- 1The First Clinical College of Medicine, Lanzhou University, Lanzhou, China
- 2School of Stomatology, Lanzhou University, Lanzhou, China
- 3Graduate Department of Shanxi Medical University, Taiyuan, Shanxi, China
- 4The Second Affiliated Hospital of Fujian University of Traditional Chinese Medicine, Fuzhou, China
- 5Department of Anesthesia, First Hospital of Lanzhou University, Lanzhou, China
- 6Department of Orthopedics, Shenzhen Children’s Hospital, Shenzhen, Guangdong, China
- 7Department of Trauma Surgery, University Hospital Regensburg, Regensburg, Germany
Background: Hypercholesterolemia, a critical contributor to cardiovascular disease, is not fully understood in terms of its relationship with serum metabolites and their role in disease pathogenesis.
Methods: This study leveraged GWAS data to explore the relationship between serum metabolites and hypercholesterolemia, pinpointing significant metabolites via Mendelian Randomization (MR) and KEGG pathway enrichment analysis. Data on metabolites were sourced from a European population, with analysis focusing on individuals diagnosed with hypercholesterolemia.
Results: Out of 486 metabolites analyzed, ten showed significant associations with hypercholesterolemia, categorized into those enhancing risk and those with protective effects. Specifically, 2-methoxyacetaminophen sulfate and 1-oleoylglycerol (1-monoolein) were identified as risk-enhancing, with odds ratios (OR) of 1.545 (95% CI: 1.230–1.939; P_FDR = 3E−04) and 1.462 (95% CI: 1.036–2.063; P_FDR = 0.037), respectively. On the protective side, 3-(cystein-S-yl)acetaminophen, hydroquinone sulfate, and 2-hydroxyacetaminophen sulfate demonstrated ORs of 0.793 (95% CI: 0.735–0.856; P_FDR = 6.18E−09), 0.641 (95% CI: 0.423–0.971; P_FDR = 0.042), and 0.607 (95% CI: 0.541–0.681; P_FDR = 5.39E−17), respectively. In addition, KEGG pathway enrichment analysis further revealed eight critical pathways, comprising “biosynthesis of valine, leucine, and isoleucine”, “phenylalanine metabolism”, and “pyruvate metabolism”, emphasizing their significant role in the pathogenesis of hypercholesterolemia.
Conclusion: This study underscores the potential causal links between particular serum metabolites and hypercholesterolemia, offering innovative viewpoints on the metabolic basis of the disease. The identified metabolites and pathways offer promising targets for therapeutic intervention and warrant further investigation.
Introduction
Cardiovascular diseases (CVD) incur global losses exceeding $863 billion, imposing a substantial economic burden projected to reach $1, 044 billion by 2030 (1), thereby exacerbating the global disease burden. Hypercholesterolemia, a key factor in CVD, is marked by elevated levels of total cholesterol (TC) and low-density lipoprotein cholesterol (LDL-C) in the bloodstream. Prolonged duration of elevated LDL-C levels markedly heightens the potential for early-onset atherosclerotic cardiovascular diseases and increases the likelihood of premature mortality (2). Hypercholesterolemia represents a critical health concern, established as a primary cause of early-onset myocardial infarction (3). Prompt diagnosis and efficacious lipid-lowering therapy are essential for these patients (3–8).
Hypercholesterolemia is a metabolic disorder, and despite significant advancements in its treatment over the past two decades (9), its exact pathophysiology and specific biomarkers are still not well understood (10). Previous studies have suggested potential risk factors such as gut microbiota and cytokines (11–14), but research focusing on the overall metabolic alterations in hypercholesterolemia is relatively understudied.
In recent years, the field of metabolomics has garnered substantial attention within systems biology, offering essential understanding of the detailed biological processes behind various diseases, such as hypercholesterolemia (15, 16). Metabolomics represents a cutting-edge and efficient analytical approach that facilitates the concurrent quantification of thousands of small molecules within biological specimens (17). Located downstream of the genome, metabolomics offers essential insights into the dynamics of intricate biological systems. It is applied across a broad spectrum of health conditions, providing new perspectives on pathogenic processes and aiding in the identification of biomarkers for detection and treatment (18). Consequently, metabolomics promotes a comprehensive biological description of organisms (19, 20). The advancement of human genome sequencing technology has pioneered an innovative period of customized healthcare, in which genetic variations can forecast the effectiveness of specific therapeutic strategies, optimizing individual disease management (21).
Additionally, the targeted modulation of metabolites holds considerable promise for managing hypercholesterolemia. For instance, bile acids have been extensively studied. Bile acid sequestrants work by decreasing the enterohepatic recirculation of bile acids, resulting in a decrease of the bile acid pool by approximately 40%. This reduction stimulates the transformation of cholesterol into bile acids (cholesterol catabolism) and increases the elimination of bile acids through fecal matter (22–24). Consequently, the stimulation of cholesterol conversion to bile acids helps lower blood lipid levels. Therefore, the targeted metabolic regulation of bile acids emerges as an encouraging therapeutic approach for managing hypercholesterolemia (25).
Investigating metabolites linked to the initiation and development of hypercholesterolemia is essential not just for early detection and prevention but also essential for understanding the biological mechanisms underlying the treatment of hypercholesterolemia. Earlier research has explored the relationship between specific metabolites and diseases using metabolomic approaches (15, 16). Nonetheless, the causal link between metabolites and hypercholesterolemia is still ambiguous owing to the limited number of prospective studies on this topic. Conventional observational studies face design limitations, such as lifestyle modifications or long-term medication after the diagnosis of hypercholesterolemia, which can alter metabolite levels, thereby obscuring the causal link between metabolites and hypercholesterolemia. Despite being the premier method for establishing causality, randomized controlled trials present substantial challenges in this area, making it difficult to ascertain definitive conclusions on the causative link between metabolites and hypercholesterolemia.
