- 1Center for Evidence Based Chinese Medicine, Institute of Basic Research in Clinical Medicine, China Academy of Chinese Medical Sciences, Beijing, China
- 2Department of Clinical Epidemiology, Beijing Institute of Respiratory Medicine and Beijing Chao-Yang Hospital, Capital Medical University, Beijing, China
- 3Beijing Institute of Traditional Chinese Medicine, Beijing Hospital of Traditional Chinese Medicine, Capital Medical University, Beijing, China
Background: This study aimed to synthesize the recommendations for prediction models in cardiovascular clinical practice guidelines (CPGs) and assess the methodological quality of the relevant primary modeling studies.
Methods: We performed a systematic literature search of all available cardiovascular CPGs published between 2018 and 2023 that presented specific recommendations (whether in support or non-support) for at least one multivariable clinical prediction model. For the guideline-recommended models, the assessment of the methodological quality of their primary modeling studies was conducted using the Prediction model Risk Of Bias ASsessment Tool (PROBAST).
Results: In total, 46 qualified cardiovascular CPGs were included, with 69 prediction models and 80 specific recommendations. Of the 80 specific recommendations, 74 supported 57 models (53 were fully recommended and 4 were conditionally recommended) in cardiovascular practice with moderate to strong strength. Most of the guideline-recommended models were focused on predicting prognosis outcomes (53/57, 93%) in primary and tertiary prevention, focusing primarily on long-term risk stratification and prognosis management. A total of 10 conditions and 7 types of target population were involved in the 57 models, while heart failure (14/57, 25%) and a general population with or without cardiovascular risk factor(s) (12/57, 21%) received the most attention from the guidelines. The assessment of the methodological quality of 57 primary studies on the development of the guideline-recommended models revealed that only 40% of the modeling studies had a low risk of bias (ROB). The causes of high ROB were mainly in the analysis and participant domains.
Conclusions: Global cardiovascular CPGs presented an unduly positive appraisal of the existing prediction models in terms of ROB, leading to stronger recommendations than were warranted. Future cardiovascular practice may benefit from well-established clinical prediction models with better methodological quality and extensive external validation.
1 Introduction
Cardiovascular diseases (CVDs) are major causes of mortality and a leading contributor to disability globally with the burden of disease continuing its decades-long rise in almost all countries (1, 2). In the United States of America, the estimated cost due to CVD for healthcare services, medications, and lost productivity was over $200 billion annually (3). This growing burden of CVD can be broadly attributed to the suboptimal implementation of prevention strategies and poor management of cardiovascular risk factors (3, 4). Hence, the assessment of CVD risk in the general population, risk stratification in suspected patients, and prevention of recurrence in patients suffering from CVD represent an opportunity for major public health gains (1, 5).
Multivariable clinical prediction models (also known as risk prediction/assessment tools or prediction rules) are usually developed based on person-level information, aiming to estimate the pretest probability or risk of an individual having (diagnosis) or developing (prognosis) a particular outcome (6–8). As they play an increasingly vital role in the screening, diagnosis, and prognosis management of conditions, prediction models have gradually received increased attention from healthcare service providers and creators of clinical practice guidelines (CPGs), especially in the cardiovascular domain (9, 10). Beyond their clinical utility, their economic value has also been demonstrated in a considerable number of pharmacoeconomics analyses (11). Currently, quite a few available prediction models have been recommended for clinical practice in cardiovascular CPGs, e.g., there are 14 models recommended for the primary and tertiary prevention of heart failure (HF) in the 2022 American Heart Association (AHA)/American College of Cardiology (ACC)/Heart Failure Society of America (HFSA) Guideline for the Management of HF (12). Furthermore, other well-known models, e.g., the Framingham Risk Score (FRS), Systematic Coronary Risk Estimation 2 (SCORE2), Pooled Cohort Equations (PCE), and the QRISK3 tool, are widely recommended for the assessment of 10-year CVD risk in European and American populations with or without risk factor(s) (13–16).
Despite the promising application prospects of the prediction models, the methodological quality of the primary studies on the development of the models has been roundly criticized in recent years. In the different medical domains, almost all the primary modeling studies are at high risk of bias (ROB), whether the models were developed based on traditional regression methods (e.g., Logistic regression) or emerging artificial intelligence (AI) technology (e.g., machine learning algorithms) (17–20). The same is true without discrepancy in the cardiovascular domain (21–23). Given the enormous impact of CPGs on clinical practice (24), an assessment of the methodological quality of primary modeling studies related to guideline-recommended models appears to be necessary.
Prior reports mainly reviewed and summarized several prediction models for cardiovascular risk classification in primary prevention, with limitations in their application to other clinical settings and target populations/conditions (10, 15, 25). To date, the recommendations for prediction models in cardiovascular practice guidelines have not been adequately synthesized, nor has methodological quality assessment been systematically conducted for primary modeling studies. Thus, we performed a systematic literature search aiming to (1) identify and provide an overview of the recommendations for prediction models in cardiovascular CPGs, (2) summarize the characteristics of the involved models in clinical practice, and (3) assess the ROB of the primary studies on the development of guideline-recommended models.
2 Materials and methods
This study was reported following the Preferred Reporting Items for Systematic Review and Meta-analysis (PRISMA) (Supplementary Table 1) (26).
2.1 Identification of eligible guidelines
We focused on searching the CPGs that recommend any CVD prediction models for clinical practice. Relevant guidelines published in English were searched in the following electronic databases on 28 December 2023: Medline (PubMed), EMBASE, and Web of Science. Considering the impact of the timeliness of the CPGs on their clinical utility, the publication date was restricted to after 2018. The search strategy was developed by a combination of Medical Subject Heading terms as well as text words related to the concepts of “cardiovascular diseases” and “practice guideline” (Supplementary Table 2). Furthermore, the following websites of governments or organizations were used for the supplementary search: World Health Organization (WHO, https://www.who.int/zh/publications), Guidelines International Network (GIN, https://g-i-n.net/), National Institute for Health and Clinical Excellence (NICE, https://www.nice.org.uk/), Scottish Intercollegiate Guidelines Network (SIGN, https://www.sign.ac.uk/), and Canadian Medical Association: Clinical Practice Guideline (CMACPG, https://www.cma.ca/). In addition, we also examined the references of the documents obtained through the aforementioned search strategies to further discover potentially eligible CPGs.
Eligible publications needed to provide a specific recommendation (whether in support or non-support) for at least one multivariable clinical prediction model that was defined as any combination of two or more predictors (variables, features) for estimating the probability or risk of an individual having (diagnosis) or developing (prognosis) a particular outcome in the cardiovascular domain. Guidelines were excluded if they were ongoing, publicly unpublished, or unavailable for full text. In addition, the older versions of guidelines developed by the same academic organization were also excluded in the screening stage. The procedure for identifying eligible guidelines was conducted according to the PRISMA flow diagram (27).
2.2 Screening process
The literature search was conducted by one reviewer (C-yJ) after consulting an experienced librarian who also assisted in developing the search strategy. All the search results were imported into Endnote V20.6 to manage the records and screen the eligible CPGs. Two reviewers (C-yJ and LZ) independently screened the titles and abstracts of all the available records. A list of potentially eligible publications was generated for the retrieval of the full texts. Thereafter, the same reviewers independently continued to assess the eligibility of these publications and determined the final included guidelines. The reasons for the exclusion of records were required to be noted. Throughout the process, any disagreement between the two reviewers was resolved by discussion until a consensus was reached or by consulting with an experienced third reviewer (XL).
