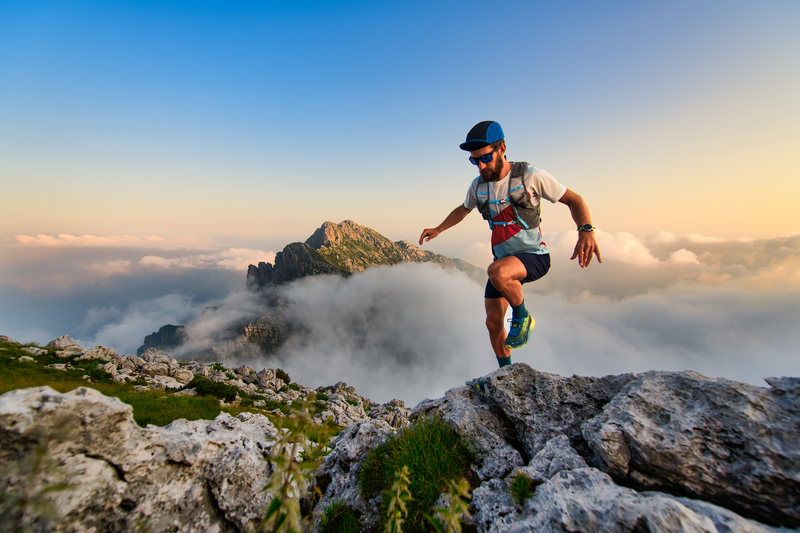
95% of researchers rate our articles as excellent or good
Learn more about the work of our research integrity team to safeguard the quality of each article we publish.
Find out more
ORIGINAL RESEARCH article
Front. Cardiovasc. Med. , 12 September 2024
Sec. Cardiac Rhythmology
Volume 11 - 2024 | https://doi.org/10.3389/fcvm.2024.1401384
Background: Atrial fibrillation (AF) is one of the most prevalent cardiac arrhythmias and has a significant economic and social burden. Whether it is associated with lifestyle behaviors and socioeconomic status is currently poorly understood. This study aimed to explore the relationship among these factors and determine the role of inflammatory cytokines.
Method: We investigated the causal effects of lifestyle behaviors and socioeconomic status on AF using bidirectional two-sample Mendelian randomization (MR). Instrumental variables were obtained from a publicly available genome-wide association study. A two-step MR was conducted to determine the mediating role of 91 inflammatory cytokines. Inverse variance weighted was used as the main method with four supplementary MR methods. To obtain more robust results, several sensitivity analyses were conducted.
Result: The results indicated that seven of the lifestyle behaviors [smoking initiation, vegetable intake, coffee consumption (cups/day), dozing, lifetime smoking index, napping, and alcohol abuse] were potential risk factors for AF. One socioeconomic status, education attainment (years of education), was causally associated with a decreased risk of AF. Moreover, we found that thymic stromal lymphopoietin, CD40l receptor, C-X-C motif chemokine 6, and C-X-C motif chemokine 11 levels mediated the causal effect, at proportions of 13.6%, 4.1%, 4.3%, and 6.9%, respectively.
Conclusion: Our findings provide insight into the relationship between lifestyle behaviors, socioeconomic status, and AF. Inflammatory cytokines are potential mediators of this relationship.
Atrial fibrillation (AF) is a rapid cardiac arrhythmia that is prevalent globally, particularly among the elderly (1, 2). It has been strongly linked to several diseases, including stroke, coronary heart disease (CHD), and heart failure. The disease is a major cause of all-cause mortality (3, 4). The risk of AF recurrence is high even after surgical or pharmacological treatment (5). Currently, there are no effective treatments to prevent AF recurrence (6).
Lifestyle factors and socioeconomic status are modifiable determinants that may influence AF risk and management. Previous research has demonstrated their association with reduced all-cause mortality (7) and other cardiovascular outcomes, including coronary artery disease, heart failure, and sudden cardiac death (8). Although these studies establish associations, they cannot determine causality. Consequently, investigating the underlying causal relationships between these factors and AF is essential for developing effective prevention and management strategies. The mechanisms of AF are not well understood and need to be explored (9). Of note, inflammatory cytokines, such as C-reactive protein (CRP) and interleukins, are known to influence the severity of AF (10, 11). Given that the development of AF is closely associated with changes in inflammatory cytokines (12, 13), it is imperative to explore the mediating role of inflammatory cytokines in the relationship between lifestyle behaviors, socioeconomic status, and AF.
Mendelian randomization (MR) is a powerful tool that utilizes genetic variants allocated randomly at birth to evaluate causal relationships. The findings detected by MR are less prone to reverse causation and confounding biases. The results of MR often mirror those of randomized controlled trials (RCTs) (14, 15). In this study, we aimed to analyze the causal relationship between lifestyle behavior, socioeconomic status, and AF using MR methods. Modifying lifestyle factors and socioeconomic conditions could effectively reduce the incidence of AF, thereby alleviating the associated financial and medical burdens. To elucidate the underlying mechanisms, this study explored the potential mediating role of 91 inflammatory cytokines in the development of AF.
In general, MR analysis should meet three fundamental assumptions: (1) instrumental variables (IVs) should be closely associated with the exposure; (2) the IVs should not be linked to any confounding factors; and (3) the IVs may contribute to the outcome only by influencing the risk factors (Figure 1). The entire MR analysis was performed using the TwoSampleMR package (version 0.5.7) and MR-PRESSO (version 1.0) in R Version (4.2.1) (16).