Due to the various limitations of randomized controlled trials, Mendelian randomization has emerged as an effective alternative for inferring causal relationships (26). MR employs single nucleotide polymorphisms (SNPs) as proxies for exposure factors to robustly assess the causal relationship between exposures and outcomes (27). This approach simulates the random allocation in randomized controlled trials, as SNPs are randomly distributed in the population, thereby reducing the impact of confounding factors such as sex on the outcomes. Moreover, since genotypes are determined before disease onset, MR minimizes the risk of reverse causation (28).
Given the limited research on the relationship between blood metabolites and hypercholesterolemia, further studies are urgently needed. This study utilizes genome-wide association studies (GWAS) data for MR analysis to infer the causal relationship between 486 blood metabolites and hypercholesterolemia.
Materials and methods
Our study employs a two-sample Mendelian Randomization approach to rigorously assess the causative relationship between metabolites identified in individual blood samples and the risk of onset of hypercholesterolemia. An effective MR study necessitates meeting the following assumptions: 1. The Genetic instruments must be strongly correlated with the exposure; 2. Genetic instruments should remain unlinked to potential confounders; 3. Genetic instruments must influence the outcome solely through the exposure. Independence from horizontal pleiotropy, relevant to the second and third assumptions, can be assessed through a range of statistical techniques. We acquired data on hypercholesterolemia from the UK Biobank GWAS consortium, as outlined in Figure 1, offering a summary of the study's design.
Data source
The GWAS data on blood metabolites are derived from the study by Shin et al. (29), which included extensive metabolomic analyses. The investigation included 7,824 participants of European descent, analyzing approximately 2.1 million SNPs and encompassing 486 metabolites. Based on the KEGG database, we identified 278 metabolites with specific chemical characteristics and classified them into eight metabolic groups (30). The data for hypercholesterolemia were retrieved from the UK Biobank dataset, which included 463,010 participants. This includes 22,622 individuals diagnosed with hypercholesterolemia and 440,388 control subjects, all of whom are of European descent.
Instrument selection
In our study, we employed various strategies to identify SNPs associated with the exposure (metabolites). Due to the lack of significant associations, we adjusted the threshold to P < 1 × 10^−5. Additionally, we utilized pair-wise linkage disequilibrium (LD) pruning within a 10,000 kb range (r^2 < 0.001) to identify the top independent SNPs. Furthermore, SNPs associated with hypercholesterolemia or confounding factors such as sex, BMI, obesity, diabetes, hypertension, smoking and medication use were excluded using the Phenoscanner database (31). The SNPs ultimately used for MR analysis are detailed in Supplementary Table S4 of Supplementary File 1. This commonly used methodology has been applied in prior MR studies. In parallel, we evaluated the F-statistic for each SNP to evaluate its statistical robustness, thereby reducing bias from weak instruments. To guarantee all SNPs generated adequate variance for their associated metabolites, we removed weak instruments with an F-statistic below 10. We also eliminated SNPs that were missing or lacked suitable proxies. Ultimately, metabolites containing a minimum of three SNPs were chosen for further analysis.
MR analysis
The inverse variance weighting (IVW) method, is recognized as one of the most reliable techniques for MR estimation, despite its susceptibility to pleiotropy bias. In our research, we employed IVW with random effects as the primary method for MR analysis, adjusting the significance threshold to P < 0.05, to initially explore the association between metabolites and disease risk (hypercholesterolemia). Additionally, we employed five other MR analysis methods for examining our study outcomes. These MR analytical techniques were utilized to perform sensitivity analyses of our research outcomes.
Statistical analysis
Statistical analysis employed the “TwoSampleMR” package (version 0.5.7) within R software (version 4.3.1). Of the six MR methods detailed previously, we designated the results from the IVW method as the main MR estimate and assessed their consistency with outcomes from the other MR approaches. Finally, a leave-one-out analysis was implemented to identify any disproportionate impact of specific SNPs on the results of each MR study.
Visualization of the results
The conclusive findings are illustrated with a volcano plot, which succinctly represents the data by correlating the magnitude of effect with statistical significance. This graphical approach efficiently highlights metabolites of substantial impact on disease risk, delineating both the strength and reliability of their associations. For metabolites demonstrating significant causal relationships, forest plots are deployed. These graphical representations meticulously detail the effect sizes and confidence intervals for each metabolite, providing a precise comprehension of their relative significance and the confidence in these effects on disease risk.
Metabolic pathway analysis
Further examination of metabolites with notable differences involves KEGG pathway enrichment analysis. This analysis is supported by MetaboAnalyst 5.0, which examines the metabolic pathways of key circulating metabolites. This platform integrates bioinformatic data and computational techniques, facilitating comprehensive characterization of the biological roles of a diverse set of genes and proteins. Results with a P-value below 0.05 are considered statistically significant, whereas those with a P-value ranging from 0.05 to 0.10 are deemed to have potential statistical significance.
Results
Table 1 presents the GWAS data sources for this study. Following suitable screening, we selected 278 metabolites out of 486 for MR analysis; the other 208 metabolites were not identified (Supplementary File 1 includes Supplementary Table S1: A summary of causal effect estimates obtained through diverse MR methodologies; Supplementary Table S2: A summary of heterogeneity analysis results obtained through diverse MR methodologies; Supplementary Table S3: A summary of pleiotropy assessments obtained through diverse MR methodologies; Supplementary Table S4: A summary of the raw data; Supplementary Table S5: Detailed summaries of causal effect estimates for significant metabolites calculated using various MR methods. The SNP count per metabolite varied between 4 and 257. An important observation was that the F-statistics for all SNPs exceeded 10, suggesting that all instrumental variables were sufficiently strong.
The causal impact of circulating metabolites on hypercholesterolemia
In the investigation of the identified 278 metabolites through MR using the IVW method, 65 metabolites showed significant associations with Disease Risk (hypercholesterolemia) as illustrated in Figure 2 and detailed in Supplementary Table S5. Nonetheless, following numerous corrections for the False Discovery Rate (FDR) and comprehensive consideration, only ten metabolites remained significantly associated with hypercholesterolemia. Among these, 2-methoxyacetaminophen sulfate demonstrated an Odds Ratio (OR) per SD increase of 1.545 (95% CI: 1.230–1.939; P = 1.78E−04, P_FDR = 3E−04), while 1-oleoylglycerol (1-monoolein) had an OR per SD increase of 1.462 (95% CI: 1.036–2.063; P = 0.031, P_FDR = 0.037), indicating a significant correlation with a heightened risk of hypercholesterolemia.
