- 1Center for Individualized and Genomic Medicine Research, Henry Ford Hospital, Detroit, MI, United States
- 2Department of Public Health Science, Henry Ford Health, Detroit, MI, United States
- 3Department of Clinical Pharmacy, University of Michigan College of Pharmacy, Ann Arbor, MI, United States
- 4Cardiovascular Division, Department of Medicine, Henry Ford Hospital, Detroit, MI, United States
- 5Translational Research Institute, Advent Health, Orlando, FL, United States
Background: Heart failure with reduced ejection fraction (HFrEF) remains a significant public health issue, with the disease advancing despite neurohormonal antagonism. Energetic dysfunction is a likely contributor to residual disease progression, and we have previously reported a strong association of plasma metabolite profiles with survival among patients with HFrEF. However, the genetic and biologic mechanisms that underlie the metabolite-survival association in HFrEF were uncertain.
Methods and results: We performed genetic mapping of the key metabolite parameters, followed by mediation analyses of metabolites and genotypes on survival, and genetic pathway analyses. Patients with HFrEF (n = 1,003) in the Henry Ford Pharmacogenomic Registry (HFPGR; 500 self-reported Black/African race patients [AA], 503 self-reported White/European race patients [EA], and 249 deaths over a median of 2.7 years) with genome-wide genotyping and targeted metabolomic profiling of plasma were included. We tested genome-wide association (GWA) of single nucleotide polymorphisms (SNPs) with the prognostic metabolite profile (PMP) and its components; first stratified by race, and then combined via meta-analysis for the entire cohort. Seven independent loci were identified as GWA significant hits in AA patients (3 for PMP and 4 for individual metabolites), one of which was also significant in the entire cohort (rs944469). No genome wide significant hits were found in White/EA patients. Among these SNPs, only rs35792152, (a hit for 3.HBA) tended to be associated with mortality in standard survival analysis (HR = 1.436, p = 0.052). The mediation analyses indicated several significant associations between SNPs, metabolites, and mortality in AA patients. Functional annotation mapping (FUMA) implicated inflammation, DNA metabolic, and mRNA splicing processes.
Conclusions: GWAS of key metabolites and survival along with FUMA pathway analysis revealed new candidate genes which unveiled molecular pathways that contribute to HF disease progression via metabolic and energetic abnormalities.
Introduction
Heart failure (HF) is one of the most common, costly, and deadly diseases. According to the American Heart Association, there are almost 900,000 new cases annually in the U.S., an estimated prevalence of 5.7 million (1). HF remains high risk even in the setting of optimal guideline directed medical therapy (2, 3). Therefore, a deeper understanding of the residual HF disease burden and associated pathophysiology toward novel interventions is critically needed. Energetics, mitochondrial function, and substrate utilization are perturbed in HFrEF and likely contribute to disease progression (4–6). However, the pathways that remain incompletely understood and are not entirely related to the well-established neurohormonal abnormalities (7). Certain circulating metabolites are associated with HF disease progression and outcomes (8–11). Similarly, a validated multi-metabolite profile (13 metabolites, HR = 1.76) is an independent predictor of mortality in HFrEF patients. This supports the notion of a key role for energetic abnormalities in HFrEF and identifies these circulating metabolites as potentially valuable surrogate markers.
While genome-wide association studies (GWAS) have illuminated disease mechanisms in many conditions, GWAS on clinical HF phenotype alone requires very large sample sizes and lacks a mechanistic focus, limiting downstream interpretation. On the other hand, GWAS power may be enhanced when focused on important mechanistic surrogates for the disease, sometimes termed “endophenotypes”. For example, this approach has been successful in asthma (12) identifying novel causative pathways for hay fever (13), and in Alzheimer's disease where it allowed identification of novel variants with increased Alzheimer's Disease (14). In the case of HF, there have been a few candidate genes discovered in large GWAS studies (15), and yet, this approach may not be sensitive. Relevant to this, metabolomics has proven to be an advantageous approach in obtaining unique insights about underlying disease states (16–21). Data from both ours and other studies show that the plasma metabolite profile is associated with HF prognosis (21, 22). Hence, plasma metabolite levels in HF patients could be an attractive endophenotype to map in order to better understand the genetic and mechanistic underpinnings of HF progression.
