- 1The Second Affiliated Hospital of Heilongjiang University of Chinese Medicine, Harbin, Heilongjiang, China
- 2Heilongjiang University of Chinese Medicine, Harbin, Heilongjiang, China
Objective: This study aimed to investigate the causal relationship between insomnia and the risk of myocardial infarction (MI) and explore potential mediators such as smoking initiation, alcohol consumption and body mass index (BMI) using mendelian randomization (MR) analysis.
Methods: Data from 1,207,228 individuals of European ancestry were obtained from the UK Biobank and 23andMe for insomnia-related genetic associations. Genetic instruments for MI, smoking initiation, alcohol consumption, and BMI were derived from large-scale genome-wide association studies. Univariate MR analysis mainly utilized the inverse variance weighting method, and multivariable MR analysis assessed the mediation effects of smoking initiation and BMI.
Results: The univariate MR analysis revealed a 96% increased risk of MI in individuals with insomnia [odds ratio (OR) = 1.96; 95% CI: 1.67, 2.31]. Smoking initiation and BMI were identified as potential mediators. The multivariable MR analysis indicated smoking initiation accounted for 29% of the total effect (95% CI: 13%, 61%), while BMI accounted for 15% (95% CI: 7%, 27%), with a combined mediation proportion of 54% (95% CI: 31%, 91%).
Conclusions: The results of this MR analysis demonstrate that insomnia increases the risk of MI. Quitting smoking and losing weight may reduce this risk; however, there is still a portion of the impact of insomnia on MI that cannot be explained. Therefore, further investigation into other potentially modifiable intermediate factors is necessary.
1 Introduction
Myocardial infarction (MI) is a serious cardiovascular disease, typically caused by the blockage of coronary arteries, leading to myocardial ischemia and necrosis (1). With the changes in social lifestyles and the increase in unhealthy habits, the incidence of MI is gradually rising, exerting a significant impact on public health, prompting scientists to delve into its potential influencing factors (2). Epidemiological studies have revealed high-risk factors for MI, including hypertension (3), hyperlipidemia (4), diabetes (5) and so on. In recent years, research on the relationship between insomnia and MI has gradually attracted widespread attention in the academic community.
Insomnia is the most common sleep disorder, known to have a negative impact on the overall health and quality of life of the population (6). Recent research reports indicated a high prevalence of insomnia in the United States, ranging from 10% to 15% (7). An increasing number of studies suggested a close association between insomnia and MI (8, 9), but the limitations of observational studies cannot be ignored. Additionally, lifestyle factors such as smoking (10, 11), alcohol consumption (12, 13), and body mass index (BMI) (14, 15) have also been confirmed to be associated with insomnia. However, these relationships are often limited by observational studies, requiring more in-depth research methods to reveal potential causal effects.
Mendelian randomization (MR) analysis provides a powerful tool in addressing the limitations of observational studies and revealing causal effects. By using genetic variations as instrumental variables (IVs) to assess the causal impact of exposure on outcomes, it effectively mitigates the potential influence of confounding factors, enhancing the reliability of study results (16, 17). This study aims to use MR methods to delve into whether smoking, alcohol consumption, and BMI mediate the causal effects of insomnia on MI, providing a scientific basis for developing more effective cardiovascular health interventions. Through this research, we hope to gain a more comprehensive understanding of the intricate relationship between lifestyle and cardiac health, offering more precise guidance for the prevention and treatment of MI.
2 Materials and methods
2.1 Data sources
The study exclusively involved individuals of European ancestry as other ancestral groups were not universally available for all features of interest, and due to genetic population structure, the mixing of ancestries could introduce confounding. Genetic associations related to insomnia come from the UK Biobank and 23andMe (18), with this analysis incorporating a large sample of 1,207,228 individuals of European ancestry, making it one of the largest genome-wide association study (GWAS) studies on insomnia to date. To explore the genetic associations with MI, we acquired data from another GWAS analysis, encompassing 395,795 participants and 10,290,368 SNPs (19). Genetic instruments for smoking initiation and alcohol consumption are derived from statistical data in a large-scale publicly available genome-wide association meta-analysis (20). In this study, a standardized analysis was conducted across 34 individual cohorts, considering adjustments for age, age squared, gender, and genetic ancestry principal components. The effects sizes of individual single nucleotide polymorphisms (SNPs) on smoking and alcohol consumption, along with their respective standard errors, were assessed. In addition, data related to BMI came from a GWAS involving 806,834 individuals of European ancestry (21). BMI values were obtained through measurements of standing height and weight during the initial assessment center visit. Table 1 presented a summary of the studied GWAS information.
