- 1Department of Clinical Nutrition, Tongde Hospital of Zhejiang Province, Hangzhou, China
- 2Department of Clinical Nutrition, Sir Run Run Shaw Hospital, Zhejiang University School of Medicine, Hangzhou, China
- 3Department of Clinical Nutrition, Shaoxing People’s Hospital, Shaoxing, China
Background: The risk of congestive heart failure (CHF) is significantly affected by obesity. However, data on the association between visceral obesity and the risk of CHF remain limited. We explored the relationship between CHF and cardiometabolic index (CMI).
Methods: Drawing from the National Health and Nutrition Examination Survey (NHANES) for 2011–2018, we enrolled 9,008 participants in a cross-sectional study. We calculated the CMI as triglyceride (TG)/high density lipid-cholesterol (HDL-C) × weight-to-height ratio (WHtR), and CMI-age as CMI × age. Then, we analyzed CMI and CMI-age as categorical and continuous variables to assess its correlation with CHF. To assess the relationships of CMI and CMI-age with CHF, we used multiple logistic regression models and performed subgroup analysis. To examine the predictive ability of CMI and CMI-age on patients with CHF, we used receiver operating characteristic (ROC) curves.
Results: The overall prevalence of CHF was 3.31%. The results revealed significant differences in demographic data, comorbidities, lifestyle variables, standing height, BMI, WC, WHtR, TG, and HDL-C among the four groups classified by CMI quartile and CMI-age quartile. When indicators were analyzed as continuous variables, CMI and CMI-age showed positive correlations with CHF in both the crude and adjusted models (all P < 0.05). When indicators were analyzed as categorical variables, it was found that in all four models, the ORs of group Q4 was significantly different compared to Q1 (all P < 0.05), suggesting the risk of CHF is significantly increased with higher CMI, and CMI-age. The associations of CMI and CMI-age with CHF were similar in all stratified populations (P for interaction > 0.05). The areas under the ROC curve (AUCs) of CMI and CMI-age in predicting CHF were 0.610 (95% CI, 0.578–0.642) and 0.697 (95% CI, 0.668–0.725) separately, suggesting that CMI-age was significantly better than the CMI in predicting CHF (P < 0.001).
Conclusions: Both CMI and CMI-age were independently correlated with the risk for CHF. These results suggested that the CMI-age, which provides new insights into the prevention and management of CHF. CMI-age could serve as effective tools to identify CHF during primary care examinations and in medically resource-limited areas.
Introduction
Functional and structural impairments of ventricular filling or blood ejection characterize the symptoms of congestive heart failure (CHF) (1). This life-threatening syndrome is responsible for significant morbidity and mortality as well as for limited quality of life and functional ability. High costs are also attributed to this complex syndrome (2, 3). Because of aging populations, the number of CHF cases is increasing, and to date, more than 64 million people suffer from CHF globally. Comorbidities and associated risk factors as well as longer survival times after myocardial infarction are also contributing to this rise in cases (2–4). Recent literature has also indicated that the HF burden in 50 years or younger is increasing (4). Hence, the early detection and aggressive modification of risk factors for CHF are of immense significance to prevent progression.
Obesity not only directly harms the myocardium but also indirectly increases the risk of CHF by promoting the development of many metabolic risk factors (5–7). Studies have revealed that the marked rise in prevalence of obesity, which is most prominent among those from impoverished socioeconomic backgrounds, has been among the strong risk factors for the development of CHF (7). Although body mass index (BMI) is very common to describe the obesity-related cardiovascular risk, it may not fully reflect the amount and distribution of body fat and fail to differentiate the increased body fat content, preserved or increased lean mass, and the body hydration status (6–8). Compared to obesity defined by BMI, the accumulation of visceral adipose is widely recognized as a more accurate predictor of morbidity and mortality in CHF (6, 7). To assess visceral obesity, a new simple and noninvasive index that combines weight-to-height ratio (WHtR) and biochemical lipid parameters has been suggested, called the cardiometabolic index (CMI) (9). Studies have shown a relationship between the CMI and diabetes mellitus (DM), cardiovascular disease (CVDs), and metabolic syndrome (MetS), suggesting that the CMI is useful for screening for these conditions (9–15). Nevertheless, there has been no investigation into the possible correlation between CMI and CHF, nor has it been tested as a screening tool for the condition. Furthermore, according to data from the European Society of Cardiology, 10% CHF patients are over 70 years old, and thus a combination of CMI with age may potentially identify CHF more strongly than CMI (16). Therefore, the aim of this study was to evaluate the association of CMI and CMI-age with CHF, and to compare the two indicators for early identification of CHF using the data of the National Health and Nutrition Examination Survey (NHANES) from 2011 to 2018.