While screening the guidelines, the primary studies on the development of the guideline-recommended prediction models were further identified through an examination of the references, and the full texts of the relevant studies were obtained by manual retrieval to gather the model details.
2.3 Data collection and synthesis
Based on our study objectives, we developed a data extraction form for the standard data collection procedure. The extraction form contained four sections: (1) general information of the guideline, including name, year developed or updated, country, location, and publication organization(s); (2) prediction model recommendations, including recommended model, overview of recommendations, classification of recommendations, level or quality of evidence, and criteria for recommendation; (3) characteristics of prediction model, including presentation, setting, predicted outcome(s), outcome type and target population or condition (Supplementary Table 3). A critical appraisal was conducted on all the recommendations in the CPGs and a narrative synthesis was performed to identify themes based on the extracted data.
2.4 Risk of bias assessment
For the guideline-recommended models, we used the Prediction model Risk Of Bias ASsessment Tool (PROBAST) to assess the ROB of the primary modeling studies (28, 29). PROBAST contains 20 signaling questions developed based on the Delphi method, which can assess the ROB according to four domains (participants, predictors, outcome, and analysis). The overall ROB was judged to be low, high, or unclear based on an assessment of all the signaling questions.
3 Results
A total of 15,296 potentially relevant records were identified from databases, 13,483 of which were screened based on titles and abstracts after removing 1,813 duplicates. We further identified 260 publications for final eligibility by assessing their full text. Of these, 37 guidelines (3, 12–14, 30–62) fulfill our predefined inclusion criteria while the other 223 publications were excluded for specific reasons (Supplementary Table 4). In addition, we included nine guidelines (16, 63–70) from 61 publications that were identified through the supplementary search. Figure 1 shows the PRISMA flow diagram for the entire CPG selection process.
3.1 Characteristics of the included CPGs
In the past 6 years, 46 cardiovascular CPGs with explicit recommendations for clinical prediction models were developed or updated globally. Except for one guideline that was developed by an international academic working group, the other 45 guidelines were published in Europe (19/45, 42%), North America (11/45, 24%), Asia (10/45, 22%), South America (3/45, 7%), and Oceania (2/45, 4%), encompassing 12 countries in total (Supplementary Table 5).
All of these CPGs were evidence-based guidelines developed based on six categories of criteria for forming recommendations. The Grading of Recommendations Assessment, Development and Evaluation (GRADE) approach and a modified GRADE approach (17/46, 37%) were most widely adopted for assessing the quality of evidence and grading recommendations, with the European Society of Cardiology (ESC) approach (15/46, 33%) and the American College of Cardiology Foundation (ACCF)/AHA approach (11/46, 24%) following closely. The American Physical Therapy Association (APTH) approach, European Society for Medical Oncology (ESMO) approach, and Traffic light signaling system-based classification were used only once (1/46, 2%), respectively. The details of all the criteria are summarized in Supplementary Table 6.
3.2 Overview of recommendations on prediction models
A total of 80 eligible recommendations and 69 relevant prediction models were identified and extracted from the included CPGs. An overview of the recommendations is given in Supplementary Table 7. Of the 80 recommendations, 74 (93%) supported the application of an appropriate prediction model in clinical practice, while the other 6 (7%) explicitly put forward a disapproving opinion of the specific model. Figure 2 synthesizes and visualizes the recommendation classifications of the 69 models based on different criteria. Thus, the involved models could be divided into three categories: (1) 53 fully recommended models (only mentioned in the supportive recommendations); (2) 4 conditionally recommended models (mentioned in both the supportive and non-supportive recommendations); and (3) 12 non-recommended models (only mentioned in the non-supportive recommendations). More importantly, for recommendations regarding the use of prognostic models, 10 CPGs recommended 16 prognostic models for further clinical decision-making or treatment selection besides risk assessment.
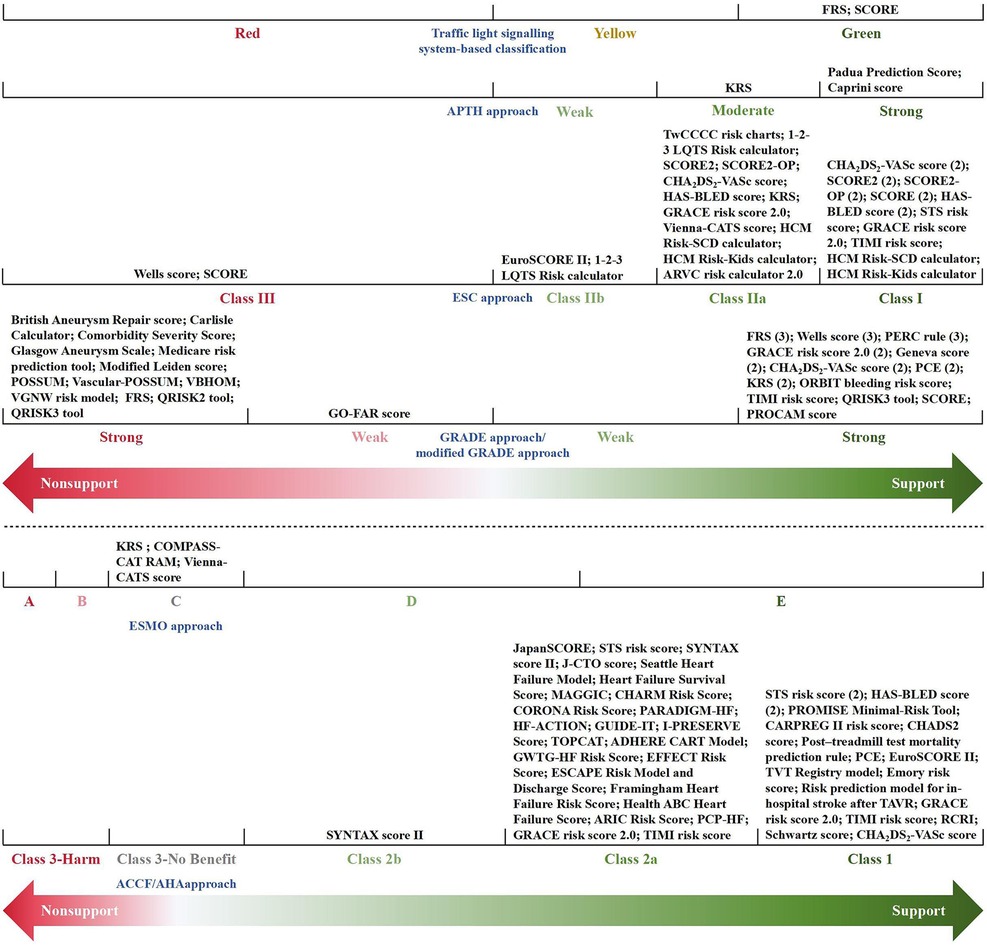
