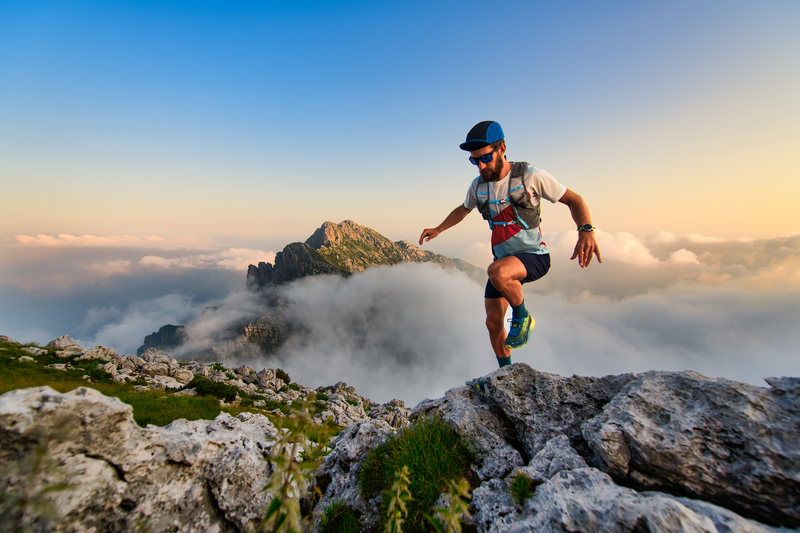
95% of researchers rate our articles as excellent or good
Learn more about the work of our research integrity team to safeguard the quality of each article we publish.
Find out more
ORIGINAL RESEARCH article
Front. Cardiovasc. Med. , 25 April 2024
Sec. Cardio-Oncology
Volume 11 - 2024 | https://doi.org/10.3389/fcvm.2024.1344515
This article is part of the Research Topic Mendelian Randomization and Cardiovascular Diseases View all 16 articles
Background: Multiple observational studies have shown associations between thyroid cancer (TC) and cardiovascular diseases (CVDs). However, the results were inconsistent, and the potential causal genetic relationship remains unclear.
Methods: The genetic instruments of TC and CVDs were derived from data obtained through genome-wide association studies (GWAS). We performed the two-sample Mendelian randomization(MR) methods to investigate the causality of TC on CVDs. Summary-level statistics for CVDs, including heart failure (HF), atrial fibrillation (AF), coronary artery disease (CAD), myocardial infarction (MI), ischemic stroke (IS) and venous thromboembolism (VTE). The primary method employed in this MR analysis was the Inverse Variance Weighted (IVW) approach, and four additional algorithms were used: MR-Egger, weighted median, simple mode, and weighted mode. Additionally, we assessed the reliability of the causal relationship through pleiotropy, heterogeneity and leave-one-out sensitivity analysis.
Results: In this MR analysis, we only detected causality of genetically predicted TC on HF (IVW method, odds ratio (OR) = 1.00134, 95% confidence interval (CI): 1.00023–1.00244, p = 0.017). However, There were no causal associations of TC with CAD, MI, AF, IS, and VTE.
Conclusion: Our results confirmed the causal association between TC and HF. It is crucial to closely monitor the incidence of HF in TC patients and give comprehensive clinical intervention based on conventional treatment.
In recent years, epidemiological data from both domestic and international sources indicated that cancer and CVDs ranked as the top two in terms of both incidence and mortality rates (1). Many individuals diagnosed with cancer face a notable prevalence of cardiovascular events, and CVDs have emerged as a leading cause of mortality among cancer patients (2).CVDs might arise from cancer treatments such as radiation therapy, chemotherapy, targeted therapy, and other medications. Additionally, disruptions in endocrine and metabolic functions caused by cancer can contribute to these cardiovascular issues (3). If patients discontinue cancer treatment due to adverse cardiovascular events, it can further exacerbate their condition, posing a life-threatening risk. Hence, having a clear understanding of the relationship between cancer and CVDs is crucial.