Materials and methods
Study population
This survey collected data including demographics, physical examinations, questionnaire, and health-related data from the NHANES database for 2011–2018. All survey methods and data are available at https://www.cdc.gov/nchs/nhanes/about_nhanes.htm. The National Center for Health Statistics Research Ethics Review Board approved the research protocols. Our exclusion criteria included age < 20 years, missing CHF status, missing CMI-related parameters, and pregnancy (Figure 1).
Definitions
The verification of CHF is based on the questionnaire from MCQ, similar to published NHANES-based articles (14). Participants were asked the following question: “has a doctor ever told you that you have CHF?” Those who responded “yes” were classified as having CHF. The criteria for hypertension included self-reported physician-diagnosed hypertension, antihypertensive drug use, or systolic and diastolic blood pressure (BP) of ≥140 or ≥90 mmHg (6). The criteria for self-reported or physician-diagnosed diabetes mellitus included (1) 126 mg/dl of fasting glucose or 200 mg/dl of plasma glucose within 2 h of taking an oral glucose tolerance test, and (2) the use of insulin or oral hypoglycemic medications (6). We used the following formulas: BMI (kg/m2) = weight/height squared. WHtR = waist circumference (WC, cm)/height (cm). CMI = Triglyceride (TG, mmol/L)/high-density lipoprotein-cholesterol (HDL-C, mmol/L) × WHtR (9). CMI-age = CMI × age.
Covariates
We obtained the particular methodologies and caliber of determination for every covariate control approach from NHANES (https://www.cdc.gov/nchs/nhanes/about_nhanes.htm). And we selected covariates, including demographic data, comorbidities, lifestyle variables, height, BMI, WC, WHtR, TG, and HDL-C, based on statistical significance and therapeutic relevance.
Statistical analysis
We used R statistical packages (The R Foundation; http://www.r-project.org; version 4.2.1) and EmpowerStats (www.empowerstats.net, X&Y solutions, Inc. Boston, Massachusetts) for all statistical analyses. We considered differences to be statistically significant at P < 0.05. Through the use of sample weights and a sophisticated sampling methodology, NHANES was able to acquire data that was nationally representative. We used the sample weight calculation method proposed by NHANES to weight the data in this paper.
We used mean ± SD to express continuous data, with the number data expressed as n (%). First, we compared the baseline characteristics of CMI quartile groups and CMI-age quartiles groups. The quintile cut-off values of the CMI are 0.27, 0.48, and 0.83. The quintile cut-off values of the CMI-age are 11.78, 23.13, and 43.88. Then, we used multiple logistic regression models to determine the relationship between CHF and CMI. We applied multivariate adjusted models and nonadjusted models. The variables were adjusted for age, sex, and race; education level, family PIR, and marital status; smoking status; moderate recreational activities; BMI, DM and hypertension status. We completed stratified and interaction analyses based on gender, age, and race; marital status; smoking status; moderate recreational activities; BMI, DM and hypertension status. Finally, we explored the associations of CMI and CMI-age with CHF using smooth curve plots. To assess the predictive efficacy of CMI and CMI-age for patients with CHF, we used receiver operating characteristic (ROC) curves.
Results
Baseline characteristics of study population
We extracted data of 9,008 participants from the NHANES database (Figure 1). Then, we divided the data into four groups by CMI quartile and CMI-age quartile. Table 1 lists the baseline characteristics for the analysis samples. CHF prevalence was 3.31%. We observed significant differences in demographic data among these CMI and CMI-age groups (e.g., race, gender, and age; marital status; education levels; and family PIR). In the higher CMI and CMI-age quartile groups, the proportion of smoking status increased and moderate recreational activities decreased. In addition, in the higher CMI and CMI-age quartile WC, BMI, WHtR, and TG tended to increase, whereas HDL-C decreased. Predictably, hypertension, diabetes, and CHF also increased in these groups. We also divided the data into two groups by CHF status, and the demographic data including gender, race, age, marital status, education levels, family PIR, smoking status, moderate recreational activities, BMI, WC, WHtR, TG, HDL-C, CMI, CMI-age hypertension status, and DM status showed significant differences between two groups (Supplementary Table S1).