Figure 2. Classification of recommendations for prediction models. The numbers in parentheses represent the sum total of the guidelines with the same classification of recommendation for a specific model. AHA, aortic dissection risk score; ADHERE, acute decompensated heart failure national registry; ARIC, atherosclerosis risk in communities; ARVC, arrhythmogenic right ventricular cardiomyopathy; CARPREG, cardiac disease in pregnancy study; CART, classification and regression tree; CHADS2, congestive heart failure, hypertension, age >75 years, diabetes, stroke/transient ischemic attack; CHA2DS2-VASc, congestive heart failure, hypertension, age ≥75 years (2 points), diabetes mellitus, stroke (2 points)-vascular disease, age 65–74 years, sex category (female); CHARM, candesartan in heart failure-assessment of reduction in mortality and morbidity; COMPASS-CAT, prospective comparison of methods for thromboembolic risk assessment with clinical perceptions and awareness in real-life patients-cancer associated thrombosis; CORONA, controlled rosuvastatin multinational trial in heart failure; EFFECT, enhanced feedback for effective cardiac treatment; ESCAPE, evaluation study of congestive heart failure and pulmonary artery catheterization effectiveness; FRS, Framingham risk score; GO-FAR, good outcome following attempted resuscitation; GRACE, global registry of acute coronary events; GUIDE-IT, guiding evidence-based therapy using biomarker intensified treatment; GWTG-HF, get with the guidelines—heart failure; HAS-BLED, hypertension, abnormal renal/liver function, stroke, bleeding history or predisposition, labile international normalized ratio, elderly (>65 years), drugs/alcohol concomitantly; HCM, hypertrophic cardiomyopathy; HF, heart failure; HF-ACTION, heart failure: a controlled trial investigating outcomes of exercise training; HFSA, Heart Failure Society of America; I-PRESERVE, irbesartan in heart failure with preserved ejection fraction study; J-CTO, multicenter chronic total occlusion registry in Japan; KRS, Khorana risk score; LQTS, long QT syndrome; MAGGIC, meta-analysis global group in chronic heart failure; PARADIGM-HF, prospective comparison of ARNI with ACEI to determine impact on global mortality and morbidity in heart failure trial; PCE, pooled cohort equations; PCP-HF, pooled cohort equations to prevent HF; PERC, pulmonary embolism rule-out criteria; POSSUM, physiological and operative severity score for enumeration of mortality; PROCAM, prospective cardiovascular Münster; PROMISE, prospective multicenter imaging study for evaluation of chest pain; RAM, risk assessment model; RCRI, revised cardiac risk index; SCD, sudden cardiac death; SCORE, systematic coronary risk estimation; SCORE2, systematic coronary risk estimation 2; SCORE2-OP, systematic coronary risk estimation 2-older persons; STS, Society of Thoracic Surgeons; SYNTAX, synergy between percutaneous coronary intervention with TAXUS and cardiac surgery; TAVR, transcatheter aortic valve replacement; TIMI, thrombolysis in myocardial infarction; TOPCAT, treatment of preserved cardiac function heart failure with an aldosterone antagonist trial; TVT, transcatheter valve therapy; TwCCCC, Taiwan Chin-Shan community cardiovascular cohort; VBHOM, vascular biochemical and haematological outcome model; VGNW, vascular governance North West; Vienna-CATS, Vienna cancer and thrombosis study.
Due to different evidence-grading criteria, there was wide variation in the level/quality of evidence for supporting the application of a model. However, almost all guideline-recommended (both fully recommended and conditionally recommended) models had a moderate to strong strength of recommendation, of which the conditionally recommended models were discouraged for populations with specific known risk factors (e.g., diabetes mellitus and familial hypercholesterolemia). Meanwhile, based on the low to moderate levels of evidence, the guidelines discouraged the use of all of the non-recommended models in unsuited target populations (e.g., pregnant women with suspected deep vein thrombosis) or conditions (e.g., in-hospital cardiac arrest). Figure 3 summarizes the recommended and non-recommended target populations/conditions in clinical practice of the three categories of models.
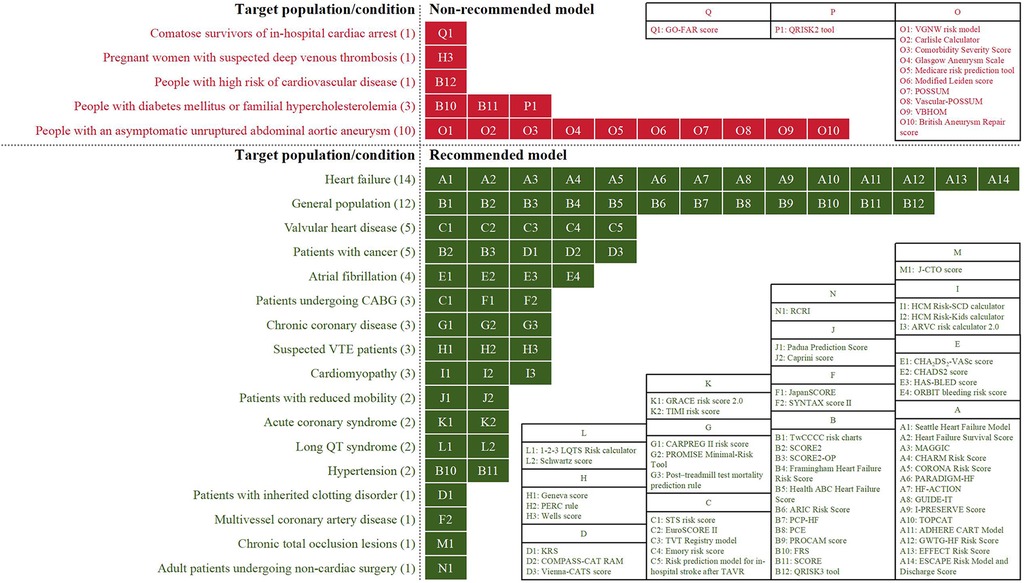
Figure 3. The recommended and non-recommended target populations/conditions in clinical practice of the prediction models.
3.3 Attributes of the guideline-recommended models in cardiovascular practice
Table 1 shows the attributes of the guideline-recommended models in cardiovascular practice. Almost all the recommended models were prognostic models (53/57, 93%) and were focused on primary (16/57, 28%) and tertiary prevention (37/57, 65%), while the remaining models (4/57, 7%) were diagnostic models and were focused on secondary prevention.
A total of 10 conditions and seven types of target population were involved in the 57 models, where HF (14/57, 25%) and a general population with or without cardiovascular risk factor(s) (12/57, 21%) had received the most attention from the guidelines. Other target conditions included valvular heart disease (5/57, 9%), atrial fibrillation (4/57, 7%), cardiomyopathy (3/57, 5%), chronic coronary disease (3/57, 5%), acute coronary syndrome (2/57, 4%), long QT syndrome (2/57, 4%), hypertension (2/57, 4%), multivessel coronary artery disease (1/57, 2%), and chronic total occlusion lesions (1/57, 2%). Suspected venous thromboembolism patients (3/57, 5%); patients who had undergone coronary artery bypass grafting (CABG) (3/57, 5%) or non-cardiac surgery (1/57, 2%); or patients with cancer (5/57, 9%), reduced mobility (2/57, 4%), or an inherited clotting disorder (1/57, 2%) were also target populations.