Thyroid cancer (TC) is the most common malignant tumor of the endocrine system, and in recent years, its incidence has been rapidly increasing worldwide (4). In 2020 alone, there were 586,000 reported cases of TC globally, and it was anticipated to become the fourth most prevalent cancer in the United States by 2030 (5–7). Globally, CVDs are one of the leading causes of death and disability (8). It has been reported that 21.7% of TC patients’ deaths are related to coronary artery diseases (CADs) (9, 10). Many observational studies have indicated that TC patients are at increased risk of heart failure (HF), atrial fibrillation (AF), coronary artery disease (CAD), venous thromboembolism (VTE), and stroke (11–15). However, a meta-analysis revealed that the risk of HF in TC patients is lower [RR = 0.98, 95% CI (0.60–1.59)]; furthermore, after adjusting for cardiovascular risk factors, TC patients did not have a significant increase in the risk of ischemic heart disease, stroke, or HF (12). Meanwhile, Another study failed to find a significant association between a high risk of CVDs or AF and patients with TC (16). It is worth noting that these observational studies are susceptible to potential confounding factors and biases related to reverse causation. Factors such as the thyroid function status, medication side effects, and surgeries undergone by TC patients could impact the outcomes of CVDs. Therefore, It is important to note that traditional observational studies cannot elucidate the causal relationship between TC and CVDs. Therefore, establishing the causal relationship between TC and CVDs is of paramount importance.
MR serves as a robust and powerful tool for causal inference, enabling examining of the influence of exposure factors on outcomes by using genetic variants as instrumental variables (IVs) (17, 18). Due to the random assignment of alleles of IVs at conception, they remain unaffected by postnatal environmental factors, effectively mitigating concerns related to reverse causation (19). Furthermore, we can disregard study costs and ethics. Given that there was currently no evidence indicating a potential causal relationship between TC and CVDs, we carried out the two-sample MR study to investigate the causality of TC on the following six CVDs outcomes including HF, AF, CAD, MI, IS, and VTE.
To explore the potential causal relationship between TC and CVDs, we performed MR analysis using the data obtained from GWAS summary statistics (https://www.ebi.ac.uk/gwas). The MR design is based on three assumptions: (1) the genetic variants are closely associated with TC. (2) The genetic variants are unrelated to any confounding factors. (3) The genetic variants are exclusively linked to CVDs via TC (20). The schematic diagram of the study design is illustrated in Figure 1.
Figure 1. Procedures for the MR analysis of causal associations between TC and CVDs (including HF, AF, CAD, MI, IS, and VTE). SNP, single nucleotide polymorphism.
We searched for traits related to TC in a large-scale GWAS (https://gwas.mrcieu.ac.uk/datasets/ieu-a-1082), including 649 cases and 431 controls (21). For the outcome dataset, HF GWAS data were derived from the FinnGen consortium, which included 23,397 cases and 194,811 controls (https://www.finngen.fi/en). GWAS data for AF were also obtained from FinnGen consortium providing a total of 10,516 cases and 116,926 controls (https://www.finngen.fi/en).The summary dataset for CAD was derived from a public GWAS meta-analysis, providing a total of 122,733 cases and 424,528 controls (22). MI GWAS data were retrieved from the FinnGen consortium, including 12,801 cases and 205,991 controls (https://www.finngen.fi/en). Summary statistics for IS were obtained from the MEASTROKE consortium, including 7,193 cases and 406,111 controls (23). Summary level data for VTE were derived from FinnGen consortium, including 9,176 cases and 209,616 controls (https://www.finngen.fi/en). As the original studies were publicly available, it was unnecessary to apply for additional ethics consent and informed consent. The characteristics of the dataset are presented in Table 1.