This study employed a meticulously designed bidirectional MR analysis to investigate the causal relationship between lifestyle factors, socioeconomic status, and AF. We examined the impact of these factors on AF risk and explored potential reverse causality by treating AF as an exposure. To avoid the influence of heterogeneity on the estimated causal effects, random effect inverse variance weighted (RE-IVW) was applied as the primary outcome. In addition, four other analytical methods (MR Egger, weighted median, simple mode, and weighted mode) were adopted to obtain more robust results. Several sensitivity analyses were performed to assess the results and identify potential confounders.
A two-step MR analysis was performed to identify the mediating role of inflammatory cytokines in the relationship between the exposures affecting AF. We first explored the causal effect of exposure on inflammatory factors (β1).
Qualified exposures were defined as risk factors influencing AF without being affected by AF itself, based on bidirectional Mendelian randomization analysis. Subsequently, we examined the influence of the selected mediator on AF (β2). The mediation proportion, representing the indirect effect relative to the total effect, was calculated as described previously (17). Figure 1 outlines the study design.
To ensure data consistency and reduce heterogeneity, our analysis primarily focused on genome-wide association study (GWAS) data from individuals of European ancestry, given their greater availability and quality. We obtained single nucleotide polymorphisms (SNPs) from publicly accessible GWAS studies and extracted summary statistics for 91 circulating inflammatory cytokines from a meta-analysis involving 14,824 participants across 11 European cohorts (18). The summary level data used in this study were obtained from https://www.phpc.cam.ac.uk/ceu/proteins and the GWAS Catalog database (accession numbers GCST90274758 to GCST90274848). The dataset used for AF was from the FinnGen consortium (R9) comprising 50,743 cases and 210,652 controls (19). All the 261,395 participants were of European ancestry. The SNPs associated with smoking initiation and alcohol consumption were derived from a GWAS and Sequencing Consortium of Alcohol and Nicotine (GSCAN) use (20), a study that investigated the effect of alcohol use and smoking initiation from a genetic perspective. Genetic associations for dozing, getting up, insomnia, morningness, napping, sensitivity to environmental stress and adversity (SESA), sleep duration, snoring, and the lifetime smoking index were obtained from the UK Biobank (21, 22). We obtained genetic variants associated with physical activity (PA) from a published meta-analysis leveraging data from the UK Biobank, encompassing up to 91,084 participants (23). SNPs linked to coffee consumption were extracted from a recent GWAS involving a maximum of 91,462 participants (24). Summary statistical data for tea intake, vegetable intake, household income, and the Townsend Deprivation Index were retrieved from the Medical Research Council Integrative Epidemiology Unit GWAS database (https://gwas.mrcieu.ac.uk/). It is important to note that this database primarily includes individuals of European ancestry. Summary statistics for alcohol abuse were obtained from the UK Biobank, encompassing data from multiple ancestry groups, with the majority of European descent (25). Summary level data for education attainment (college completion) and education attainment (years of education) were obtained from a GWAS comprising 470,941 participants of European ancestry (26). The detailed information and definition of the above traits are presented in Supplementary Table S1.
Initially, we extracted SNPs robustly associated with exposure at the genome-wide significance threshold (p < 5 × 10−8). However, when the number of SNPs was limited, a more lenient threshold for the genome-wide significance level was set at p < 5 × 10−6, which had been used in previous studies (27). Next, SNP clumping (kb = 10,000, r2 < 0.001) was conducted using the European sample to avoid the linkage disequilibrium (LD). We ensured consistent effect allele orientation across all datasets and excluded palindromic sequences with ambiguous allele frequencies. We identified and excluded SNPs potentially associated with both the outcome (atrial fibrillation) and outcome-related confounders using the PhenoScanner database tool (http://www.phenoscanner.medschl.cam.ac.uk/). Specific confounders excluded included body mass index, age, smoking status, chronic inflammation, hypertension, coronary artery disease, chronic renal failure, diabetes, and the use of medications such as anticoagulants, antiplatelet agents, and lipid-lowering drugs. To investigate the statistical strength of the variables, we calculated the F-statistic using the following formula: (28). SNPs with F < 10 were filtered to select instruments variables (IVs) with strong power. Detailed information on all the included IVs are presented in Supplementary Table S2–S4.
To assess the potential causal relationships between lifestyle behaviors, socioeconomic status, inflammatory cytokines, and AF, we employed a RE-IVW approach. This method mimics the RCT design by leveraging the random assignment principle, increasing statistical power and reliability (29). Nonetheless, the RE-IVW method remains susceptible to bias due to pleiotropy. Four other MR methods (MR Egger, weighted median, simple mode, and weighted mode) were employed to further assess the stability of the results. A statistical significance threshold of p < 0.05 was selected to determine the causal relationship.
Several sensitivity analyses were conducted to identify cases of pleiotropy. Cochrane's Q statistic and funnel plots were used to measure heterogeneity among the estimates from each SNP by the IVW and MR Egger methods. Heterogeneity among genetic instruments was assessed using Cochran's Q statistic, with a p-value < 0.05 indicating significant heterogeneity. To detect horizontal pleiotropy, we employed the MR-PRESSO global test (30). Directional pleiotropy was evaluated using the MR Egger test, with a p-value < 0.05 indicating its presence. Sensitivity analysis using leave-one-out analysis assessed the influence of individual SNPs on the overall results by sequentially excluding each SNP (31). The forest plots and scatter plots were constructed to display the results of the five MR methods.