In this study, we tested genetic association of prognostic metabolites and assessed mediation between the genetic factors and metabolites with survival outcomes. We then performed pathway analysis to identify key processes and groups of related genes that may be novel therapeutic targets in HF.
Methods
Sample collection
Participants for this study were enrolled into the Henry Ford heart failure Pharmacogenomic registry (HFPGR), the details of which are previously described (22). The study was approved by the Institutional Review Board at Henry Ford Health System in Detroit, MI, USA. After being educated about the study all participants provided written informed consent at the time of enrollment. Briefly, the registry consists of >1,700 HF patients in total that met the Framingham criteria for HF and who were covered for at least 1 year by the affiliated health insurance plan (the “Health Alliance Plan”) and primarily received their care through Henry Ford Health System. All patients have detailed clinical and electronic health record data, claims collected as part of the registry, and had donated blood for DNA and biomarker analyses. At the time of enrollment, fasting blood samples were collected and centrifuged to isolate plasma, aliquoted and stored at −70 °C until being assayed. This study is focused on HFrEF patients defined here as having at least one an ejection fraction (EF) <50% measured by any modality. From this registry, we collected patients with reduced EF, and they were separated into two groups according to their self-reported race: White (EA) and African American (AA). After quality control (QC), i.e., accounting for missing SNP and metabolite data, there were a total of 1,003 participants (500 AA and 503 EA) for this study.
DNA genotyping
Genotyping was performed on all enrolled participants using the Axiom Biobank array (Affymetrix Inc, Santa Clara, CA). This array contained ∼600k SNPs including: (1) 300k GWAS markers with minor allele frequencies >1%, (2) >250k with low frequency (<1%) coding variants from the exome sequencing project, and (3) additional 50k variants to improve African race coverage (YRI Booster). This array provides excellent coverage of genomic variation, capturing 90% in European race and 80% in African race patients; and it also allows for ancestral quantification and admixture mapping. Standard quality control steps were adopted to clean the raw genotypes from the array (23). Clean genotypes were submitted to Michigan Imputation Server for imputation to 1,000 Genome Reference panel Phase 3 v5 (24). To avoid population differences, imputations were done separately for EA and AA. Only high-quality genotyped and imputed SNPs were included in the downstream association analysis. Minor allele frequency (MAF) cut-off was set at 0.05, and imputation score (r2) cut-off was set at 0.5.
Metabolomic profiling
Quantitative and targeted metabolite profiling of individual amino acids (AA), organic acids (OA), and acylcarnitines (AC) were performed at the Sanford Burnham Prebys Medical Discovery Institute using HPLC/mass spectrometry or GC/mass spectrometry (22). Targeted metabolite profiling of 8 OAs, 23 AAs, and 57 ACs were measured using plasma samples (see Supplementary Material). A prognostic metabolite profile (PMP) that significantly predicted HF mortality was derived and validated in our cohort (22). This PMP was the primary independent variable for the metabolite quantitative trait loci (mQTL) GWAS. As secondary GWAS analyses, we analyzed the 13 individual metabolites that had significant independent association to mortality and were included in the PMP [Arginine, Leucine, Phenylalanine, Valine, C2, C4.Isobutyryl, C5.Isovaleryl, C5.DC, C18.1, 3-hydroxy butyric acid (3HBA), Succinate, Fumarate, and α-ketoglutarate (KG)]. We used these 14 molecular phenotypes (i.e., the PMP and each of the 13 individual metabolites) to search for mQTL associations in this study.
Statistical analyses
To perform genome-wide association analysis on the metabolites that are potential endotypes of heart failure survival, typical GWAS protocol was used to link genome-wide SNPs with each metabolite and overall PMP. SNPs were coded as 0, 1 or 2 copies of the minor alleles. The primary analysis was performed for PMP and then secondary analyses for each of its component metabolites. A linear regression model implemented in PLINK was tested between SNP dosage and quantitative traits, with adjustment of the top 5 principal components (PC) (25). PCs were estimated from linkage disequilibrium pruned SNP sets using R package “Genesis” (26). Single variant association was done separately for EA and AA. To adopt a more powerful two-stage GWAS analysis across ethnic groups, AA GWAS was used as discovery analysis, while EA GWAS was used for replication. METAL was then used to combine their summary statistics together by fixed-effect meta-analysis (27). The significance threshold was set at 5 × 10−8 for SNP-level analysis.
