- 1Department of Radiology, First Affiliated Hospital of Zhengzhou University, Zhengzhou, China
- 2CT Imaging Research Center, GE Healthcare China, Beijing, China
Objective: This study aims to investigate the image quality of a high-resolution, low-dose coronary CT angiography (CCTA) with deep learning image reconstruction (DLIR) and second-generation motion correction algorithms, namely, SnapShot Freeze 2 (SSF2) algorithm, and its diagnostic accuracy for in-stent restenosis (ISR) in patients after percutaneous coronary intervention (PCI), in comparison with standard-dose CCTA with high-definition mode reconstructed by adaptive statistical iterative reconstruction Veo algorithm (ASIR-V) and the first-generation motion correction algorithm, namely, SnapShot Freeze 1 (SSF1).
Methods: Patients after PCI and suspected of having ISR scheduled for high-resolution CCTA (randomly for 100 kVp low-dose CCTA or 120 kVp standard-dose) and invasive coronary angiography (ICA) were prospectively enrolled in this study. After the basic information pairing, a total of 105 patients were divided into the LD group (60 patients underwent 100 kVp low-dose CCTA reconstructed with DLIR and SSF2) and the SD group (45 patients underwent 120 kVp standard-dose CCTA reconstructed with ASIR-V and SSF1). Radiation and contrast medium doses, objective image quality including CT value, image noise (standard deviation), signal-to-noise ratio (SNR), and contrast-to-noise ratio (CNR) for the aorta, left main artery (LMA), left ascending artery (LAD), left circumflex artery (LCX), and right coronary artery (RCA) of the two groups were compared. A five-point scoring system was used for the overall image quality and stent appearance evaluation. Binary ISR was defined as an in-stent neointimal proliferation with diameter stenosis ≥50% to assess the diagnostic performance between the LD group and SD group with ICA as the standard reference.
Results: The LD group achieved better objective and subjective image quality than that of the SD group even with 39.1% radiation dose reduction and 28.0% contrast media reduction. The LD group improved the diagnostic accuracy for coronary ISR to 94.2% from the 83.8% of the SD group on the stent level and decreased the ratio of false-positive cases by 19.2%.
Conclusion: Compared with standard-dose CCTA with ASIR-V and SSF1, the high-resolution, low-dose CCTA with DLIR and SSF2 reconstruction algorithms further improves the image quality and diagnostic performance for coronary ISR at 39.1% radiation dose reduction and 28.0% contrast dose reduction.
Key points
• Coronary computed tomography angiography with deep learning image reconstruction (DLIR) and SnapShot Freeze 2 (SSF2) technologies provides better diagnostic performance on in-stent restenosis (ISR).
• The scan protocol combining 100 kVp tube voltage and high-strength deep learning image reconstruction algorithm protocol can be used to significantly reduce radiation and contrast doses in coronary computed tomography angiography compared with the conventional protocol.
• The second-generation motion correction algorithm (SSF2) further reduces heart motion artifacts compared with the first-generation motion correction algorithm (SSF1) in CCTA.
Introduction
Cardiovascular diseases (CADs) have gradually become one of the most critical factors threatening human health (1). Percutaneous coronary intervention (PCI), which could relieve coronary stenosis for myocardial revascularization with a high postoperative recanalization rate exceeding 90%, significantly improves the survival rates and quality of life for patients with CADs (2, 3). However, in-stent restenosis (ISR) remains one of the significant challenges for PCI. Although drug-eluting stents have significantly reduced the incidence of postoperative ISR compared with bare metal stents, nearly 10% of patients still suffer from ISR, which may be diagnosed by using the non-invasive coronary computed tomography angiography (CCTA) (4–6).
Various new imaging techniques are used to improve the spatial and temporal resolution in CCTA and to improve the visualization of the in-stent lumen and the diagnostic accuracy of ISR, such as wide-coverage CT detectors, high-resolution CT scan mode, motion correction algorithms, and image reconstruction algorithms (7, 8).
On the one hand, the metal artifacts introduced by the stents themselves could cause unclear stent boundaries that affect the observation of thrombus and calcified plaques on the vessel wall, which may cause the overdiagnosis of ISR. These metal artifacts may be reduced by using high tube voltages and high spatial resolution. On the other hand, the high spatial resolution required by CCTAs in patients with stents is still typically associated with the high radiation dosage, and the contrast medium dose is increased accordingly. Therefore, there is a need to reduce radiation dose and contrast dose while still maintaining high spatial resolution in imaging metal stents (9, 10).