In the forward MR analysis, smoking initiation [odds ratio (OR): 1.187, 95% confidence interval (CI): 1.088–1.294, p < 0.001], vegetable intake (OR: 2.425, 95% CI: 1.005–5.848, p = 0.048), coffee consumption (cups/day) (OR: 1.117, 95% CI: 1.020–1.224, p = 0.017), coffee consumption (cases vs. controls) (OR: 1.078, 95% CI: 1.016–1.143, p = 0.011), lifetime smoking index (OR: 1.819, 95% CI: 1.304–2.536, p < 0.001), napping (OR: 1.256, 95% CI: 1.100–1.433, p < 0.001), alcohol abuse (OR: 1.703, 95% CI: 1.178–2.462, p = 0.004), education attainment (college completion) (OR: 0.501, 95% CI: 0.383–0.655, p < 0.001), and education attainment (years of education) (OR: 0.942, 95% CI: 0.917–0.969, p < 0.001) were associated with the risk of AF, as determined using the IVW method. No statistically significant correlations were found between alcohol consumption, physical activity, tea intake, sleep patterns (dozing, getting up, insomnia, morningness, sleep duration, and snoring), socioeconomic status (household income and the Townsend deprivation index), and AF (Table 1, Figure 2).
Table 1. Forward MR reveals causal links between lifestyle behaviors, socioeconomic status, and AF incidence by the IVW method.
Figure 2. Evaluation of the forward and reverse relationship between lifestyle behaviors, socioeconomic status and AF by using the IVW method: (A) Forward MR and (B) Reverse MR. AF, atrial fibrillation; IVW, inverse variance weighted; MR, Mendelian randomization; PA, physical activity; SESA, sensitivity to environmental stress and adversity; OR, odds ratio.
Surprisingly, the results obtained by the MR Egger and IVW methods were not aligned perfectly in terms of direction for vegetable intake and educational attainment (college completion). The results of the rest of the tests were stable (Supplementary Table S5). All IVs had an F value exceeding 10 (Supplementary Table S2).
To account for potential heterogeneity, random effects models were used. MR Egger regression identified horizontal pleiotropy in tea intake and educational attainment (college completion) (Egger intercept p = 0.019 and 0.003, respectively), rendering these results unreliable. The remaining findings were robust (Egger intercept p > 0.05). Additional sensitivity analyses using MR-PRESSO corroborated these results, as detailed in Supplementary Table S6.
The results of the reverse MR analysis showing the association of AF on lifestyle behaviors and socioeconomic status are presented in Table 2, Supplementary Table S7, and Figure 2. All the included SNPs met the criteria of an F value greater than 10, calculated using the formula listed above (Supplementary Table S3). Our findings indicated a causal relationship between increased vegetable intake and an increased risk of AF (OR: 1.009, 95% CI: 1.002–1.015, p = 0.006). In contrast, no causal association was observed between AF and other lifestyle factors or socioeconomic status (Table 2, Figure 2). Given the discrepancy between the IVW and MR Egger methods for vegetable intake and PA, sensitivity analyses were conducted. Although the overall results were consistent across multiple MR methods (Supplementary Table S7), the MR Egger intercept p-values for PA and vegetable intake (0.009 and 0.030, respectively) suggested potential horizontal pleiotropy, necessitating cautious interpretation of these findings.
Table 2. Reverse MR reveals causal links between AF and changes in lifestyle behaviors and socioeconomic status.
Forward MR analysis identified smoking initiation, coffee consumption (both cups per day and cases vs. controls), lifetime smoking, napping, alcohol abuse, and educational attainment as causal factors influencing AF risk. We selected SNPs associated with these traits to examine their impact on 91 inflammatory cytokines (Supplementary Table S2). The primary and sensitivity analysis results are presented in Supplementary Table S9. A rigorous selection process narrowed down 91 inflammatory cytokines to four potential mediators influencing the relationship between lifestyle factors, socioeconomic status, and AF: CXCL11, thymic stromal lymphopoietin, CD40l receptor, and CXCL6. Detailed information about the selected genetic instrumental variables, all exhibiting F-statistics greater than 10, is presented in Supplementary Table S4. There was a significant association between smoke and low CXCL11 levels (OR: 0.863, 95% CI: 0.775–0.962, p = 0.008). Elevated CXCL11 levels were associated with an increased risk of AF (OR: 1.068, 95% CI: 1.001–1.139, p = 0.046), mediating 6.9% of the overall effect. Conversely, coffee consumption was linked to decreased thymic stromal lymphopoietin levels (OR: 0.892, 95% CI: 0.824–0.966, p = 0.005) but paradoxically increased AF risk (OR: 1.094, 95% CI: 1.021–1.172, p = 0.011), with a mediation proportion of 13.6%. Among the 91 inflammatory cytokines examined, only CD40l receptor and CXCL6 levels were identified as potential mediators between educational attainment and AF risk. For CD40l receptor and CXCL6 levels, education attainment (years of education) was associated with a higher level of inflammatory cytokines (OR: 0.965, 95% CI: 0.943–0.988, p = 0.003; and OR: 0.954, 95% CI: 0.931–0.977, p = 0.011). Through the IVW method, CD40l receptor levels (OR: 0.935, 95% CI: 0.901–0.970, p < 0.001) and CXCL6 (OR: 1.055, 95% CI: 1.001–1.113, p = 0.048) exhibited opposite effects on AF. Moreover, the proportion mediated by these two was 4.1% and 4.3%, respectively (Figures 3, 4).