Bioinformatics functional annotation and pathway analyses
SNPs reaching genome-wide significance were interrogated for functional annotation (gene disrupting, expression quantitative trait locus, genomic regulation) that may link to HF pathology by public resources including The Encyclopedia of DNA Elements (ENCODE), Genotype-Tissue Expression (GTEx) and Human Metabolome database (HMDB) (28, 29) and metabolomics GWAS server (http://metabolomics.helmholtz-muenchen.de/gwas/).
Gene and gene-set association analyses were performed to identify functional pathways enriched by significant SNPs or genes. ENCODE annotation was used to mark functional genomic regions/genes. Combining the evidence of association from all SNPs within each gene/region was done using an extended Simes procedure to yield an overall gene-based association p-value (30). The gene-focused approach enabled assessment of pathways to allow investigation of multi-site effects and leverage other pre-existing data such as known protein-protein interactions or regulatory pathways (i.e., FUMA).
Mediation analysis and heart failure mortality
Two lists of SNPs were included in the mediation analysis, which was used to build up the causal relationship between candidate SNPs, metabolites, and HF mortality. The first list was from mQTL analysis in this study, and the second list was from another independent GWAS on HF survival phenotype. Metabolites were treated as mediators, and HF mortality was treated as the outcome. Both model-based mediation analysis and its corresponding sensitivity analysis were done by the R package “Mediation” (31). A significant mediation effect was defined when mediation analysis returned a p-value < 0.01 and sensitivity analysis returned a p-value >0.05. The top associated SNPs, genes, or pathways were tested for mortality association in Cox proportional models adjusted for the first 5 PCs, clinical risk score (MAGGIC score) (32) and NT-proBNP levels. Multiple testing was corrected by Bonferroni method using the total number of genetic risk variables tested (i.e., the total number of hits from mQTL analysis).
Results
The baseline characteristics for the HFrEF patients in our analytic cohort are shown in Table 1. Among a total of 1,003 patients, 35% were female, 49% self-identified as AA, and the cohort average age was 68 years. The average ejection fraction was 34.9%, and 57% of the cohort had HF of ischemic origin.
We initially performed systematic genetic analysis for the multi-metabolite profile metabolite levels (PMP) in HFrEF patients, these results are depicted in Figure 1. Among AA participants, 3 SNPs were identified that met whole-genome significance (p < 5 × 10−8), while no SNPs were significant among EA participants (Table 2). Among the 3 significant SNPs, one was located in a protein coding gene (ARHGEF28) and the other two were in inter-genic regions. We then examined the individual metabolites that make up the previously published prognostic profile. An additional 4 independent SNPs were identified as GWAS hits for human metabolites in AA HFrEF patients. The multi-race meta-analytic GWAS resulted in no SNPs meeting genome wide significance. No significant SNPs were identified in the EA patients. Among AA patients, one metabolite hit, rs35792152 for 3-HBA (Figure 2), was moderately associated with HF mortality (HR = 1.436, p = 0.052).