The predicted outcome of the recommended models was mostly concerned with the risk of mortality (23/57, 40%), followed by the risk of incident fatal or non-fatal CVD (8/57, 14%) and the risk of incident venous thromboembolism (5/57, 9%). Others mainly focused on predicting the risk of incident cardiovascular complications and evaluating the feasibility/necessity of the interventions under consideration. A point scoring system (29/57, 51%) was the most common presentation format of the prediction models for use in clinical settings, followed by a website calculator (14/57, 25%). A nomogram (2/57, 4%) or graphical score chart (1/57, 2%) was relatively rare. In addition, the presentation of eight (14%) models combined two of the above four formats, while the remaining three (5%) models were unclear.
3.4 Risk of bias assessment of the primary modeling studies
Through the references provided in the guidelines and from manual retrieval, we further identified 57 primary studies on the development of the guideline-recommended models (Supplementary Table 8). Of the 57 primary studies on the guideline-recommended prediction models, 23 (40%) studies were rated as having a low ROB, 3 (4%) as having an unclear ROB, and the remaining 31 (56%) studies had a high ROB after PROBAST analysis (Figures 4, 5). The details of the ROB assessment of 20 signaling questions are shown in Supplementary Table 9.
The causes of high ROB were mainly in the analysis domain as 23 (40%) studies inappropriately evaluated the calibration of the relevant models (e.g., ignored the calibration or evaluated the calibration using the Hosmer–Lemeshow test only) and 17 (30%) studies did not account for the fitting of their models (e.g., a lack of internal validation or the dataset was randomly split into only derivation and validation sets). Other reasons for a high ROB included incorrect handling in continuous/categorical predictors and improper variable selection (i.e., based only on univariable analysis). A non-prospective data source was the major cause of high ROB in the participant domain, resulting in six (11%) studies being rated as having a high ROB. This also led to an unclear ROB in the blinding of predictor or outcome assessment with regard to the reporting of relevant missing content in such studies. Beyond that, there was no information about handling missing data and consistency in reporting the predictor weighting in 11 (19%) and 8 (14%) studies, respectively, leading to an unclear ROB for the relevant signaling questions.
4 Discussion
In this global survey of 46 qualified cardiovascular CPGs involving 80 recommendations for 69 relevant prediction models from 2018 to 2023, we found that 74 recommendations supported 57 models in cardiovascular practice with moderate to strong strength. Most of the guideline-recommended models were focused on predicting prognosis outcomes in primary and tertiary prevention, mainly concentrating on long-term risk stratification and prognosis management. Moreover, a further assessment of the methodological quality of the primary studies on the development of the guideline-recommended models revealed that only 40% of the modeling studies had a low risk of bias.
The findings of this study indicate that prediction models have been generally recommended for cardiovascular practice in global CPGs, although their primary application was concentrated in developed countries in Europe and the Americas, geographically. Given the abundance of developed prediction models and the rapidly increasing number of similar modeling studies, how to evaluate and recommend models with genuine clinical utility still remains a major dilemma for guideline creators and methodologists. Indeed, a phenomenon—the overall level of evidence for the recommendations of moderate to strong strength was moderate or even lower—emerged in this study. It is common for a recommendation of strong strength to only be supported by consensus/expert opinion-level evidence (41, 46, 50, 52). This may be attributed to the stability (via internal validation) and generalizability (via external validation) of the validated models despite the paucity of high-level evidence (71).
With regards to evidence-based CPGs, evidence is the cornerstone of formulating recommendations, and high-level evidence is universally defined as results from high-quality randomized controlled trials (RCTs) or meta-analyses (24). However, when conducting RCTs or meta-analyses for prediction models that are treated as specific interventions, additional challenges need to be considered more carefully. First, the applicable study design for investigating the impact of models on cardiovascular practice is a cluster randomized trial (CRT) (72, 73). Compared to the conventional RCT design, a CRT requires a considerably larger sample size for a statistically significant effect size and this sometimes faces several particular medical ethical challenges, such as the plausibility of informed consent waivers and the possible neglect of vulnerable individuals (74, 75). Second, the availability and heterogeneity of the original data, mainly resulting from reporting and selection biases, may hinder the synthesis of evidence in meta-analyses (29). Third, there are no established indicators or criteria that can be used to make a fully objective distinction between the superiority or inferiority of a model based on the results of meta-analyses, despite the use of the most common methods of discrimination such as the area under the receiver operating characteristic curve (AUC). But in view of poor methodological quality and ubiquitous high ROB, almost all the systematic reviews with or without a meta-analysis have adopted a deliberative attitude toward the application of prediction models in cardiovascular practice, regardless of their discrimination (76, 77). Addressing the aforementioned challenges will be critical for obtaining more high-level evidence in the future. More importantly, in terms of the prediction models themselves, how and when to update the existing models have been problems in an increasingly complex real-world medical setting, especially for certain specific cardiovascular diseases where treatment modalities and drugs are rapidly evolving. Thus, for static models (also called time-independent models), it is crucial to address calibration drift to maintain effective and efficient performance when the treatment is part of the predictors (78). Correspondingly, due to the lag inherent to the CPGs themselves, it will be wise and prudent for recommendations to take into account information regarding the updating (including frequency, aim, and methods of updating) of the recommended prediction model.
We also observed that the same models were recommended for heterogeneous target populations or conditions in different cardiovascular CPGs. For example, in addition to the general population (14), cancer patients treated with endocrine therapies were another target population recommended for the application of SCORE2 in the 2022 ESC Guidelines on cardio-oncology (41). Similar examples included the FRS (for the general population or patients with hypertension) (13, 54), Khorana risk score (for patients with cancer or inherited clotting disorder) (34, 42), and Society of Thoracic Surgeons risk score (for patients undergoing CABG or with valvular heart disease) (40, 46). Such excellent generalizability via external validation indicates the great application potential of a single well-established prediction model, which may contribute to research resource savings by reducing superfluous modeling studies (79).
To the best of our knowledge, this study was the first to systematically synthesize the recommendations for prediction models in cardiovascular CPGs published in the last 5 years while also conducting a methodological quality assessment of the primary studies on the development of the guideline-recommended models. The results may be of referential value to both clinicians and guideline methodologists. For future studies, substantial efforts are still required to improve the methodological quality of clinical prediction models, as well as their extensive external validation.
The limitations of this study merit further consideration. First, in consideration of the particularity of CPGs in terms of timeliness, we only included cardiovascular CPGs from 2018 to 2023, possibly omitting qualified guidelines that were published before 2018 and had an update period longer than 6 years. As a result, the few early-developed prediction models still in use may not have been assessed in this study. Second, in the case of the primary studies on the development of the guideline-recommended models identified through the references provided in the guidelines and from manual retrieval, there was no guaranteed consistency in model version in the 57 primary studies used for the assessment of methodological quality. This is due to individual models being updated over time, with multiple versions of primary modeling studies. Third, we did not further explore the methodological differences between the recommended and non-recommended models as these were not a major factor that supported or discouraged their use to provide clinical decision-making support. Finally, given the heterogeneous criteria for forming recommendations, we did not analyze the relevance between the strength of recommendation and the overall ROB of the prediction models. Thus, it is difficult to determine what role the ROB plays in the process of recommending models for cardiovascular practice. In any case, a high ROB implies that the performance of these models in new samples will probably be worse than that in the modeling samples, which could lead to improper clinical decisions and thus compromise the benefit for patients in healthcare settings (18, 80).