We selected qualified SNPs as the genetic IVs for MR analysis. The stringent criteria for IVs are as follows: (1) Remove SNPs with linkage disequilibrium (r2 < 0.001 within windows 10,000 kb for variants in the locus), SNPs significantly associated with TC (p < 5 × 10−8) (24); (2) Non-rare SNPs (MAF ≥ 0.01); (3)Not using SNP proxies. (4) Calculating the strength of IVs to avoid weak-tool bias: F = R² (N-K-1)/[K(1-R²)]; R² = {β/[se(β) * √N]}2 (25, 26). Here, N represents the sample size, and K represents the number of SNPs, we will exclude SNPs if F < 10. (5) To exclude potential pleiotropic effects, we comprehensively searched previously published literature for risk factors associated with CVDs, And by searching for SNP information on the PhenoScanner V2 website (http://www.phenoscanner.medschl.cam.ac.uk/) (27).
All statistical analyses were conducted using the “TwoSampleMR” package in R version 4.3.1. We harmonized the aggregated SNP-TC and SNP-CVDs statistics to ensure the consistency of alleles for each SNP between TC and CVDs. And, we investigated the causal association of TC with HF, AF, CAD, MI, IS, and VTE. The primary analytical method for assessing the causal relationship is the IVW method, along with four additional algorithms, including MR-Egger, Weighted Median, Simple Mode, and Weighted Mode. p-value < 0.05 indicates statistical significance. MR-Egger intercept is applied to assess horizontal pleiotropy of SNPs, and if p < 0.05, the IVW estimate might be biased (28, 29). Cochran's Q test is performed to estimate the heterogeneity of SNPs. P-value indicates significant heterogeneity in the analysis results (30). Furthermore, we used the MR pleiotropy residual sum and outlier (MR-PRESSO) test to detect outliers. If outliers are identified, they will be removed, and the analysis will be re-conducted with the remaining SNPs (31). In addition, we conducted a “leave-one-out” sensitivity analysis to determine whether significant results were driven by a single SNP (32).
The associations between TC and CVDs were quantified using the OR and corresponding 95%CI. A p-value less than 0.05 suggests a potential causal relationship.
For the IVs of TC, 347 SNPs that reached the generally accepted genome-wide significance threshold (p < 5 × 10−8, r2 < 0.001, kb = 10,000). None of these 347 SNPs were excluded as the F-statistics for each selected SNP was greater than 10 (range from 29.136 to 1,330.179). Subsequently, after assessing the SNP dataset using the PhenoScanner database, We excluded 8 SNPs associated with confounding factors: rs2566511, r1096258, rs7623609, r6546667, rs461599, rs12441088, rs8007859, and rs2157787. The remaining 339 SNPs were used for further analysis. (Detailed information can be found in Supplementary Material S1).
The results of the analysis are shown in Figure 2. The IVW method showed that higher genetically determined TC was associated with an increased risk of HF [OR = 1.00134, 95% CI (1.00023–1.00244), p = 0.017]. In other words, the incidence of HF in TC patients was 1.00134 times higher than in the control group. But there were no significant associations of TC with AF[OR = 1.00020, 95% CI (0.99830–1.00211), p = 0.834], CAD [OR = 1.00031, 95% CI (0.99957–1.00106), p = 0.406], MI (OR = 1.00110, 95% CI [0.99961–1.00259], p = 0.147), IS [OR = 1.00070, 95% CI (0.99883–1.00256), p = 0.464], and VTE [OR = 0.99987, 95% CI (0.99840–1.00135), p = 0.865].
Figure 2. The risk association between TC and CVDs (including HF, AF, CAD, MI, IS, and VTE) in the training set visualized in a forest plot.
The results are shown in Table 2. Sensitivity analyses include tests for pleiotropy, heterogeneity, and leave-one-out analyses. For HF, AF, CAD, MI, IS and VTE, there were no evidences of horizontal pleiotropy across the analyses in the Egger regression (p for intercept >0.05). In addition, We conducted IVW analysis and MR-Egger analysis to assess heterogeneity. For HF, MI, IS, and VTE, Both IVW analysis and MR-Egger analysis did not indicate the heterogeneity. However, the sensitivity analyses for TC on AF and CAD showed heterogeneity. MR-PRESSO was used to identify and remove outliers. If outliers were detected, they would be removed, and the remaining IVs would be reanalyzed. For AF, three outlier SNPs were identified: rs1889562, rs2239214, and rs8076927. After removing these three outliers, the results still indicated no causal relationship between TC and AF, with no signs of horizontal pleiotropy and heterogeneity (Detailed information can be found in Supplementary Material S2). For CAD, there is no significant outliers.