Association between CMI, CMI-age and CHF
The relationships of CMI and CMI-age with CHF are shown in Table 2. When indicators were analyzed as continuous variables, CMI and CMI-age showed positive correlations with CHF in both the crude and adjusted models (all P < 0.05). When indicators were analyzed as categorical variables, it was found that in all models, the ORs of group Q4 was significantly different compared to Q1 (all P < 0.05), suggesting the risk of CHF is significantly increased with higher CMI and higher CMI-age. In both the crude and adjusted models, the overall trend showed that the higher CMI quartiles and higher CMI-age quartiles were strongly associated with the incidence of CHF.
Subgroup analysis
We used sex, race, age, education level, marital status, smoking status, BMI, moderate recreational activities, hypertension, and diabetes as stratification variables, and performed stratified analysis to assess the effects of CMI and CMI-age on CHF. As shown in Table 3, associations of CMI and CMI-age with CHF were similar in all stratified populations (P for interaction > 0.05).
Nonlinear associations and ROC curves
We also used smooth curve plots to examine the associations of CMI and CMI-age with CHF (Figure 2). To distinguish CHF, we analyzed the ROC curves for the CMI and CMI-age (Figure 3). Among the entire cohort, the area under the ROC curve (AUC) of the CMI for the identification of CHF was 0.610 (95% CI: 0.578–0.642, P < 0.001), the AUC of the CMI-age for the identification of CHF was 0.697 (95% CI, 0.668–0.725, P < 0.001). The AUC of the CMI-age was significantly higher than CMI (P < 0.001). Furthermore, we compared the AUCs of the CMI, CMI-age and BMI for the identification of CHF (Figure 3), suggesting that CMI-age was significantly better than CMI, BMI and CMI-BMI in predicting CHF (P < 0.001).
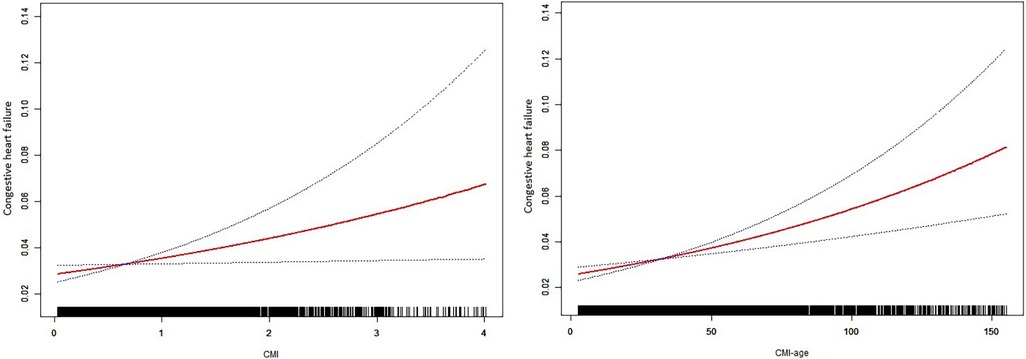
Figure 2. Smooth curve plots between CMI and heart failure. CMI, cardiometabolic index. Age, sex, race/ethnicity, educational level, family PIR, diabetes status, marital status, BMI, hypertension status, smoking status, and moderate recreational activities were adjusted.