Recently, new CT technologies, including the 230 μm high spatial resolution scan mode with focal-spot wabble (Hi-Res mode), the second-generation motion correction algorithm [SnapShot Freeze 2 (SSF2)], and the state-of-the-art deep learning image reconstruction (DLIR), have been introduced and integrated into a new CT scanner. Studies have shown that based on the deep convolutional neural network, DLIR could assist the “double-low” scan protocol (low radiation dose and contrast medium dose) while producing high-quality clinical CCTA images (11–13).
We thus hypothesize that the combination of these new CT technologies could be used to further improve image temporal and spatial resolution and reduce image noise at even reduced radiation and contrast medium doses. To our knowledge, few studies have been established to investigate the combination of the Hi-Res scan mode with SSF2 and DLIR algorithm on radiation and contrast dose reduction for CCTA in patients after PCI. Therefore, in this study, we assessed the image quality and accuracy in diagnosing ISR using “double-low” CCTA reconstructed with DLIR and SSF2 compared with standard-dose CCTA under high-definition reconstruction mode with volume-based adaptive statistical iterative reconstruction (ASIR-V) algorithm and first-generation motion correction algorithm (SSF1).
Materials and methods
Study population
This prospective study was approved by the ethics committee of our hospital, and all patients provided informed consent. A total of 123 patients who had undergone PCI with drug-eluting stents and who were scheduled for CCTA (randomly underwent low-dose CCTA or standard-dose CCTA) and invasive coronary angiography (ICA) from January 2022 to July 2022 were prospectively enrolled (Figure 1). The inclusion criteria included patients with prior PCI and implantation of a drug-eluting stent and those undergoing CCTA and ICA for suspected in-stent restenosis. The exclusion criteria included the following: (a) iodine contrast agent allergy; severe liver and kidney insufficiency; (b) decompensated cardiac insufficiency; (c) absence of ICA examinations or a CCTA and ICA interval longer than 1 month; and (e) patients with stent-in-stent or missing stent records (14). Finally, 105 patients were included in the study and were divided into the low-dose group (LD group) and standard-dose group (SD group) according to the CCTA protocols.
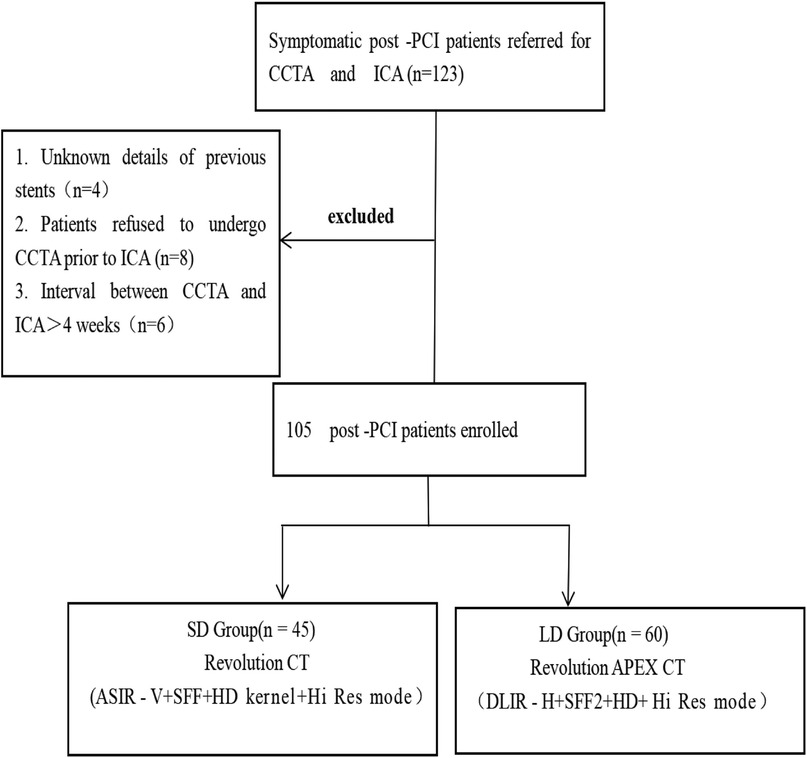
Figure 1 Flowchart of the study design. CCTA, coronary computed tomography angiography; ICA, invasive coronary angiography; PCI, percutaneous coronary intervention; DLIR-H, high-strength deep learning image reconstruction algorithm; ASIR-V, adaptive statistical iterative reconstruction Veo algorithm; Hi-Res mode, high-resolution mode; HD kernel, high-definition kernel.
