeRosa G, Forcada P. Reduced cardiac expression of plasminogen activator inhibitor 1 and transforming growth factor Beta1 in Obese Zucker rats by perindopril. Heart. (2005) 91(1):80–6. doi: 10.1136/hrt.2003.022707
67. Song X, Qian X, Shen M, Jiang R, Wagner MB, Ding G, et al. Protein kinase C promotes cardiac fibrosis and heart failure by modulating galectin-3 expression. Biochim Biophys Acta. (2015) 1853(2):513–21. doi: 10.1016/j.bbamcr.2014.12.001
68. Holmager P, Egstrup M, Gustafsson I, Schou M, Dahl JS, Rasmussen LM, et al. Galectin-3 and fibulin-1 in systolic heart failure—relation to glucose metabolism and left ventricular Contractile reserve. BMC Cardiovasc Disord. (2017) 17(1):22. doi: 10.1186/s12872-016-0437-6
69. Margadant C, van den Bout I, van Boxtel AL, Thijssen VL, Sonnenberg A. Epigenetic regulation of galectin-3 expression by Β1 integrins promotes cell adhesion and migration. J Biol Chem. (2012) 287(53):44684–93. doi: 10.1074/jbc.M112.426445
70. Ruebel KH, Jin L, Qian X, Scheithauer BW, Kovacs K, Nakamura N, et al. Effects of DNA methylation on galectin-3 expression in pituitary tumors. Cancer Res. (2005) 65(4):1136–40. doi: 10.1158/0008-5472.Can-04-3578
71. Li H, Yao W, Irwin MG, Wang T, Wang S, Zhang L, et al. Adiponectin ameliorates hyperglycemia-induced cardiac hypertrophy and dysfunction by concomitantly activating Nrf2 and Brg1. Free Radic Biol Med. (2015) 84:311–21. doi: 10.1016/j.freeradbiomed.2015.03.007
72. Yuan J, Li F, Cui B, Gao J, Yu Z, Lu Z. Inhibition of Gcn2 alleviates cardiomyopathy in type 2 diabetic mice via attenuating lipotoxicity and oxidative stress. Antioxidants. (2022) 11(7):1379. doi: 10.3390/antiox11071379
73. Wang M, Zhang S, Tian J, Yang F, Chen H, Bai S, et al. Impaired iron-sulfur cluster synthesis induces mitochondrial parthanatos in diabetic cardiomyopathy. Adv Sci (Weinh). (2024):e2406695. doi: 10.1002/advs.202406695
74. Berger S, Polotsky VY. Leptin and leptin resistance in the pathogenesis of obstructive sleep apnea: a possible link to oxidative stress and cardiovascular complications. Oxid Med Cell Longev. (2018) 2018:5137947. doi: 10.1155/2018/5137947
75. Ren J. Leptin and hyperleptinemia—from friend to foe for cardiovascular function. J Endocrinol. (2004) 181(1):1–10. doi: 10.1677/joe.0.1810001
76. Cui X, Wang Y, Liu H, Shi M, Wang J, Wang Y. The molecular mechanisms of defective copper metabolism in diabetic cardiomyopathy. Oxid Med Cell Longev. (2022) 2022:5418376. doi: 10.1155/2022/5418376
77. Elendu C, Amaechi DC, Elendu TC, Ashna M, Ross-Comptis J, Ansong SO, et al. Heart failure and diabetes: understanding the bidirectional relationship. Medicine. (2023) 102(37):e34906. doi: 10.1097/md.0000000000034906
78. Bhatia V, Nayyar P, Dhindsa S. Brain natriuretic peptide in diagnosis and treatment of heart failure. J Postgrad Med. (2003) 49(2):182–5.12867703
79. Tanase DM, Radu S, Al Shurbaji S, Baroi GL, Florida Costea C, Turliuc MD, et al. Natriuretic peptides in heart failure with preserved left ventricular ejection fraction: from molecular evidences to clinical implications. Int J Mol Sci. (2019) 20(11):2629. doi: 10.3390/ijms20112629