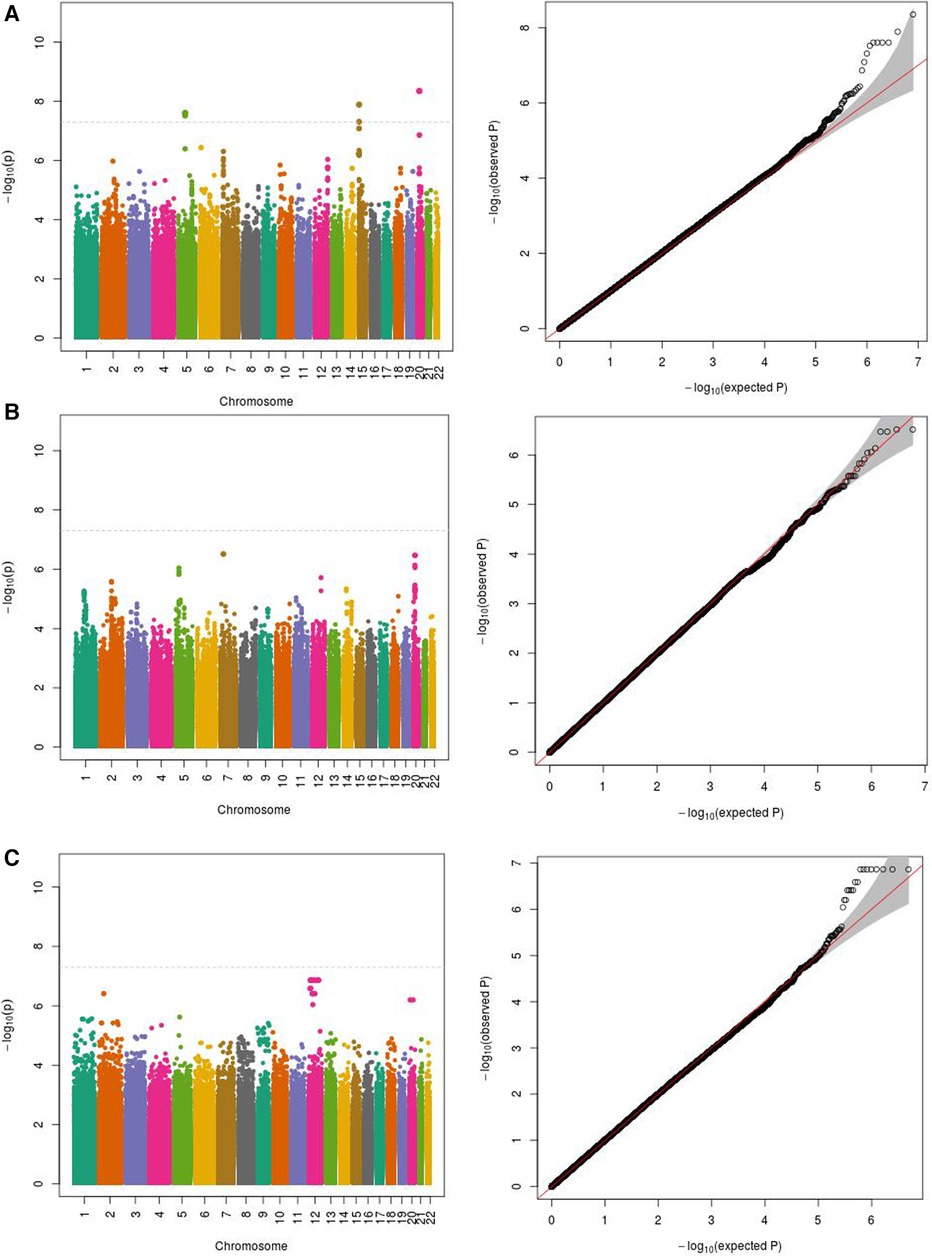
Figure 1 Manhattan and QQ plots for PMP association in (A) African Americans (−500 subjects); (B) European Americans; (C) meta-analysis. (A) PMP as phenotype in African Americans (500 subjects). (B) PMP as phenotype in European Americans (503 subjects). (C) PMP meta-analytic GWAS in African American and European Americans subjects (n = 1,003).
To gain a composite view of all genetic associations and use this to identify key pathways, we took a systems biology approach using FUMA, leveraging the primary GWAS output. Top associated gene-sets using FUMA for the multi-metabolite profile are shown in Table 3. Results derived using FUMA highlighted some additional intriguing gene ontology groups such as endoribonuclease activity, regulation of RNA splicing, Holliday junction resolving, and defense from virus. Finally, mediation models were used to assess the relationship of genetic signals, metabolite traits and clinical outcome (mortality), results of which are summarized in Table 4. Mediation analysis of individual SNP hits did not reveal any metabolites mediating the effect of single SNP genotypes on HF mortality (all p > 0.05).
Discussion
Metabolomic profile is a potentially useful endophenotype for HF progression since plasma metabolite levels are associated with risk of death in HF, and are linked to mitochondrial function and energetics, which represents a key pathophysiologic pathway in HF. Thus, the study of genetic influences on metabolites as endophenotypes may provide a short-cut to understanding the mechanisms involved that contribute to worsening HFrEF. The present study examined this, utilizing a combined approach by predicting mortality using genetic GWAS hits combined with a metabolite profile to identify novel candidate genes for HF (COX1, ARHGEF28, and TEN1) and underscoring key gene ontology groups (33) that likely have impact in HF development and progression (metabolic and apoptotic pathways, among others).