Scatter and funnel plots can provide a more intuitive representation of heterogeneity, as illustrated in Supplementary Figures S1, S3, S4. The leave-one-out method indicated the combined results of the remaining SNPs showed a predominantly linear trend after deleting each SNP. Thus, the robustness of our results was not influenced by any single SNP (Detailed information can be found in Supplementary Figures S2, S5–S10.
The MR-Egger intercept test indicated the lack of horizontal pleiotropy, suggesting that the IVs did not impact the outcome through factors unrelated to TC. The p-value for Cochran's Q statistic was greater than 0.05, indicating no heterogeneity among SNPs. Additionally, leave-one-out analysis suggested that the potential causal relationship between TC and the risk of HF was not driven by a single SNP. These sensitivity test results confirmed the robustness of the findings. However, we did not find sufficient evidence to support the association of TC with AF, CAD, MI, IS, or VTE.
Our results were not entirely consistent with previous studies. A observational study had shown that there was no significant increase in the risk of HF in TC patients compared to the general population [relative risk = 0.98, 95%CI(0.60–1.59)] (12). Despite this study revealed that the risk of HF in TC patients is lower. We found that in this meta-analysis, all the studied TC patients underwent thyroidectomy. Due to the intervention received by the study population, this influenced the outcome to a certain extent. This may be the reason for the inconsistency with our research results. In addition, a single-centered, cross-sectional study indicated TC patients had a significant burden of HF, which to some extent supports our research findings (11).
Two meta-analyses suggested that the risk of AF in TC was approximately 1.5 times that of the healthy control group (33, 34).This may be attributed to the Thyroidectomy as well as the iatrogenic hyperthyroid state caused by long-term suppressive therapy with thyroid hormone. Additionally, a study suggested that the risk of CAD was significantly increased in patients with TC compared to the general population (33). However, these changes were almost invariably related to alterations in thyroid function or long-term suppression of thyroid-stimulating hormone due to treatment. Besides, studies by Pajamaki et al. and Toulis et al. did not demonstrate a significant risk of CAD in patients with TC (35, 36). Qiang et al. found suggested that, following adjustments for cardiovascular risk factors, individuals with TC faced an elevated risk of AF; And however, there was no significant increase in the risk of ischemic heart disease, stroke, or HF (12). They pointed out significant heterogeneity in the study results, influenced by potential confounding factors in the original research. The impact of confounding factors such as TC treatment, thyroid hormone levels, and heterogeneity in the study population could not be ruled out.
A meta-analysis indicated a significant association between TC and a higher risk of cerebrovascular disease and AF (10). It was worth noting that the authors did not specifically indicate which cerebrovascular diseases were associated with TC. As is well known, there is an association between cancer and a higher incidence of VTE. In a case-control study, it was found that patients with TC who had distant metastasis or recent surgery had an increased risk of developing VTE (15). However, this study did not consider factors such as pregnancy, targeted therapy, systemic treatment, and radiation therapy as risk factors for VTE occurrence.
In summary,we are aware that observational studies have their limitations. Firstly, the number of eligible studies included in meta-analyses is often limited. Secondly, observational studies are susceptible to various confounding factors and biases, and their internal validity can be compromised. Thirdly, there is inevitable heterogeneity in exposure measurement, endpoint determination, statistical analysis, data collection methods, drug interventions, disease states, etc in each study. Moreover, the primary role of the thyroid is to synthesize, store, and release thyroid hormones (TH), including T3 and T4, which are regulated and controlled by thyroid-stimulating hormone (TSH). TC can lead to thyroid dysfunction, including hyperthyroidism or hypothyroidism. At the same time, there are TH receptors in myocardial and vascular tissues, and minor fluctuations in TH concentrations can affect cardiovascular physiology (37). Baumgartner et al. found that Even if thyroid hormone levels are within the normal range, an elevated FT4 level with a relatively low TSH level may still be associated with an increased risk of AF (38). However, an MR analysis did not show a causal relationship between normal-range thyroid function and CAD (39).