Figure 3. The mediators identified through two-step MR and their relationships with lifestyle behaviors, socioeconomic status, and AF. AF, atrial fibrillation; MR, Mendelian randomization.
Figure 4. The two-step MR estimates for mediators between lifestyle behaviors, socioeconomic status, and AF. MR, Mendelian randomization; AF, atrial fibrillation.
Sensitivity analyses consistently supported the primary findings (Supplementary Table S10). Leave-one-out analysis confirmed the robustness of the causal effects by excluding potentially influential instrumental variables. Symmetrical funnel plots, along with results from additional analyses (Supplementary Figures S1–S4), further corroborated the reliability of our findings.
This study aimed to investigate the bidirectional relationship between lifestyle behaviors, socioeconomic status, and AF. In addition, the mediating role of inflammatory cytokines was investigated. The results indicated that smoking initiation (age at smoking initiation), coffee consumption (cups/day), coffee consumption (cases vs. controls), dozing, lifetime smoking index, napping, and alcohol abuse are associated with an elevated risk of AF. In contrast, educational attainment (years of education) was negatively associated with the development of AF. Two-step MR analysis revealed that the causal relationship of educational attainment (years of education) with the risk of AF was mediated by CD40l receptor levels and CXCL6 (mediation proportions of 4.1% and 4.3%, respectively). Coffee consumption (cases vs. controls) had a casual effect on AF, and this effect was mediated by the level of thymic stromal lymphopoietin (a mediation proportion of 13.6%). When smoke initiation was treated as an exposure, it only affected the incidence of AF by affecting CXCL11 levels, which mediated 9.7% of the causal relationship. These novel findings underscore the significance of targeting the modifiable risk factors, such as lifestyle behaviors and socioeconomic status, as a strategy for preventing the occurrence of AF.
Currently, AF is the most prevalent rapid cardiac arrhythmia (32). Compared with patients without AF, those with AF experience a higher economic burden and poorer quality of life (33, 34). The high recurrence rate of AF underscores the importance of identifying modifiable risk factors. Although traditional observational studies cannot definitively establish causality, MR offers a valuable approach to assess potential causal relationships.
Previous studies have demonstrated that smoke is one of the modifiable risk factors of AF, but evidence for this conclusion is limited (35–37). Studies have indicated that the incidence of AF in active and former smokers is higher than that in patients who never smoked (HR: 1.71, 95% CI: 1.14–2.56; and HR: 1.16, 95% CI: 1.00–1.30, respectively) (38). A recent investigation observed that a higher exposure to secondhand smoking increases the risk of AF compared with non-smokers, with an OR of 3.81 (95% CI 2.02–7.18) (39). Our findings align with previous research regarding the impact of smoking initiation and cumulative smoking exposure on AF. Our results did not support the suggestion by some studies that there was a potential 11% increased risk of venous thromboembolism (VTE) with one to four cups of coffee daily (40). The findings of our study are consistent with those reported by others that coffee consumption (cups/day) and coffee consumption (cases vs. controls) are risk factors of AF. Therefore, we postulate that coffee consumption increases the prevalence of VTE, which in turn elevates the risk of AF. Although drinking at a low concentration is considered to be beneficial for human health, especially in terms of cardiovascular health (41), several other investigations have concluded that alcohol consumption at a high concentration may contribute to the risk of AF (42). Potential mechanisms linking alcohol abuse to AF include an impaired vagal tone, acetaldehyde toxicity, free radical production, and hyperadrenergic states (42). Our findings support alcohol abuse as a risk factor for AF. Further research is needed to explore the association between daytime napping and AF. It has been shown that sleep duration may be an independent risk factor for AF (43). Kanagasabai and Ardern demonstrated that individuals who take midday naps are more likely to develop AF (44). This is consistent with our findings. Specifically, the present results indicated that those who take midday naps are more likely to experience sleeplessness at night. Inadequate sleep will, in turn, trigger inflammation and oxidative stress, potentially contributing to the onset of AF (44). Although previous observational studies have confirmed the relationship between education and health status, particularly in the context of cardiovascular diseases (CVDs), whether there is causality remains to be determined (45, 46). The protective association between higher education and health outcomes may be partially attributed to healthier lifestyle behaviors, such as reduced smoking and increased physical activity (47, 48). In addition, individuals with higher education levels tend to have lower BMIs (49), further contributing to the reduced CVD risk.
There was an accumulation of evidence showing that inflammation has a connection to the occurrence of AF (50). In addition, low-grade systemic inflammation was connected to inflammation in the atrial tissue. For example, Liuba et al. reported that patients with permanent AF had elevated IL-8 levels in the systemic circulation, right atrium, and coronary sinus, and the correlation was high among them (51). The increase in inflammatory cytokines in the circulation causes atrial structural remodeling through collagen turnover (52). Similar to a previous study, TNF-α injection into mice activated myofibroblasts by increasing the secretion of matrix metalloproteinases (MMPs) (53).