CCTA acquisition
An oral and/or intravenous beta-blocker or non-dihydropyridine calcium channel was administered to patients presenting with a heart rate (HR) above 70 beats per minute to lower it below 70 beats per minute. However, in this subset of selected patients, further therapy was limited if it was deemed clinically unsafe to do so, based on symptoms such as lightheadedness or dyspnea or concerns of hypotension.
All scans were acquired in high-resolution mode (Hi-Res mode) with an in-plane spatial resolution of 230 μm, a layer thickness and an interval of 0.625 mm, a tube rotation time of 0.28 s, and a reconstruction matrix of 512 × 512. The patients in the low-dose group (LD group) were examined on a new 16 cm wide-detector CT scanner (Revolution Apex, GE HealthCare). The other scanning parameters for the LD group with Apex CT were as follows: tube voltage, 100 kVp; noise index, 36 HU; and tube current automatically adjusted between 500 mA and 1,300 mA. Images were reconstructed with high-level DLIR under the high-definition kernel and the second-generation SnapShot Freeze algorithm for cardiac motion correction [SnapShot Freeze 2 (SSF2), GE HealthCare]. The patients in the standard-dose group (SD group) were examined on a 16 cm wide-detector CT scanner (Revolution CT, GE HealthCare). The other scanning parameters for the SD group with Revolution CT were as follows: tube voltage, 120 kVp; noise index, 26 HU; and tube current automatically adjusted between 100 mA and 720 mA. Images were reconstructed with ASIR-V with 50% weight, and the first-generation SnapShot Freeze (SSF1, GE HealthCare) algorithm for cardiac motion correction (Table 1).
All CCTA scans used the prospective ECG-triggered axial scan mode. The z-axis coverages were chosen to be 120 mm, 140 mm, or 160 mm based on the heart size of different patients to optimize dose performance. The scan range for both groups covered 1 cm below the tracheal ridge to about 1 cm below the apex of the heart. According to the patient's heart rate before scanning, the auto-gating technique was used to select the ideal cardiac phases. For patients whose heart rates were <66 bpm, the exposure time window was 70%–80% of the RR interval; for patients with HR >86 bpm, the exposure time window was 40%–55% of the RR interval. Images of the whole heart would be acquired in one heartbeat for patients with an irregular heart rate (heart rate variability over 10 beats/min).
The patients were placed in the supine position for both groups, and sublingual nitroglycerin (0.5 mg) was administered to the patients before scanning to dilate the coronary arteries. Using the intelligent automatic tracking technology for the contrast agent, the trigger point was placed in the descending aorta (1 cm below the tracheal bifurcation), the trigger threshold was set to 150 HU, and the image acquisition started with a delay of 2 s after the threshold was reached. All patients in both LD and SD groups were injected through the right anterior elbow veins using a double-tube syringe with a non-ionizing contrast agent (ultrafast injection, concentration of 370 mgI/ml, Bayer Medicine BAYX). Regarding the contrast injection protocol, the LD group used a low-contrast medium protocol with a weight-dependent contrast dose rate of 18 mgI/kg/s, while the SD group used 32 mgI/kg/s. Both groups used 10 s injection time, and the flow rate for contrast injection was determined by dividing the total contrast medium volume by the 10 s injection time. For example, in the LD group, for patients with a body weight of 50 kg, the total specific volume would be (18 mgI/kg/s × 50 kg × 10 s)/(370 mg/ml) = 24 ml, and the flow rate would be 24 ml/10 s = 2.4 ml/s. After the injection of the contrast medium, both groups were injected with 30 ml of normal saline at the same flow rate.
Post-processing and image quality evaluation
For the objective evaluation, the axial images were transferred to the AW 4.7 Workstation, and all patient information was made anonymous. The maximum intensity projection (MPR), volume rendering (VR), maximum intensity projection (MIP), and curved planar reformat (CPR) images were then generated for image post-processing. Two radiologists both with 5 years of experience in CCTA conducted the objective assessment. A region of interest (ROI) of 2 mm diameter was used for coronary artery branches, avoiding vessel walls, plaques, and stenosis as much as possible. The ROIs for both groups were rigorously kept in the same size and placed in a similar place for both groups.