5 Conclusion
Global cardiovascular CPGs presented an unduly positive appraisal of the existing prediction models in terms of ROB, leading to stronger recommendations than were warranted. Future cardiovascular practice may benefit from well-established clinical prediction models with better methodological quality and extensive external validation.
Data availability statement
The original contributions presented in the study are included in the article/Supplementary Material, further inquiries can be directed to the corresponding author.
Author contributions
C-yJ: Conceptualization, Data curation, Formal Analysis, Methodology, Software, Visualization, Writing – original draft, Writing – review & editing. LZ: Data curation, Writing – review & editing. LF: Formal Analysis, Methodology, Writing – review & editing. J-cL: Formal Analysis, Methodology, Writing – review & editing. L-rL: Project administration, Writing – review & editing. JH: Project administration, Writing – review & editing. XL: Conceptualization, Funding acquisition, Project administration, Supervision, Writing – review & editing.
Funding
The author(s) declare financial support was received for the research, authorship, and/or publication of this article. This study was funded by China Academy of Chinese Medical Sciences Innovation Fund (No. CI2021A00701-3; No. CI2021A05503), National Natural Science Foundation of China (No. 82174239), and China Center for Evidence Based Traditional Chinese Medicine (No. 2020YJSZX-2). The funders had no role in the design and conduct of the study; collection, management, analysis, and interpretation of the data; preparation, review, or approval of the manuscript; and decision to submit the manuscript for publication.
Conflict of interest
XL reported receiving grants from National Natural Science Foundation of China, China Academy of Chinese Medical Sciences, and China Center for Evidence Based Traditional Chinese Medicine.
The remaining authors declare that the research was conducted in the absence of any commercial or financial relationships that could be construed as a potential conflict of interest.
Publisher's note
All claims expressed in this article are solely those of the authors and do not necessarily represent those of their affiliated organizations, or those of the publisher, the editors and the reviewers. Any product that may be evaluated in this article, or claim that may be made by its manufacturer, is not guaranteed or endorsed by the publisher.
Supplementary material
The Supplementary Material for this article can be found online at: https://www.frontiersin.org/articles/10.3389/fcvm.2024.1449058/full#supplementary-material
Abbreviations
ACC, American College of Cardiology; ACCF, American College of Cardiology Foundation; AHA, American Heart Association; AI, artificial intelligence; APTH, American Physical Therapy Association; AUC, area under the receiver operating characteristic curve; CABG, coronary artery bypass grafting; CMACPG, Canadian Medical Association: Clinical Practice Guideline; CPG, clinical practice guidelines; CRT, cluster randomized trial; CVD, cardiovascular diseases; ESC, European Society of Cardiology; ESMO, European Society for Medical Oncology; FRS, Framingham Risk Score; GIN, Guidelines International Network; GRADE, Grading of Recommendations Assessment, Development and Evaluation; HF, heart failure; NICE, National Institute for Health and Clinical Excellence; PCE, pooled cohort equations; PRISMA, Preferred Reporting Items for Systematic Review and Meta-Analysis; PROBAST, Prediction model Risk Of Bias ASsessment Tool; RCT, randomized controlled trial; ROB, risk of bias; SCORE2, Systematic Coronary Risk Estimation 2; SIGN, Scottish Intercollegiate Guidelines Network; WHO, World Health Organization.
References
1. Abbafati C, Abbas KM, Abbasi M, Abbasifard M, Abbasi-Kangevari M, Abbastabar H, et al. Global burden of 369 diseases and injuries in 204 countries and territories, 1990–2019: a systematic analysis for the global burden of disease study 2019. Lancet. (2020) 396(10258):1204–22. doi: 10.1016/S0140-6736(20)30925-9
2. Roth GA, Mensah GA, Johnson CO, Addolorato G, Ammirati E, Baddour LM, et al. Global burden of cardiovascular diseases and risk factors, 1990–2019. J Am Coll Cardiol. (2020) 76(25):2982–3021. doi: 10.1016/j.jacc.2020.11.010
3. Arnett DK, Blumenthal RS, Albert MA, Buroker AB, Goldberger ZD, Hahn EJ, et al. 2019 ACC/AHA guideline on the primary prevention of cardiovascular disease. J Am Coll Cardiol. (2019) 74(10):e177–232. doi: 10.1016/j.jacc.2019.03.010
4. Murray CJL, Aravkin AY, Zheng P, Abbafati C, Abbas KM, Abbasi-Kangevari M, et al. Global burden of 87 risk factors in 204 countries and territories, 1990–2019: a systematic analysis for the global burden of disease study 2019. Lancet. (2020) 396(10258):1223–49. doi: 10.1016/S0140-6736(20)30752-2
5. Tsao CW, Aday AW, Almarzooq ZI, Anderson CAM, Arora P, Avery CL, et al. Heart disease and stroke statistics—2023 update: a report from the American Heart Association. Circulation. (2023) 147(8):e93–621. doi: 10.1161/cir.0000000000001123
6. Bouwmeester W, Zuithoff NP, Mallett S, Geerlings MI, Vergouwe Y, Steyerberg EW, et al. Reporting and methods in clinical prediction research: a systematic review. PLoS Med. (2012) 9(5):1–12. doi: 10.1371/journal.pmed.1001221
7. Steyerberg EW, Moons KG, van der Windt DA, Hayden JA, Perel P, Schroter S, et al. Prognosis research strategy (PROGRESS) 3: prognostic model research. PLoS Med. (2013) 10(2):e1001381. doi: 10.1371/journal.pmed.1001381
8. Moons KG, Royston P, Vergouwe Y, Grobbee DE, Altman DG. Prognosis and prognostic research: what, why, and how? Br Med J. (2009) 338:b375. doi: 10.1136/bmj.b375
9. Jensen JK. Risk prediction Circulation. (2016) 134(19):1441–3. doi: 10.1161/CIRCULATIONAHA.116.024941
10. Rossello X, Dorresteijn JA, Janssen A, Lambrinou E, Scherrenberg M, Bonnefoy-Cudraz E, et al. Risk prediction tools in cardiovascular disease prevention: a report from the ESC prevention of CVD programme led by the European Association of Preventive Cardiology (EAPC) in collaboration with the Acute Cardiovascular Care Association (ACCA) and the Association of Cardiovascular Nursing and Allied Professions (ACNAP). Eur J Prev Cardiol. (2019) 26(14):1534–44. doi: 10.1177/2047487319846715
11. Stevanovic J, Postma MJ, Pechlivanoglou P. A systematic review on the application of cardiovascular risk prediction models in pharmacoeconomics, with a focus on primary prevention. Eur J Prev Cardiol. (2012) 19(2 Suppl):42–53. doi: 10.1177/2047487312448994
12. Heidenreich PA, Bozkurt B, Aguilar D, Allen LA, Byun JJ, Colvin MM, et al. 2022 AHA/ACC/HFSA guideline for the management of heart failure. J Am Coll Cardiol. (2022) 79(17):e263–421. doi: 10.1016/j.jacc.2021.12.012
13. Jain R, Stone JA, Agarwal G, Andrade JG, Bacon SL, Bajaj HS, et al. Canadian cardiovascular harmonized national guideline endeavour (C-CHANGE) guideline for the prevention and management of cardiovascular disease in primary care: 2022 update. CMAJ. (2022) 194(43):E1460–80. doi: 10.1503/cmaj.220138
14. Visseren FLJ, Mach F, Smulders YM, Carballo D, Koskinas KC, Bäck M, et al. 2021 ESC guidelines on cardiovascular disease prevention in clinical practice. Eur Heart J. (2021) 42(34):3227–337. doi: 10.1093/eurheartj/ehab484
15. Goff DC Jr, Lloyd-Jones DM, Bennett G, Coady S, D’Agostino RB, Gibbons R, et al. 2013 ACC/AHA guideline on the assessment of cardiovascular risk. Circulation. (2014) 129(25 Suppl 2):S49–73. doi: 10.1161/01.cir.0000437741.48606.98
16. National Institute for Health and Clinical Excellence. Cardiovascular Disease: Risk Assessment and Reduction, Including Lipid Modification (NG238). United Kingdom (2023). Available online at: https://www.nice.org.uk/guidance/cg181 (accessed December 28, 2023).