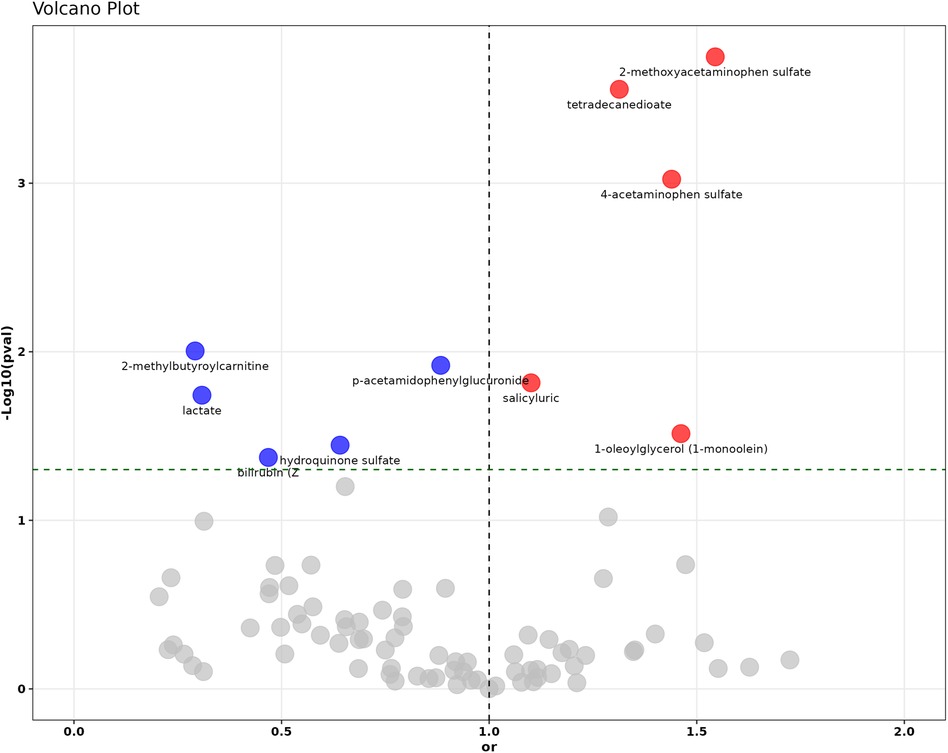
Figure 2 The volcano plot illustrates the causal relationship assessment and statistical significance of 278 circulating metabolites with hypercholesterolemia. Red dots represent statistically significant risk factors (OR >1), blue dots indicate statistically significant protective factors (OR <1), and gray dots denote metabolites without statistical significance.
Conversely, metabolites such as 3-(cystein-S-yl)acetaminophen exhibited an OR per SD increase of 0.793 (95% CI: 0.735–0.856; P = 2.39E-09, P_FDR = 6.18E-09), hydroquinone sulfate had an OR per SD increase of 0.641 (95% CI: 0.423–0.971; P = 0.035, P_FDR = 0.042), and epiandrosterone sulfate showed an OR per SD increase of 0.353 (95% CI: 0.305–0.400; P = 2.64E-45, P_FDR = 1.63E-44), demonstrated significant causal relationships with hypercholesterolemia, potentially offering protective effects. The detailed findings are presented in Figure 3, with additional information available in Supplementary Table S1.
Evaluation of heterogeneity and horizontal pleiotropy
In the assessment of heterogeneity related to 10 circulating metabolites significantly associated with hypercholesterolemia, heterogeneity was observed in 2-methoxyacetaminophen sulfate, 1-oleoylglycerol (1-monoolein), 3-(cystein-S-yl)acetaminophen, 2-hydroxyacetaminophen sulfate, and epiandrosterone sulfate. No evidence of heterogeneity was found in the additional circulating metabolites. Supplementary Table S2 provides the complete outcomes. Pleiotropy analysis showed that the effects of metabolites on hypercholesterolemia causality were not influenced by horizontal pleiotropy at the SNP level. The specific findings are presented in Supplementary Table S3. Additionally, findings from the LOO analysis verified that the MR estimates of individual SNPs were unbiased, as illustrated in Supplementary Figures S1–S10.
Metabolic pathway analysis
During the KEGG pathway enrichment analysis, significant changes in 74 circulating metabolites were assessed, leading to the identification of eight potential metabolic pathways linked to hypercholesterolemia. Of these pathways, the three most crucial were “Valine, leucine and isoleucine biosynthesis, “Phenylalanine metabolism,” and “Pyruvate metabolism,” with P-values of 0.00016584, 0.0058945, and 0.046341 (all P < 0.05). The fourth key pathway identified was also “Phenylalanine metabolism, “ playing a vital part in the context of hypercholesterolemia, as depicted in Figure 4.
Discussion
In this study, utilizing GWAS data, we identified 10 out of 486 blood metabolites linked to hypercholesterolemia. Of the identified metabolites, four metabolites exhibited harmful effects related to an increased risk of hypercholesterolemia, including 2-methoxyacetaminophen sulfate, 1-oleoylglycerol (1-monoolein), glycocholate, and salicyluric, while six metabolites showed protective effects against hypercholesterolemia, namely 3-(cystein-S-yl)acetaminophen, hydroquinone sulfate, 2-hydroxyacetaminophen sulfate, trans-4-hydroxyproline, Z-bilirubin, and epiandrosterone sulfate. Various analytical methods further validated these findings.