We identified several genome wide significant hits for the PMP, but interestingly, all of them appear in the AA-only analysis. The reason for this is unclear. While the lack of findings across both cohorts could reflect chance findings, it also could be that the greater genetic diversity present in AA patients provides greater power in this moderate sized study. One SNP was near (approximately 3 kb upstream) a known coding gene, Rho Guanine Nucleotide Exchange Factor 28 (ARHGEF28). While this precise gene has not previously been linked to HF, GEFs are involved in processes across a wide range of tissues interacting with integrins and are linked to autophagy and apoptotic pathways; both processes known to be active in the setting of HF as well as being linked to mitochondrial and energetic cellular functions. Indeed, other GEF family members have been linked to cardiac remodeling and cardiac fibrosis in model systems (34, 35).
We identified only one SNP (rs35792152) that was significant for one of the prognostic metabolites (3HBA) and that was also associated with mortality. This SNP lies in or near multiple genes; it is within Telomere Length Regulation Protein gene (TEN1) and TEN1-CDK3 (a read-through long non-coding RNA gene), and near (5 kb upstream) Acyl-CoA Oxidase 1 (ACOX1). TEN1 is a key part of a protein complex involved in telomere maintenance during DNA replication (36). Telomere length and maintenance is linked to aging and senescent cell processes, but also more specific links to energetic function and age-related diseases (like HF) have been widely proposed (37). More obviously, ACOX1 catalyzes the initial and rate-limiting step in peroxisomal beta-oxidation of fatty acids, a process with emerging implications for heart failure (38). There are intriguing links between TEN-CDK3, a lncRNA gene, with dextro-cardia and cardiomyopathy. In considering the possible gene links of this SNP, the fact that it is associated with 3HBA levels and is located near ACOXI could be seen as pointing to energetic mechanisms or substrate utilization. However, as described, the other potential candidates also have plausible biologic mechanisms. Further investigation is needed to clarify possible causation and mechanism.
One possible limitation of the study is that the plasma metabolite profile may not reflect HF disease progression per se. However, this is unlikely given that most patients with HFrEF die from cardiac complications and not another disease. Another possible limitation of the study is that the plasma metabolite profile may reflect HF progression but not myocardial energetics specifically. With regards to alternative approaches to the metabolomic studies themselves, one could consider global/untargeted metabolomics. However, existing literature on metabolomics in cardiovascular disease has focused our attention on several very promising metabolite classes including ACs, AAs and OAs, and the strength of our data associating these metabolites with survival in HF patients suggests we stay focused on fewer, quantifiable analytes. Moreover, a formidable challenge of global metabolomics is identification of unknown (unannotated) metabolites, which is key for elucidating mechanism and developing new intervention strategies. Future studies are needed to look over more metabolomics wide markers, not just targeted assays. Lastly, an additional limitation of the study is the relatively small sample size (N = 1,003).
Moreover, the patient metabolite profile was measured only at one time point i.e., the time of study enrollment. The metabolite profile was not taken in relation to any disease stage, and no direct replication of the profile was performed. We have developed a novel method to link genetic variants with changes in plasma metabolite levels and HF mortality. Several causal relationships were uniquely significant in the AA population, suggesting this technique could be useful in generating prognostic factors in at-risk populations.
Data availability statement
The datasets presented in this study can be found in online repositories. The names of the repository/repositories and accession number(s) can be found below: https://www.ncbi.nlm.nih.gov/gap/, phs001501.v1.p1.
Ethics statement
The studies involving humans were approved by IRB of Henry Ford Health System, 1 Ford Place, Detroit, MI 48202, USA. The studies were conducted in accordance with the local legislation and institutional requirements. The participants provided their written informed consent to participate in this study.
Author contributions
VR: Validation, Visualization, Writing – original draft, Writing – review & editing. RS: Formal Analysis, Visualization, Writing – original draft, Writing – review & editing. HG: Formal Analysis, Investigation, Methodology, Validation, Visualization, Writing – original draft, Writing – review & editing. JL: Funding acquisition, Methodology, Supervision, Validation, Writing – original draft, Writing – review & editing. TB: Writing – original draft, Writing – review & editing. ZM: Writing – original draft, Writing – review & editing. LW: Funding acquisition, Project administration, Resources, Supervision, Writing – original draft, Writing – review & editing. HS: Conceptualization, Data curation, Investigation, Methodology, Project administration, Resources, Supervision, Validation, Writing – original draft, Writing – review & editing, Funding acquisition. SG: Conceptualization, Data curation, Writing – original draft, Writing – review & editing. DL: Conceptualization, Data curation, Funding acquisition, Investigation, Methodology, Project administration, Resources, Supervision, Validation, Visualization, Writing – original draft, Writing – review & editing.