17. Andaur Navarro CL, Damen JAA, Takada T, Nijman SWJ, Dhiman P, Ma J, et al. Risk of bias in studies on prediction models developed using supervised machine learning techniques: systematic review. Br Med J. (2021) 375:n2281. doi: 10.1136/bmj.n2281
18. Wynants L, Van Calster B, Collins GS, Riley RD, Heinze G, Schuit E, et al. Prediction models for diagnosis and prognosis of COVID-19: systematic review and critical appraisal. Br Med J. (2020) 369:m1328. doi: 10.1136/bmj.m1328
19. Tan J, Ma C, Zhu C, Wang Y, Zou X, Li H, et al. Prediction models for depression risk among older adults: systematic review and critical appraisal. Ageing Res Rev. (2023) 83:101803. doi: 10.1016/j.arr.2022.101803
20. Kareemi H, Vaillancourt C, Rosenberg H, Fournier K, Yadav K. Machine learning versus usual care for diagnostic and prognostic prediction in the emergency department: a systematic review. Acad Emerg Med. (2021) 28(2):184–96. doi: 10.1111/acem.14190
21. de Winter MA, van Es N, Büller HR, Visseren FLJ, Nijkeuter M. Prediction models for recurrence and bleeding in patients with venous thromboembolism: a systematic review and critical appraisal. Thromb Res. (2021) 199:85–96. doi: 10.1016/j.thromres.2020.12.031
22. Cai R, Wu X, Li C, Chao J. Prediction models for cardiovascular disease risk in the hypertensive population: a systematic review. J Hypertens. (2020) 38(9):1632–9. doi: 10.1097/HJH.0000000000002442
23. Kee OT, Harun H, Mustafa N, Abdul Murad NA, Chin SF, Jaafar R, et al. Cardiovascular complications in a diabetes prediction model using machine learning: a systematic review. Cardiovasc Diabetol. (2023) 22(1):13. doi: 10.1186/s12933-023-01741-7
24. Institute of Medicine (US). Clinical Practice Guidelines We Can Trust. Washington, DC: The National Academies Press (2011).
25. Petretta M, Cuocolo A. Prediction models for risk classification in cardiovascular disease. Eur J Nucl Med Mol Imaging. (2012) 39(12):1959–69. doi: 10.1007/s00259-012-2254-1
26. von Elm E, Altman DG, Egger M, Pocock SJ, Gøtzsche PC, Vandenbroucke JP. The strengthening the reporting of observational studies in epidemiology (STROBE) statement: guidelines for reporting observational studies. J Clin Epidemiol. (2008) 61(4):344–9. doi: 10.1016/j.jclinepi.2007.11.008
27. Page MJ, McKenzie JE, Bossuyt PM, Boutron I, Hoffmann TC, Mulrow CD, et al. The PRISMA 2020 statement: an updated guideline for reporting systematic reviews. J Clin Epidemiol. (2021) 134:178–89. doi: 10.1016/j.jclinepi.2021.03.001
28. Wolff RF, Moons KGM, Riley RD, Whiting PF, Westwood M, Collins GS, et al. PROBAST: a tool to assess the risk of bias and applicability of prediction model studies. Ann Intern Med. (2019) 170(1):51–8. doi: 10.7326/M18-1376
29. Moons KGM, Wolff RF, Riley RD, Whiting PF, Westwood M, Collins GS, et al. PROBAST: a tool to assess risk of bias and applicability of prediction model studies: explanation and elaboration. Ann Intern Med. (2019) 170(1):W1–33. doi: 10.7326/M18-1377
30. Virani SS, Newby LK, Arnold SV, Bittner V, Brewer LC, Demeter SH, et al. 2023 AHA/ACC/ACCP/ASPC/NLA/PCNA guideline for the management of patients with chronic coronary disease: a report of the American Heart Association/American College of Cardiology joint committee on clinical practice guidelines. Circulation. (2023) 148(9):e9–119. doi: 10.1161/CIR.0000000000001168
31. Ueng KC, Chiang CE, Chao TH, Wu YW, Lee WL, Li YH, et al. 2023 guidelines of the Taiwan Society of Cardiology on the diagnosis and management of chronic coronary syndrome. Acta Cardiologica Sinica. (2023) 39(1):4–96. doi: 10.6515/ACS.202301_39(1).20221103A
32. Takase B, Ikeda T, Shimizu W, Abe H, Aiba T, Chinushi M, et al. JCS/JHRS 2022 guideline on diagnosis and risk assessment of arrhythmia. Circ J. (2024) 88(9):1509–95. doi: 10.1253/circj.CJ-22-0827
33. Rajajee V, Muehlschlegel S, Wartenberg KE, Alexander SA, Busl KM, Chou SHY, et al. Guidelines for neuroprognostication in comatose adult survivors of cardiac arrest. Neurocrit Care. (2023) 38(3):533–63. doi: 10.1007/s12028-023-01688-3
34. Key NS, Khorana AA, Kuderer NM, Bohlke K, Lee AYY, Arcelus JI, et al. Venous thromboembolism prophylaxis and treatment in patients with cancer: ASCO guideline update. J Clin Oncol. (2023) 41(16):3063–71. doi: 10.1200/JCO.23.00294
35. Hiraoka E, Tanabe K, Izuta S, Kubota T, Kohsaka S, Kozuki A, et al. JCS 2022 guideline on perioperative cardiovascular assessment and management for non-cardiac surgery. Circ J. (2023) 87(9):1253–337. doi: 10.1253/circj.CJ-22-0609
36. Falanga A, Ay C, Di Nisio M, Gerotziafas G, Jara-Palomares L, Langer F, et al. Venous thromboembolism in cancer patients: ESMO clinical practice guideline. Ann Oncol. (2023) 34(5):452–67. doi: 10.1016/j.annonc.2022.12.014
37. Byrne RA, Rossello X, Coughlan JJ, Barbato E, Berry C, Chieffo A, et al. 2023 ESC guidelines for the management of acute coronary syndromes. Eur Heart J. (2023) 44(38):3720–826. doi: 10.1093/eurheartj/ehad191
38. Arbelo E, Protonotarios A, Gimeno JR, Arbustini E, Arbelo E, Barriales-Villa R, et al. 2023 ESC guidelines for the management of cardiomyopathies. Eur Heart J. (2023) 44(37):3503–626. doi: 10.1093/eurheartj/ehad194
39. Zeppenfeld K, Tfelt-Hansen J, De Riva M, Winkel BG, Behr ER, Blom NA, et al. 2022 ESC guidelines for the management of patients with ventricular arrhythmias and the prevention of sudden cardiac death. Eur Heart J. (2022) 43(40):3997–4126. doi: 10.1093/eurheartj/ehac262
