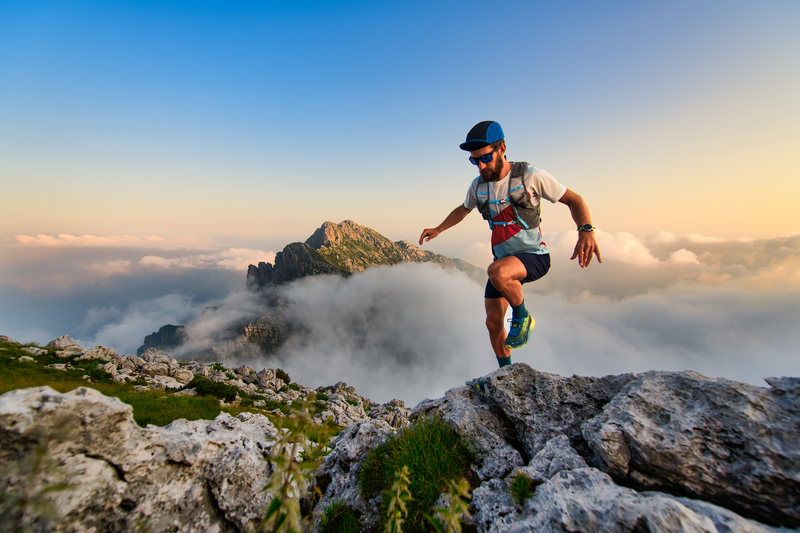
95% of researchers rate our articles as excellent or good
Learn more about the work of our research integrity team to safeguard the quality of each article we publish.
Find out more
ORIGINAL RESEARCH article
Front. Cardiovasc. Med. , 22 August 2024
Sec. Cardiovascular Epidemiology and Prevention
Volume 11 - 2024 | https://doi.org/10.3389/fcvm.2024.1361088
Background: Systemic immune-inflammation index (SII) and systemic inflammation response index (SIRI) are comprehensive markers of inflammatory status. However, the correlation between SII and SIRI and the prevalence of cardiovascular disease (CVD) in populations with obesity remains unknown.
Methods: This is a cross-sectional study with data obtained from the National Health and Nutrition Examination Survey from 1999 to 2018. SII and SIRI were calculated using the following equations: SII = (platelet count × neutrophil count)/lymphocyte count. SIRI = (neutrophil count × monocyte count)/lymphocyte count. Spearman’s rank correlation coefficient was used to assess the relationship between SII and SIRI and baseline variables. Logistic regression models and generalized additive model (GAM) with a spline smoothing function were used to evaluate the association between SIRI and CVD prevalence. Nomogram and receiver operating characteristic curve (ROC) analysis were used to assess the value of the risk prediction model.
Results: A total of 17,261 participants with obesity and SII and SIRI publicly available data were used for this study. Multivariate logistic regression analysis revealed that SIRI, rather than SII, was an independent risk factor for CVD prevalence. For every standard deviation increase in SIRI, there was a 13%, 15%, and 28% increase in the odds ratios of CVD prevalence (OR = 1.13, 95% CI: 1.04–1.22, P = 0.01), coronary heart disease (OR = 1.15, 95% CI: 1.05–1.26, P = 0.002), and congestive heart failure (OR = 1.28, 95% CI: 1.16–1.41, P < 0.001). ROC results demonstrated that SIRI had a certain accuracy in predicting CVD prevalence (AUC = 0.604), especially when combined with other variables used in the nomogram (AUC = 0.828). The smooth curve fitting regression analysis demonstrated a significant linear association between the risk of SIRI and the odds ratio of CVD prevalence (P for nonlinear = 0.275).
Conclusions: SIRI is a relatively stable indicator of inflammation and is independently associated with the prevalence of CVD. It may serve as a novel inflammatory indicator to estimate CVD prevalence in populations with obesity.
The increasing prevalence of obesity (BMI ≥ 30 kg/m2) is a serious public health concern worldwide. In the United States, the prevalence of obesity in adults has risen from 30.5% to 42.4% over the past two decades, with nearly 10% classified as severely obese (BMI ≥ 40 kg/m2) (1). Elevated adipokines levels, secreted by adipocytes trigger a chronic inflammatory response that can lead to various chronic systemic diseases, including heart attack, stroke, nonalcoholic fatty liver disease (NAFLD), diabetes mellitus (DM), and multiple cancer types (2–4). Consequently, obesity and its related conditions have significant physical and economic impacts, placing an additional burden on individuals and health insurance systems (5, 6).
Although previous studies have reported that several indicators (e.g., vitamin D, interleukin- 6 [IL-6], C-reactive protein [CRP]) could reflect inflammation levels and affect the prognosis of chronic diseases (7–10), using single or a few inflammatory makers are insufficient to assess the overall inflammatory state of the body since these markers may have anti- or pro-inflammatory functions in different biological processes (11). Moreover, composite inflammatory indicators evaluated using different hematological parameters are more representative of the overall inflammatory state.