Funding
The author(s) declare financial support was received for the research, authorship, and/or publication of this article.
This study was funded by NIH grant R01HL103871 (DL), R01HL132154 (DL, HS), and K08HL146990 (JL).
Acknowledgments
We thank all the participants of the Henry Ford Genomic Registry for their consent and contribution to our scientific research.
Conflict of interest
DL has received research funding or support from Akros, Astra Zeneca, Eli Lilly, Pfizer, Illumina, Janssen and SomaLogic, and has acted as consultant to ACI Clinical (Abbott Laboratories), AstraZeneca, Cytokinetics, Duke Clinical Research Institute (CONNECT-HF), Illumina, Janssen, Martin Pharmaceuticals, Ortho Clinical Diagnostics, Otsuka, and ARMGO. HS has received research funding from Novartis, and Impulse Dynamics Inc. and is consultant to Novartis, Impulse Dynamics, Vascular Dynamics, and ViCardia.
The remaining authors declare that the research was conducted in the absence of any commercial or financial relationships that could be construed as a potential conflict of interest.
Publisher's note
All claims expressed in this article are solely those of the authors and do not necessarily represent those of their affiliated organizations, or those of the publisher, the editors and the reviewers. Any product that may be evaluated in this article, or claim that may be made by its manufacturer, is not guaranteed or endorsed by the publisher.
Supplementary material
The Supplementary Material for this article can be found online at: https://www.frontiersin.org/articles/10.3389/fcvm.2024.1409340/full#supplementary-material
References
1. Virani SS, Alonso A, Benjamin EJ, Bittencourt MS, Callaway CW, Carson AP, et al. Heart disease and stroke statistics-2020 update: a report from the American heart association. Circulation. (2020) 141:e139–596. doi: 10.1161/CIR.0000000000000757
2. Greene SJ, Khan MS. Quadruple medical therapy for heart failure: medications working together to provide the best care. J Am Coll Cardiol. (2021) 77:1408–11. doi: 10.1016/j.jacc.2021.02.006
3. Bauersachs J. Heart failure drug treatment: the fantastic four. Eur Heart J. (2021) 42:681–3. doi: 10.1093/eurheartj/ehaa1012
4. Jarreta D, Orús J, Barrientos A, Miró O, Roig E, Heras M, et al. Mitochondrial function in heart muscle from patients with idiopathic dilated cardiomyopathy. Cardiovasc Res. (2000) 45:860–5. doi: 10.1016/S0008-6363(99)00388-0
5. Lopez R, Marzban B, Gao X, Lauinger E, Van den Bergh F, Whitesall SE, et al. Impaired myocardial energetics causes mechanical dysfunction in decompensated failing hearts. Function (Oxf). (2020) 1:zqaa018. doi: 10.1093/function/zqaa018
6. Turer AT. Using metabolomics to assess myocardial metabolism and energetics in heart failure. J Mol Cell Cardiol. (2013) 55:12–8. doi: 10.1016/j.yjmcc.2012.08.025
7. Packer M. The neurohormonal hypothesis: a theory to explain the mechanism of disease progression in heart failure. J Am Coll Cardiol. (1992) 20:248–54. doi: 10.1016/0735-1097(92)90167-L
8. Hunter WG, Kelly JP, McGarrah RW 3rd, Khouri MG, Craig D, Haynes C, et al. Metabolomic profiling identifies novel circulating biomarkers of mitochondrial dysfunction differentially elevated in heart failure with preserved versus reduced ejection fraction: evidence for shared metabolic impairments in clinical heart failure. J Am Heart Assoc. (2016) 5(8):e003190. doi: 10.1161/JAHA.115.003190
9. Lopaschuk GD, Karwi QG, Tian R, Wende AR, Abel ED. Cardiac energy metabolism in heart failure. Circ Res. (2021) 128:1487–513. doi: 10.1161/CIRCRESAHA.121.318241