The pathophysiology of AF is complex. Previous studies have implicated subclinical inflammation and chronic oxidative stress as key contributors to AF development (54), findings supported by research on CRP (55). CXCL11 and CXCL6, two chemokines involved in inflammation and immune responses, are likely to play pivotal roles in this process. In our study, these two chemokines were found to be associated with the occurrence of AF and their influence was subject to several modifiable risk factors. Similarly, a prospective observational cohort study reported that the C-X-C motif chemokine 12 (CXCL12) level was higher in the AF group than in the control group [3.91 (2.80–10.49) vs. 2.32 (1.57–4.48)] (56). Thymic stromal lymphopoietin, on the other hand, is a cytokine that mainly regulates allergic and inflammatory responses. It is produced by various cell types, including epithelial cells. Although some scholars have demonstrated the association between thymic stromal lymphopoietin and atrial fibrosis, others have shown that thymic stromal lymphopoietin upregulates the expression of CCL2 in primary human lung fibroblasts (57). CD40l, a membrane protein, is an important signaling molecule involved in the activation of T cells. The CD40–CD40l interaction facilitates intercellular communication within the immune system. Soluble CD40l (sCD40l), a cleaved form of CD40l, has been linked to atrial structural alterations in AF patients (58). Given the established role of inflammatory factors in atrial fibrosis, we hypothesize that they may mediate the influence of lifestyle factors and socioeconomic status on AF development. However, further research is required to validate this proposed mechanism.
Despite the strengths of our findings, this study has several limitations that should be acknowledged. First, we verified the effects of lifestyle behaviors and socioeconomic status on AF. Although we included potential mediators to explain the relationship, they only account for part of the relationship, and a greater fraction remains unexplained. Therefore, further studies are advocated to investigate the mechanisms of AF. Second, we did not differentiate between paroxysmal AF, persistent AF, long-standing persistent AF, and permanent AF in GWASs related to AF, which make it difficult to perform a subgroup analysis that might be meaningful. Third, the limited availability of GWAS data on atrial inflammation hindered a comprehensive exploration of the underlying pathophysiological mechanisms. Future studies should address this knowledge gap. Fourth, a potential overlap between exposure and outcome populations may have influenced our findings, necessitating more detailed participant characterization in subsequent research. Finally, the predominantly European focus of the included GWASs limits the generalizability of our results to diverse ethnic populations.
Our findings strongly suggest that lifestyle factors and socioeconomic status are associated with an increased risk of AF. To effectively reduce AF incidence, public health interventions should target these modifiable determinants. The underlying mechanisms may involve inflammatory cytokine pathways, but further investigations are advocated. Large-scale multi-ethnic randomized controlled trials are needed to corroborate our findings and elucidate the causal relationships.
The original contributions presented in the study are included in the article/Supplementary Material, further inquiries can be directed to the corresponding author.
YL: Conceptualization, Formal Analysis, Methodology, Writing – original draft. MH: Data curation, Formal Analysis, Investigation, Software, Writing – original draft. YS: Data curation, Resources, Software, Visualization, Writing – original draft. WD: Funding acquisition, Methodology, Writing – review & editing.
The authors declare that no financial support was received for the research, authorship, and/or publication of this article.
We want to acknowledge all the authors of the included studies for their excellent work. In addition, we want to acknowledge the participants for their dedication.
The authors declare that the research was conducted in the absence of any commercial or financial relationships that could be construed as a potential conflict of interest.
All claims expressed in this article are solely those of the authors and do not necessarily represent those of their affiliated organizations, or those of the publisher, the editors and the reviewers. Any product that may be evaluated in this article, or claim that may be made by its manufacturer, is not guaranteed or endorsed by the publisher.
The Supplementary Material for this article can be found online at: https://www.frontiersin.org/articles/10.3389/fcvm.2024.1401384/full#supplementary-material
1. Kornej J, Börschel CS, Benjamin EJ, Schnabel RB. Epidemiology of atrial fibrillation in the 21st century: novel methods and new insights. Circ Res. (2020) 127:4–20. doi: 10.1161/circresaha.120.316340