80. Shearer J, Fueger PT, Wang Z, Bracy DP, Wasserman DH, Rottman JN. Metabolic implications of reduced heart-type fatty acid binding protein in insulin resistant cardiac muscle. Biochim Biophys Acta. (2008) 1782(10):586–92. doi: 10.1016/j.bbadis.2008.07.003
81. Lorenzo-Almorós A, Tuñón J, Orejas M, Cortés M, Egido J, Lorenzo Ó. Diagnostic approaches for diabetic cardiomyopathy. Cardiovasc Diabetol. (2017) 16(1):28. doi: 10.1186/s12933-017-0506-x
82. Teupe C, Rosak C. Diabetic cardiomyopathy and diastolic heart failure—difficulties with relaxation. Diabetes Res Clin Pract. (2012) 97(2):185–94. doi: 10.1016/j.diabres.2012.03.008
83. Takenaka K, Sakamoto T, Amano K, Oku J, Fujinami K, Murakami T, et al. Left ventricular filling determined by Doppler echocardiography in diabetes Mellitus. Am J Cardiol. (1988) 61(13):1140–3. doi: 10.1016/0002-9149(88)90149-x
84. Di Bonito P, Moio N, Cavuto L, Covino G, Murena E, Scilla C, et al. Early detection of diabetic cardiomyopathy: usefulness of tissue Doppler imaging. Diabet Med. (2005) 22(12):1720–5. doi: 10.1111/j.1464-5491.2005.01685.x
85. Urlic H, Kumric M, Vrdoljak J, Martinovic D, Dujic G, Vilovic M, et al. Role of echocardiography in diabetic cardiomyopathy: from mechanisms to clinical practice. J Cardiovasc Dev Dis. (2023) 10(2):46. doi: 10.3390/jcdd10020046
86. Wang J, Khoury DS, Yue Y, Torre-Amione G, Nagueh SF. Preserved left ventricular twist and circumferential deformation, but depressed longitudinal and radial deformation in patients with diastolic heart failure. Eur Heart J. (2007) 29(10):1283–9. doi: 10.1093/eurheartj/ehn141
87. Greenberg NL, Firstenberg MS, Castro PL, Main M, Travaglini A, Odabashian JA, et al. Doppler-Derived myocardial systolic strain rate is a strong index of left ventricular contractility. Circulation. (2002) 105(1):99–105. doi: 10.1161/hc0102.101396
88. Miki T, Yuda S, Kouzu H, Miura T. Diabetic cardiomyopathy: pathophysiology and clinical features. Heart Fail Rev. (2013) 18(2):149–66. doi: 10.1007/s10741-012-9313-3
89. Vinazzer H. Risk factors for venous thromboembolism. Wien Med Wochenschr. (1989) 139(23):538–42.2696212
90. Liu X, Yang ZG, Gao Y, Xie LJ, Jiang L, Hu BY, et al. Left ventricular subclinical myocardial dysfunction in uncomplicated type 2 diabetes mellitus is associated with impaired myocardial perfusion: a contrast-enhanced cardiovascular magnetic resonance study. Cardiovasc Diabetol. (2018) 17(1):139. doi: 10.1186/s12933-018-0782-0
91. Mochizuki Y, Tanaka H, Matsumoto K, Sano H, Shimoura H, Ooka J, et al. Impaired mechanics of left ventriculo-atrial coupling in patients with diabetic nephropathy. Circ J. (2016) 80(9):1957–64. doi: 10.1253/circj.CJ-16-0488
92. Tadic M, Cuspidi C, Calicchio F, Grassi G, Mancia G. Diabetic cardiomyopathy: how can cardiac magnetic resonance help? Acta Diabetol. (2020) 57(9):1027–34. doi: 10.1007/s00592-020-01528-2
93. Collier P, Phelan D, Klein A. A test in context: myocardial strain measured by speckle-tracking echocardiography. J Am Coll Cardiol. (2017) 69(8):1043–56. doi: 10.1016/j.jacc.2016.12.012
94. Aneja A, Tang WH, Bansilal S, Garcia MJ, Farkouh ME. Diabetic cardiomyopathy: insights into pathogenesis, diagnostic challenges, and therapeutic options. Am J Med. (2008) 121(9):748–57. doi: 10.1016/j.amjmed.2008.03.046
95. Fischer SE, McKinnon GC, Maier SE, Boesiger P. Improved myocardial tagging contrast. Magn Reson Med. (1993) 30(2):191–200. doi: 10.1002/mrm.1910300207