Systematic immune inflammation index (SII) and systemic inflammation response index (SIRI) are novel markers of inflammatory diseases (12). These two indexes used neutrophil counts and lymphocyte counts respectively. The calculation formula for SII is and for lymphocyte count. The principle behind these new indexes is based on the roles of different types of blood cells in the inflammatory response, comprehensively reflecting the state of immunity and inflammation (13). Recent studies have explored the value of SII and SIRI in predicting cardiovascular events (13, 14). Obese individuals have a higher risk of cardiovascular disease (CVD) due to metabolic disorders and more intense inflammatory responses (2). However, the association between SII and SIRI and the prevalence of CVD in individuals with obesity remains unclear. In this study, we comprehensively investigated the relationship between SII and SIRI with the prevalence of CVD in obese patients.
This cross-sectional study utilized data from the National Health and Nutrition Examination Survey (NHANES) conducted between 1999 and 2018. NHANES is a survey of the civilian non-institutionalized population in the United States that uses a complex stratified multistage probability design (15). The survey includes interviews, physical examinations [at home or a mobile examination center (MEC)], and laboratory tests and is conducted every 2 years. During the period 1999–2018, NHANES conducted 10 separate cycles. Each cycle included entirely new participants, and each participant had a unique identification number, ensuring the uniqueness of the data. Therefore, we could determine that there was no overlap of participants between cycles. In addition, when participants were included in a particular cycle, their baseline clinical characteristics were interviewed or collected during that cycle. NHANES was conducted by the National Center for Health Statistics of the Centers for Disease Control and Prevention (CDC) and approved by the NHANES Institutional Review Board, with written informed consent obtained from all participants.
A total of 23,480 adults (age >18 years), who were classified as obese, were included in the NHANES survey between 1999 and 2018. Of these, 3,997 participants were excluded due to the inability to calculate SII or SIRI data. Of the remaining 19,483 participants, 542 pregnant women and 1,680 cancer patients were excluded, leaving 17,261 obese adults for the analysis.
The Beckman Coulter MAXM instrument at the MEC produced complete blood cell counts on blood specimens. Detailed specimen collection and processing instructions are available in the Laboratory/Medical Technologists Procedures Manual (16).
SII and SIRI were calculated using the following formulas (17, 18):
SII = (platelet count × neutrophil count)/lymphocyte count
SIRI = (neutrophil count × monocyte count)/lymphocyte count
CVD is a composite of a group of specific cardiovascular diseases, including heart attack, angina, congestive heart failure, coronary heart disease, and stroke (19). The diagnosis of specific CVD was based on self-reported medical history in the medical conditions section of NHANES. The primary outcome of this study is the prevalence of CVD. The secondary outcome is the prevalence of five specific CVDs, including heart attack, angina, congestive heart failure, coronary heart disease, and stroke.
Standardized questionnaires were used to collect information on age, gender, ethnicity, education level, family income, smoking and drinking behavior, medical history, and medication use. Medical history was based on self-reported previous medical records from a healthcare professional or a physician. Biochemical parameters were measured using a rigorous procedure, the details of which are provided in the NHANES Procedures Manual for Laboratory/Medical Technologists (16). To facilitate data integration, the following variables were further classified:
(i) Ethnicity: Non-Hispanic white, non-Hispanic black, Mexican American, or other ethnicities
(ii) Educational level: Less than 9th grade, 9–11th grade/high school or equivalent, college graduate, or above
(iii) Smoking status: Never (<100 cigarettes/lifetime), former smoker (≥100 cigarettes/lifetime and currently not smoking), or current smoker (>100 cigarettes/lifetime and currently smoking some days or every day) (20)
(iv) Drinking status: Never (<12 drinks/lifetime), former drinker (≥12 drinks/lifetime but not in the past year), current light/moderate drinker (≤1 drink/day for women and ≤2 drinks/day for men in the past year), or current heavy drinker (>1 drink/day for women and >2 drinks/day for men in the past year) (21)
To provide nationally representative estimates, MEC weights were utilized in data analysis to account for oversampling, non-response, and non-coverage. Details on weighting methods are available on the NHANES website (https://wwwn.cdc.gov/nchs/nhanes).
Baseline demographic characteristics were presented as means (standard error, SE) and continuous variables and weighted percentages (95% confidence interval, CI) for categorical variables. Spearman's rank correlation coefficient was used to determine the association between SII and SIRI and baseline variables, including age, BMI, alanine aminotransferase (ALT), aspartate aminotransferase (AST), total cholesterol (TC), high-density lipoprotein cholesterol (HDL), estimated glomerular filtration rate (eGFR), and medical history. Quartiles were used to categorize the level of SII and SIRI as categorical variables. A standard normal distribution of SII and SIRI [mean = 0, standard deviation (SD) = 1] was created by standardizing the Z-scores.
Multivariate logistic regression models were used to calculate odds ratios (ORs) and 95% CIs between SII and SIRI levels (quartiles and per-SD) and CVD prevalence. Baseline variables were considered as candidate predictors for the multivariate regression model. Confounding covariates were progressively added to different models. Subgroup analyses were performed stratified by clinical characteristics, including sex (male or female), age (<65 or ≥65 years), body mass index (30–34.9, 35–39.9, or ≥40 kg/m2), ethnicity (non-Hispanic white, non-Hispanic black, Mexican American, and others), DM (no or yes), hypertension (no or yes), hyperlipidemia (no or yes), and asthma (no or yes). In subgroup analyses, the effect of SIRI level on the odds ratio of CVD prevalence with each 1 SD increase was assessed. Meanwhile, interaction P values between each stratification variable (e.g., male/female) were calculated to examine the consistency of the trend toward CVD prevalence with each 1 SD increase in SIRI level. If an interaction p-value of less than 0.05 is observed, this suggests that the association between SIRI and CVD prevalence in this stratification is significantly different across subgroups, with the association likely to be more pronounced in one subgroup than the others.