2.2 Genetic instrument selection
In our MR analysis, we utilized SNPs as IVs to estimate the overall impact of insomnia on MI. When the genome-wide significance threshold was P < 5 × 10−8, SNPs associated with each exposure were selected as potential IVs. To ensure the independence of genetic variations used as IVs, we set the linkage disequilibrium threshold for grouping to R2 < 0.01, with a window size of 1,000 kb. If suitable replacement SNPs were not available, the SNP was discarded. Simultaneously, we set a minimum allele frequency (MAF) of 0.3 to ensure SNP commonality (22, 23). Additionally, we standardized genetic variations from various studies based on their effects, excluding any palindromic variations. To assess the strength of the instruments, we calculated the F-statistic. We consider an F-statistic greater than 10 as an indicator of robust instrument strength. The F-statistic was calculated using the formula ((N-K-1)/K × [R2/(1−R2)]), where R2 was the variance explained by each SNP for each exposure, N was the sample size of the exposure GWAS, K was the number of SNPs, and the R2 formula was expressed as R2 = 2 × MAF × (1 − MAF) × [β2/(SE2 × N)], where MAF represented the minor allele frequency, β was the effect size of the exposure, and SE denoted the standard error of the effect size (24).
2.3 Statistical analysis
2.3.1 Univariate MR analysis
In our study, we employed the Inverse Variance Weighting (IVW) method as the primary approach for univariate analysis. This technique utilized a random-effects meta-analysis approach to combine results obtained from individual SNPs. To ensure consistency in the causal direction, we also conducted additional analyses using other methods: MR Egger, Weighted Median, Weighted Mode, and Simple Mode. To ensure the reliability of the results, significance in the IVW method results was required, and the results from the other four methods should be directionally consistent with the IVW results. To control for the type I error rate, the Benjamini–Hochberg method was used to adjust for multiple testing. The false discovery rate (FDR) threshold was set at 0.05 for significance. After the MR analysis, Cochran's Q test was used to assess the heterogeneity of the ratio estimates of the IVs related to the exposure on the outcome risk (25). When test results indicated heterogeneity (P < 0.05), we introduced the MR-PRESSO method to remove IVs with heterogeneity and then reanalyzed those not identified as heterogeneous IVs (26). We also employed the MR-Egger intercept method to assess evidence of horizontal pleiotropy within selected SNPs (the presence of horizontal pleiotropy was speculated for P < 0.05).
2.3.2 Multivariable MR analysis
In our study, we utilized multivariable MR to investigate the causal relationship between insomnia and MI risk, with smoking initiation and BMI as mediators. The specific methods were follows: firstly, the estimated value of the mediating effect of insomnia (a in Figure 1B) was multiplied by the estimated value of the mediating effect on MI (b in Figure 1B) to obtain the estimate of the indirect effect. Then, the indirect effect was divided by the total causal effect of insomnia on MI (c in Figure 1A) to obtain the proportion of mediation. In the presence of multiple mediators, the difference method was employed, subtracting the direct effect from the total causal effect (c’ in Figure 1C) to obtain the indirect effect. Then, we further divide the indirect effect by the total causal effect to determine the proportion of mediated mediation.
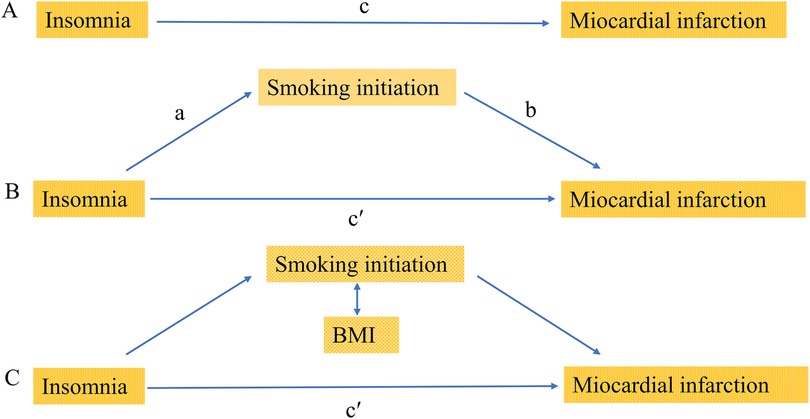
Figure 1. Diagrams illustrating associations examined in this study. (A) The total effect of insomnia on miocardial infarction (MI), c, was derived using univariable MR (i.e. genetically predicted insomnia as exposure and miocardial infarction as outcome). (B) The total effect was decomposed into: (i) indirect effect using a two-step approach (where a is the total effect of insomnia on smoking initiation, and b is the effect of smoking initiation on MI) and the product method (a × b) and (ii) direct effect (c′ = c − a × b). The same process applied to mediation analysis of body mass index (BMI). (C) For mediation by both smoking initiation and BMI combined (arrows represent their bidirectional causal relationship), the indirect effect was derived using the difference method (c − c′). Proportion mediated was the indirect effect divided by the total effect.
All statistical analyses in this study were conducted using the “TwoSampleMR” package in R software (version 4.1.0). All presented P-values were two-sided, and statistical significance was set at the 5% level. We adhered to the guidance of the STROBE-MR guidelines when reporting MR studies (27).