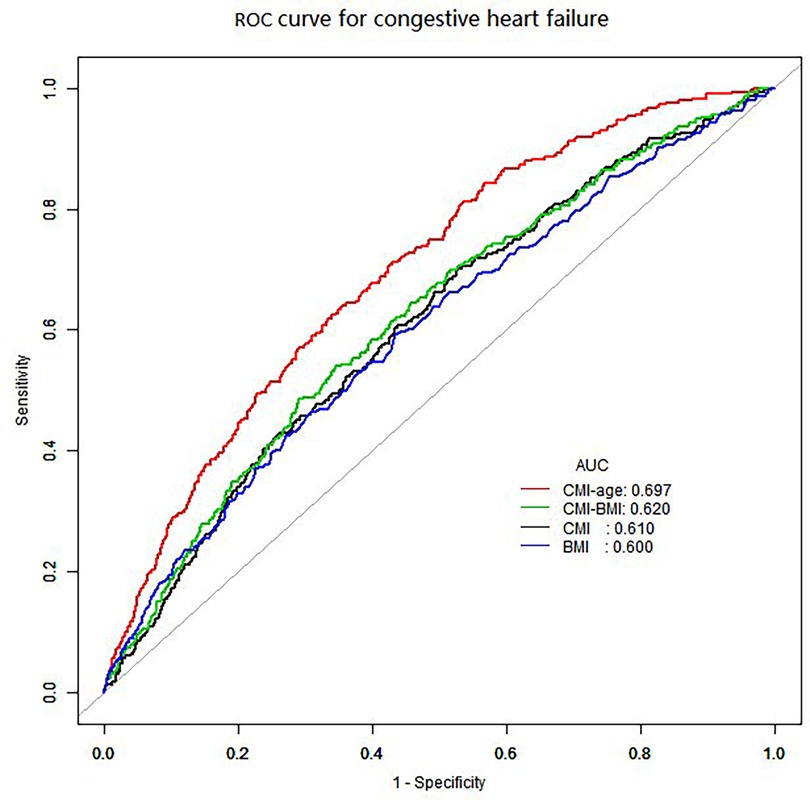
Figure 3. ROC curves for the identification of heart failure. CMI: AUC = 0.610 (95% CI, 0.578–0.642, specificity 0.472, sensitivity 0.701). CMI-age: AUC = 0.697 (95% CI, 0.668–0.725, specificity 0.648, sensitivity 0.638). BMI: AUC = 0.600 (95% CI, 0.566–0.633, specificity 0.565, sensitivity 0.593). CMI-BMI: AUC = 0.620 (95% CI, 0.588–0.653, specificity 0.710, sensitivity 0.485). CMI, cardiometabolic index; ROC, receiver operating characteristic curve; AUC, ROC area.
Discussion
We evaluated the associations of CMI and CMI-age with the risk of CHF in US adults according to NHANES data for 2011–2018. The results showed that the overall prevalence of CHF was 3.31%, which was consistent with previous studies (6, 14). We provided evidence that CMI and CMI-age were independently associated with CHF and exhibited near-linear dose-response relationships. A deeper understanding of CMI, CMI-age and CHF in various populations also can be gained through subgroup analysis, which indicated that the direction of correlations between CMI, CMI-age and CHF in various subgroups was consistent with those in the study population as a whole.
Conventional risk factors (e.g., age, smoking, obesity, hypertension, and DM) are associated with CHF, according to population-based research (4, 17). Although obesity has posed an independent risk factor for CHF, the diversity of obesity phenotypes may result in differences in incidence, treatment outcomes, and mortality of CHF (18, 19). Recent literature has shown that increases in visceral adipose tissue (VAT) rather than subcutaneous fat are a significant risk factor for the development of CHF (20–22). This may due to the higher degrees of adipocyte hypertrophy, free fatty acids elevation, and insulin resistance among patients with increased VAT (23). CMI is now being used to evaluate VAT because it is noninvasive index that combines TG/HDL-C and WHtR (9). To measure abdominal obesity, WHtR is a valuable parameter and helps to identify cardiovascular disease (24, 25). To identify metabolic disorders and CVDs, TG/HDL-C is suitable (26–28). As a result, we proposed the use of CMI to identify CHF in adults.
Previous studies have concluded that CMI is associated with DM, CVDs, and MetS (9–15). Data are limited, however, on the relationship between the risk of CHF and CMI. We believe we are the first to examine the correlation between CMI and CHF in a significant sample of US adults. The logistic regression analysis showed that higher CMI quartiles were associated independently with elevated risk factors for CHF. We observed positive and robust correlations between CHF and CMI, regardless of multiple confounding factors. The ROC analysis showed that the diagnostic capacity of CMI was adequate, with an AUC of 0.610 (95% CI: 0.578–0.642). Together, these findings highlighted the clinical value of CMI for screening CHF among US adults. Previous studies also have found a strong correlation between CHF and VAT, which is consistent with our results (6, 20, 21). Past studies have measured VAT with magnetic resonance imaging and abdominal computed tomography. These methods, although accurate, are expensive and inefficient. Ye et al. demonstrated that CMI was independently correlated with left ventricular diastolic dysfunction among asymptomatic Chinese adults (10). Similar to our findings, it was also found that the diagnostic capacity of CMI was moderate and had an AUC of 0.615 (95% CI: 0.587–0.643) (10). Along a complex path from risk to fully developed CHF, a booming number of proteins related to damage, remodeling, and neurohormonal activation have been discovered (2). However, CMI can be calculated by measuring TG, HDL-C, height, and WC, which are simple and easy to obtain.