A generalized additive model (GAM) with a spline smoothing function was used to assess the dose-response relationship between SIRI levels and CVD prevalence. Nonlinear P-values were obtained using the log-likelihood ratio test (22). If nonlinear P-value is less than 0.05, the fitted curve is nonlinear and vice versa. For nonlinear association, threshold effect analysis based on a two-piecewise linear regression model was performed to calculate the inflection point in the smoothing curve at which the relationship between SIRI and CVD prevalence changed significantly (23).
Nomograms were constructed for clinical applications using logistic regression models. Variables were screened using univariate analysis and multivariate logistic regression. The discriminative power of the model was assessed using the area under the curve (AUC) and calibration of risk predictions via the bias-corrected calibration intercept (i.e., predicted vs. observed outcome) (24). Furthermore, decision curve analysis (DCA) was performed by estimating the net benefit at different threshold probabilities to determine the suitability of the established column line graphs for clinical application (25).
All analyses were conducted using the statistical packages R (version 4.2.1, R Foundation) and EmpowerStats (version 4.2.0, www.R-project.org, X&Y Solutions, Inc., Boston, MA). Bilateral P-values less than 0.05 were considered statistically significant.
A total of 17,261 participants with obesity were included, representing 68,648,287 individuals. The average age of the participants was 48.3 years, and 44.9% were male. Participants with obesity were divided into Q1, Q2, Q3, and Q4 based on SII and SIRI values. Significant differences were found in age, poverty income ratio (PIR), BMI, HDL, TC, ALT, AST, gender, ethnicity, education levels, DM, hyperlipidemia, smoking, and antihypertensive use (all P < 0.05) for SII. Similarly, for SIRI, significant differences were observed between participants with higher and lower SIRI values in age, PIR, BMI, HDL, TC, ALT, gender, ethnicity, education levels, DM, hypertension, smoking, drinking, antihypertensive use and glucose-lowering drug use (all P < 0.05). The detailed results of baseline characteristics of participants with obesity are presented in Supplementary Table S1 (for SII) and Table 1 (for SIRI).
Table 1. Survey-weighted baseline characteristics of the obese population in NHANES from 1999 to 2018 according to SIRI quartiles (N = 17,261, representing 68,648,287 individuals with obesity).
Spearman correlation analysis revealed that, for SII, the prevalence of CVD was negatively correlated with age [Spearman correlation coefficient (rho) = −0.05, P < 0.001], gender (rho = −0.10, P < 0.001), ethnicity (rho = −0.06, P < 0.001), ALT (rho = −0.09, P < 0.001), and AST (rho = −0.13, P < 0.001). However, the prevalence of CVD was positively correlated with BMI (rho = 0.1, P < 0.001) and hyperlipidemia (rho = 0.03, P < 0.001). Moreover, the prevalence of CVD was not statistically correlated with the variables, including HDL (P = 0.31), TC (P = 0.21), eGFR (P = 0.09), DM (P = 0.14), hypertension (P = 0.54), and asthma (P = 0.70). Additionally, for SIRI, CVD prevalence was negatively correlated with ethnicity (rho = −0.12, P < 0.001), HDL (rho = −0.09, P < 0.001), TC (rho = −0.08, P < 0.001), and eGFR (rho = −0.11, P < 0.001) and positively correlated with age (rho = 0.07, P < 0.001), gender (rho = 0.13, P < 0.001), BMI (rho = 0.06, P < 0.001), DM (rho = 0.07, P < 0.001), hypertension (rho = 0.07, P < 0.001), and hyperlipidemia (rho = 0.02, P < 0.001) but not significantly correlated with ALT, AST, and asthma (all P > 0.05). The results are presented in Supplementary Table S2.
Survey-weighted logistic regression was performed to examine the relationship between SII and SIRI levels and the prevalence of CVD among the obese population. However, we found no evidence to suggest that SII was an independent risk factor for the prevalence of CVD (Supplementary Table S3). Additionally, in the crude model, the odds ratios of CVD prevalence increased significantly with the increase in SIRI level, including heart attack, angina, coronary heart disease, congestive heart failure, and stroke in the crude model (P for trend <0.001). Moreover, per-SD increase in SIRI levels increased the prevalence of CVD by 35% (1.35 [1.27–1.44]), heart attack by 32% [1.32 (1.24–1.41)], angina by 23% [1.23 (1.14–1.32)], coronary heart disease by 37% [1.37 (1.27–1.47)], congestive heart failure by 41% [1.41 (1.31–1.53)], and stroke by 24% [1.24 (1.16–1.32)], respectively. Besides, this relationship persisted in model 1 after adjusting for demographic variables, including age, gender, ethnicity, education levels, and PIR, except for angina (P for trend = 0.067). Furthermore, in models 2 and 3, multivariable regression analysis revealed that SIRI levels were independent risk factors for CVD, including coronary heart disease and congestive heart failure. Moreover, per-SD increase in SIRI was associated with a 13%, 15%, and 28% increase in the risk of CVD (OR = 1.13, 95% CI: 1.04–1.22, P = 0.01), coronary heart disease (OR = 1.15, 95% CI: 1.05–1.26, P = 0.002), and congestive heart failure (OR = 1.28, 95% CI: 1.16–1.41, P < 0.001), respectively, in the corresponding model 3, with maximum adjusted covariates (Table 2).