The CT attenuation value and image noise value in HU of the aorta, left main artery (LMA), left ascending artery (LAD), left circumflex artery (LCX), and right coronary artery (RCA) in the two groups were recorded and compared. The signal-to-noise ratio (SNR) and contrast-to-noise ratio (CNR) were calculated as follows:
where CT valuevessel is the mean CT value in HU of an ROI placed in the proximal coronary artery, Image Noisevessel is the mean image noise value in HU of an ROI placed in the proximal coronary artery, and CT valueadjacent epicardial fat is the mean CT value in HU of the adjacent epicardial fat tissue.
Subjective evaluation of the stents
The readers were blinded to clinical and group information. Two other radiologists, both with more than 8 years of experience in cardiovascular imaging, independently conducted the subjective evaluation of the images from the two groups using a five-point Likert scale for both the overall image quality and appearance of stents. For the overall image quality assessment: 5 = excellent (very little image noise and extremely readable images); 4 = good (good image quality without diagnostic limitation); 3 = acceptable (usual images in image interpretation); 2 = suboptimal (with some diagnostic limitation), and 1 = unacceptable (massive image noise and scarcely readable images). For the appearance of stent assessment: 5 = excellent (the edges of the stents are sharp, excellent attenuation of the vessel lumen, and clear delineation of the vessel walls); 4 = good (clear stent strut definition, minimal blooming artifacts from the stent); 3 = acceptable (minimal stent margin definition, some blooming artifacts); 2 = suboptimal (major artifacts affecting visualization of major structures of the stents), and 1 = unacceptable (poor vessel wall definition and severe artifacts). Images with a score of >2 were considered diagnostically acceptable (15).
ICA inspection
We used the ISR diagnosis by invasive coronary angiography (ICA) within 30 days as the standard reference to assess the diagnostic performance of CCTA on ISR. Binary ISR is defined as stenosis ≥50% in the stent or within 5 mm of both ends of the stent (14). We have identified stenosis ≥50% in the stents or within 5 mm of both ends of the stents on CCTA and then assessed accuracy, sensitivity, specificity, positive predictive value (PPV), and negative predictive value (NPV) for binary ISR of the LD and SD groups on patient level and stent level. Accuracies were further analyzed for large-caliber stents (diameter, ≥3 mm) and small-caliber stents (diameter, <3 mm).
Radiation dose and contrast dose
CT dose index (CTDIvol) and dose length product (DLP) were recorded after the scanning, and the effective dose was calculated as ED = k × DLP, where k = 0.014 mSv/mGy cm. Meanwhile, the contrast medium dose was also calculated and recorded.
Statistical analysis
The SPSS (v 21.0, IBM Corp.) statistical analysis software was used for statistical analysis. The normality of measurement data was tested using the Kolmogorov–Smirnov test. Measurement data that conformed to normal distribution were expressed as mean ± standard deviation, and the count data were expressed as frequencies and percentages. The normal distribution variables between the two groups were compared using the two independent samples Student's t-test. The Mann–Whitney test was used to compare groups of measurement data that did not obey the normal distribution. The chi-square test or Fisher’s exact test was used for the categorical variables for the two groups. A p-value of <0.05 was considered statistically significant.
Results
Baseline characteristics
A total of 123 patients underwent CCTAs, and 105 patients with 231 stents were eligible for enrollment, including 32 males and 73 females, aged 38–79 years, with an average age of 64.7 ± 10.9 years. There were no clinical differences between the LD and SD groups. Sixty patients in the LD group had a total of 138 stents implanted, and there were 53 stents with a diameter of ≥3 mm and 40 stents with a diameter of <3 mm. Forty-five patients in the SD group had a total of 93 stents implanted, and there were 81 stents with a diameter of ≥3 mm and 57 stents with a diameter of <3 mm. There was no significant difference in stent position between the two groups (Table 2).
Radiation dose and contrast dose
Compared with the SD group, the LD group had a 39.0% reduction in effective dose (1.00 ± 0.64 mSv vs. 1.64 ± 0.49 mSv, p < 0.001). Notably, compared with the SD group, the tube current of the LD group was increased by 35.7% (893.85 ± 119.47 mA vs. 574.34 ± 45.90 mA, p < 0.001) due to the use of lower tube voltage. Meanwhile, the contrast dose was also significantly reduced in the LD group with a reduction of 28.0% (34.74 ± 5.77 ml vs. 48.25 ± 7.45 ml, p < 0.001) (Table 2).