40. Nakamura M, Yaku H, Ako J, Arai H, Asai T, Chikamori T, et al. JCS/JSCVS 2018 guideline on revascularization of stable coronary artery disease. Circ J. (2022) 86(3):477–588. doi: 10.1253/circj.CJ-20-1282
41. Lyon AR, López-Fernánde T, Couch LS, Asteggiano R, Aznar MC, Bergler-Klei J, et al. 2022 ESC guidelines on cardio-oncology developed in collaboration with the European Hematology Association (EHA), the European Society for Therapeutic Radiology and Oncology (ESTRO) and the International Cardio-Oncology Society (IC-OS). Eur Heart J. (2022) 43(41):4229–361. doi: 10.1093/eurheartj/ehac244
42. Hillegass E, Lukaszewicz K, Puthoff M. Role of physical therapists in the management of individuals at risk for or diagnosed with venous thromboembolism: evidence-based clinical practice guideline 2022. Phys Ther. (2022) 102(8):pzac057. doi: 10.1093/ptj/pzac057
43. Farge D, Frere C, Connors JM, Khorana AA, Kakkar A, Ay C, et al. 2022 international clinical practice guidelines for the treatment and prophylaxis of venous thromboembolism in patients with cancer, including patients with COVID-19. Lancet Oncol. (2022) 23(7):e334–47. doi: 10.1016/S1470-2045(22)00160-7
44. Albricker ACL, Freire CMV, Dos Santos SN, De Alcantara ML, Saleh MH, Cantisano AL, et al. Joint guideline on venous thromboembolism, 2022. Arq Bras Cardiol. (2022) 118(4):797–857. doi: 10.36660/abc.20220213
45. Tsutsui H, Ide T, Ito H, Kihara Y, Kinugawa K, Kinugawa S, et al. JCS/JHFS 2021 guideline focused update on diagnosis and treatment of acute and chronic heart failure. J Card Fail. (2021) 27(12):1404–44. doi: 10.1016/j.cardfail.2021.04.023
46. Otto CM, Nishimura RA, Bonow RO, Carabello BA, Erwin JP, Gentile F, et al. 2020 ACC/AHA guideline for the management of patients with valvular heart disease: a report of the American College of Cardiology/American Heart Association joint committee on clinical practice guidelines. Circulation. (2021) 143(5):E72–227. doi: 10.1161/CIR.0000000000000923
47. Lawton JS, Tamis-Holland JE, Bangalore S, Bates ER, Beckie TM, Bischoff JM, et al. 2021 ACC/AHA/SCAI guideline for coronary artery revascularization: a report of the American College of Cardiology/American Heart Association joint committee on clinical practice guidelines. Circulation. (2022):CIR0000000000001038. doi: 10.1161/CIR.0000000000001038
48. Kakkos SK, Gohel M, Baekgaard N, Bauersachs R, Bellmunt-Montoya S, Black SA, et al. Editor’s choice—European Society for Vascular Surgery (ESVS) 2021 clinical practice guidelines on the management of venous thrombosis. Eur J Vasc Endovasc Surg. (2021) 61(1):9–82. doi: 10.1016/j.ejvs.2020.09.023
49. Hindricks G, Potpara T, Dagres N, Arbelo E, Bax JJ, Blomström-Lundqvist C, et al. 2020 ESC guidelines for the diagnosis and management of atrial fibrillation developed in collaboration with the European association for cardio-thoracic surgery (EACTS). Eur Heart J. (2021) 42(5):373–498. doi: 10.1093/eurheartj/ehaa612
50. Mach F, Baigent C, Catapano AL, Koskinas KC, Casula M, Badimon L, et al. 2019 ESC/EAS guidelines for the management of dyslipidaemias: lipid modification to reduce cardiovascular risk. Eur Heart J. (2020) 41(1):111–88. doi: 10.1093/eurheartj/ehz455
51. Liao PD, Chen KJ, Ge JB, Zhang MZ. Clinical practice guideline of integrative Chinese and western medicine for acute myocardial infarction. Chin J Integr Med. (2020) 26(7):539–51. doi: 10.1007/s11655-019-3154-z
52. Knuuti J, Wijns W, Saraste A, Capodanno D, Barbato E, Funck-Brentano C, et al. 2019 ESC guidelines for the diagnosis and management of chronic coronary syndromes. Eur Heart J. (2020) 41(3):407–77. doi: 10.1093/eurheartj/ehz425
53. Hajjar LA, Da Costa IBSS, Lopes MACQ, Hoff PMG, Diz MDPE, Fonseca SMR, et al. Diretriz Brasileira de cardio-oncologia—2020. Arq Bras Cardiol. (2020) 115(5):1006–43. doi: 10.36660/abc.20201006
54. Tykarski A, Filipiak KJ, Januszewicz A, Litwin M, Narkiewicz K, Prejbisz A, et al. 2019 guidelines for the management of hypertension—part 1–7. Arterial Hypertension (Poland). (2019) 23(2):41–87. doi: 10.5603/AH.a2019.0008
55. Tran HA, Gibbs H, Merriman E, Curnow JL, Young L, Bennett A, et al. New guidelines from the thrombosis and haemostasis society of Australia and New Zealand for the diagnosis and management of venous thromboembolism. Med J Aust. (2019) 210(5):227–35. doi: 10.5694/mja2.50004
56. Sousa-Uva M, Neumann FJ, Ahlsson A, Alfonso F, Banning AP, Benedetto U, et al. 2018 ESC/EACTS guidelines on myocardial revascularization. Eur J Cardiothorac Surg. (2019) 55(1):4–90. doi: 10.1093/ejcts/ezy289
57. Kimura K, Kimura T, Ishihara M, Nakagawa Y, Nakao K, Miyauchi K, et al. JCS 2018 guideline on diagnosis and treatment of acute coronary syndrome. Circ J. (2019) 83(5):1085–196. doi: 10.1253/circj.CJ-19-0133
58. Feitosa-Filho GS, Peixoto JM, Pinheiro JES, Neto AA, de Albuquerque ALT, Cattani ÁC, et al. Updated geriatric cardiology guidelines of the Brazilian society of cardiology—2019. Arq Bras Cardiol. (2019) 112(5):649–705. doi: 10.5935/abc.20190086
59. Chantrarat T, Kunjara Na Ayudhya R, Phrominthikul A, Ariyachaipanich A, Krittayaphong R. Heart failure council of Thailand (HFCT) 2019 heart failure guideline: atrial fibrillation in heart failure guidelines. J Med Assoc Thail. (2019) 102(4):513–7.