Table 2. Survey-weighted logistic regression examining the association of SIRI with the prevalence of cardiovascular diseases in the obese population.
Subgroup analyses assessed the performance of SIRI with respect to the prevalence of CVD in some subpopulations. Our findings were robust in most subgroups, including gender, age, BMI, ethnicity, DM, asthma, hyperlipidemia, and hypertension (all P for interaction > 0.05). However, the association between SIRI and CVD differed by age in the subgroups (Figure 1). The findings revealed that SIRI was more likely to promote the development of CVD in participants aged ≥65 years (OR = 1.23, 95% CI: 1.13–1.34, P = 0.01).
Figure 1. Subgroups were analyzed for the association between an increase in SIRI per one standard deviation and the odds ratios of CVD prevalence, stratified according to different clinical characteristics. The model was adjusted for age, gender, ethnicity, education levels, poverty income ratio (PIR), body mass index (BMI), estimated glomerular filtration rate (eGFR), alanine aminotransferase (ALT), total cholesterol (TC), high-density lipoprotein cholesterol (HDL), diabetes mellitus (DM), hypertension, antihypertensives and glucose-lowering drugs, and smoking and drinking behavior.
We constructed a nomogram to assess the probability of CVD prevalence using baseline variables that were screened by univariate analysis and multivariate logistic regression models (Supplementary Tables S4 and S5). Finally, ten variables were selected for nomogram construction, including SIRI, age, gender, PIR, BMI, eGFR, DM, hyperlipidemia, hypertension, and smoke. Each variable had a corresponding hazard point, and total points were obtained by summing the points of each variable (Figure 2A).
Figure 2. Nomogram and receiver operating characteristics (ROC) curves to evaluate the risk of CVD in participants with obesity. (A) Nomogram to predict CVD prevalence. In the DM, hypertension, and hyperlipidemia groups, 0 means “no,” and 1 means “yes”. In the smoking group, 0 means “never”, 1 means “former”, and 2 means “current”. In the gender group, 0 means “female”, and 2 means “male”. (B) ROC curve of SIRI for the prevalence of CVD model. (C) ROC curves for SIRI combined with clinical variables in the nomogram for the CVD prevalence model. SIRI, systemic inflammation response index; ROC, receiver operating characteristic.
Moreover, ROC analyses were used to evaluate the predictive value of SIRI in CVD prevalence. SIRI showed a certain accuracy in predicting CVD prevalence (AUC = 0.604, 95% CI: 0.59–0.62) (Figure 2B). In addition, a robust result was revealed when we performed ROC analysis in combination with other variables in the nomogram (AUC = 0.832, 95% CI: 0.82–0.84) (Figure 2C). Finally, calibration curves (Supplementary Figure S1A) and decision curve analysis (DCA) (Supplementary Figure S1B) demonstrated a stable consistency between predicted and actual probabilities.
Smooth curve fitting regression analysis was conducted to estimate the relationship between the odds ratio of SIRI and CVD prevalence based on model 3 (Figure 3). A significant linear association was observed between the odds ratio of SIRI and CVD prevalence (P for nonlinear = 0.275). Specifically, for each 1-unit increase in SIRI, the prevalence of CVD increased by 20% (OR = 1.20, 95% CI: 1.12–1.30, P < 0.001).
Figure 3. Smooth spline analysis of the relationship between SIRI and the risk of CVD prevalence. Smooth spline analysis was performed through generalized addictive model 3. In the plot, the risk of CVD prevalence increased proportionally with the increment of SIRI, and P for nonlinear = 0.275, confirming that the correlation between SIRI and CVD prevalence was linear in the whole range of SIRI.
This study is the first cross-sectional study based on participants with obesity to analyze the association between SII, SIRI, and CVD. The study included 17,261 individuals with obesity, and the results can be described as follows, (1) SIRI was an independent risk factor for the prevalence of CVD in participants, whereas SII was not; (2) Higher SIRI values were significantly associated with an increased odds ratio of CVD prevalence; (3) SIRI showed a linear relationship with the risk of CVD prevalence, with a 20% increase in risk per 1-unit increase in SIRI.
Previous studies have explored the association between SII and SIRI and CVD and have investigated the predictive value of these markers using different epidemiological methods. A meta-analysis demonstrated that higher SII was an independent risk factor for the incidence of CVD (HR = 1.39, 95% CI: 1.20–1.61, P < 0.001) (26). SII and SIRI were also demonstrated to have a significant value in predicting coronary artery disease and acute coronary syndrome (27). Moreover, a population-based cohort of 85,154 participants in Kailuan exhibited that SII (HR = 1.246, 95% CI: 1.157–1.382) and SIRI (HR = 1.194, 95% CI: 1.087–1.313) were positively associated with an increased risk of stroke (28). However, in our study, we did not observe an association between SII and CVD, including heart attack, angina, coronary heart disease, congestive heart failure, and stroke. The results of univariate cox regression analysis confirmed that SII was not an independent risk factor for the prevalence of CVD. In contrast, SIRI was found to be closely associated with the prevalence of CVD.