2. Teppo K, Airaksinen KEJ, Jaakkola J, Halminen O, Linna M, Haukka J, et al. Trends in treatment and outcomes of atrial fibrillation during 2007–17 in Finland. Eur Heart J Qual Care Clin Outcomes. (2023) 9:673–9. doi: 10.1093/ehjqcco/qcac086
3. Ruddox V, Sandven I, Munkhaugen J, Skattebu J, Edvardsen T, Otterstad JE. Atrial fibrillation and the risk for myocardial infarction, all-cause mortality and heart failure: a systematic review and meta-analysis. Eur J Prev Cardiol. (2017) 24:1555–66. doi: 10.1177/2047487317715769
4. Chyou J, Barkoudah E, Dukes J, Goldstein L, Joglar J, Lee A, et al. Atrial fibrillation occurring during acute hospitalization: a scientific statement from the American Heart Association. Circulation. (2023) 147:e676–69. doi: 10.1161/cir.0000000000001133
5. Lau DH, Nattel S, Kalman JM, Sanders P. Modifiable risk factors and atrial fibrillation. Circulation. (2017) 136:583–96. doi: 10.1161/circulationaha.116.023163
6. Link M, Haïssaguerre M, Natale AJC. Ablation of atrial fibrillation: patient selection, periprocedural anticoagulation, techniques, and preventive measures after ablation. Circulation. (2016) 134:339–52. doi: 10.1161/circulationaha.116.021727
7. Stringhini S, Carmeli C, Jokela M, Avendaño M, Muennig P, Guida F, et al. Socioeconomic status and the 25 × 25 risk factors as determinants of premature mortality: a multicohort study and meta-analysis of 1·7 million men and women. Lancet. (2017) 389:1229–37. doi: 10.1016/s0140-6736(16)32380-7
8. So-Ryoung L, Eue-Keun C, Sang-Hyeon P, Seung-Woo L, Kyung-Do H, Seil O, et al. Clustering of unhealthy lifestyle and the risk of adverse events in patients with atrial fibrillation. Front Cardiovasc Med. (2022) 9:885016. doi: 10.3389/fcvm.2022.885016
9. Pathak R, Middeldorp M, Meredith M, Mehta A, Mahajan R, Wong C, et al. Long-term effect of goal-directed weight management in an atrial fibrillation cohort: a long-term follow-up study (LEGACY). Int J Stroke. (2015) 65:2159–69. doi: 10.1016/j.jacc.2015.03.002
10. Chung M, Martin D, Sprecher D, Wazni O, Kanderian A, Carnes C, et al. C-reactive protein elevation in patients with atrial arrhythmias: inflammatory mechanisms and persistence of atrial fibrillation. Circulation. (2001) 104:2886–91. doi: 10.1161/hc4901.101760
11. Marott S, Nordestgaard B, Zacho J, Friberg J, Jensen G, Tybjaerg-Hansen A, et al. Does elevated C-reactive protein increase atrial fibrillation risk? A Mendelian randomization of 47,000 individuals from the general population. J Am Coll Cardiol. (2010) 56:789–95. doi: 10.1016/j.jacc.2010.02.066
12. Zhao M, Song L, Zhao Q, Chen Y, Li B, Xie Z, et al. Elevated levels of body mass index and waist circumference, but not high variability, are associated with an increased risk of atrial fibrillation. BMC Med. (2022) 20:215. doi: 10.1186/s12916-022-02413-1
13. Virani SS, Alonso A, Benjamin EJ, Bittencourt MS, Callaway CW, Carson AP, et al. Heart disease and stroke statistics-2020 update: a report from the American Heart Association. Circulation. (2020) 141:e139–596. doi: 10.1161/cir.0000000000000757
14. Emdin CA, Khera AV, Kathiresan S. Mendelian randomization. JAMA. (2017) 318:1925–6. doi: 10.1001/jama.2017.17219
15. Lawlor DA, Harbord RM, Sterne JA, Timpson N, Davey Smith G. Mendelian randomization: using genes as instruments for making causal inferences in epidemiology. Stat Med. (2008) 27:1133–63. doi: 10.1002/sim.3034
16. Yavorska O, Burgess S. Mendelian randomization: an R package for performing Mendelian randomization analyses using summarized data. Int J Epidemiol. (2017) 46:1734–9. doi: 10.1093/ije/dyx034
17. Carter AR, Sanderson E, Hammerton G, Richmond RC, Davey Smith G, Heron J, et al. Mendelian randomisation for mediation analysis: current methods and challenges for implementation. Eur J Epidemiol. (2021) 36:465–78. doi: 10.1007/s10654-021-00757-1
18. Zhao JH, Stacey D, Eriksson N, Macdonald-Dunlop E, Hedman ÅK, Kalnapenkis A, et al. Genetics of circulating inflammatory proteins identifies drivers of immune-mediated disease risk and therapeutic targets. Nat Immunol. (2023) 24:1540–51. doi: 10.1038/s41590-023-01588-w
19. Kurki M, Karjalainen J, Palta P, Sipilä T, Kristiansson K, Donner K, et al. Finngen provides genetic insights from a well-phenotyped isolated population. Nature. (2023) 613:508–18. doi: 10.1038/s41586-022-05473-8
20. Liu M, Jiang Y, Wedow R, Li Y, Brazel D, Chen F, et al. Association studies of up to 1.2 million individuals yield new insights into the genetic etiology of tobacco and alcohol use. Nat Genet. (2019) 51:237–44. doi: 10.1038/s41588-018-0307-5
21. Sudlow C, Gallacher J, Allen N, Beral V, Burton P, Danesh J, et al. UK biobank: an open access resource for identifying the causes of a wide range of complex diseases of middle and old age. PLoS Med. (2015) 12:e1001779. doi: 10.1371/journal.pmed.1001779