Compared to traditional observational epidemiological studies, MR research utilizes large-scale GWAS datasets. The MR study was conducted based on a population with European ancestry to minimize the impact of population stratification on the research results. In MR analysis, genetic variants were used as IVs, simulating, to some extent, the design of a randomized controlled trial. This approach helpes mitigate biases introduced by confounding factors and eliminates the possibility of reverse causation, thereby strengthening causal inference. Therefore, our study results have specific clinical implications. For diagnosed TC patients, clinicians should pay more attention to the potential risk of HF and intervene promptly for treatment.
However, our study has some limitations. First, to minimize the impact of population bias or heterogeneity as much as possible, the participants in the study were Europeans. However, this study did not involve trans-ethnic populations, such as East Asian populations. So the participants in the study were primarily Europeans, so the representativeness of the study results across the entire population needs further validation and the study results lack generalizability. Second, due to the complex and poorly understood biological functions of many genetic variants, we could not completely eliminate the impact of horizontal pleiotropy on the results. Third, the relatively low OR values limit their clinical relevance and guidance. Fourth, because detailed demographic information and clinical characteristics of the subjects are lacking, we are unable to perform a subgroup analysis. Finaly, regrettably, we failed to find some assumed clinical cases demonstrating the same OR level in the cardiovascular field. This will also be a focus of our future research exploration efforts.
In this MR analysis-based study, we identified a potential causal relationship between TC and HF, suggesting a possible association between TC and cardiovascular health. However, our study results did not support a risk relationship between TC and other CVDs, such as AF, CAD, MI, IS, and VTE. These findings suggest that the impact of TC on cardiovascular health may be limited, but there might be a particular association with the risk of HF. Therefore, given study's limitations, we emphasize the necessity for further research to validate these results and gain a more comprehensive understanding of the relationship between TC and CVDs.
The original contributions presented in the study are included in the article/Supplementary Material, further inquiries can be directed to the corresponding author.
YG: Writing – review & editing, Writing – original draft, Visualization, Validation, Supervision, Software, Methodology, Investigation, Formal Analysis, Data curation, Conceptualization. ZW: Writing – review & editing, Visualization, Software, Methodology, Investigation, Formal Analysis, Data curation. JY: Writing – review & editing, Visualization, Methodology, Investigation, Formal Analysis, Data curation. LC: Writing – review & editing, Visualization, Supervision, Resources, Methodology, Funding acquisition, Formal Analysis, Conceptualization.
The author(s) declare that no financial support was received for the research, authorship, and/or publication of this article.
This study is based on the database created and maintained by the Integrative Epidemiology Unit Open GWAS database at the University of Bristol, UK. The IEU Open GWAS database provides a significant resource for the scientific community and researchers to understand the impact of genes on health and disease. We appreciate their contributions as well as the participants and researchers involved in these studies; without them, this work would not have been possible.
The authors declare that the research was conducted in the absence of any commercial or financial relationships that could be construed as a potential conflict of interest.
All claims expressed in this article are solely those of the authors and do not necessarily represent those of their affiliated organizations, or those of the publisher, the editors and the reviewers. Any product that may be evaluated in this article, or claim that may be made by its manufacturer, is not guaranteed or endorsed by the publisher.