According to data from the European Society of Cardiology, 10% CHF patients are over 70 years old (16). The number of CHF is rising as a result of aging, an increase in the load of comorbidities and risk factors for the condition, and longer survival times following myocardial infarction (1–3, 16). Besides, a Japanese population based study showed that CMI and its association with DM are potently influenced by age (29). Thus, we proposed that a combination of CMI with age may potentially identify CHF more strongly. We evaluated the association between CMI-age and CHF, and compared indicators of CMI and CMI-age for early identification of CHF. The ROC curve investigation affirmed that CMI-age is a favorable surrogate indicator of CHF, and CMI-age could be an effective way to detect CHF during primary care examinations (30, 31).
Strengths and limitations
The present study demonstrated that the CMI-age can be utilized as predictive tool for assessing the likelihood of developing CHF. And this finding establishes a foundation for promoting health and implementing preventive measures to manage and control CHF. However, the present study still has some limitations. Firstly, this was an observational study. This established the associations of CMI and CMI-age with CHF without establishing a causative relationship. Secondly, it is challenging to determine the severity of CHF due to limitations of the NHANES database. Thirdly, this study was conducted among adult Americans and had a limited population size, thereby limiting the generalizability of the results to CHF population from different geographical areas.
Conclusion
The present study found that CMI and CMI-age were all independently correlated with CHF risk. Furthermore, high CMI and CMI-age warranted greater attention to prevent CHF risk. By combining biochemical and anthropometric lipid parameters, this novel index could be an effective way to detect CHF. The CMI-age index was significantly better than CMI in predicting CHF, which provides new insights into the prevention and management of CHF. CMI-age could be an effective way to detect CHF during primary care examinations, most notably in areas with limited medical resources.
Data availability statement
The original contributions presented in the study are included in the article/Supplementary Material, further inquiries can be directed to the corresponding author.
Ethics statement
The studies involving humans were approved by National Health and Nutrition Examination Survey. The studies were conducted in accordance with the local legislation and institutional requirements. The participants provided their written informed consent to participate in this study.
Author contributions
XL: Conceptualization, Data curation, Methodology, Writing – original draft. BC: Writing – review & editing.
Funding
The author(s) declare financial support was received for the research, authorship, and/or publication of this article. This research supported by Chinese Medicine Research Program of Zhejiang Province (Grant No. 2024ZL349).
Conflict of interest
The authors declare that the research was conducted in the absence of any commercial or financial relationships that could be construed as a potential conflict of interest.
Publisher's note
All claims expressed in this article are solely those of the authors and do not necessarily represent those of their affiliated organizations, or those of the publisher, the editors and the reviewers. Any product that may be evaluated in this article, or claim that may be made by its manufacturer, is not guaranteed or endorsed by the publisher.
Supplementary material
The Supplementary Material for this article can be found online at: https://www.frontiersin.org/articles/10.3389/fcvm.2024.1433950/full#supplementary-material
References
1. Heidenreich PA, Bozkurt B, Aguilar D, Allen LA, Byun JJ, Colvin MM, et al. 2022 AHA/ACC/HFSA guideline for the management of heart failure: a report of the American College of Cardiology/American Heart Association joint committee on clinical practice guidelines. Circulation. (2022) 145(18):e895–e1032. doi: 10.1161/CIR.0000000000001063