10. Willner S, Gardell S, She R, Li J, Zeld N, Williams KL, et al. Circulating metabolomic profile predicts change in ejection fraction in heart failure patients. J Card Fail. (2023) 29:569. doi: 10.1016/j.cardfail.2022.10.061
11. Tahir UA, Katz DH, Zhao T, Ngo D, Cruz DE, Robbins JM, et al. Metabolomic profiles and heart failure risk in black adults: insights from the Jackson heart study. Circ Heart Fail. (2021) 14:e007275. doi: 10.1161/CIRCHEARTFAILURE.120.007275
12. Henderson AJ. Childhood asthma phenotypes in the twenty-first century. Breathe. (2014) 10:100–8. doi: 10.1183/20734735.014613
13. Ferreira MA, Matheson MC, Tang CS, Granell R, Ang W, Hui J, et al. Genome-wide association analysis identifies 11 risk variants associated with the asthma with hay fever phenotype. J Allergy Clin Immunol. (2014) 133:1564–71. doi: 10.1016/j.jaci.2013.10.030
14. Harold D, Abraham R, Hollingworth P, Sims R, Gerrish A, Hamshere ML, et al. Genome-wide association study identifies variants at CLU and PICALM associated with Alzheimer’s disease. Nat Genet. (2009) 41:1088–93. doi: 10.1038/ng.440
15. van der Ende MY, Said MA, van Veldhuisen DJ, Verweij N, van der Harst P. Genome-wide studies of heart failure and endophenotypes: lessons learned and future directions. Cardiovasc Res. (2018) 114:1209–25. doi: 10.1093/cvr/cvy083
16. Shah SH, Kraus WE, Newgard CB. Metabolomic profiling for the identification of novel biomarkers and mechanisms related to common cardiovascular diseases: form and function. Circulation. (2012) 126:1110–20. doi: 10.1161/CIRCULATIONAHA.111.060368
17. Shah SH, Sun JL, Stevens RD, Bain JR, Muehlbauer MJ, Pieper KS, et al. Baseline metabolomic profiles predict cardiovascular events in patients at risk for coronary artery disease. Am Heart J. (2012) 163:844–50.e1. doi: 10.1016/j.ahj.2012.02.005
18. Salek R, Cheng KK, Griffin J. The study of mammalian metabolism through NMR-based metabolomics. Methods Enzymol. (2011) 500:337–51. doi: 10.1016/B978-0-12-385118-5.00017-7
19. Griffin JL, Atherton H, Shockcor J, Atzori L. Metabolomics as a tool for cardiac research. Nat Rev Cardiol. (2011) 8:630–43. doi: 10.1038/nrcardio.2011.138
20. Dunn WB, Broadhurst DI, Deepak SM, Buch MH, McDowell G, Spasic I, et al. Serum metabolomics reveals many novel metabolic markers of heart failure, including pseudouridine and 2-oxoglutarate. Metabolomics. (2007) 3:413–26. doi: 10.1007/s11306-007-0063-5
21. Ahmad T, Kelly JP, McGarrah RW, Hellkamp AS, Fiuzat M, Testani JM, et al. Prognostic implications of long-chain acylcarnitines in heart failure and reversibility with mechanical circulatory support. J Am Coll Cardiol. (2016) 67:291–9. doi: 10.1016/j.jacc.2015.10.079
22. Lanfear DE, Gibbs JJ, Li J, She R, Petucci C, Culver JA, et al. Targeted metabolomic profiling of plasma and survival in heart failure patients. JACC Heart Fail. (2017) 5:823–32. doi: 10.1016/j.jchf.2017.07.009
23. Weale ME. Quality control for genome-wide association studies. Methods Mol Biol. (2010) 628:341–72. doi: 10.1007/978-1-60327-367-1_19
24. Das S, Forer L, Schönherr S, Sidore C, Locke AE, Kwong A, et al. Next-generation genotype imputation service and methods. Nat Genet. (2016) 48:1284–7. doi: 10.1038/ng.3656
25. Purcell S, Neale B, Todd-Brown K, Thomas L, Ferreira MA, Bender D, et al. PLINK: a tool set for whole-genome association and population-based linkage analyses. Am J Hum Genet. (2007) 81:559–75. doi: 10.1086/519795