3 Results
3.1 Instrumental variables
Summary data of SNP-phenotype associations were obtained from GWAS for each phenotype (Supplementary Tables S1–S4). A total of 429 SNPs were chosen as IVs for insomnia, with an F-statistic of 19.87. For smoking initiation, alcohol consumption, and BMI, we extracted 77 SNPs (F = 18.33), 34 SNPs (F = 23.38), and 60 SNPs (F = 24.48), respectively, ensuring the absence of weak IVs.
3.2 Effects of insomnia and potential mediators on MI
In the univariate mendelian randomization (UVMR) analysis, the risk of MI increased by 96% in patients with insomnia, with an odds ratio (OR) of 1.96 (95% CI: 1.67, 2.31). For the three potential mediators analyzed, alcohol consumption was not associated with MI (OR = 0.89; 95% CI: 0.72, 1.12). The unadjusted associations of smoking initiation and BMI with MI were 0.73 (95% CI: 0.67–0.80) and 1.48 (95% CI: 1.32–1.66), respectively. The impact of insomnia on smoking initiation was (OR = 0.53; 95% CI: 0.46, 0.62), as well as on alcohol consumption (OR = 0.95; 95% CI: 0.91, 1.00) and BMI (OR = 1.30; 95% CI: 1.15, 1.48). These findings were depicted in Figure 2.
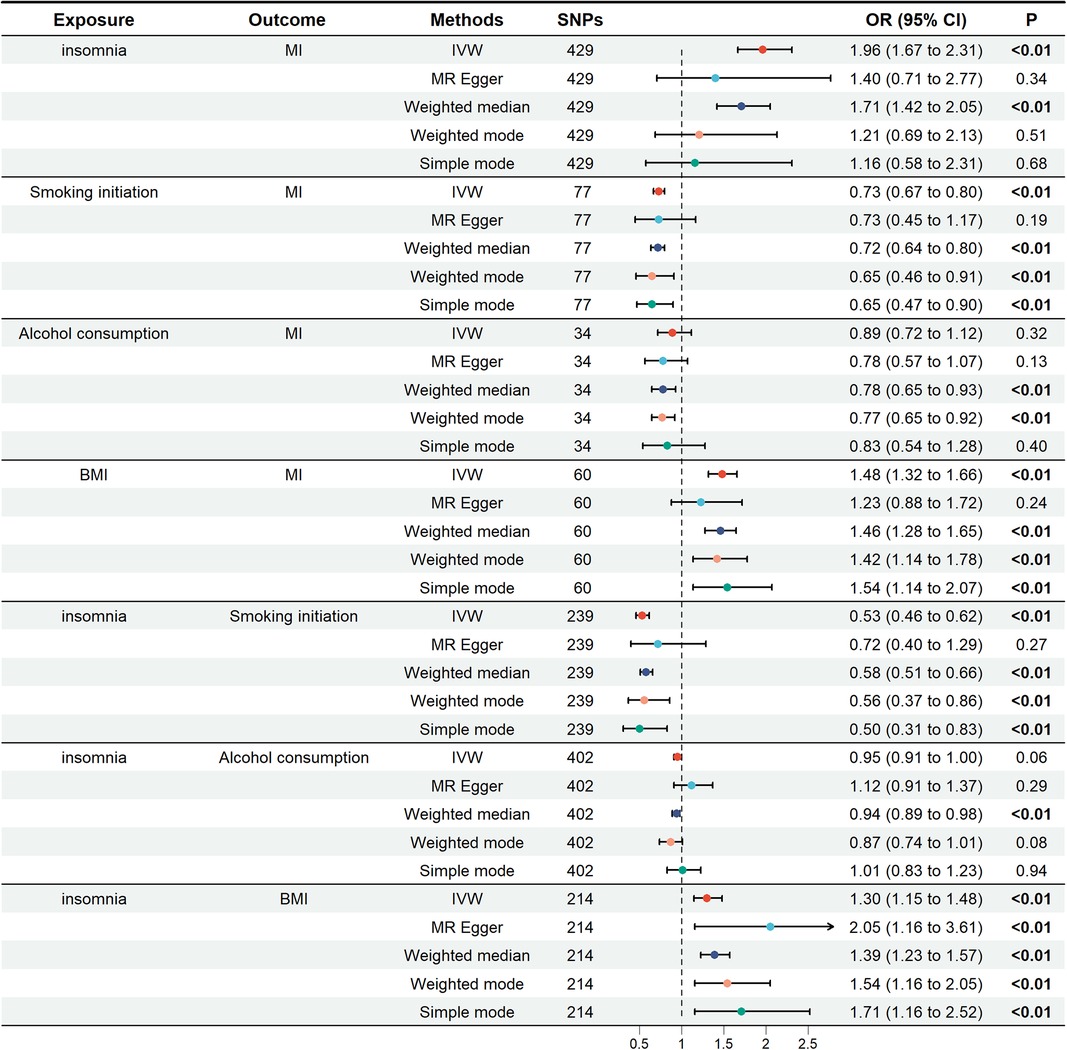
Figure 2. Univariate Mendelian randomization analysis. The figure showed the impact of insomnia and potential mediators on MI, as well as the effects of insomnia on potential mediators. Potential mediators include smoking initiation, alcohol consumption and BMI. MI, miocardial infarction; BMI, body mass index; SNPs, single nucleotide polymorphisms; OR, odds ratio; IVW, inverse variance weighting.