22. Wootton R, Richmond R, Stuijfzand B, Lawn R, Sallis H, Taylor G, et al. Evidence for causal effects of lifetime smoking on risk for depression and schizophrenia: a Mendelian randomisation study. Psychol Med. (2020) 50:2435–43. doi: 10.1017/s0033291719002678
23. Klimentidis YC, Raichlen DA, Bea J, Garcia DO, Wineinger NE, Mandarino LJ, et al. Genome-wide association study of habitual physical activity in over 377,000 UK biobank participants identifies multiple variants including CADM2 and APOE. Int J Obes (Lond). (2018) 42:1161–76. doi: 10.1038/s41366-018-0120-3
24. Zhong VW, Kuang A, Danning RD, Kraft P, van Dam RM, Chasman DI, et al. A genome-wide association study of bitter and sweet beverage consumption. Hum Mol Genet. (2019) 28:2449–57. doi: 10.1093/hmg/ddz061
25. Thijssen AB, Spit for Science Working Group, Dick DM, Posthuma D, Savage JE. Investigating genetically stratified subgroups to better understand the etiology of alcohol misuse. Mol Psychiatry. (2023) 28:4225–33. doi: 10.1038/s41380-023-02174-0
26. Loh P, Kichaev G, Gazal S, Schoech AP, Price AL. Mixed-model association for biobank-scale datasets. Nat Genet. (2018) 50:906–8. doi: 10.1038/s41588-018-0144-6
27. Xue M, Yao W, Xin C, Jun G. The levels of circulating cytokines and risk of neuromyelitis optica spectrum disorder: a Mendelian randomization study. Front Immunol. (2024) 15:1418309. doi: 10.3389/fimmu.2024.1418309
28. Palmer TM, Lawlor DA, Harbord RM, Sheehan NA, Tobias JH, Timpson NJ, et al. Using multiple genetic variants as instrumental variables for modifiable risk factors. Stat Methods Med Res. (2012) 21:223–42. doi: 10.1177/0962280210394459
29. Pierce BL, Burgess S. Efficient design for Mendelian randomization studies: subsample and 2-sample instrumental variable estimators. Am J Epidemiol. (2013) 178:1177–84. doi: 10.1093/aje/kwt084
30. Verbanck M, Chen CY, Neale B, Do R. Publisher correction: detection of widespread horizontal pleiotropy in causal relationships inferred from Mendelian randomization between complex traits and diseases. Nat Genet. (2018) 50:1196. doi: 10.1038/s41588-018-0164-2
31. Martin FZ, Fraser A, Zuccolo L. Alcohol intake and hypertensive disorders of pregnancy: a negative control analysis in the ALSPAC cohort. J Am Heart Assoc. (2022) 11:e025102. doi: 10.1161/jaha.121.025102
32. GBD 2015 Disease and Injury Incidence and Prevalence Collaborators. Global, regional, and national incidence, prevalence, and years lived with disability for 310 diseases and injuries, 1990–2015: a systematic analysis for the global burden of disease study 2015. Lancet. (2016) 388:1545–602. doi: 10.1016/s0140-6736(16)31678-6
33. Aune D, Schlesinger S, Norat T, Riboli E. Tobacco smoking and the risk of atrial fibrillation: a systematic review and meta-analysis of prospective studies. Eur J Prev Cardiol. (2018) 25:1437–51. doi: 10.1177/2047487318780435
34. Ye X, Li L, Gao Y, Zhou S, Yang Y, Chen S. Dose-response relations between second-hand smoke exposure and depressive symptoms among middle-aged women. Psychiatry Res. (2015) 229:533–8. doi: 10.1016/j.psychres.2015.06.008
35. Chamberlain A, Alonso A, Gersh B, Manemann S, Killian J, Weston S, et al. Multimorbidity and the risk of hospitalization and death in atrial fibrillation: a population-based study. Am Heart J. (2017) 185:74–84. doi: 10.1016/j.ahj.2016.11.008
36. Zhu W, Yuan P, Shen Y, Wan R, Hong K. Association of smoking with the risk of incident atrial fibrillation: a meta-analysis of prospective studies. Int J Cardiol. (2016) 218:259–66. doi: 10.1016/j.ijcard.2016.05.013
37. Chamberlain A. Secondhand smoke and atrial fibrillation: importance of managing modifiable risk factors. J Am Coll Cardiol. (2019) 74:1665–6. doi: 10.1016/j.jacc.2019.08.015
38. Lin G, Lloyd-Jones D, Colangelo L, Szklo M, Heckbert S, Chen L, et al. Secondhand tobacco smoke exposure, urine cotinine, and risk of incident atrial fibrillation: the multi-ethnic study of atherosclerosis. Prog Cardiovasc Dis. (2022) 74:38–44. doi: 10.1016/j.pcad.2022.10.006
39. Regev-Avraham Z, Rosenfeld I, Sharabi-Nov A, Halabi M. Is second hand smoking associated with atrial fibrillation risk among women in Israel? A case-control study. Int J Cardiol. (2020) 304:56–60. doi: 10.1016/j.ijcard.2020.01.071
40. Lippi G, Mattiuzzi C, Franchini M. Venous thromboembolism and coffee: critical review and meta-analysis. Ann Transl Med. (2015) 3:152. doi: 10.3978/j.issn.2305-5839.2015.06.14
41. O’Keefe E, DiNicolantonio J, O’Keefe J, Lavie CJ. Alcohol and CV health: Jekyll and Hyde J-curves. Prog Cardiovasc Dis. (2018) 61:68–75. doi: 10.1016/j.pcad.2018.02.001
42. Qiao Y, Shi R, Hou B, Wu L, Zheng L, Ding L, et al. Impact of alcohol consumption on substrate remodeling and ablation outcome of paroxysmal atrial fibrillation. J Am Heart Assoc. (2015) 4:e002349. doi: 10.1161/jaha.115.002349
43. Khawaja O, Sarwar A, Albert C, Gaziano J, Djoussé L. Sleep duration and risk of atrial fibrillation (from the physicians’ health study). Am J Cardiol. (2013) 111:547–51. doi: 10.1016/j.amjcard.2012.10.038