Monocytes, neutrophils, and lymphocytes are key components of SIRI (18). Monocytes have a critical role in the inflammatory response and the progression of atherosclerosis (29, 30). Specifically, monocytes migrate to the subendothelium by binding adhesion molecules expressed on the damaged vascular endothelium and gradually mature into macrophages. These cells subsequently bind to class A scavenger receptors (SR-A) and CD-36 to take up oxidized low-density lipoproteins (LDL) and release pro-inflammatory and pro-oxidant cytokines at inflammation sites, causing atherosclerosis (31, 32). Furthermore, the pro-inflammatory and pro-oxidative effects of monocytes are manifested by inhibiting the migration of macrophages and the oxidation of LDL and promoting the accumulation of cholesterol in the vascular wall to lower HDL levels, contributing to the incidence of CVD (33–35).
Neutrophils exhibit a unique defense role in the inflammatory response through degranulation, phagocytosis, production of reactive oxygen species, and construction of neutrophil extethnicityllular traps (NETs) (36). These cytokines activate sterile inflammatory responses in the body, while interactions with vascular endothelial cells and platelets promote immune thrombosis, leading to atherosclerosis and CVD (36, 37). A large cohort study involving 775,231 participants demonstrated that neutrophils counts are closely associated with the incidence of CVD, including heart failure (HR: 2.04, 95% CI: 1.82–2.29), peripheral arterial disease (HR: 1.95, 95% CI: 1.72–2.21), unheralded coronary death (HR: 1.78, 95% CI: 1.51–2.10), abdominal aortic aneurysm (HR: 1.72, 95% CI: 1.34–2.21), and nonfatal myocardial infarction (HR: 1.58, 95% CI: 1.42–1.76) (38).
In contrast, lymphocytes manifest different roles than neutrophils and monocytes in regulating the inflammatory response of the body. In vivo experiments also demonstrated that B lymphocyte deficiency promotes the development of atherosclerosis (39). Additionally, clinical studies have shown that low lymphocyte counts are associated with poorer prognosis in patients with CVD, including heart failure, chronic ischemic heart disease, and acute coronary syndrome (40–42). These results suggest the value of SIRI at the cellular level in assessing the inflammatory response of the organism.
A subgroup analysis was performed to estimate if our findings were stable in common subgroups. The survey-weighted logistic regression model was adjusted for all covariates used in model 3. The analysis showed that our findings were robust regarding gender, BMI, ethnicity, DM, asthma, hyperlipidemia, and hypertension. However, a significant difference was found between SIRI and CVD prevalence varied by age. The increase in SIRI may contribute to an increased risk of CVD prevalence in participants aged ≥65 years. Progressive decline in the structure and function of multiple organs are the distinctive features of aging (43). During this complex biological process, vascular remodeling, endothelial dysfunction, and loss of vascular compliance significantly increased the incidence of CVD (44–46). Moreover, aging leads to an increase in fat mass and exhibits chronic low-grade inflammation, as well as is associated with the development of insulin resistance, dyslipidemia, hypertension, and type 2 diabetes mellitus (T2DM) (47, 48). The interaction of these factors increases the risk of CVD prevalence. SIRI is a composite indicator that represents the inflammation in the body. This may explain the exacerbated risk of CVD by SIRI in participants with obesity aged ≥65 years. Similarly, Jun et al. showed that the effect of SIRI on CVD was more likely to be found in participants aged ≥50 years (OR = 1.43, 95% CI: 1.17–1.74) than in those aged <50 years (OR = 1.35, 95% CI: 1.00–1.81) (49). These results indicate that our findings are stable in most populations.
This study was a seminal evaluation of the association of SIRI with CVD prevalence in obese individuals. We revealed a correlation between the dynamic model of SIRI and CVD prevalence, which could be utilized to assess the risk of CVD prevalence in populations with obesity.
However, there are still some limitations to our study. First, because the participants were solely recruited from the United States, our findings can only reflect the situation in this region. Therefore, multicenter investigations are needed in the future. Second, this cross-sectional study lacked follow-up data to demonstrate a causal relationship between SIRI and CVD prevalence or to predict patient outcomes. Third, the U.S. is a diverse country with a population from various ethnic and cultural backgrounds. NHANES assesses the health status and related factors of U.S. permanent residents The NHANES study categorizes race as non-Hispanic black, non-Hispanic white, Mexican American, other Hispanic, and other multiracial. BMI is a global tool used to assess whether an individual is overweight or obese. The cutoff value for obesity may vary due to differences in body composition and health risks among different races. Finally, we did not collect information on participants’ infectious diseases and drugs, which could influence peripheral blood counts and partially affect the results.
SIRI is independently associated with the prevalence of CVD in individuals with obesity. SIRI demonstrated a better performance in predicting CVD prevalence than SII. Therefore, SIRI could be considered a novel inflammatory indicator to estimate the prevalence of CVD in patients with obesity.
The original contributions presented in the study are included in the article/Supplementary Materials, further inquiries can be directed to the corresponding author.
The studies involving humans were approved by National Center for Health Statistics Institutional Review Board. The studies were conducted in accordance with the local legislation and institutional requirements. The participants provided their written informed consent to participate in this study.