26. Conomos MP, Miller MB, Thornton TA. Robust inference of population structure for ancestry prediction and correction of stratification in the presence of relatedness. Genet Epidemiol. (2015) 39:276–93. doi: 10.1002/gepi.21896
27. Willer CJ, Li Y, Abecasis GR. METAL: fast and efficient meta-analysis of genomewide association scans. Bioinformatics. (2010) 26:2190–1. doi: 10.1093/bioinformatics/btq340
28. Consortium EP. An integrated encyclopedia of DNA elements in the human genome. Nature. (2012) 489:57–74. doi: 10.1038/nature11247
29. Consortium GT. The genotype-tissue expression (GTEx) project. Nat Genet. (2013) 45:580–5. doi: 10.1038/ng.2653
30. Li MX, Gui HS, Kwan JS, Sham PC. GATES: a rapid and powerful gene-based association test using extended simes procedure. Am J Hum Genet. (2011) 88:283–93. doi: 10.1016/j.ajhg.2011.01.019
31. Tingley DYT, Hirose K, Keele L, Imai K. Mediation: r package for causal mediation analysis. J Stat Softw. (2014) 59:1–38. doi: 10.18637/jss.v059.i05
32. Mafort Rohen F, Xavier de Avila D, Martins Cabrita Lemos C, Santos R, Ribeiro M, Villacorta H. The MAGGIC risk score in the prediction of death or hospitalization in patients with heart failure: comparison with natriuretic peptides. Rev Port Cardiol. (2022):S0870-2551(22)00363-8. doi: 10.1016/j.repc.2021.07.015
33. Mahajan P, Fiehn O, Barupal D. IDSLGOA: gene ontology analysis for interpreting metabolomic datasets. Sci Rep. (2024) 14(1):1299. doi: 10.1038/s41598-024-51992-x
34. Takefuji M, Kruger M, Sivaraj KK, Kaibuchi K, Offermanns S, Wettschureck N. RhoGEF12 controls cardiac remodeling by integrating G protein- and integrin-dependent signaling cascades. J Exp Med. (2013) 210:665–73. doi: 10.1084/jem.20122126
35. Numaga-Tomita T, Kitajima N, Kuroda T, Nishimura A, Miyano K, Yasuda S, et al. TRPC3-GEF-H1 Axis mediates pressure overload-induced cardiac fibrosis. Sci Rep. (2016) 6:39383. doi: 10.1038/srep39383
36. Price CM, Boltz KA, Chaiken MF, Stewart JA, Beilstein MA, Shippen DE. Evolution of CST function in telomere maintenance. Cell Cycle. (2010) 9:3157–65. doi: 10.4161/cc.9.16.12547
37. Gao X, Yu X, Zhang C, Wang Y, Sun Y, Sun H, et al. Telomeres and mitochondrial metabolism: implications for cellular senescence and age-related diseases. Stem Cell Rev Rep. (2022) 18:2315–27. doi: 10.1007/s12015-022-10370-8
Keywords: plasma metabolites, heart failure, GWAS, QTL, mediation analysis
Citation: Revathi Venkateswaran V, She R, Gui H, Luzum JA, Bryson TD, Malouf ZE, Williams L.K, Sabbah HN, Gardell SJ and Lanfear DE (2024) Genetic drivers of human plasma metabolites that determine mortality in heart failure patients with reduced ejection fraction. Front. Cardiovasc. Med. 11:1409340. doi: 10.3389/fcvm.2024.1409340
Received: 29 March 2024; Accepted: 20 June 2024;
Published: 9 July 2024.
Edited by:
Massimo Iacoviello, University of Foggia, ItalyReviewed by:
Genesio Karere, Wake Forest University, United StatesMichele Correale, Azienda Ospedaliero-Universitaria Ospedali Riuniti di Foggia, Italy
© 2024 Revathi Venkateswaran, She, Gui, Luzum, Bryson, Malouf, Williams, Sabbah, Gardell and Lanfear. This is an open-access article distributed under the terms of the Creative Commons Attribution License (CC BY). The use, distribution or reproduction in other forums is permitted, provided the original author(s) and the copyright owner(s) are credited and that the original publication in this journal is cited, in accordance with accepted academic practice. No use, distribution or reproduction is permitted which does not comply with these terms.
*Correspondence: David E. Lanfear, dlanfea1@hfhs.org