Image quality evaluation
There was no significant difference in CT values at AO, RCA, LAD, and LCX between the LD and SD groups. The image noise values in all vessels of the LD group were significantly lower than those of the SD group, and the SNR and CNR values in all vessels of the LD group were significantly higher than those of the SD group (all p < 0.001) (Figure 2). The subjective scores of both overall image quality and stent appearance of the LD group were better than those of the SD group. CT image quality was at least good in almost all patients, and 99.05% (104/105) of patients were scored over 3 (Table 3 and Figure 3).
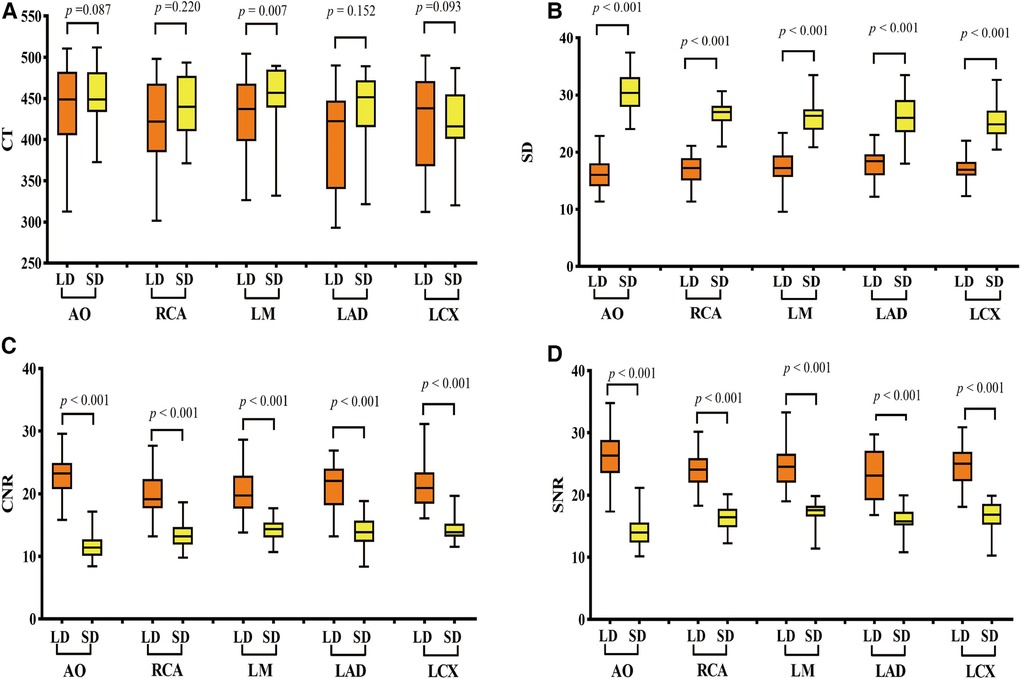
Figure 2 Comparison of objective image quality scores of vessels between the two groups. (A) CT values, (B) image noise values, (C) SNR values, and (D) CNR value comparison between the LD and HD groups. LD stands for low-dose group and HD stands for high-definition group. SNR, signal-to-noise ratio; CNR, contrast-to-noise ratio; AO, aortic root; RCA, right coronary artery; LMA, left main artery; LAD, left anterior descending branch; LCX, left circumflex branch. p < 0.001 was a significant difference.
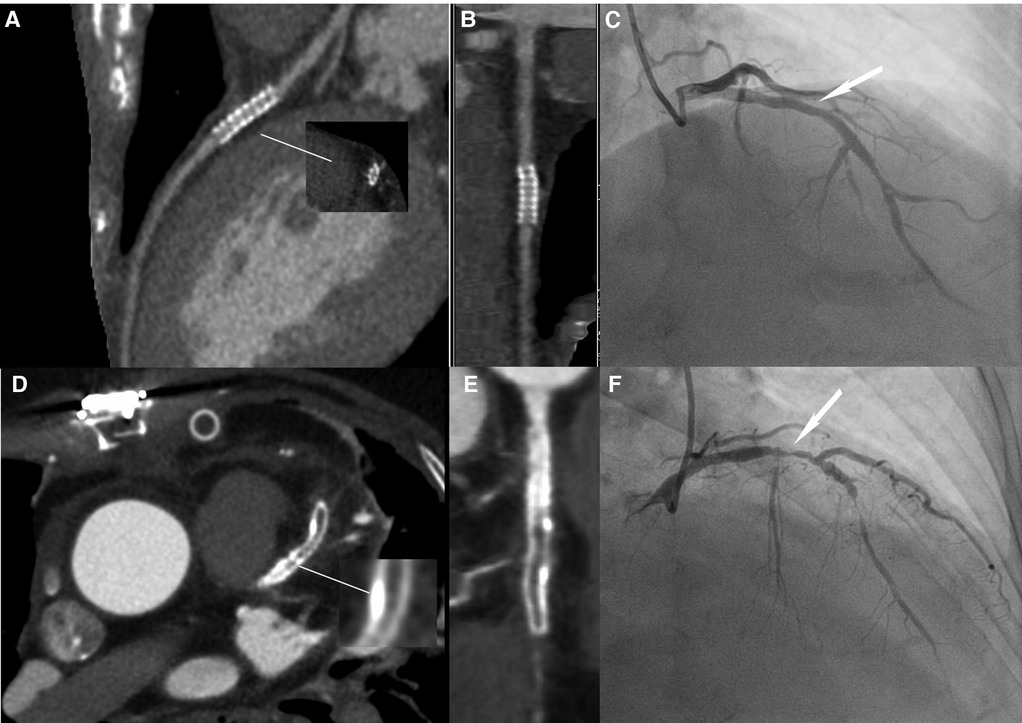
Figure 3 Image quality comparison between the LD and HD groups. (A–C) A 63-year-old male patient in the HD group, with a BMI of 24.53 kg/m2. (A) The image quality of CCTA reached a subjective score of 3: with minimal stent margin definition, some blooming artifacts, acceptable diagnostic information, and an image noise of 37.7 HU. (B) CPR image of LCX showed the absence of ISR in a 2.5 mm coronary stent (resolute, 2.5 × 21 mm, JHLD). (C) ICA of LCX confirmed the absence of ISR (white arrow). (D–F) A 61-year-old male patient in the LD group with a BMI of 24.06 kg/m2. (D) The image quality of CCTA reached a subjective score of 5: clear stent strut definition with a clear border of the blood vessel and an image noise of 16.7 HU. (E) CPR image of LAD showed focal ISR in a 3.0 mm coronary stent (resolute, 4.0 × 33 mm, RDES). (F) ICA of left anterior descending the diagnosis of focal ISR (white arrow). CCTA, coronary computed tomography angiography; CPR, curved planar reformation; ICA, invasive coronary angiography; ISR, in-stent restenosis; LCX, left circumflex; LAD, left anterior descending.