96. Schuijf JD, Bax JJ, Jukema JW, Lamb HJ, Vliegen HW, Salm LP, et al. Noninvasive angiography and assessment of left ventricular function using multislice computed tomography in patients with type 2 diabetes. Diabetes Care. (2004) 27(12):2905–10. doi: 10.2337/diacare.27.12.2905
97. Mordi IR. Non-invasive imaging in diabetic cardiomyopathy. J Cardiovasc Dev Dis. (2019) 6(2):18. doi: 10.3390/jcdd6020018
98. Di Carli MF, Hachamovitch R. Should we screen for occult coronary artery disease among asymptomatic patients with diabetes? J Am Coll Cardiol. (2005) 45(1):50–3. doi: 10.1016/j.jacc.2004.09.055
99. Sasso FC, Rambaldi PF, Carbonara O, Nasti R, Torella M, Rotondo A, et al. Perspectives of nuclear diagnostic imaging in diabetic cardiomyopathy. Nutr Metab Cardiovasc Dis. (2010) 20(3):208–16. doi: 10.1016/j.numecd.2009.08.013
100. Gropler RJ. Recent advances in metabolic imaging. J Nucl Cardiol. (2013) 20(6):1147–72. doi: 10.1007/s12350-013-9786-z
101. Peterson LR, Gropler RJ. Radionuclide imaging of myocardial metabolism. Circ Cardiovasc Imaging. (2010) 3(2):211–22. doi: 10.1161/circimaging.109.860593
102. American Diabetes Association. Consensus development conference on the diagnosis of coronary heart disease in people with diabetes: 10–11 February 1998, Miami, Florida. Diabetes Care. (1998) 21(9):1551–9. doi: 10.2337/diacare.21.9.1551
103. van den Brom CE, Huisman MC, Vlasblom R, Boontje NM, Duijst S, Lubberink M, et al. Altered myocardial substrate metabolism is associated with myocardial dysfunction in early diabetic cardiomyopathy in rats: studies using positron emission tomography. Cardiovasc Diabetol. (2009) 8:39. doi: 10.1186/1475-2840-8-39
104. Peterson LR, Gropler RJ. Metabolic and molecular imaging of the diabetic cardiomyopathy. Circ Res. (2020) 126(11):1628–45. doi: 10.1161/circresaha.120.315899
105. Sanger F, Nicklen S, Coulson AR. DNA sequencing with chain-terminating inhibitors. Proc Natl Acad Sci. (1977) 74(12):5463–7. doi: 10.1073/pnas.74.12.5463
106. Green PM, Giannelli F. Direct sequencing of Pcr-amplified DNA. Mol Biotechnol. (1994) 1(2):117–24. doi: 10.1007/bf02921552
107. Lagerkvist A, Stewart J, Lagerström-Fermér M, Landegren U. Manifold sequencing: efficient processing of large sets of sequencing reactions. Proc Natl Acad Sci. (1994) 91(6):2245–9. doi: 10.1073/pnas.91.6.2245
108. Turcatti G, Romieu A, Fedurco M, Tairi AP. A new class of cleavable fluorescent nucleotides: synthesis and optimization as reversible terminators for DNA sequencing by synthesis. Nucleic Acids Res. (2008) 36(4):e25. doi: 10.1093/nar/gkn021
109. Drmanac R, Sparks AB, Callow MJ, Halpern AL, Burns NL, Kermani BG, et al. Human genome sequencing using unchained base reads on self-assembling DNA nanoarrays. Science. (2010) 327(5961):78–81. doi: 10.1126/science.1181498
110. Goodwin S, McPherson JD, McCombie WR. Coming of age: ten years of next-generation sequencing technologies. Nat Rev Genet. (2016) 17(6):333–51. doi: 10.1038/nrg.2016.49
111. Zong C, Lu S, Chapman AR, Xie XS. Genome-wide detection of single-nucleotide and copy-number variations of a single human cell. Science. (2012) 338(6114):1622–6. doi: 10.1126/science.1229164