2. Castiglione V, Aimo A, Vergaro G, Saccaro L, Passino C, Emdin M. Biomarkers for the diagnosis and management of heart failure. Heart Fail Rev. (2022) 27(2):625–43. doi: 10.1007/s10741-021-10105-w
3. Savarese G, Becher PM, Lund LH, Seferovic P, Rosano GMC, Coats AJS. Global burden of heart failure: a comprehensive and updated review of epidemiology. Cardiovasc Res. (2023) 118(17):3272–87. doi: 10.1093/cvr/cvac013
4. Groenewegen A, Rutten FH, Mosterd A, Hoes AW. Epidemiology of heart failure. Eur J Heart Fail. (2020) 22(8):1342–56. doi: 10.1002/ejhf.1858
5. Souza ACDAH, Rosenthal MH, Moura FA, Divakaran S, Osborne MT, Hainer J, et al. Body composition, coronary microvascular dysfunction, and future risk of cardiovascular events including heart failure. JACC Cardiovasc Imaging. (2024) 17(2):179–91. doi: 10.1016/j.jcmg.2023.07.014
6. Zhang X, Sun Y, Li Y, Wang C, Wang Y, Dong M, et al. Association between visceral adiposity index and heart failure: a cross-sectional study. Clin Cardiol. (2023) 46(3):310–9. doi: 10.1002/clc.23976
7. Aryee EK, Ozkan B, Ndumele CE. Heart failure and obesity: the latest pandemic. Prog Cardiovasc Dis. (2023) 78:43–8. doi: 10.1016/j.pcad.2023.05.003
8. Scicchitano P, Massari F. The role of bioelectrical phase angle in patients with heart failure. Rev Endocr Metab Disord. (2023) 24(3):465–77. doi: 10.1007/s11154-022-09757-2
9. Wakabayashi I, Daimon T. The “cardiometabolic index” as a new marker determined by adiposity and blood lipids for discrimination of diabetes mellitus. Clin Chim Acta. (2015) 438:274e8. doi: 10.1016/j.cca.2014.08.042
10. Zuo Y-Q, Gao Z-H, Yin Y-L, Yang X, Feng P-Y. Association between the cardiometabolic index and hyperuricemia in an asymptomatic population with normal body mass index. Int J Gen Med. (2021) 14:8603–10. doi: 10.2147/IJGM.S340595
11. Ye R, Zhang X, Zhang Z, Wang S, Liu L, Jia S, et al. Association of cardiometabolic and triglyceride-glucose index with left ventricular diastolic function in asymptomatic individuals. Nutr Metab Cardiovasc Dis. (2024) 34(7):1590–600. doi: 10.1016/j.numecd.2024.02.008
12. Wang H, Sun Y, Wang S, Qian H, Jia P, Chen Y, et al. Body adiposity index, lipid accumulation product, and cardiometabolic index reveal the contribution of adiposity phenotypes in the risk of hyperuricemia among Chinese rural population. Clin Rheumatol. (2018) 37(8):2221–31. doi: 10.1007/s10067-018-4143-x
13. Miao M, Deng X, Wang Z, Jiang D, Lai S, Yu S, et al. Cardiometabolic index is associated with urinary albumin excretion and renal function in aged person over 60: data from NHANES 2011–2018. Int J Cardiol. (2023) 384:76–81. doi: 10.1016/j.ijcard.2023.04.017
14. Yan L, Hu X, Wu S, Cui C, Zhao S. Association between the cardiometabolic index and NAFLD and fibrosis. Sci Rep. (2024) 14(1):13194. doi: 10.1038/s41598-024-64034-3
15. Song J, Li Y, Zhu J, Liang J, Xue S, Zhu Z. Non-linear associations of cardiometabolic index with insulin resistance, impaired fasting glucose, and type 2 diabetes among US adults: a cross-sectional study. Front Endocrinol (Lausanne). (2024) 15:1341828. doi: 10.3389/fendo.2024.1341828
16. Lelonek M, Grabowski M, Kasprzak JD, Leszek P, Nessler J, Pawlak A, et al. An expert opinion of the heart failure association of the polish cardiac society on the 2021 European society of cardiology guidelines for the diagnosis and treatment of acute and chronic heart failure: heart failure guidelines from a national perspective. Kardiol Pol. (2022) 80(2):239–46. doi: 10.33963/KP.a2022.0021
17. Obokata M, Sorimachi H, Harada T, Kagami K, Saito Y, Ishii H. Epidemiology, pathophysiology, diagnosis, and therapy of heart failure with preserved ejection fraction in Japan. J Card Fail. (2023) 29(3):375–88. doi: 10.1016/j.cardfail.2022.09.018
18. Tutor AW, Lavie CJ, Kachur S, Milani RV, Ventura HO. Updates on obesity and the obesity paradox in cardiovascular diseases. Prog Cardiovasc Dis. (2023) 78:2–10. doi: 10.1016/j.pcad.2022.11.013