3.3 Mediation analysis
In the UVMR analysis, alcohol consumption had no effect on MI and was therefore excluded from subsequent multivariable mendelian randomization (MVMR) analysis. When screening potential mediators, we constructed three models, each adjusting for different mediators, as shown in Figure 3. Consistent results indicated a direct impact of insomnia on MI, with no evidence of complete mediation. In Model 1, the adjusted causal effect of smoking initiation on MI was OR = 0.76 (95% CI: 0.69, 0.85). In Model 2, the adjusted causal effect of BMI on MI was OR = 1.34 (95% CI: 1.18, 1.51). In Model 3, the adjusted causal effects of smoking initiation and BMI on MI were OR = 0.83 (95% CI: 0.73, 0.94) and OR = 1.39 (95% CI: 1.22, 1.58), respectively.
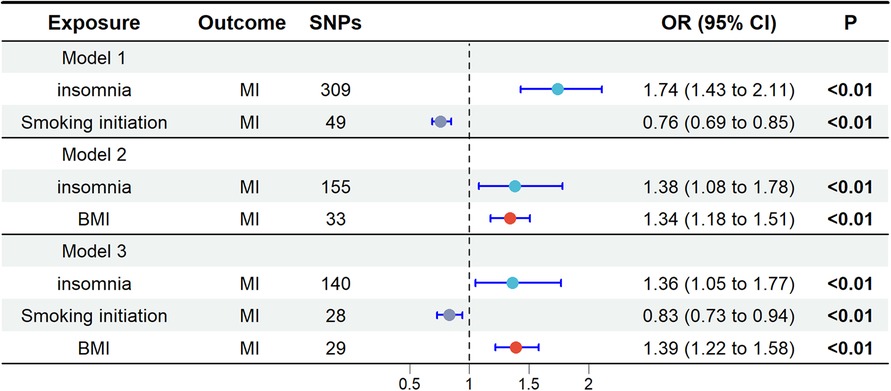
Figure 3. Multivariate Mendelian randomization analysis. The OR is derived from the method of inverse variance weighting. BMI, body mass index; MI, miocardial infarction; OR, odds ratio; SNPs, single nucleotide polymorphisms.
In the MR analysis, the total effect of insomnia on MI was 0.674 (95% CI: 0.512, 0.836). The direct effect in the MR analysis was 0.309 (95% CI: 0.045, 0.573). Mediation analysis showed that in the MR study, smoking initiation accounted for 29% of the total effect (95% CI: 13%, 61%), while BMI accounted for 15% (95% CI: 7%, 27%), with a combined mediation proportion of 54% (95% CI: 31%, 91%) see Table 2 for details.
3.4 Sensitivity analysis
In the MR analysis, heterogeneity tests revealed significant heterogeneity among the selected IVs (P < 0.05). Given this, the IVW random-effects model was used in all MR analyses (Table 3). MR-Egger_intercept analyses did not detect potential horizontal pleiotropy (P > 0.05), indicating that IVs did not significantly affect the outcome through pathways outside the exposure (Table 4).
4 Discussion
Our study results support a potential causal relationship between genetically predicted insomnia and MI risk. Compared to the general population, insomnia patients have a 96% increased risk of MI. Of this impact, 29% is mediated by smoking, and 15% is mediated by BMI. These two major risk factors for MI account for 54% of the total effect of insomnia. To our knowledge, this is the first application of MR mediation analysis to study mediators of insomnia and MI risk.
Our study results were consistent with previous study on the impact of insomnia on MI. Hu et al.'s meta-analysis found that in individuals with no prior MI, insomnia was associated with a higher risk of MI (9). Similar results were also found in another meta-analysis (28). The study found that patients with insomnia, compared to healthy individuals, experience changes in the hypothalamic-pituitary-adrenal axis, with a significant increase in adrenocortical hormones and cortisol levels (29). Elevated cortisol was associated with MI, as evidenced by a significant increase in cortisol levels in patients with acute MI one month before the onset of the disease (30). Animal experiments suggest that chronic stress and elevated cortisol can accelerate atherosclerosis, potentially leading to MI (31). Insomnia patients, due to insufficient sleep, suffer from chronic stress and elevated cortisol, exacerbating their risk of MI.