Hypercholesterolemia is a metabolic disorder marked by increased levels of TC and LDL-C in the blood. This condition adversely affects the cardiovascular system and can trigger cardiovascular diseases (2). While genetic, nutritional, and lifestyle factors contribute to the progression of hypercholesterolemia, its precise mechanisms remain not fully elucidated (32–34). Early diagnosis and intervention are crucial for preventing cardiovascular events, highlighting the necessity of reliable biomarkers to optimize therapeutic strategies for hypercholesterolemia.
Metabolomics has garnered widespread attention for its potential to identify metabolites associated with hypercholesterolemia. Blood metabolites can reveal both endogenous and exogenous metabolic activities, offering valuable insights into disease mechanisms. For example, Haihua Bai et al. utilized LC/MS for serum analysis of healthy controls and hypercholesterolemia patients, revealing significant differences in serum metabolites between these groups (15). Additionally, numerous studies have reported increased oxidative stress in hypercholesterolemia patients, particularly a reduction in the cellular antioxidant glutathione (35). Considering that the metabolomic alterations in blood mirror intracellular changes, it is crucial to precisely study metabolic regulation in different cell types of hypercholesterolemia patients. While the association between metabolites and hypercholesterolemia has been preliminarily established, further research is required to determine a definitive causal relationship and to gain a deeper insight into the fundamental mechanisms (16). These examinations could broaden our comprehension of hypercholesterolemia's development and may aid in devising robust strategies for early identification and prevention. Hence, we conducted this study to elucidate the causal link between blood metabolites and hypercholesterolemia, providing novel approaches for the screening and management of this condition.
In our study, we identified that genetic predispositions to four blood metabolites [2-methoxyacetaminophen sulfate, 1-oleoylglycerol (1-monoolein), glycocholate, and salicyluric] are linked to an increased risk of hypercholesterolemia. Despite limited reports discussing their impacts, some insights suggest further investigation is warranted. For instance, 2-methoxyacetaminophen sulfate, an acetamide compound, acts as a drug metabolite. Historically, acetamides have been considered negatively correlated with levels of glutathione and N-acetylcysteine (NAC) (36). NAC has been used to prevent cardiovascular conditions like coronary artery disease and myocardial infarction, primarily due to its antioxidant properties (37). Research has demonstrated that NAC pre-treatment can markedly suppress the conversion of mouse 3T3-L1 preadipocytes to adipocytes, thereby reducing intracellular lipid buildup and the expression of proteins related to obesity (38), indicating its important role in lipoprotein metabolism. Nevertheless, the precise roles and mechanisms of 2-methoxyacetaminophen sulfate are still not fully understood and require additional investigation. Notably, glycocholate, a bile acid, is associated with cardiovascular diseases. In the human body, bile acids are primarily synthesized from cholesterol (39). Substantial evidence links alterations in bile acid metabolism to cardiovascular diseases (40). Research indicates that bile acids can activate multiple signaling pathways, leading to elevated levels of total cholesterol, VLDL, and LDL, while reducing HDL levels (41–43). Currently, bile acid sequestrants targeting bile acids have been clinically used to treat hypercholesterolemia and have shown promising efficacy (25, 44).
Furthermore, our study identified that several metabolites, including 3-(cystein-S-yl)acetaminophen, hydroquinone sulfate, 2-hydroxyacetaminophen sulfate, trans-4-hydroxyproline, Z-bilirubin, and epiandrosterone sulfate, might have protective effects against hypercholesterolemia. Both 2-hydroxyacetaminophen sulfate and 3-(cystein-S-yl)acetaminophen are acetaminophen metabolites, and current research primarily focuses on their roles in liver injury (45, 46). Liver function impairment is associated with alterations in circulating lipids (47, 48), often manifesting as reduced levels of plasma cholesterol, lipoproteins, and triglycerides (49). Several observations support that patients with cirrhosis have significantly lower serum total cholesterol and TG levels compared to healthy individuals, possibly due to liver damage influencing clinical manifestations, including bacterial infections, hematological complications, malnutrition, and adrenal insufficiency (50). We speculate that the protective effects of 2-hydroxyacetaminophen sulfate and 3-(cystein-S-yl) acetaminophen on hypercholesterolemia may be mediated through liver injury mechanisms. Z-bilirubin, an isomer of bilirubin, forms as an ultimate product of heme catabolism (51). Studies over the past decade have shown that moderately elevated bilirubin levels can prevent metabolic and cardiovascular diseases (52–54). Studies have demonstrated that bilirubin treatment lowers total cholesterol levels in diet-induced obese mice (55), possibly by enhancing the ubiquitination and subsequent degradation of HMGCR, a key enzyme in cholesterol synthesis (56). Additionally, bilirubin alleviates oxidative stress by modulating Th17 immune responses and suppressing Toll-like receptor 4-induced ROS production (57, 58). Therapies targeting bilirubin have shown promising results in conditions such as pancreatitis, cardiac ischemia-reperfusion injury (IRI), and atherosclerosis (59, 60), suggesting potential efficacy for treating hypercholesterolemia. Epiandrosterone sulfate, a metabolite of dehydroepiandrosterone (DHEA), has been inversely associated with atherosclerosis in clinical studies (61). However, literature on the effects of epiandrosterone sulfate is limited, and its relationship with hypercholesterolemia warrants further investigation.
Furthermore, KEGG pathway enrichment analysis revealed eight significant metabolic pathways associated with hypercholesterolemia. These pathways include “Valine, leucine and isoleucine biosynthesis,” “Phenylalanine metabolism,” and “Pyruvate metabolism.” Notably, leucine, isoleucine, and valine have been significantly associated with atherosclerosis and are considered surrogate markers for this condition (62, 63). The valine metabolite, 3-hydroxyisobutyric acid, can stimulate trans-endothelial transport of fatty acids, resulting in localized lipid buildup and lipotoxicity (64). Additionally, BCAAs can disrupt mitochondrial pyruvate utilization by inhibiting the pyruvate dehydrogenase complex and reducing glucose oxidation (65), potentially causing the body to rely more on fatty acids and resulting in lipid metabolism disorders (66). Additionally, elevated phenylalanine levels can impact HMGR activity, subsequently inhibiting cholesterol synthesis (67). Pyruvate metabolism is essential to cholesterol production, functioning as a precursor for acetyl-coenzyme A, which is critical for cholesterol synthesis (68, 69). These metabolic disruptions may contribute to the development of hypercholesterolemia.