112. Lieberman-Aiden E, van Berkum NL, Williams L, Imakaev M, Ragoczy T, Telling A, et al. Comprehensive mapping of long-range interactions reveals folding principles of the human genome. Science. (2009) 326(5950):289–93. doi: 10.1126/science.1181369
113. Buenrostro JD, Giresi PG, Zaba LC, Chang HY, Greenleaf WJ. Transposition of native chromatin for fast and sensitive epigenomic profiling of open chromatin, DNA-binding proteins and nucleosome position. Nat Methods. (2013) 10(12):1213–8. doi: 10.1038/nmeth.2688
114. Eid J, Fehr A, Gray J, Luong K, Lyle J, Otto G, et al. Real-time DNA sequencing from single polymerase molecules. Science. (2009) 323(5910):133–8. doi: 10.1126/science.1162986
115. Bayley H. Nanopore sequencing: from imagination to reality. Clin Chem. (2015) 61(1):25–31. doi: 10.1373/clinchem.2014.223016
116. Lüth T, Laβ J, Schaake S, Wohlers I, Pozojevic J, Jamora RDG, et al. Elucidating hexanucleotide repeat number and methylation within the X-linked dystonia-parkinsonism (Xdp)-related sva retrotransposon in Taf1 with nanopore sequencing. Genes. (2022) 13(1):126. doi: 10.3390/genes13010126
117. Yu M, Shan X, Liu Y, Zhu J, Cao Q, Yang F, et al. RNA-Seq analysis and functional characterization revealed lncrna nonratt007560.2 regulated cardiomyocytes oxidative stress and apoptosis induced by high glucose. J Cell Biochem. (2019) 120(10):18278–87. doi: 10.1002/jcb.29134
118. Xie R, Fan J, Wen J, Jin K, Zhan J, Yuan S, et al. Lncrna Znf593-as alleviates diabetic cardiomyopathy via suppressing Irf3 signaling pathway. Mol Ther Nucleic Acids. (2023) 32:689–703. doi: 10.1016/j.omtn.2023.04.025
119. Xi Y, Chen D, Dong Z, Lam H, He J, Du K, et al. Rna sequencing of cardiac in a rat model uncovers potential target lncrna of diabetic cardiomyopathy. Front Genet. (2022) 13:848364. doi: 10.3389/fgene.2022.848364
120. Mathur P, Rani V. Investigating micrornas in diabetic cardiomyopathy as tools for early detection and therapeutics. Mol Cell Biochem. (2023) 478(2):229–40. doi: 10.1007/s11010-022-04473-6
121. Dong S, Tu C, Ye X, Li L, Zhang M, Xue A, et al. Expression profiling of circular Rnas and their potential role in early-stage diabetic cardiomyopathy. Mol Med Rep. (2020) 22(3):1958–68. doi: 10.3892/mmr.2020.11248
122. Xie SY, Liu SQ, Zhang T, Shi WK, Xing Y, Fang WX, et al. Usp28 serves as a key suppressor of mitochondrial morphofunctional defects and cardiac dysfunction in the diabetic heart. Circulation. (2024) 149(9):684–706. doi: 10.1161/circulationaha.123.065603
123. Meng L, Lu Y, Wang X, Cheng C, Xue F, Xie L, et al. Nprc deletion attenuates cardiac fibrosis in diabetic mice by activating Pka/Pkg and Inhibiting Tgf-Β1/Smad pathways. Sci Adv. (2023) 9(31):eadd4222. doi: 10.1126/sciadv.add4222
124. Luo W, Lin K, Hua J, Han J, Zhang Q, Chen L, et al. Schisandrin B attenuates diabetic cardiomyopathy by targeting Myd88 and inhibiting Myd88-dependent inflammation. Adv Sci. (2022) 9(31):e2202590. doi: 10.1002/advs.202202590
125. Chen Z, Li S, Liu M, Yin M, Chen J, Li Y, et al. Nicorandil alleviates cardiac microvascular ferroptosis in diabetic cardiomyopathy: role of the mitochondria-localized Ampk-Parkin-Acsl4 signaling pathway. Pharmacol Res. (2024) 200:107057. doi: 10.1016/j.phrs.2024.107057