44. Kanagasabai T, Ardern CI. Contribution of inflammation, oxidative stress, and antioxidants to the relationship between sleep duration and cardiometabolic health. Sleep. (2015) 38:1905–12. doi: 10.5665/sleep.5238
45. Veronesi G, Ferrario MM, Kuulasmaa K, Bobak M, Chambless LE, Salomaa V, et al. Educational class inequalities in the incidence of coronary heart disease in Europe. Heart. (2016) 102:958–65. doi: 10.1136/heartjnl-2015-308909
46. Mina T, Yew YW, Ng HK, Sadhu N, Wansaicheong G, Dalan R, et al. Adiposity impacts cognitive function in Asian populations: an epidemiological and Mendelian randomization study. Lancet Reg Health West Pac. (2023) 33:100710. doi: 10.1016/j.lanwpc.2023.100710
47. Liberatos P, Link BG, Kelsey JL. The measurement of social class in epidemiology. Epidemiol Rev. (1988) 10:87–121. doi: 10.1093/oxfordjournals.epirev.a036030
48. Gage SH, Bowden J, Davey Smith G, Munafò MR. Investigating causality in associations between education and smoking: a two-sample Mendelian randomization study. Int J Epidemiol. (2018) 47:1131–40. doi: 10.1093/ije/dyy131
49. Böckerman P, Viinikainen J, Pulkki-Råback L, Hakulinen C, Pitkänen N, Lehtimäki T, et al. Does higher education protect against obesity? Evidence using Mendelian randomization. Prev Med. (2017) 101:195–8. doi: 10.1016/j.ypmed.2017.06.015
50. Guo Y, Lip G, Apostolakis S. Inflammation in atrial fibrillation. Am Coll Cardiol. (2012) 60:2263–70. doi: 10.1016/j.jacc.2012.04.063
51. Ioan L, Henrik A, Lena J, Anders E, Anders J, Kåge S, et al. Source of inflammatory markers in patients with atrial fibrillation. Europace. (2008) 10:848–53. doi: 10.1093/europace/eun111
52. Dragsbæk K, Neergaard JS, Hansen HB, Byrjalsen I, Alexandersen P, Kehlet SN, et al. Matrix metalloproteinase mediated type I collagen degradation—an independent risk factor for mortality in women. EBioMedicine. (2015) 2:723–9. doi: 10.1016/j.ebiom.2015.04.017
53. Liew R, Khairunnisa K, Gu Y, Tee N, Yin N, Naylynn T, et al. Role of tumor necrosis factor-α in the pathogenesis of atrial fibrosis and development of an arrhythmogenic substrate. Circ J. (2013) 77:1171–9. doi: 10.1253/circj.cj-12-1155
54. Xu S, Li X, Zhang S, Qi C, Zhang Z, Ma R, et al. Oxidative stress gene expression, DNA methylation, and gut microbiota interaction trigger Crohn’s disease: a multi-omics Mendelian randomization study. BMC Med. (2023) 21:179. doi: 10.1186/s12916-023-02878-8
55. Sinner MF, Stepas KA, Moser CB, Krijthe BP, Aspelund T, Sotoodehnia N, et al. B-type natriuretic peptide and C-reactive protein in the prediction of atrial fibrillation risk: the CHARGE-AF consortium of community-based cohort studies. Europace. (2014) 16:1426–33. doi: 10.1093/europace/euu175
56. Meihui T, Haiyan S, Hao W, Xiao M, Meng G, Qing C, et al. Pilot study of peripheral blood chemokines as biomarkers for atrial fibrillation-related thromboembolism and bleeding in elderly patients. Front Public Health. (2022) 10:844087. doi: 10.3389/fpubh.2022.844087
57. Xuefeng X, Huaping D, Zhang J. The potential role of interleukin (IL)-25/IL-33/thymic stromal lymphopoietin (TSLP) on the pathogenesis of idiopathic pulmonary fibrosis. Clin Respir J. (2022) 16:696–707. doi: 10.1111/crj.13541
Keywords: atrial fibrillation, Mendelian randomization, inflammatory cytokines, lifestyle, socioeconomic
Citation: Liu Y, Huang M, Sun Y and Dai W (2024) Exploring the effect of lifestyle behaviors and socioeconomic status on atrial fibrillation: the mediating role of 91 inflammatory cytokines. Front. Cardiovasc. Med. 11:1401384. doi: 10.3389/fcvm.2024.1401384
Received: 8 April 2024; Accepted: 26 August 2024;
Published: 12 September 2024.
Edited by:
Faisal Syed, University of North Carolina at Chapel Hill, United StatesReviewed by:
Li-Da Wu, Nanjing Medical University, ChinaCopyright: © 2024 Liu, Huang, Sun and Dai. This is an open-access article distributed under the terms of the Creative Commons Attribution License (CC BY). The use, distribution or reproduction in other forums is permitted, provided the original author(s) and the copyright owner(s) are credited and that the original publication in this journal is cited, in accordance with accepted academic practice. No use, distribution or reproduction is permitted which does not comply with these terms.
*Correspondence: Weiran Dai, ZGFpd2VpcmFuQGhvc3BpdGFsLmNxbXUuZWR1LmNu
Disclaimer: All claims expressed in this article are solely those of the authors and do not necessarily represent those of their affiliated organizations, or those of the publisher, the editors and the reviewers. Any product that may be evaluated in this article or claim that may be made by its manufacturer is not guaranteed or endorsed by the publisher.
Research integrity at Frontiers
Learn more about the work of our research integrity team to safeguard the quality of each article we publish.