The Supplementary Material for this article can be found online at: https://www.frontiersin.org/articles/10.3389/fcvm.2024.1344515/full#supplementary-material
1. Lipshultz SE, Adams MJ, Colan SD, Constine LS, Herman EH, Hsu DT, et al. Long-term cardiovascular toxicity in children, adolescents, and young adults who receive cancer therapy: pathophysiology, course, monitoring, management, prevention, and research directions: a scientific statement from the American heart association. Circulation. (2013) 128(17):1927–95. doi: 10.1161/CIR.0b013e3182a88099
2. Koene RJ, Prizment AE, Blaes A, Konety SH. Shared risk factors in cardiovascular disease and cancer. Circulation. (2016) 133(11):1104–14. doi: 10.1161/CIRCULATIONAHA.115.020406
3. Rossello X. Cardiovascular risk assessment in survivors of cancer. Lancet. (2023) 401(10374):321–3. doi: 10.1016/S0140-6736(22)02582-X
4. Liu Y, Wang J, Hu X, Pan Z, Xu T, Xu J, et al. Radioiodine therapy in advanced differentiated thyroid cancer: resistance and overcoming strategy. Drug Resist Updat. (2023) 68:100939. doi: 10.1016/j.drup.2023.100939
5. Rahib L, Smith BD, Aizenberg R, Rosenzweig AB, Fleshman JM, Matrisian LM. Projecting cancer incidence and deaths to 2030: the unexpected burden of thyroid, liver, and pancreas cancers in the United States. Cancer Res. (2014) 74(11):2913–21. doi: 10.1158/0008-5472.CAN-14-0155
6. La Vecchia C, Malvezzi M, Bosetti C, Garavello W, Garavello P, Levi F, et al. Thyroid cancer mortality and incidence: a global overview. Int J Cancer. (2015) 136(9):2187–95. doi: 10.1002/ijc.29251
7. Miranda-Filho A, Lortet-Tieulent J, Bray F, Cao B, Franceschi S, Vaccarella S, et al. Thyroid cancer incidence trends by histology in 25 countries: a population-based study. Lancet Diabetes Endocrinol. (2021) 9(4):225–34. doi: 10.1016/S2213-8587(21)00027-9
8. Roth GA, Mensah GA, Johnson CO, Addolorato G, Ammiratiet E, Baddour LM, et al. Global burden of cardiovascular diseases and risk factors, 1990–2019: update from the GBD 2019 study. J Am Coll Cardiol. (2020) 76(25):2982–3021. doi: 10.1016/j.jacc.2020.11.010
9. Du B, Wang F, Wu L, Wang Z, Zhang D, Huang Z, et al. Cause-specific mortality after diagnosis of thyroid cancer: a large population-based study. Endocrine. (2021) 72(1):179–89. doi: 10.1007/s12020-020-02445-8
10. Tsai WH, Zeng YH, Lee CC, Chien MN, Liu SC, Chien KL, et al. Association between thyroid cancer and cardiovascular disease: a meta-analysis. Front Cardiovasc Med. (2023) 10:1075844. doi: 10.3389/fcvm.2023.1075844
11. Li Q, Liu F, Tang Y, Lee S, Lang C, Bai L, et al. The distribution of cardiovascular-related comorbidities in different adult-onset cancers and related risk factors: analysis of 10 year retrospective data. Front Cardiovasc Med. (2021) 8:695454. doi: 10.3389/fcvm.2021.695454
12. Qiang JK, Alwithenani R, Uleryk E, Ezzat S, Lipscombe LL, Sawka AM. Risk of adverse cardiovascular outcomes in differentiated thyroid cancer survivors: a systematic review and meta-analysis. Thyroid. (2023) 33(2):192–202. doi: 10.1089/thy.2022.0209
13. Suh B, Shin DW, Park Y, Lim H, Yun JM, Song SO, et al. Increased cardiovascular risk in thyroid cancer patients taking levothyroxine: a nationwide cohort study in Korea. Eur J Endocrinol. (2019) 180(1):11–20. doi: 10.1530/EJE-18-0551
14. Izkhakov E, Keinan-Boker L, Barchana M, Shacham Y, Yaish I, Carmel Neiderman NN, et al. Long-term all-cause mortality and its association with cardiovascular risk factors in thyroid cancer survivors: an Israeli population-based study. BMC Cancer. (2020) 20(1):892. doi: 10.1186/s12885-020-07401-3