This MR analysis has several notable strengths. To our knowledge, this study is distinguished by its comprehensive examination of 486 blood metabolites and their possible effects on hypercholesterolemia. We applied stringent methodologies to mitigate potential issues and ensure the robustness of our findings. Various techniques were implemented to appropriately handle and exclude factors that violate MR assumptions. The study is committed to generating reliable estimates, as demonstrated by our careful management of potential confounders. Through the use of rigorous methodologies and the consideration of multiple variables, the researchers successfully produced dependable findings. Sensitivity analyses further validated our outcomes across different conditions and assumptions.
Our current research faces several challenges. First, Due to the categorization of the initial dataset, we were unable to evaluate the severity of hypercholesterolemia and had to analyze the condition in its entirety. Second, the analysis focused exclusively on individuals of European descent, thus our findings may not be generalizable to other populations; hence, subsequent research should seek to validate the metabolites identified in populations beyond European subjects to confirm the universality of our findings. Third, although our study has inferred associations between multiple metabolites and hypercholesterolemia, additional studies are required to elucidate the specific functions of these metabolites in the development of hypercholesterolemia.
Among these identified metabolites, Z-bilirubin has shown effective action against hypercholesterolemia and may offer a potential avenue for therapy. This study also revealed several metabolic pathways potentially associated with the development of hypercholesterolemia. These metabolites and their corresponding pathways could prove valuable in a medical setting for the early identification and prevention of hypercholesterolemia. These metabolites also hold promise as emerging treatment targets for hypercholesterolemia in the future.
Data availability statement
The original contributions presented in the study are included in the article/Supplementary Material, further inquiries can be directed to the corresponding author.
Author contributions
WW: Data curation, Writing – original draft, Writing – review & editing, Conceptualization, Formal Analysis, Funding acquisition, Investigation, Methodology, Project administration, Resources, Software, Supervision, Validation, Visualization. JQ: Writing – original draft, Writing – review & editing. ZS: Writing – original draft, Writing – review & editing. HW: Writing – original draft, Writing – review & editing. JW: Data curation, Funding acquisition, Methodology, Writing – review & editing. YL: Conceptualization, Data curation, Formal Analysis, Funding acquisition, Investigation, Methodology, Project administration, Resources, Software, Supervision, Validation, Visualization, Writing – original draft, Writing – review & editing. RL: Conceptualization, Data curation, Formal Analysis, Funding acquisition, Investigation, Methodology, Project administration, Resources, Software, Supervision, Validation, Visualization, Writing – original draft, Writing – review & editing. NM: Methodology, Writing – review & editing
Funding
The author(s) declare financial support was received for the research, authorship, and/or publication of this article.
This research was funded by the Joint Research Fund Project of Gansu Province (23JRRA1496), the Innovation and Entrepreneurship Action Plan of Lanzhou University (20230060103, 20230060114), the Medical Innovation and Development Project of Lanzhou University (lzuyxcx-2022-99), the National College Student Innovation and Entrepreneurship Program (202210730172), the Ministry of Science and Technology Senior Foreign Expert Program (G2019028023, G2020028007), the Sanming Project of Medicine in Shenzhen (SZSM202011012), and the Guangdong High-level Hospital Construction Fund. These grants were crucial in supporting our investigation into the intricate connections between serum metabolites and hypercholesterolemia.
Acknowledgments
We extend our profound gratitude to the researchers and participants involved in the GWAS work featured in this article. Special thanks to Professor Weilin Jin for his invaluable guidance throughout the research process. We also extend our gratitude to Weining Wang for providing useful suggestions during the review of our article.
Conflict of interest
The authors declare that the research was conducted in the absence of any commercial or financial relationships that could be construed as a potential conflict of interest.
Publisher's note
All claims expressed in this article are solely those of the authors and do not necessarily represent those of their affiliated organizations, or those of the publisher, the editors and the reviewers. Any product that may be evaluated in this article, or claim that may be made by its manufacturer, is not guaranteed or endorsed by the publisher.
Supplementary material
The Supplementary Material for this article can be found online at: https://www.frontiersin.org/articles/10.3389/fcvm.2024.1410006/full#supplementary-material