ZL: Conceptualization, Data curation, Formal Analysis, Investigation, Methodology, Resources, Software, Validation, Visualization, Writing – original draft, Writing – review & editing. LZ: Conceptualization, Data curation, Formal Analysis, Investigation, Methodology, Project administration, Resources, Software, Supervision, Validation, Visualization, Writing – original draft, Writing – review & editing.
The author(s) declare that no financial support was received for the research, authorship, and/or publication of this article.
The authors declare that the research was conducted in the absence of any commercial or financial relationships that could be construed as a potential conflict of interest.
All claims expressed in this article are solely those of the authors and do not necessarily represent those of their affiliated organizations, or those of the publisher, the editors and the reviewers. Any product that may be evaluated in this article, or claim that may be made by its manufacturer, is not guaranteed or endorsed by the publisher.
The Supplementary Material for this article can be found online at: https://www.frontiersin.org/articles/10.3389/fcvm.2024.1361088/full#supplementary-material
1. Hales CM, Carroll MD, Fryar CD, Ogden CL. Prevalence of obesity and severe obesity among adults: United States, 2017-2018. NCHS Data Brief. (2020) (360):1–8.
2. Chartrand DJ, Murphy-Després A, Alméras N, Lemieux I, Larose E, Després JP. Overweight, obesity, and CVD risk: a focus on visceral/ectopic fat. Curr Atheroscler Rep. (2022) 24(4):185–95. doi: 10.1007/s11883-022-00996-x
3. Rohm TV, Meier DT, Olefsky JM, Donath MY. Inflammation in obesity, diabetes, and related disorders. Immunity. (2022) 55(1):31–55. doi: 10.1016/j.immuni.2021.12.013
4. Kim DS, Scherer PE. Obesity, diabetes, and increased cancer progression. Diabetes Metab J. (2021) 45(6):799–812. doi: 10.4093/dmj.2021.0077
5. Cawley J, Biener A, Meyerhoefer C, Ding Y, Zvenyach T, Smolarz BG, et al. Direct medical costs of obesity in the United States and the most populous states. J Manag Care Spec Pharm. (2021) 27(3):354–66.33470881
6. Apovian CM. Obesity: definition, comorbidities, causes, and burden. Am J Manag Care. (2016) 22(7 Suppl):s176–85.27356115
7. Finch SL, Rosenberg AM, Kusalik AJ, Maleki F, Rezaei E, Baxter-Jones A, et al. Higher concentrations of vitamin D in Canadian children with juvenile idiopathic arthritis compared to healthy controls are associated with more frequent use of vitamin D supplements and season of birth. Nutr Res. (2021) 92:139–49. doi: 10.1016/j.nutres.2021.05.007
8. van de Logt F, Day AS. S100a12: a noninvasive marker of inflammation in inflammatory bowel disease. J Dig Dis. (2013) 14(2):62–7. doi: 10.1111/1751-2980.12012
9. Eichberger J, Resch E, Resch B. Diagnosis of neonatal sepsis: the role of inflammatory markers. Front Pediatr. (2022) 10:840288. doi: 10.3389/fped.2022.840288
10. Jones DR, Graham-Engeland JE. Positive affect and peripheral inflammatory markers among adults: a narrative review. Psychoneuroendocrinology. (2021) 123:104892. doi: 10.1016/j.psyneuen.2020.104892
11. Zlotnik A, Yoshie O. The chemokine superfamily revisited. Immunity. (2012) 36(5):705–16. doi: 10.1016/j.immuni.2012.05.008
12. Wang RH, Wen WX, Jiang ZP, Du ZP, Ma ZH, Lu AL, et al. The clinical value of neutrophil-to-lymphocyte ratio (NLR), systemic immune-inflammation index (SII), platelet-to-lymphocyte ratio (PLR) and systemic inflammation response index (SIRI) for predicting the occurrence and severity of pneumonia in patients with intracerebral hemorrhage. Front Immunol. (2023) 14:1115031. doi: 10.3389/fimmu.2023.1115031
13. Li Q, Ma X, Shao Q, Yang Z, Wang Y, Gao F, et al. Prognostic impact of multiple lymphocyte-based inflammatory indices in acute coronary syndrome patients. Front Cardiovasc Med. (2022) 9:811790. doi: 10.3389/fcvm.2022.811790
14. Wang P, Guo X, Zhou Y, Li Z, Yu S, Sun Y, et al. Monocyte-to-high-density lipoprotein ratio and systemic inflammation response index are associated with the risk of metabolic disorders and cardiovascular diseases in general rural population. Front Endocrinol (Lausanne). (2022) 13:944991. doi: 10.3389/fendo.2022.944991
15. Parker JD, Kruszon-Moran D, Mohadjer LK, Dohrmann SM, Van de Kerckhove W, Clark J, et al. National health and nutrition examination survey: California and Los Angeles county, estimation methods and analytic considerations, 1999–2006 and 2007–2014. Vital Health Stat 2. (2017) (173):1–26.