Diagnostic performance for ISR
There were 93 stents in 45 patients (2.07 per patient) for the SD group and 138 stents in 60 patients (2.30 per patient) for the LD group. The average stenosis values are listed in Table 4.
Compared with the SD group, the LD group had better diagnostic performance in both patient-level and stent-level analyses. Specifically, the accuracy of ISR diagnosis was 94.2% for the LD group and 83.8% for the SD group, and PPV of ISR diagnosis was 81.8% for the LD group and 62.5% for the SD group (all p < 0.05) (Table 5). There were 23 cases, 15 cases in the SD group (3 in RCA, 8 in LAD, and 4 in LCX) and 8 cases (1 in RCA, 5 in LAD, and 3 in LCX) in the LD group, in which stenoses were detected only at CCTA but not at ICA.
For ISR diagnostic performance on the large-caliber stents, the PPV of the LD group was nearly 10% higher than that of the SD group. For ISR diagnostic performance on the small-caliber stents, the PPV of the LD group was nearly 20% higher than that of the SD group (Table 5).
Discussion
In this study, by comparing the low-dose CCTA with DLIR and SSF2 with standard-dose CCTA with ASIR-V and SSF1, we demonstrated that the low-dose CCTA with DLIR and SSF2 could further improve the image quality and diagnostic performance for coronary ISR in reduced radiation dose and contrast medium dose conditions. As far as we know, this was the first clinical study to investigate the radiation dose and contrast dose saving abilities of DLIR in a Hi-Res model in combination with a motion correction algorithm.
Multiple CCTA studies have confirmed that images reconstructed with DLIR have better image quality than hybrid iterative reconstructions (16–18). Furthermore, some “double-low” studies have shown the ability of radiation dose and contrast dose saving for CCTA for patients without stents. In our study, we further extended the study scope by establishing a “double-low” CCTA protocol in combination with DLIR and SSF2 algorithms for patients after PCI. Our study proved that CCTA reconstructed with DLIR and SSF2 could reduce 39.1% radiation dose and 28% contrast dose while maintaining the image quality and diagnostic performance compared with CCTA with ASIR-V 50% and SSF (19, 20).