References
1. Ding D, Lawson KD, Kolbe-Alexander TL, Finkelstein EA, Katzmarzyk PT, van Mechelen W, et al. The economic burden of physical inactivity: a global analysis of major non-communicable diseases. Lancet (London, England). (2016) 388(10051):1311–24. doi: 10.1016/S0140-6736(16)30383-X
3. Brænne I, Kleinecke M, Reiz B, Graf E, Strom T, Wieland T, et al. Systematic analysis of variants related to familial hypercholesterolemia in families with premature myocardial infarction. Eur J Hum Genet. (2016) 24(2):191–7. doi: 10.1038/ejhg.2015.100
4. Nordestgaard BG, Chapman MJ, Humphries SE, Ginsberg HN, Masana L, Descamps OS, et al. Familial hypercholesterolaemia is underdiagnosed and undertreated in the general population: guidance for clinicians to prevent coronary heart disease: consensus statement of the European atherosclerosis society. Eur Heart J. (2013) 34(45):3478–90a. doi: 10.1093/eurheartj/eht273
5. Hegele RA, Borén J, Ginsberg HN, Arca M, Averna M, Binder CJ, et al. Rare dyslipidaemias, from phenotype to genotype to management: a European atherosclerosis society task force consensus statement. Lancet Diabetes Endocrinol. (2020) 8(1):50–67. doi: 10.1016/S2213-8587(19)30264-5
6. Kayıkçıoğlu M, Kısmalı E, Can L, Payzin S. Long-term follow-up in patients with homozygous familial hypercholesterolemia; 13-year experience of a university hospital lipid clinic. Turk Kardiyol Dern Ars. (2014) 42(7):599–611. doi: 10.5543/tkda.2014.09633
7. Defesche JC, Gidding SS, Harada-Shiba M, Hegele RA, Santos RD, Wierzbicki ASJNrDp. Familial hypercholesterolaemia. Nat Rev Dis Primers. (2017) 3(1):1–20. doi: 10.1038/nrdp.2017.93
8. Kayikcioglu M, Tokgozoglu L, Dogan V, Ceyhan C, Tuncez A, Kutlu M, et al. What have we learned from Turkish familial hypercholesterolemia registries (A-HIT1 and A-HIT2)? Atherosclerosis. (2018) 277:341–6. doi: 10.1016/j.atherosclerosis.2018.08.012
9. Lui DTW, Lee ACH, Tan KCB. Management of familial hypercholesterolemia: current status and future perspectives. J Endocr Soc. (2021) 5(1):bvaa122. doi: 10.1210/jendso/bvaa122
10. Sniderman AD, Glavinovic T, Thanassoulis G. Key questions about familial hypercholesterolemia: JACC review topic of the week. J Am Coll Cardiol. (2022) 79(10):1023–31. doi: 10.1016/j.jacc.2022.01.010
11. Yan S, Chen J, Zhu L, Guo T, Qin D, Hu Z, et al. Oryzanol alleviates high fat and cholesterol diet-induced hypercholesterolemia associated with the modulation of the gut microbiota in hamsters. Food Funct. (2022) 13(8):4486–501. doi: 10.1039/D1FO03464B
12. Liu D, Pi J, Zhang B, Zeng H, Li C, Xiao Z, et al. Phytosterol of lotus seed core powder alleviates hypercholesterolemia by regulating gut microbiota in high-cholesterol diet-induced C57BL/6J mice. Food Biosci. (2023) 51:102279. doi: 10.1016/j.fbio.2022.102279
13. Yang C, Huang S, Lin Z, Chen H, Xu C, Lin Y, et al. Polysaccharides from enteromorpha prolifera alleviate hypercholesterolemia via modulating the gut microbiota and bile acid metabolism. Food Funct. (2022) 13(23):12194–207. doi: 10.1039/D2FO02079C
14. Collado A, Domingo E, Piqueras L, Sanz M-J. Primary hypercholesterolemia and development of cardiovascular disorders: cellular and molecular mechanisms involved in low-grade systemic inflammation and endothelial dysfunction. Int J Biochem Cell Biol. (2021) 139:106066. doi: 10.1016/j.biocel.2021.106066
15. Zhao R, Tang Y, Cao W, Zhao L, Wu Z, Chen X, et al. Identification of multiple plasma lipids as diagnostic biomarkers of hypercholesterolemia and the underlying mechanisms based on pseudo-targeted lipidomics. Rapid Commun Mass Spectrom. (2024) 38(9):e9723. doi: 10.1002/rcm.9723
16. Gu P-S, Su K-W, Yeh K-W, Huang J-L, Lo F-S, Chiu C-Y. Metabolomics analysis reveals molecular signatures of metabolic complexity in children with hypercholesterolemia. Nutrients. (2023) 15(7):1726. doi: 10.3390/nu15071726
17. Yang Q, Zhang A-H, Miao J-H, Sun H, Han Y, Yan G-L, et al. Metabolomics biotechnology, applications, and future trends: a systematic review. RSC Adv. (2019) 9(64):37245–57. doi: 10.1039/C9RA06697G
18. Medina S, Dominguez-Perles R, Gil JI, Ferreres F, Gil-Izquierdo A. Metabolomics and the diagnosis of human diseases–a guide to the markers and pathophysiological pathways affected. Curr Med Chem. (2014) 21(7):823–48. doi: 10.2174/0929867320666131119124056
19. Chu SH, Huang M, Kelly RS, Benedetti E, Siddiqui JK, Zeleznik OA, et al. Integration of metabolomic and other omics data in population-based study designs: an epidemiological perspective. Metabolites. (2019) 9(6):117. doi: 10.3390/metabo9060117
20. Lin C, Tian Q, Guo S, Xie D, Cai Y, Wang Z, et al. Metabolomics for clinical biomarker discovery and therapeutic target identification. Molecules (Basel, Switzerland). (2024) 29(10):2198. doi: 10.3390/molecules29102198
21. Newgard CB. Metabolomics and metabolic diseases: where do we stand? Cell Metab. (2017) 25(1):43–56. doi: 10.1016/j.cmet.2016.09.018
22. Chiang JYL. Bile acid metabolism and signaling. Compr Physiol. (2013) 3(3):1191. doi: 10.1002/cphy.c120023
23. Feng Y, Li Q, Ou G, Yang M, Du L. Bile acid sequestrants: a review of mechanism and design. J Pharm Pharmacol. (2021) 73(7):855–61. doi: 10.1093/jpp/rgab002