17. Hu B, Yang XR, Xu Y, Sun YF, Sun C, Guo W, et al. Systemic immune-inflammation index predicts prognosis of patients after curative resection for hepatocellular carcinoma. Clin Cancer Res. (2014) 20(23):6212–22. doi: 10.1158/1078-0432.CCR-14-0442
18. Qi Q, Zhuang L, Shen Y, Geng Y, Yu S, Chen H, et al. A novel systemic inflammation response index (SIRI) for predicting the survival of patients with pancreatic cancer after chemotherapy. Cancer. (2016) 122(14):2158–67. doi: 10.1002/cncr.30057
19. Einarson TR, Acs A, Ludwig C, Panton UH. Prevalence of cardiovascular disease in type 2 diabetes: a systematic literature review of scientific evidence from across the world in 2007–2017. Cardiovasc Diabetol. (2018) 17(1):83. doi: 10.1186/s12933-018-0728-6
20. ALHarthi SSY, Natto ZS, Midle JB, Gyurko R, O’Neill R, Steffensen B. Association between time since quitting smoking and periodontitis in former smokers in the national health and nutrition examination surveys (NHANES) 2009 to 2012. J Periodontol. (2019) 90:16–25. doi: 10.1002/JPER.18-0183
21. Hicks CW, Wang D, Matsushita K, Windham BG, Selvin E. Peripheral neuropathy and all-cause and cardiovascular mortality in U.S. adults: a prospective cohort study. Ann Intern Med. (2021) 174:167–74. doi: 10.7326/M20-1340
22. Garzotto M, Beer TM, Hudson RG, Peters L, Hsieh Y-C, Barrera E, et al. Improved detection of prostate cancer using classification and regression tree analysis. J Clin Oncol. (2005) 23:4322–9. doi: 10.1200/JCO.2005.11.136
23. Liu Y, Zhao P, Cheng M, Yu L, Cheng Z, Fan L, et al. AST to ALT ratio and arterial stiffness in non-fatty liver Japanese population: a secondary analysis based on a cross-sectional study. Lipids Health Dis. (2018) 17:275. doi: 10.1186/s12944-018-0920-4
24. van der Naalt J, Timmerman ME, de Koning ME, van der Horn HJ, Scheenen ME, Jacobs B, et al. Early predictors of outcome after mild traumatic brain injury (UPFRONT): an observational cohort study. Lancet Neurol. (2017) 16(7):532–40. doi: 10.1016/S1474-4422(17)30117-5
25. Vickers AJ, Holland F. Decision curve analysis to evaluate the clinical benefit of prediction models. Spine J. (2021) 21(10):1643–8. doi: 10.1016/j.spinee.2021.02.024
26. Ye Z, Hu T, Wang J, Xiao R, Liao X, Liu M, et al. Systemic immune-inflammation index as a potential biomarker of cardiovascular diseases: a systematic review and meta-analysis. Front Cardiovasc Med. (2022) 9:933913. doi: 10.3389/fcvm.2022.933913
27. Dziedzic EA, Gąsior JS, Tuzimek A, Paleczny J, Junka A, Dąbrowski M, et al. Investigation of the associations of novel inflammatory biomarkers-systemic inflammatory Index (SII) and systemic inflammatory response index (SIRI)-with the severity of coronary artery disease and acute coronary syndrome occurrence. Int J Mol Sci. (2022) 23(17):9553. doi: 10.3390/ijms23179553
28. Jin Z, Wu Q, Chen S, Gao J, Li X, Zhang X, et al. The associations of two novel inflammation indexes, SII and SIRI with the risks for cardiovascular diseases and all-cause mortality: a ten-year follow-up study in 85,154 individuals. J Inflamm Res. (2021) 14:131–40. doi: 10.2147/JIR.S283835
29. Jaipersad AS, Lip GY, Silverman S, Shantsila E. The role of monocytes in angiogenesis and atherosclerosis. J Am Coll Cardiol. (2014) 63(1):1–11. doi: 10.1016/j.jacc.2013.09.019
30. Groh L, Keating ST, Joosten LAB, Netea MG, Riksen NP. Monocyte and macrophage immunometabolism in atherosclerosis. Semin Immunopathol. (2018) 40(2):203–14. doi: 10.1007/s00281-017-0656-7
31. Kundi H, Kiziltunc E, Cetin M, Cicekcioglu H, Cetin ZG, Cicek G, et al. Association of monocyte/HDL-C ratio with SYNTAX scores in patients with stable coronary artery disease. Herz. (2016) 41(6):523–9. doi: 10.1007/s00059-015-4393-1
32. Ganjali S, Gotto AM Jr, Ruscica M, Atkin SL, Butler AE, Banach M, et al. Monocyte-to-HDL-cholesterol ratio as a prognostic marker in cardiovascular diseases. J Cell Physiol. (2018) 233(12):9237–46. doi: 10.1002/jcp.27028
33. Usta A, Avci E, Bulbul CB, Kadi H, Adali E. The monocyte counts to HDL cholesterol ratio in obese and lean patients with polycystic ovary syndrome. Reprod Biol Endocrinol. (2018) 16(1):34. doi: 10.1186/s12958-018-0351-0
34. Ponec M, Kempenaar JA, Havekes L, Van der Schroeff JG, Emeis JJ, Vermeer BJ. Effects of LDL, HDL and their combination on the endogenous cholesterol synthesis in monocyte macrophages. Biochim Biophys Acta. (1981) 666(3):405–10. doi: 10.1016/0005-2760(81)90299-X