15. van der Boom T, Klein Hesselink EN, Kooistra H, Meijer K, van der Horst-Schrivers A, Lefrandt JD, et al. Risk factors for venous thromboembolism in patients treated for differentiated thyroid carcinoma. Endocr Relat Cancer. (2017) 24(6):267–73. doi: 10.1530/ERC-17-0013
16. Zoltek M, Andersson TM, Hedman C, Ihre-Lundgren C, Nordenvall C. Cardiovascular incidence in 6900 patients with differentiated thyroid cancer: a Swedish nationwide study. World J Surg. (2020) 44(2):436–41. doi: 10.1007/s00268-019-05249-8
17. Smith GD, Ebrahim S. ‘Mendelian randomization’: can genetic epidemiology contribute to understanding environmental determinants of disease. Int J Epidemiol. (2003) 32(1):1–22. doi: 10.1093/ije/dyg070
18. Davey Smith G, Hemani G. Mendelian Randomization: genetic anchors for causal inference in epidemiological studies. Hum Mol Genet. (2014) 23(R1):R89–98. doi: 10.1093/hmg/ddu328
19. Nattel S. Canadian journal of cardiology January 2013: genetics and more. Can J Cardiol. (2013) 29(1):1–2. doi: 10.1016/j.cjca.2012.11.015
20. Scosyrev E. Identification of causal effects using instrumental variables in randomized trials with stochastic compliance. Biom J. (2013) 55(1):97–113. doi: 10.1002/bimj.201200104
21. Köhler A, Chen B, Gemignani F, Elisei R, Romei C, Figlioli G, et al. Genome-wide association study on differentiated thyroid cancer. J Clin Endocrinol Metab. (2013) 98(10):E1674–81. doi: 10.1210/jc.2013-1941
22. van der Harst P, Verweij N. Identification of 64 novel genetic loci provides an expanded view on the genetic architecture of coronary artery disease. Circ Res. (2018) 122(3):433–43. doi: 10.1161/CIRCRESAHA.117.312086
23. Malik R, Chauhan G, Traylor M, Sargurupremraj M, Okada Y, Mishra A, et al. Multiancestry genome-wide association study of 520,000 subjects identifies 32 loci associated with stroke and stroke subtypes. Nat Genet. (2018) 50(4):524–37. doi: 10.1038/s41588-018-0058-3
24. Altshuler D, Durbin RM, Abecasis GR, Bentley DR, Chakravarti A, Clark AG, et al. A map of human genome variation from population-scale sequencing. Nature. (2010) 467(7319):1061–73. doi: 10.1038/nature09534
25. Pritchard JK, Przeworski M. Linkage disequilibrium in humans: models and data. Am J Hum Genet. (2001) 69(1):1–14. doi: 10.1086/321275
26. Dai Z, Xu W, Ding R, Peng X, Shen X, Song J, et al. Two-sample Mendelian randomization analysis evaluates causal associations between inflammatory bowel disease and osteoporosis. Front Public Health. (2023) 11:1151837. doi: 10.3389/fpubh.2023.1151837
27. Kamat MA, Blackshaw JA, Young R, Surendran P, Burgess S, Danesh J, et al. Phenoscanner V2: an expanded tool for searching human genotype-phenotype associations. Bioinformatics. (2019) 35(22):4851–3. doi: 10.1093/bioinformatics/btz469
28. Bowden J, Del Greco MF, Minelli C, Davey Smith G, Sheehan NA, Thompson JR. Assessing the suitability of summary data for two-sample Mendelian randomization analyses using MR-egger regression: the role of the I2 statistic. Int J Epidemiol. (2016) 45(6):1961–74. doi: 10.1093/ije/dyw220
29. Verbanck M, Chen CY, Neale B, Do R. Detection of widespread horizontal pleiotropy in causal relationships inferred from Mendelian randomization between complex traits and diseases. Nat Genet. (2018) 50(5):693–8. doi: 10.1038/s41588-018-0099-7
30. Kulinskaya E, Dollinger MB. An accurate test for homogeneity of odds ratios based on cochran’s Q-statistic. BMC Med Res Methodol. (2015) 15:49. doi: 10.1186/s12874-015-0034-x