60. Li YH, Wang YC, Wang YC, Liu JC, Lee CH, Chen CC, et al. 2018 guidelines of the Taiwan Society of Cardiology, Taiwan Society of Emergency Medicine and Taiwan Society of Cardiovascular Interventions for the management of non ST-segment elevation acute coronary syndrome. J Formos Med Assoc. (2018) 117(9):766–90. doi: 10.1016/j.jfma.2018.06.002
61. Joung B, Lee JM, Lee KH, Kim TH, Choi EK, Lim WH, et al. 2018 Korean guideline of atrial fibrillation management. Korean Circ J. (2018) 48(12):1033–80. doi: 10.4070/kcj.2018.0339
62. Brieger D, Amerena J, Attia J, Bajorek B, Chan KH, Connell C, et al. National Heart Foundation of Australia and the Cardiac Society of Australia and New Zealand: Australian clinical guidelines for the diagnosis and management of atrial fibrillation 2018. Heart Lung Circ. (2018) 27(10):1209–66. doi: 10.1016/j.hlc.2018.06.1043
63. National Institute for Health and Clinical Excellence. Familial Fypercholesterolaemia: Identification and Management (CG71). United Kingdom. (2019). Available online at: https://www.nice.org.uk/guidance/cg71 (accessed December 28, 2023).
64. National Institute for Health and Clinical Excellence. Abdominal Aortic Aneurysm: Diagnosis and Management (NG156). United Kingdom. (2020). Available online at: https://www.nice.org.uk/guidance/ng156 (accessed December 28, 2023).
65. National Institute for Health and Clinical Excellence. Venous Thromboembolic Diseases: Diagnosis, Management and Thrombophilia Testing (NG158). United Kingdom. (2023). Available online at: https://www.nice.org.uk/guidance/ng158 (accessed December 28, 2023).
66. National Institute for Health and Clinical Excellence. Acute Coronary Syndromes (NG185). (2020). United Kingdom. Available online at: https://www.nice.org.uk/guidance/ng185 (accessed December 28, 2023).
67. National Institute for Health and Clinical Excellence. Atrial Fibrillation: Diagnosis and Management (NG196). United Kingdom. (2021). Available online at: https://www.nice.org.uk/guidance/ng196 (accessed December 28, 2023).
68. American Diabetes Association, European Society of Endocrinology. Primary Prevention of ASCVD and T2DM in Patients at Metabolic Risk: An Endocrine Society* Clinical Practice Guideline. United States. (2019). Available online at: https://guidelines.ebmportal.com/primary-prevention-ascvd-and-t2dm-patients-metabolic-risk-endocrine-societyclinical-practice (accessed December 28, 2023).
69. European Society of Endocrinology. Lipid Management in Patients with Endocrine Disorders: An Endocrine Society Clinical Practice Guideline. United States. (2020). Available online at: https://guidelines.ebmportal.com/lipid-management-patients-endocrinedisorders-endocrine-society-clinical-practice-guideline (accessed December 28, 2023).
70. Guidelines and Protocols and Advisory Committee. Cardiovascular Disease—Primary Prevention. (2021). Canada. Available online at: https://www2.gov.bc.ca/assets/gov/health/practitioner-pro/bc-guidelines/gpac_cardiovasculardisease_full_guideline_dec2021_updated_aug2023.pdf (accessed December 28, 2023).
71. Steyerberg EW, Vergouwe Y. Towards better clinical prediction models: seven steps for development and an ABCD for validation. Eur Heart J. (2014) 35(29):1925–31. doi: 10.1093/eurheartj/ehu207
72. Vagholkar S, Zwar N, Jayasinghe UW, Denney-Wilson E, Patel A, Campbell T, et al. Influence of cardiovascular absolute risk assessment on prescribing of antihypertensive and lipid-lowering medications: a cluster randomized controlled trial. Am Heart J. (2014) 167(1):28–35. doi: 10.1016/j.ahj.2013.10.002
73. Svendsen K, Jacobs DR, Morch-Reiersen LT, Garstad KW, Henriksen HB, Telle-Hansen VH, et al. Evaluating the use of the heart age tool in community pharmacies: a 4-week cluster-randomized controlled trial. Eur J Public Health. (2020) 30(6):1139–45. doi: 10.1093/eurpub/ckaa048
74. McRae AD, Weijer C, Binik A, Grimshaw JM, Boruch R, Brehaut JC, et al. When is informed consent required in cluster randomized trials in health research? Trials. (2011) 12:202. doi: 10.1186/1745-6215-12-202
75. Weijer C, Grimshaw JM, Eccles MP, McRae AD, White A, Brehaut JC, et al. The Ottawa statement on the ethical design and conduct of cluster randomized trials. PLoS Med. (2012) 9(11):e1001346. doi: 10.1371/journal.pmed.1001346
76. Soares C, Kwok M, Boucher KA, Haji M, Echouffo-Tcheugui JB, Longenecker CT, et al. Performance of cardiovascular risk prediction models among people living with HIV: a systematic review and meta-analysis. JAMA Cardiol. (2023) 8(2):139–49. doi: 10.1001/jamacardio.2022.4873
77. Kaboré EG, Macdonald C, Kaboré A, Didier R, Arveux P, Meda N, et al. Risk prediction models for cardiotoxicity of chemotherapy among patients with breast cancer: a systematic review. JAMA Netw Open. (2023) 6(2):e230569. doi: 10.1001/jamanetworkopen.2023.0569
78. Davis SE, Lasko TA, Chen G, Siew ED, Matheny ME. Calibration drift in regression and machine learning models for acute kidney injury. J Am Med Inform Assoc. (2017) 24(6):1052–61. doi: 10.1093/jamia/ocx030
79. Glasziou P, Altman DG, Bossuyt P, Boutron I, Clarke M, Julious S, et al. Reducing waste from incomplete or unusable reports of biomedical research. Lancet. (2014) 383(9913):267–76. doi: 10.1016/S0140-6736(13)62228-X
Keywords: prediction model, recommendation, clinical practice guideline, cardiovascular diseases, methodological quality, risk of bias
Citation: Jing C-y, Zhang L, Feng L, Li J-c, Liang L-r, Hu J and Liao X (2024) Recommendations for prediction models in clinical practice guidelines for cardiovascular diseases are over-optimistic: a global survey utilizing a systematic literature search. Front. Cardiovasc. Med. 11:1449058. doi: 10.3389/fcvm.2024.1449058
Received: 18 June 2024; Accepted: 25 September 2024;
Published: 17 October 2024.
Edited by:
Junfeng Wang, Utrecht University, NetherlandsReviewed by:
Alessandra Cartocci, University of Siena, ItalySatish Chandrasekhar Nair, Tawam Hospital, United Arab Emirates
Copyright: © 2024 Jing, Zhang, Feng, Li, Liang, Hu and Liao. This is an open-access article distributed under the terms of the Creative Commons Attribution License (CC BY). The use, distribution or reproduction in other forums is permitted, provided the original author(s) and the copyright owner(s) are credited and that the original publication in this journal is cited, in accordance with accepted academic practice. No use, distribution or reproduction is permitted which does not comply with these terms.
*Correspondence: Xing Liao, b2tmcm9tMjAwOEBob3RtYWlsLmNvbQ==