35. Inonu Koseoglu H, Pazarli AC, Kanbay A, Demir O. Monocyte count/HDL cholesterol ratio and cardiovascular disease in patients with obstructive sleep apnea syndrome: a multicenter study. Clin Appl Thromb Hemost. (2018) 24(1):139–44. doi: 10.1177/1076029616677803
36. Shirakawa K, Sano M. Neutrophils and neutrophil extracellular traps in cardiovascular disease: an overview and potential therapeutic approaches. Biomedicines. (2022) 10(8):1850. doi: 10.3390/biomedicines10081850
37. Sanda GE, Belur AD, Teague HL, Mehta NN. Emerging associations between neutrophils, atherosclerosis, and psoriasis. Curr Atheroscler Rep. (2017) 19(12):53. doi: 10.1007/s11883-017-0692-8
38. Shah AD, Denaxas S, Nicholas O, Hingorani AD, Hemingway H. Neutrophil counts and initial presentation of 12 cardiovascular diseases: a CALIBER cohort study. J Am Coll Cardiol. (2017) 69(9):1160–9. doi: 10.1016/j.jacc.2016.12.022
39. Major AS, Fazio S, Linton MF. B-lymphocyte deficiency increases atherosclerosis in LDL receptor-null mice. Arterioscler Thromb Vasc Biol. (2002) 22(11):1892–8. doi: 10.1161/01.ATV.0000039169.47943.EE
40. Núñez J, Miñana G, Bodí V, Núñez E, Sanchis J, Husser O, et al. Low lymphocyte count and cardiovascular diseases. Curr Med Chem. (2011) 18(21):3226–33. doi: 10.2174/092986711796391633
41. Núñez J, Sanchis J, Bodí V, Núñez E, Mainar L, Heatta AM, et al. Relationship between low lymphocyte count and major cardiac events in patients with acute chest pain, a non-diagnostic electrocardiogram and normal troponin levels. Atherosclerosis. (2009) 206(1):251–7. doi: 10.1016/j.atherosclerosis.2009.01.029
42. Ommen SR, Gibbons RJ, Hodge DO, Thomson SP. Usefulness of the lymphocyte concentration as a prognostic marker in coronary artery disease. Am J Cardiol. (1997) 79(6):812–4. doi: 10.1016/S0002-9149(96)00878-8
43. Buford TW. Hypertension and aging. Ageing Res Rev. (2016) 26:96–111. doi: 10.1016/j.arr.2016.01.007
44. Han K, Shi D, Yang L, Wang Z, Li Y, Gao F, et al. Prognostic value of systemic inflammatory response index in patients with acute coronary syndrome undergoing percutaneous coronary intervention. Ann Med. (2022) 54(1):1667–77. doi: 10.1080/07853890.2022.2083671
45. Paneni F, Diaz Cañestro C, Libby P, Lüscher TF, Camici GG. The aging cardiovascular system: understanding it at the cellular and clinical levels. J Am Coll Cardiol. (2017) 69(15):1952–67. doi: 10.1016/j.jacc.2017.01.064
46. Donato AJ, Morgan RG, Walker AE, Lesniewski LA. Cellular and molecular biology of aging endothelial cells. J Mol Cell Cardiol. (2015) 89(Pt B):122–35. doi: 10.1016/j.yjmcc.2015.01.021
47. Franceschi C, Campisi J. Chronic inflammation (inflammaging) and its potential contribution to age-associated diseases. J Gerontol A Biol Sci Med Sci. (2014) 69(Suppl 1):S4–9. doi: 10.1093/gerona/glu057
48. Dominguez LJ, Barbagallo M. The biology of the metabolic syndrome and aging. Curr Opin Clin Nutr Metab Care. (2016) 19(1):5–11. doi: 10.1097/MCO.0000000000000243
Keywords: systemic immune-inflammation index, systemic inflammation response index, cardiovascular diseases, inflammation, obesity, prevalence
Citation: Liu Z and Zheng L (2024) Associations between SII, SIRI, and cardiovascular disease in obese individuals: a nationwide cross-sectional analysis. Front. Cardiovasc. Med. 11:1361088. doi: 10.3389/fcvm.2024.1361088
Received: 24 December 2023; Accepted: 30 July 2024;
Published: 22 August 2024.
Edited by:
Otto Alexander Sanchez, Minneapolis Heart Institute Foundation (MHIF), United StatesReviewed by:
Zhongyan Du, Zhejiang Chinese Medical University, ChinaCopyright: © 2024 Liu and Zheng. This is an open-access article distributed under the terms of the Creative Commons Attribution License (CC BY). The use, distribution or reproduction in other forums is permitted, provided the original author(s) and the copyright owner(s) are credited and that the original publication in this journal is cited, in accordance with accepted academic practice. No use, distribution or reproduction is permitted which does not comply with these terms.
*Correspondence: Longxuan Zheng, emhlbmdsb25neHVhbjFAMTYzLmNvbQ==
†These authors share first authorship
Disclaimer: All claims expressed in this article are solely those of the authors and do not necessarily represent those of their affiliated organizations, or those of the publisher, the editors and the reviewers. Any product that may be evaluated in this article or claim that may be made by its manufacturer is not guaranteed or endorsed by the publisher.
Research integrity at Frontiers
Learn more about the work of our research integrity team to safeguard the quality of each article we publish.