31. Ong JS, MacGregor S. Implementing MR-PRESSO and GCTA-GSMR for pleiotropy assessment in Mendelian randomization studies from a practitioner’s perspective. Genet Epidemiol. (2019) 43(6):609–16. doi: 10.1002/gepi.22207
32. Cheng H, Garrick DJ, Fernando RL. Efficient strategies for leave-one-out cross validation for genomic best linear unbiased prediction. J Anim Sci Biotechnol. (2017) 8:38. doi: 10.1186/s40104-017-0164-6
33. Lee EK, Ahn HY, Ku EJ, Yoo WS, Lee YK, Nam KH, et al. Cardiovascular outcomes in thyroid cancer patients treated with thyroidectomy: a meta-analysis. J Clin Endocrinol Metab. (2021) 106(12):3644–54. doi: 10.1210/clinem/dgab576
34. Kostopoulos G, Doundoulakis I, Antza C, Bouras E, Nirantharakumar K, Tsiachris D, et al. Incident atrial fibrillation in patients with differentiated thyroid cancer: a meta-analysis. Endocr Relat Cancer. (2021) 28(5):325–35. doi: 10.1530/ERC-20-0496
35. Toulis KA, Viola D, Gkoutos G, Keerthy D, Boelaert K, Nirantharakumar K. Risk of incident circulatory disease in patients treated for differentiated thyroid carcinoma with no history of cardiovascular disease. Clin Endocrinol (Oxf). (2019) 91(2):323–30. doi: 10.1111/cen.13990
36. Pajamäki N, Metso S, Hakala T, Ebeling T, Huhtala H, Ryödi E, et al. Long-term cardiovascular morbidity and mortality in patients treated for differentiated thyroid cancer. Clin Endocrinol (Oxf). (2018) 88(2):303–10. doi: 10.1111/cen.13519
37. Razvi S, Jabbar A, Pingitore A, Danzi S, Biondi B, Klein I, et al. Thyroid hormones and cardiovascular function and diseases. J Am Coll Cardiol. (2018) 71(16):1781–96. doi: 10.1016/j.jacc.2018.02.045
38. Baumgartner C, da Costa BR, Collet TH, Feller M, Floriani C, Bauer DC. Thyroid function within the normal range, subclinical hypothyroidism, and the risk of atrial fibrillation. Circulation. (2017) 136(22):2100–16. doi: 10.1161/CIRCULATIONAHA.117.028753
Keywords: thyroid cancer, cardiovascular diseases, Mendelian randomization, the causal link, genome-wide association study
Citation: Gao Y, Wang Z, Yu J and Chen L (2024) Thyroid cancer and cardiovascular diseases: a Mendelian randomization study. Front. Cardiovasc. Med. 11:1344515. doi: 10.3389/fcvm.2024.1344515
Received: 26 November 2023; Accepted: 4 March 2024;
Published: 25 April 2024.
Edited by:
Panpan Hao, Shandong University, ChinaReviewed by:
Toru Maruyama, Kyushu University, Japan© 2024 Gao, Wang, Yu and Chen. This is an open-access article distributed under the terms of the Creative Commons Attribution License (CC BY). The use, distribution or reproduction in other forums is permitted, provided the original author(s) and the copyright owner(s) are credited and that the original publication in this journal is cited, in accordance with accepted academic practice. No use, distribution or reproduction is permitted which does not comply with these terms.
*Correspondence: Lijun Chen MTUxMjI0NzE3MjBAMTYzLmNvbQ==
Disclaimer: All claims expressed in this article are solely those of the authors and do not necessarily represent those of their affiliated organizations, or those of the publisher, the editors and the reviewers. Any product that may be evaluated in this article or claim that may be made by its manufacturer is not guaranteed or endorsed by the publisher.
Research integrity at Frontiers
Learn more about the work of our research integrity team to safeguard the quality of each article we publish.