126. Ju W, Liu K, Ouyang S, Liu Z, He F, Wu J. Changes in N6-methyladenosine modification modulate diabetic cardiomyopathy by reducing myocardial fibrosis and myocyte hypertrophy. Front Cell Dev Biol. (2021) 9:702579. doi: 10.3389/fcell.2021.702579
127. Su Q, Huang W, Huang Y, Dai R, Chang C, Li QY, et al. Single-cell insights: pioneering an integrated atlas of chromatin accessibility and transcriptomic landscapes in diabetic cardiomyopathy. Cardiovasc Diabetol. (2024) 23(1):139. doi: 10.1186/s12933-024-02233-y
128. Nakamura K, Miyoshi T, Yoshida M, Akagi S, Saito Y, Ejiri K, et al. Pathophysiology and treatment of diabetic cardiomyopathy and heart failure in patients with diabetes mellitus. Int J Mol Sci. (2022) 23(7):3587. doi: 10.3390/ijms23073587
129. Grant SF, Thorleifsson G, Reynisdottir I, Benediktsson R, Manolescu A, Sainz J, et al. Variant of transcription factor 7-like 2 (Tcf7l2) gene confers risk of type 2 diabetes. Nat Genet. (2006) 38(3):320–3. doi: 10.1038/ng1732
130. Steinthorsdottir V, Thorleifsson G, Sulem P, Helgason H, Grarup N, Sigurdsson A, et al. Identification of low-frequency and rare sequence variants associated with elevated or reduced risk of type 2 diabetes. Nat Genet. (2014) 46(3):294–8. doi: 10.1038/ng.2882
131. Sedlazeck FJ, Lee H, Darby CA, Schatz MC. Piercing the dark matter: bioinformatics of long-range sequencing and mapping. Nat Rev Genet. (2018) 19(6):329–46. doi: 10.1038/s41576-018-0003-4
132. Mahmoud M, Gobet N, Cruz-Dávalos DI, Mounier N, Dessimoz C, Sedlazeck FJ. Structural variant calling: the long and the short of it. Genome Biol. (2019) 20(1):246. doi: 10.1186/s13059-019-1828-7
133. Singh VP, Bali A, Singh N, Jaggi AS. Advanced glycation end products and diabetic complications. Korean J Physiol Pharmacol. (2014) 18(1):1–14. doi: 10.4196/kjpp.2014.18.1.1
134. Vlassara H, Uribarri J. Advanced glycation end products (age) and diabetes: cause, effect, or both? Curr Diab Rep. (2014) 14(1):453. doi: 10.1007/s11892-013-0453-1
135. Chaurasiya AH, Khilari AA, Kazi R, Jaiswal MR, Bhoite GM, Padwal MK, et al. Nanopore sequencing of rage gene polymorphisms and their association with type 2 diabetes. ACS Omega. (2023) 8(29):25727–38. doi: 10.1021/acsomega.3c00297
136. Sedaghat-Hamedani F, Rebs S, Kayvanpour E, Zhu C, Amr A, Müller M, et al. Genotype complements the phenotype: identification of the pathogenicity of an lmna splice variant by nanopore long-read sequencing in a large Dcm family. Int J Mol Sci. (2022) 23(20):12230. doi: 10.3390/ijms232012230
137. Nutter CA, Jaworski EA, Verma SK, Deshmukh V, Wang Q, Botvinnik OB, et al. Dysregulation of Rbfox2 is an early event in cardiac pathogenesis of diabetes. Cell Rep. (2016) 15(10):2200–13. doi: 10.1016/j.celrep.2016.05.002
138. Cao J, Routh AL, Kuyumcu-Martinez MN. Nanopore sequencing reveals full-length tropomyosin 1 isoforms and their regulation by Rna-binding proteins during rat heart development. J Cell Mol Med. (2021) 25(17):8352–62. doi: 10.1111/jcmm.16795
Glossary
2D-STE two-dimensional speckle tracking echocardiography
3D-STE three-dimensional speckle tracking echocardiography
AGEs advanced glycation end products
AS alternative splicing
AMPK AMP-activated protein kinase
APN adiponectin
ATAC-Seq assay for transposase-accessible chromatin using sequencing