Moreover, we proved that the “double-low” CCTA with DLIR and SSF2 outperformed the standard-dose CCTA in the diagnosis of ISR with ICA as the reference (21). It is known that CCTA under high-resolution mode is suitable for patients with many calcified lesions or after PCI, which can effectively reduce the interference of metal artifacts and make the lumen of coronary stents more clearly displayed. However, the increase in image noise could make it more challenging to display the small intimal hyperplasia and stenosis in stents, especially in stents with a diameter of ≤3 mm (22), making the diagnosis potentially less accurate. Studies have utilized modified subtraction CCTA imaging techniques (23), iterative algorithms (24), or imaging markers [such as fractional flow reserve (25) and perivascular fat attenuation index (26)] to improve visualization of coronary ISR. In our study, we assessed the combination of the most advanced CT technologies for CCTA in patients with stents, including 16 cm wide-coverage detectors with 230 μm spatial resolution and high temporal resolution assisted by the new-generation motion correction algorithm SSF2 and DLIR. Eckert et al. (27) found that nearly two-thirds of symptomatic patients after PCI could rule out ISR by CCTA, with 99% NPV and 75% PPV. In our study, the NPV and PPV for the LD group were 100% and 94.4%, respectively. Li et al. (14) used the third-generation dual-source CT (SOMATOM Force) combined with low-dose imaging to accurately diagnose coronary ISR with large-bore (≥3 mm) and small-bore (<3 mm) stents. The diagnostic accuracy of ISR of small-bore and large-bore stents were 88.5% and 98.7% with 1.30 ± 0.72 mSv effective dose and 50 ml contrast media. In our study, the diagnostic accuracy of ISR of small-bore and large-bore stents were 87.7% and 98.7% with a reduced effective dose of 1.00 ± 0.64 mSv and contrast medium dose of 34.74 ± 5.77 ml in the LD group. Notably, the patient heart rate range during acquisition in the study of Li et al. was 63–83 bpm, while the heart rate of the patients in the LD group during acquisition in our study was 50–93 bpm. We suggest both the study of Li et al. (14) and our study have testified the clinical benefits of the new CT technologies in CCTA for patients with ISR and provided essential high-quality clinical information for follow-up after PCI, which is of great significance for improving curative effect and prognosis.
Previous studies have shown that CCTA was the first-line non-invasive imaging technique in patients with suspected coronary artery disease and ICA remained the standard reference method for the identification and characterization of coronary artery stenosis. When anatomical coronary narrowing >50% on ICA was considered a reference standard, CCTA had a specificity of 78%. CCTA with high-resolution CT could achieve a specificity of 88% in a per-vessel analysis compared with ICA in patients with high calcium scores provided promising preliminary data demonstrating a high diagnostic accuracy with a specificity of 88% in a per-vessel analysis compared with ICA (28, 29). In our study, in agreement with previous studies, the specificity values were 77.9% for the SD group and 94.2% for the LD group in a per-vessel analysis compared with ICA for patients after PCI. Our results were coordinated with the finding of the previous study that the high-resolution mode CCTA protocol could decrease the false-positive findings than the traditional mode CCTA (28). Furthermore, we have noticed that the diagnostic accuracy and PPV for ISR in the LD group were higher than those in the SD group, which proved that compared with the traditional iterative reconstruction in combination with SSF1, CCTA images of DLIR and SSF2 technologies had better diagnostic performance on ISR with better noise containment and motion correction capability. Specifically, the accuracy and PPV of ISR diagnosis were 10.4% and 19.3% higher for the LD group than those for the SD group. We further reviewed the misdiagnosed 23 cases, 15 (4 for the large-caliber stent subgroup and 11 for the small-caliber stent subgroup) were misdiagnosed in the SD group, and only 8 (1 for the large-caliber stent subgroup and 7 for the small-caliber stent subgroup) were misdiagnosed in the LD group. We noticed that the misdiagnosed rates of the SD group were higher than those of the LD group. We have found that all the misdiagnosed 23 cases were overestimation of luminal stenosis, which we speculate is the combination of heart motion, excessive calcium plaque load, small stent size, and particularly the still limited spatial resolution of the CT systems. However, the fact that the LD group had a much lower misdiagnosis rate and that the LD group with SSF2 provided much better performance on the visibility of luminal stenosis (Figures 4, 5) demonstrated the importance of motion correction in providing accurate IRS diagnosis for patients after PCI.