24. Einarsson K, Ericsson S, Ewerth S, Reihner E, Rudling M, Ståhlberg D, et al. Bile acid sequestrants: mechanisms of action on bile acid and cholesterol metabolism. Eur J Clin Pharmacol. (1991) 40(Suppl 1):S53–8. doi: 10.1007/BF03216291
25. Islam MS, Sharif A, Kwan N, Tam KC. Bile acid sequestrants for hypercholesterolemia treatment using sustainable biopolymers: recent advances and future perspectives. Mol Pharm. (2022) 19(5):1248–72. doi: 10.1021/acs.molpharmaceut.2c00007
26. Zuccolo L, Holmes MV. Commentary: mendelian randomization-inspired causal inference in the absence of genetic data. Int J Epidemiol. (2017) 46(3):962–5. doi: 10.1093/ije/dyw327
27. Richmond RC, Smith GD. Mendelian randomization: concepts and scope. Cold Spring Harbor Perspect Med. (2022) 12(1):a040501. doi: 10.1101/cshperspect.a040501
28. Burgess S, Butterworth A, Thompson SG. Mendelian randomization analysis with multiple genetic variants using summarized data. Genet Epidemiol. (2013) 37(7):658–65. doi: 10.1002/gepi.21758
29. Shin S-Y, Fauman EB, Petersen A-K, Krumsiek J, Santos R, Huang J, et al. An atlas of genetic influences on human blood metabolites. Nat Genet. (2014) 46(6):543–50. doi: 10.1038/ng.2982
30. Kanehisa M, Goto S, Sato Y, Furumichi M, Tanabe M. KEGG for integration and interpretation of large-scale molecular data sets. Nucleic Acids Res. (2012) 40(Database issue):D109–14. doi: 10.1093/nar/gkr988
31. Kamat MA, Blackshaw JA, Young R, Surendran P, Burgess S, Danesh J, et al. Phenoscanner V2: an expanded tool for searching human genotype-phenotype associations. Bioinformatics. (2019) 35(22):4851–3. doi: 10.1093/bioinformatics/btz469
32. Vrablik M, Tichý L, Freiberger T, Blaha V, Satny M, Hubacek JA. Genetics of familial hypercholesterolemia: new insights. Front Genet. (2020) 11:574474. doi: 10.3389/fgene.2020.574474
33. Cha D, Park Y. Association between dietary cholesterol and their food sources and risk for hypercholesterolemia: the 2012–2016 Korea national health and nutrition examination survey. Nutrients. (2019) 11(4):846. doi: 10.3390/nu11040846
34. Febriani D, Besral. The effect of lifestyle on hypercholesterolemia. Open Public Health J. (2018) 11(1):TOPHJ-11-526. doi: 10.2174/1874944501811010526
35. Real JT, Martínez-Hervás S, Tormos MC, Domenech E, Pallardó FV, Sáez-Tormo G, et al. Increased oxidative stress levels and normal antioxidant enzyme activity in circulating mononuclear cells from patients of familial hypercholesterolemia. Metab Clin Exp. (2010) 59(2):293–8. doi: 10.1016/j.metabol.2009.07.026
36. Mitchell JR, Jollow DJ, Potter WZ, Gillette JR, Brodie BB. Acetaminophen-induced hepatic necrosis. IV. Protective role of glutathione. J Pharmacol Exp Ther. (1973) 187(1):211–7. PMID: 4746329.4746329
37. Cui Y, Zhu Q, Hao H, Flaker GC, Liu Z. N-acetylcysteine and atherosclerosis: promises and challenges. Antioxidants. (2023) 12(12):2073. doi: 10.3390/antiox12122073
38. Calzadilla P, Gómez-Serrano M, García-Santos E, Schiappacasse A, Abalde Y, Calvo JC, et al. N-acetylcysteine affects obesity-related protein expression in 3T3-L1 adipocytes. Redox Rep. (2013) 18(6):210–8. doi: 10.1179/1351000213Y.0000000066
39. Al-Ani Z, Ko J, Petrov MS. Relationship of serum bile acids with fat deposition in the pancreas, liver, and skeletal muscle. Clin Exp Gastroenterol. (2023) 16:137–46. doi: 10.2147/CEG.S422995
40. Zhang R, Ma W-Q, Fu M-J, Li J, Hu C-H, Chen Y, et al. Overview of bile acid signaling in the cardiovascular system. World J Clin Cases. (2021) 9(2):308. doi: 10.12998/wjcc.v9.i2.308
41. Brown JM, Hazen SL. Microbial modulation of cardiovascular disease. Nat Rev Microbiol. (2018) 16(3):171–81. doi: 10.1038/nrmicro.2017.149
42. Staudinger JL, Goodwin B, Jones SA, Hawkins-Brown D, MacKenzie KI, LaTour A, et al. The nuclear receptor PXR is a lithocholic acid sensor that protects against liver toxicity. Proc Natl Acad Sci USA. (2001) 98(6):3369–74. doi: 10.1073/pnas.051551698
43. Zhou C, King N, Chen KY, Breslow JL. Activation of PXR induces hypercholesterolemia in wild-type and accelerates atherosclerosis in apoE deficient mice. J Lipid Res. (2009) 50(10):2004–13. doi: 10.1194/jlr.M800608-JLR200
44. Esan O, Viljoen A, Wierzbicki AS. Colesevelam–a bile acid sequestrant for treating hypercholesterolemia and improving hyperglycemia. Expert Opin Pharmacother. (2022) 23(12):1363–70. doi: 10.1080/14656566.2022.2112945
45. Matthews AM, Roberts DW, Hinson JA, Pumford NR. Acetaminophen-induced hepatotoxicity. Analysis of total covalent binding vs. Specific binding to cysteine. Drug Metab Dispos. (1996) 24(11):1192–6. PMID: 8937852.8937852
46. James LP, Mayeux PR, Hinson JA. Acetaminophen-induced hepatotoxicity. Drug Metab Dispos. (2003) 31(12):1499–506. doi: 10.1124/dmd.31.12.1499
47. Marzo A, Ghirardi P, Sardini D, Prandini BD, Albertini A. Serum lipids and total fatty acids in chronic alcoholic liver disease at different stages of cell damage. Klin Wochenschr. (1970) 48:949–50. doi: 10.1007/BF01487639
48. McIntyre N. Plasma lipids and lipoproteins in liver disease. Gut. (1978) 19(6):526–30. doi: 10.1136/gut.19.6.526
49. Agorastos J, Boswell C, Harry DS, McIntyre N. Plasma Lipoproteins in Liver Disease. London, UK: Portland Press Ltd (1976).