19. Powell-Wiley TM, Poirier P, Burke LE, Després JP, Gordon-Larsen P, Lavie CJ, et al. Obesity and cardiovascular disease: a scientific statement from the American Heart Association. Circulation. (2021) 143(21):e984–e1010. doi: 10.1161/CIR.0000000000000973
20. Alagiakrishnan K, Banach M, Ahmed A, Aronow WS. Complex relationship of obesity and obesity paradox in heart failure-higher risk of developing heart failure and better outcomes in established heart failure. Ann Med. (2016) 48(8):603–13. doi: 10.1080/07853890.2016.1197415
21. Rao VN, Fudim M, Mentz RJ, Michos ED, Felker GM. Regional adiposity and heart failure with preserved ejection fraction. Eur J Heart Fail. (2020) 22(9):1540–50. doi: 10.1002/ejhf.1956
22. Xu C, Guo Y, Zhang S, Lai Y, Huang M, Zhan R, et al. Visceral adiposity index and the risk of heart failure, late-life cardiac structure, and function in ARIC study. Eur J Prev Cardiol. (2023) 30(12):1182–92. doi: 10.1093/eurjpc/zwad099
23. Borlaug BA, Jensen MD, Kitzman DW, Lam CSP, Obokata M, Rider OJ. Obesity and heart failure with preserved ejection fraction: new insights and pathophysiological targets. Cardiovasc Res. (2023) 118(18):3434–50. doi: 10.1093/cvr/cvac120
24. Gibson S, Ashwell M. A simple cut-off for waist-to-height ratio (0.5) can act as an indicator for cardiometabolic risk: recent data from adults in the health survey for England. Br J Nutr. (2020) 123:681–90. doi: 10.1017/S0007114519003301
25. Chen J, Li M, Hao B, Cai Y, Li H, Zhou W, et al. Waist to height ratio is associated with an increased risk of mortality in Chinese patients with heart failure with preserved ejection fraction. BMC Cardiovasc Disord. (2021) 21(1):263. doi: 10.1186/s12872-021-02080-9
26. Gu Z, Zhu P, Wang Q, He H, Xu J, Zhang L, et al. Obesity and lipid-related parameters for predicting metabolic syndrome in Chinese elderly population. Lipids Health Dis. (2018) 17:289. doi: 10.1186/s12944-018-0927-x
27. Wang H, Li Z, Guo X, Chen Y, Chang Y, Chen S, et al. The impact of nontraditional lipid profiles on left ventricular geometric abnormalities in general Chinese population. BMC Cardiovasc Disord. (2018) 18:88. doi: 10.1186/s12872-018-0829-x
28. Tani S, Imatake K, Suzuki Y, Yagi T, Takahashi A, Monden M, et al. Triglyceride/high-density lipoprotein cholesterol ratio may be a better index of cardiometabolic risk in women than in men in Japan. Nutr Metab Cardiovasc Dis. (2024) 34(4):868–81. doi: 10.1016/j.numecd.2024.01.0
29. Wakabayashi I. Relationship between age and cardiometabolic index in Japanese men and women. Obes Res Clin Pract. (2018) 12(4):372–7. doi: 10.1016/j.orcp.2016.12.008
30. Berezin AE, Berezina TA, Hoppe UC, Lichtenauer M, Berezin AA. Methods to predict heart failure in diabetes patients. Expert Rev Endocrinol Metab. (2024) 19(3):241–56. doi: 10.1080/17446651.2024.2342812
Keywords: congestive heart failure, age, cardiometabolic index, visceral fat, NHANES
Citation: Luo X and Cai B (2024) Association between cardiometabolic index and congestive heart failure among US adults: a cross-sectional study. Front. Cardiovasc. Med. 11:1433950. doi: 10.3389/fcvm.2024.1433950
Received: 16 May 2024; Accepted: 26 August 2024;
Published: 10 September 2024.
Edited by:
Maryanne Caruana, University of Malta, MaltaReviewed by:
Sepiso Kenias Masenga, Mulungushi University, ZambiaMarco Matteo Ciccone, University of Bari Aldo Moro, Italy
Hiroki Teragawa, JR Hiroshima Hospital, Japan
Copyright: © 2024 Luo and Cai. This is an open-access article distributed under the terms of the Creative Commons Attribution License (CC BY). The use, distribution or reproduction in other forums is permitted, provided the original author(s) and the copyright owner(s) are credited and that the original publication in this journal is cited, in accordance with accepted academic practice. No use, distribution or reproduction is permitted which does not comply with these terms.
*Correspondence: Bin Cai, Y2FpYmluQHpqdS5lZHUuY24=