The mediation analysis results emphasize that in the causal relationship between insomnia and MI, smoking and BMI serve as key intermediate variables. This finding was consistent with past research results, highlighting the close association between insomnia and higher rates of smoking and obesity, both identified as factors increasing the risk of MI (15, 32, 33). Smoking, as a behavioral habit, was not only closely associated with insomnia but has also been confirmed as an independent risk factor for MI (34). This suggests that insomnia may increase the risk of MI by promoting smoking behavior. Meanwhile, BMI, as an indicator of physical health, plays a crucial mediating role between insomnia and MI. There is an association between obesity and insomnia, and higher BMI has been confirmed to be closely related to heart disease (35). Therefore, insomnia may trigger complex and interwoven effects in the pathogenesis of MI by influencing smoking and BMI. The results of this study provide valuable insights for the management and prevention of heart disease. When formulating intervention strategies, it is essential to not only focus on insomnia itself but also comprehensively consider the associated behaviors and physiological factors, especially smoking and BMI. Taking these intermediate variables into account may contribute to the development of more effective measures to reduce MI risk.
Another interesting aspect of our study was the finding that smoking and BMI jointly mediate 54% of the impact of insomnia on MI, while there was still a portion of the impact that remains unexplained. It is well known that insomnia may trigger depression and emotional fluctuations, and depression itself is considered an independent risk factor for MI (36, 37). Under depressive states, biological changes may lead to an imbalance in hormone levels, such as increased release of cortisol and overactivity of the autonomic nervous system (38, 39). These physiological changes are closely related to the health of the cardiovascular system, thereby increasing the risk of MI. Furthermore, factors such as the triglyceride glucose index-waist circumference (40), hepatic steatosis index (41), plasma aldosterone concentration (42), and weight-adjusted-waist index (43) may also serve as potential risk factors for MI. These indices reflect metabolic and hormonal disturbances that can exacerbate cardiovascular issues, particularly in individuals experiencing insomnia and depression. Additionally, depression may lead to unhealthy lifestyle choices, such as lack of exercise and poor dietary habits, further aggravating heart health issues. Therefore, insomnia indirectly influences the risk of MI by triggering depression, highlighting the intricate relationship between sleep, mental health, biology, and heart disease. Consequently, these non-lifestyle factors deserve further investigation as mediators of the impact of insomnia on MI.
This study has several notable strengths. Firstly, despite previous MR studies on insomnia and MI (44, 45), we are the first to use the mediation MR method to study the causal relationship between insomnia and MI and discover the mediating roles of smoking and BMI. Secondly, we utilized summary-level data from recent extensive sample studies in GWAS, covering a more comprehensive set of SNPs, thereby enhancing the statistical power of the data. Finally, employing the mediation MR method helped reduce biases caused by confounding between exposure factors, mediators, outcomes, and measurement errors.
However, we should not overlook several limitations. Firstly, although smoking and BMI largely explain the impact of insomnia on the risk of MI, the remaining portion of the effect still needs further exploration. Secondly, despite some heterogeneity in this study, our data came from public databases, and specific factor sub-analyses (such as age, gender, etc.) to explore the source of heterogeneity were not feasible. Additionally, the overlap in samples from the UK Biobank for both insomnia and MI may also impact our findings, but quantifying this overlap was not possible due to data source limitations. Future research on the correlation between insomnia and MI should consider the overall impact of these factors. Finally, GWAS summary data primarily came from European populations, so there may be some potential biases if extrapolated to non-European ancestries. These limitations emphasize the need for further research to refine the evidence in this field.
5 Conclusions
In summary, we demonstrated the hazardous effect of insomnia on the risk of MI. Quitting smoking and losing weight may help reduce this risk, especially crucial for those at higher risk of MI. However, nearly half of the impact of insomnia on MI remains difficult to explain. To comprehensively reduce the risk, efforts to improve lifestyle habits need to be strengthened, and further study is required to investigate other potential influencing factors not controlled by lifestyle factors.
Data availability statement
The datasets presented in this study can be found in online repositories. The names of the repository/repositories and accession number(s) can be found in the article/Supplementary Material.
Ethics statement
The studies involving humans were approved by Ethics Committee of the Genome-Wide Association Studies. The studies were conducted in accordance with the local legislation and institutional requirements. The participants provided their written informed consent to participate in this study.
Author contributions
LD: Conceptualization, Data curation, Writing – original draft, Writing – review & editing. YG: Data curation, Methodology, Writing – original draft. DW: Formal Analysis, Investigation, Software, Writing – review & editing. ZD: Conceptualization, Formal Analysis, Investigation, Writing – review & editing. YS: Data curation, Investigation, Methodology, Writing – review & editing. JG: Investigation, Visualization, Writing – original draft. WZ: Conceptualization, Formal Analysis, Visualization, Writing – original draft. QX: Funding acquisition, Project administration, Supervision, Writing – original draft, Writing – review & editing.
Funding
The author(s) declare financial support was received for the research, authorship, and/or publication of this article. This work was funded by Scientific Research Project of traditional Chinese Medicine in Heilongjiang Province (ZHY2022-166).
Conflict of interest
The authors declare that the research was conducted in the absence of any commercial or financial association that could be construed as a potential conflict of interest.