BNP brain natriuretic peptide
bp base pairs
CCND2 cyclin D2
CE conventional echocardiography
circRNAs circular RNAs
CMR cardiovascular magnetic resonance
CRP C-reactive protein
CT-1 Cardiotrophin-1
DCM diabetic cardiomyopathy
ddNTPs dideoxy-nucleoside triphosphates
DM diabetes mellitus
EF ejection fraction
EMB endomyocardial biopsy
ET-1 endothelin-1
Gal-3 galectin-3
GLS global longitudinal strain
G-SPECT gated single-photon emission computed tomography
GWAS genome-wide association studies
H-FABP heat-fatty acid binding protein
Hi-C high-throughput chromosome conformation
IL-1 interleukin-1
IL-1β interleukin-1β
IL-6 interleukin-6
IRF3 interferon regulatory factor 3
lncRNAs long ncRNAs
LRS long-read sequencing
LV left ventricular
LVD left ventricular diameter
LVEF left ventricular ejection fraction
m6A N6-methyladenosine
Mfn2 mitofusin 2
MSCT multi-slice computed tomography
mTOR mammalian target of rapamycin
NF-κB nuclear factor kappa B
NGS next-generation sequencing
NPRC natriuretic peptide receptor C
NT-ProBNP N-terminal pro-brain natriuretic peptide
PAM peptidylglycine α-amidating monooxygenase
PCR polymerase chain reaction
PDX1 pancreatic duodenal homeobox 1
PET positron emission tomography
PKA protein kinase A
PKG protein kinase G
RAGE receptor for advanced glycation end products
RCA rolling circle amplification
RBFOX2 RNA binding fox-1 homolog 2
ROS reactive oxygen species
SMAD small mother against decapentaplegic
SMRT single-molecule real-time
sST2 soluble growth stimulation expressed gene2
STE speckle tracking echocardiography
T1DM type 1 diabetes mellitus
T2DM type 2 diabetes mellitus
TCF7L2 transcription factor 7-like 2
TDI tissue doppler imaging
TGF-β transforming growth factor beta
TGS third-generation sequencing
TNF-α tumor necrosis factor alpha
TPM1 tropomyosin 1
T2DM type 2 diabetes mellitus
WES whole-exome sequencing
WGS whole-genome sequencing
Keywords: diabetic cardiomyopathy, biomarkers, early diagnosis, diagnostic imaging, inflammatory mediator, speckle tracking echocardiography, sequencing
Citation: He Q, Lai Z, Zhai Z, Zou B, Shi Y and Feng C (2025) Advances of research in diabetic cardiomyopathy: diagnosis and the emerging application of sequencing. Front. Cardiovasc. Med. 11:1501735. doi: 10.3389/fcvm.2024.1501735
Received: 25 September 2024; Accepted: 27 December 2024;
Published: 13 January 2025.
Edited by:
Yafei Li, Nanjing Medical University, ChinaReviewed by:
Alexander E. Berezin, Paracelsus Medical University, AustriaEsma Nur Okatan, University of Istinye, Türkiye
Chenxi Xia, Peking University, China
Ashot Avagimyan, Yerevan State Medical University, Armenia
Copyright: © 2025 He, Lai, Zhai, Zou, Shi and Feng. This is an open-access article distributed under the terms of the Creative Commons Attribution License (CC BY). The use, distribution or reproduction in other forums is permitted, provided the original author(s) and the copyright owner(s) are credited and that the original publication in this journal is cited, in accordance with accepted academic practice. No use, distribution or reproduction is permitted which does not comply with these terms.
*Correspondence: Chao Feng, ODAxMzAxMEB6anUuZWR1LmNu
†These authors have contributed equally to this work