50. Privitera G, Spadaro L, Marchisello S, Fede G, Purrello F. Abnormalities of lipoprotein levels in liver cirrhosis: clinical relevance. Dig Dis Sci. (2018) 63:16–26. doi: 10.1007/s10620-017-4862-x
51. Kang SJ, Lee C, Kruzliak P. Effects of serum bilirubin on atherosclerotic processes. Ann Med. (2014) 46(3):138–47. doi: 10.3109/07853890.2014.895588
52. Creeden JF, Gordon DM, Stec DE, Hinds TD Jr. Bilirubin as a metabolic hormone: the physiological relevance of low levels. Am J Physiol Endocrinol Metab. (2021) 320(2):E191–207. doi: 10.1152/ajpendo.00405.2020
53. Hinds TD Jr, Stec DE. Bilirubin safeguards cardiorenal and metabolic diseases: a protective role in health. Curr Hypertens Rep. (2019) 21(11):87. doi: 10.1007/s11906-019-0994-z
54. Hinds TD Jr, Stec DE. Bilirubin, a cardiometabolic signaling molecule. Hypertension. (2018) 72(4):788–95. doi: 10.1161/HYPERTENSIONAHA.118.11130
55. Liu J, Dong H, Zhang Y, Cao M, Song L, Pan Q, et al. Bilirubin increases insulin sensitivity by regulating cholesterol metabolism, adipokines and PPARγ levels. Sci Rep. (2015) 5(1):9886. doi: 10.1038/srep09886
56. Wen G, Yao L, Hao Y, Wang J, Liu J. Bilirubin ameliorates murine atherosclerosis through inhibiting cholesterol synthesis and reshaping the immune system. J Transl Med. (2022) 20:1–18. doi: 10.1186/s12967-021-03207-4
57. Idelman G, Smith DLH, Zucker SD. Bilirubin inhibits the up-regulation of inducible nitric oxide synthase by scavenging reactive oxygen species generated by the toll-like receptor 4-dependent activation of NADPH oxidase. Redox Biol. (2015) 5:398–408. doi: 10.1016/j.redox.2015.06.008
58. Longhi MS, Vuerich M, Kalbasi A, Kenison JE, Yeste A, Csizmadia E, et al. Bilirubin suppresses Th17 immunity in colitis by upregulating CD39. JCI insight. (2017) 2(9):e92791. doi: 10.1172/jci.insight.92791
59. Adin CA. Bilirubin as a therapeutic molecule: challenges and opportunities. Antioxidants. (2021) 10(10):1536. doi: 10.3390/antiox10101536
60. Nocentini A, Bonardi A, Pratesi S, Gratteri P, Dani C, Supuran CT. Pharmaceutical strategies for preventing toxicity and promoting antioxidant and anti-inflammatory actions of bilirubin. J Enzyme Inhib Med Chem. (2022) 37(1):487–501. doi: 10.1080/14756366.2021.2020773
61. Jia X, Sun C, Tang O, Gorlov I, Nambi V, Virani SS, et al. Plasma dehydroepiandrosterone sulfate and cardiovascular disease risk in older men and women. J Clin Endocrinol Metab. (2020) 105(12):e4304–27. doi: 10.1210/clinem/dgaa518
62. Shah SH, Bain JR, Muehlbauer MJ, Stevens RD, Crosslin DR, Haynes C, et al. Association of a peripheral blood metabolic profile with coronary artery disease and risk of subsequent cardiovascular events. Circ Cardiovasc Genet. (2010) 3(2):207–14. doi: 10.1161/CIRCGENETICS.109.852814
63. Yang R, Dong J, Zhao H, Li H, Guo H, Wang S, et al. Association of branched-chain amino acids with carotid intima-media thickness and coronary artery disease risk factors. PLoS One. (2014) 9(6):e99598. doi: 10.1371/journal.pone.0099598
64. Jang C, Oh SF, Wada S, Rowe GC, Liu L, Chan MC, et al. A branched-chain amino acid metabolite drives vascular fatty acid transport and causes insulin resistance. Nat Med. (2016) 22(4):421–6. doi: 10.1038/nm.4057
65. Karusheva Y, Strassburger K, Markgraf DF, Zaharia O-P, Bódis K, Kössler T, et al. Branched-chain amino acids associate negatively with postprandial insulin secretion in recent-onset diabetes. J Endocr Soc. (2021) 5(6):bvab067. doi: 10.1210/jendso/bvab067
66. Biswas D, Duffley L, Pulinilkunnil T. Role of branched-chain amino acid-catabolizing enzymes in intertissue signaling, metabolic remodeling, and energy homeostasis. FASEB J. (2019) 33(8):8711–31. doi: 10.1096/fj.201802842RR
67. Shefer S, Tint GS, Jean-Guillaume D, Daikhin E, Kendler A, Nguyen LB, et al. Is there a relationship between 3-hydroxy-3-methylglutaryl coenzyme a reductase activity and forebrain pathology in the PKU mouse? J Neurosci Res. (2000) 61(5):549–63. doi: 10.1002/1097-4547(20000901)61:5%3C549::AID-JNR10%3E3.0.CO;2-0
68. Cha J-Y, Lee H-J. Targeting lipid metabolic reprogramming as anticancer therapeutics. J Cancer Prev. (2016) 21(4):209–15. doi: 10.15430/JCP.2016.21.4.209
Keywords: Mendelian randomization, causality, hypercholesterolemia, metabolites, treatment
Citation: Wang W, Qiao J, Su Z, Wei H, Wu J, Liu Y, Lin R and Michael N (2024) Serum metabolites and hypercholesterolemia: insights from a two-sample Mendelian randomization study. Front. Cardiovasc. Med. 11: 1410006. doi: 10.3389/fcvm.2024.1410006
Received: 31 March 2024; Accepted: 12 July 2024;
Published: 25 July 2024.
Edited by:
Venkatesh Sundararajan, West Virginia University, United StatesReviewed by:
Sithara Thomas, University of Texas Health Science Center at Houston, United StatesSoumya Krishnan, Downstate Health Sciences University, United States
© 2024 Wang, Qiao, Su, Wei, Wu, Liu, Lin and Michael. This is an open-access article distributed under the terms of the Creative Commons Attribution License (CC BY). The use, distribution or reproduction in other forums is permitted, provided the original author(s) and the copyright owner(s) are credited and that the original publication in this journal is cited, in accordance with accepted academic practice. No use, distribution or reproduction is permitted which does not comply with these terms.
*Correspondence: Yatao Liu, doctorliuyt@163.com
†These authors have contributed equally to this work