Publisher's note
All claims expressed in this article are solely those of the authors and do not necessarily represent those of their affiliated organizations, or those of the publisher, the editors and the reviewers. Any product that may be evaluated in this article, or claim that may be made by its manufacturer, is not guaranteed or endorsed by the publisher.
Supplementary material
The Supplementary Material for this article can be found online at: https://www.frontiersin.org/articles/10.3389/fcvm.2024.1456918/full#supplementary-material
Abbreviations
MI, myocardial infarction; BMI, body mass index; MR, Mendelian randomized; IV, instrumental variable; GWAS, Genome-wide association studies; SNP, single nucleotide polymorphism; MAF, minimum allele frequency; IVW, inverse variance weighting; UVMR, univariate mendelian randomization; MVMR, multivariable mendelian randomization; OR, odds ratio; CI, confidence interval; β regression coefficient; SE, standard error; FDR, false discovery rate.
References
1. Lindahl B, Baron T, Albertucci M, Prati F. Myocardial infarction with non-obstructive coronary artery disease. EuroIntervention. (2021) 17(11):e875–e87. doi: 10.4244/EIJ-D-21-00426
2. Li S, Gao X, Yang J, Xu H, Wang Y, Zhao Y, et al. Number of standard modifiable risk factors and mortality in patients with first-presentation ST-segment elevation myocardial infarction: insights from China acute myocardial infarction registry. BMC Med. (2022) 20(1):217. doi: 10.1186/s12916-022-02418-w
3. Liu X, Song Q, Wu S, Zhou W, Wang X. Prediabetes and risk for myocardial infarction by hypertension status in a Chinese population: a prospective cohort study. J Hypertens. (2021) 39(1):77–83. doi: 10.1097/HJH.0000000000002607
4. Chen P, Zhang M, Zhang Y, Su X, Chen J, Xu B, et al. Economic burden of myocardial infarction combined with dyslipidemia. Front Public Health. (2021) 9:648172. doi: 10.3389/fpubh.2021.648172
5. Plakht Y, Elkis Hirsch Y, Shiyovich A, Abu Tailakh M, Liberty IF, Gilutz H. Heterogenicity of diabetes as a risk factor for all-cause mortality after acute myocardial infarction: age and sex impact. Diabetes Res Clin Pract. (2021) 182:109117. doi: 10.1016/j.diabres.2021.109117
6. Darchia N, Oniani N, Sakhelashvili I, Supatashvili M, Basishvili T, Eliozishvili M, et al. Relationship between sleep disorders and health related quality of life-results from the Georgia SOMNUS study. Int J Environ Res Public Health. (2018) 15(8):1588. doi: 10.3390/ijerph15081588
8. He Q, Zhang P, Li G, Dai H, Shi J. The association between insomnia symptoms and risk of cardio-cerebral vascular events: a meta-analysis of prospective cohort studies. Eur J Prev Cardiol. (2017) 24(10):1071–82. doi: 10.1177/2047487317702043
9. Hu S, Lan T, Wang Y, Ren L. Individual insomnia symptom and increased hazard risk of cardiocerebral vascular diseases: a meta-analysis. Front Psychiatry. (2021) 12:654719. doi: 10.3389/fpsyt.2021.654719
10. Hagg SA, Ljunggren M, Janson C, Holm M, Franklin KA, Gislason T, et al. Smokers with insomnia symptoms are less likely to stop smoking. Respir Med. (2020) 170:106069. doi: 10.1016/j.rmed.2020.106069
11. Cohen A, Szepsenwol O, Haimov I. Insomnia severity mediates the association between COVID-19 related anxiety and increase in tobacco smoking during the COVID-19 pandemic among adults. Behav Sleep Med. (2023) 21(5):608–19. doi: 10.1080/15402002.2022.2147934
12. Verlinden JJ, Moloney ME, Whitehurst LN, Weafer J. Sex differences in the association between poor sleep quality and alcohol-related problems among heavy drinkers with insomnia. Front Behav Neurosci. (2022) 16:875168. doi: 10.3389/fnbeh.2022.875168
13. Miller MB, Carpenter RW, Freeman LK, Dunsiger S, McGeary JE, Borsari B, et al. Effect of cognitive behavioral therapy for insomnia on alcohol treatment outcomes among US veterans: a randomized clinical trial. JAMA Psychiatry. (2023) 80(9):905–13. doi: 10.1001/jamapsychiatry.2023.1971
14. Kauffman BY, Kotov R, Garey L, Ruggero CJ, Luft BJ, Zvolensky MJ. The association between body mass Index and anxious arousal, depressive, and insomnia symptoms among world trade center responders. Health Behav Res. (2022) 5(1):3. doi: 10.4148/2572-1836.1107
15. Johnson KA, Gordon CJ, Chapman JL, Hoyos CM, Marshall NS, Miller CB, et al. The association of insomnia disorder characterised by objective short sleep duration with hypertension, diabetes and body mass index: a systematic review and meta-analysis. Sleep Med Rev. (2021) 59:101456. doi: 10.1016/j.smrv.2021.101456
16. Zhao SS, Mackie SL, Zheng J. Why clinicians should know about Mendelian randomization. Rheumatology (Oxford). (2021) 60(4):1577–9. doi: 10.1093/rheumatology/keab007
17. Davies NM, Holmes MV, Davey Smith G. Reading Mendelian randomisation studies: a guide, glossary, and checklist for clinicians. Br Med J. (2018) 362:k601. doi: 10.1136/bmj.k601
18. Watanabe K, Jansen PR, Savage JE, Nandakumar P, Wang X, Me Research T, et al. Genome-wide meta-analysis of insomnia prioritizes genes associated with metabolic and psychiatric pathways. Nat Genet. (2022) 54(8):1125–32. doi: 10.1038/s41588-022-01124-w
19. Hartiala JA, Han Y, Jia Q, Hilser JR, Huang P, Gukasyan J, et al. Genome-wide analysis identifies novel susceptibility loci for myocardial infarction. Eur Heart J. (2021) 42(9):919–33. doi: 10.1093/eurheartj/ehaa1040
20. Liu M, Jiang Y, Wedow R, Li Y, Brazel DM, Chen F, et al. Association studies of up to 1.2 million individuals yield new insights into the genetic etiology of tobacco and alcohol use. Nat Genet. (2019) 51(2):237–44. doi: 10.1038/s41588-018-0307-5
21. Pulit SL, Stoneman C, Morris AP, Wood AR, Glastonbury CA, Tyrrell J, et al. Meta-analysis of genome-wide association studies for body fat distribution in 694 649 individuals of European ancestry. Hum Mol Genet. (2019) 28(1):166–74. doi: 10.1093/hmg/ddy327
22. Lu W, Pu B, Wang S, Li M, An Y, Lian J, et al. Bidirectional two-sample Mendelian randomization analysis identifies causal associations between cardiovascular diseases and frozen shoulder. J Orthop Surg Res. (2024) 19(1):116. doi: 10.1186/s13018-024-04600-7
23. Pu B, Gu P, Luo L, Yue D, Xin Q, Zeng Z, et al. Causal effects of tea intake on multiple types of fractures: a two-sample Mendelian randomization study. Medicine (Baltimore). (2023) 102(22):e33542. doi: 10.1097/MD.0000000000033542
24. Burgess S, Thompson SG, Collaboration CCG. Avoiding bias from weak instruments in Mendelian randomization studies. Int J Epidemiol. (2011) 40(3):755–64. doi: 10.1093/ije/dyr036
25. Bowden J, Del Greco MF, Minelli C, Zhao Q, Lawlor DA, Sheehan NA, et al. Improving the accuracy of two-sample summary-data Mendelian randomization: moving beyond the NOME assumption. Int J Epidemiol. (2019) 48(3):728–42. doi: 10.1093/ije/dyy258
26. Verbanck M, Chen CY, Neale B, Do R. Detection of widespread horizontal pleiotropy in causal relationships inferred from Mendelian randomization between complex traits and diseases. Nat Genet. (2018) 50(5):693–8. doi: 10.1038/s41588-018-0099-7
27. Skrivankova VW, Richmond RC, Woolf BAR, Yarmolinsky J, Davies NM, Swanson SA, et al. Strengthening the reporting of observational studies in epidemiology using Mendelian randomization: the STROBE-MR statement. JAMA. (2021) 326(16):1614–21. doi: 10.1001/jama.2021.18236
28. Dean YE, Shebl MA, Rouzan SS, Bamousa BAA, Talat NE, Ansari SA, et al. Association between insomnia and the incidence of myocardial infarction: a systematic review and meta-analysis. Clin Cardiol. (2023) 46(4):376–85. doi: 10.1002/clc.23984
29. Vgontzas AN, Tsigos C, Bixler EO, Stratakis CA, Zachman K, Kales A, et al. Chronic insomnia and activity of the stress system: a preliminary study. J Psychosom Res. (1998) 45(1):21–31. doi: 10.1016/S0022-3999(97)00302-4
30. Faresjo T, Stromberg S, Jones M, Stomby A, Karlsson JE, Ostgren CJ, et al. Elevated levels of cortisol in hair precede acute myocardial infarction. Sci Rep. (2020) 10(1):22456. doi: 10.1038/s41598-020-80559-9
31. Okutsu M, Lira VA, Higashida K, Peake J, Higuchi M, Suzuki K. Corticosterone accelerates atherosclerosis in the apolipoprotein E-deficient mouse. Atherosclerosis. (2014) 232(2):414–9. doi: 10.1016/j.atherosclerosis.2013.11.076
32. Laugsand LE, Vatten LJ, Platou C, Janszky I. Insomnia and the risk of acute myocardial infarction: a population study. Circulation. (2011) 124(19):2073–81. doi: 10.1161/CIRCULATIONAHA.111.025858
33. Hu N, Wang C, Liao Y, Dai Q, Cao S. Smoking and incidence of insomnia: a systematic review and meta-analysis of cohort studies. Public Health. (2021) 198:324–31. doi: 10.1016/j.puhe.2021.07.012
34. Chen LJ, Steptoe A, Chen YH, Ku PW, Lin CH. Physical activity, smoking, and the incidence of clinically diagnosed insomnia. Sleep Med. (2017) 30:189–94. doi: 10.1016/j.sleep.2016.06.040
35. Yoshida N, Ogawa M, Nakai M, Kanaoka K, Sumita Y, Emoto T, et al. Impact of body mass index on in-hospital mortality for six acute cardiovascular diseases in Japan. Sci Rep. (2022) 12(1):18934. doi: 10.1038/s41598-022-23354-y
36. Aw PY, Pang XZ, Wee CF, Tan NHW, Peck EW, Teo YN, et al. Co-prevalence and incidence of myocardial infarction and/or stroke in patients with depression and/or anxiety: a systematic review and meta-analysis. J Psychosom Res. (2023) 165:111141. doi: 10.1016/j.jpsychores.2022.111141
37. Wang M, Cheng L, Gao Z, Li J, Ding Y, Shi R, et al. Investigation of the shared molecular mechanisms and hub genes between myocardial infarction and depression. Front Cardiovasc Med. (2023) 10:1203168. doi: 10.3389/fcvm.2023.1203168
38. Druzhkova TA, Yakovlev AA, Rider FK, Zinchuk MS, Guekht AB, Gulyaeva NV. Elevated Serum cortisol levels in patients with focal epilepsy, depression, and comorbid epilepsy and depression. Int J Mol Sci. (2022) 23(18):10414. doi: 10.3390/ijms231810414
39. Teo CH, Wong ACH, Sivakumaran RN, Parhar I, Soga T. Gender differences in cortisol and cortisol receptors in depression: a narrative review. Int J Mol Sci. (2023) 24(8):7129. doi: 10.3390/ijms24087129
40. Hu J, Cai X, Li N, Zhu Q, Wen W, Hong J, et al. Association between triglyceride glucose index-waist circumference and risk of first myocardial infarction in Chinese hypertensive patients with obstructive sleep apnoea: an observational cohort study. Nat Sci Sleep. (2022) 14:969–80. doi: 10.2147/NSS.S362101
41. Wen W, Cai X, Zhu Q, Hu J, Hong J, Zhang X, et al. Linear relationship between hepatic steatosis Index and Major adverse cardiovascular events in hypertensive patients with obstructive sleep apnea: a real-world cohort study from China. Rev Cardiovasc Med. (2023) 24(10):280. doi: 10.31083/j.rcm2410280
42. Cai X, Song S, Hu J, Zhu Q, Shen D, Yang W, et al. Association of the trajectory of plasma aldosterone concentration with the risk of cardiovascular disease in patients with hypertension: a cohort study. Sci Rep. (2024) 14(1):4906. doi: 10.1038/s41598-024-54971-4
43. Zhao J, Cai X, Hu J, Song S, Zhu Q, Shen D, et al. J-Shaped relationship between weight-adjusted-Waist Index and cardiovascular disease risk in hypertensive patients with obstructive sleep apnea: a cohort study. Diabetes Metab Syndr Obes. (2024) 17:2671–81. doi: 10.2147/DMSO.S469376
44. Yang Y, Fan J, Shi X, Wang Y, Yang C, Lian J, et al. Causal associations between sleep traits and four cardiac diseases: a Mendelian randomization study. ESC Heart Fail. (2022) 9(5):3160–6. doi: 10.1002/ehf2.14016
Keywords: insomnia, myocardial infarction, smoking, body mass index, alcohol consumption, Mendelian randomization
Citation: Deng L, Gao Y, Wan D, Dong Z, Shao Y, Gao J, Zhai W and Xu Q (2024) Genetically predicted smoking and body mass index mediate the relationship between insomnia and myocardial infarction. Front. Cardiovasc. Med. 11:1456918. doi: 10.3389/fcvm.2024.1456918
Received: 29 June 2024; Accepted: 30 October 2024;
Published: 13 November 2024.
Edited by:
Panagiota Anyfanti, Aristotle University of Thessaloniki, GreeceReviewed by:
Xinpei Wang, The University of Chicago, United StatesXintian Cai, People’s Hospital of Xinjiang Uygur Autonomous Region, China
Copyright: © 2024 Deng, Gao, Wan, Dong, Shao, Gao, Zhai and Xu. This is an open-access article distributed under the terms of the Creative Commons Attribution License (CC BY). The use, distribution or reproduction in other forums is permitted, provided the original author(s) and the copyright owner(s) are credited and that the original publication in this journal is cited, in accordance with accepted academic practice. No use, distribution or reproduction is permitted which does not comply with these terms.
*Correspondence: Qian Xu, dz13256916857@163.com
†These authors have contributed equally to this work and share first authorship