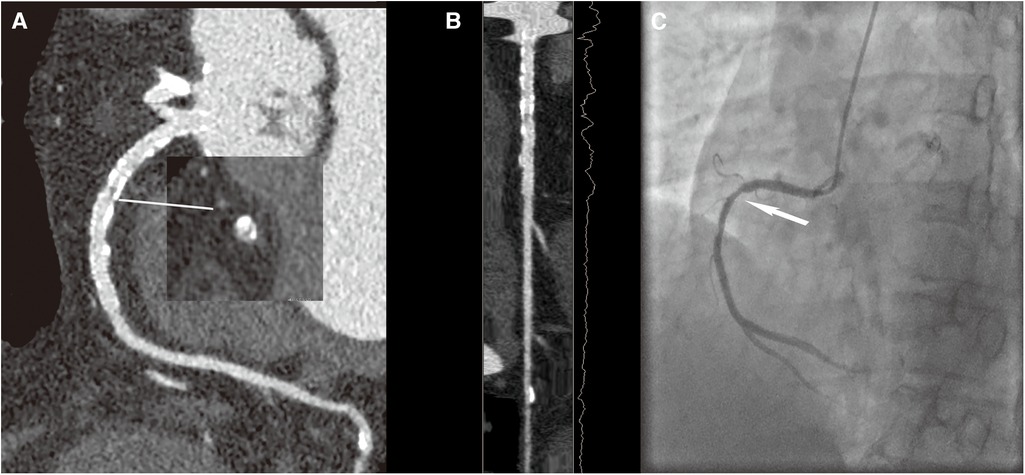
Figure 4 A 70-year-old female patient with ISR in the HD group, with a BMI of 26.4 kg/m2. (A) The image quality reached a subjective score of 4 with minimal stent margin definition, some blooming artifacts, acceptable diagnostic information, and an image noise of 35 HU. (B) CPR image of RCA showed focal ISR in a 3.0 mm coronary stent (resolute 3.0 × 33 mm, Firebird). (C) ICA of the right coronary artery confirmed the diagnosis of focal ISR (white arrow).
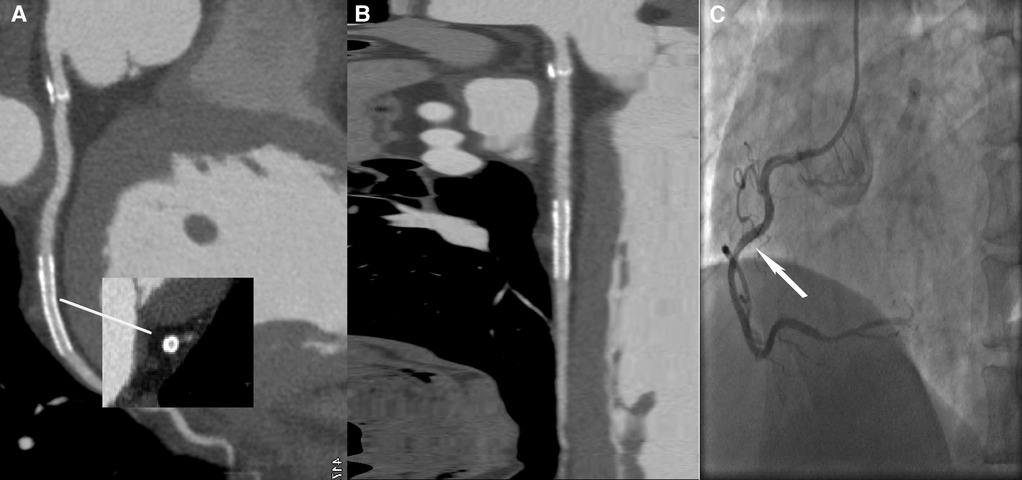
Figure 5 A 59-year-old male patient with ISR in the LD group, with a BMI of 25.56 kg/m2. (A) The image quality reached a subjective score of 5: clear stent strut definition with a clear border of the blood vessel and an image noise of 16 HU. (B) CPR image of LCX showed the absence of ISR in a 2.5 mm coronary stent (resolute, 2.5 × 22 mm, XIENCE Alpine). (C) ICA of the left circumflex confirmed the stent patency (white arrow).
This study has several limitations. First, all images were acquired on two CT scanners from the same vendor to minimize systemic errors. Therefore, the applicability of these findings to scans obtained in other types of scanners from different vendors may be limited. Second, we only compared ASIR-V 50% and DLIR-H in our study. We noticed that 50% was the most used iterative reconstruction algorithm and DLIR-H provided the highest potential on radiation dosage and contrast medium dosage reduction among the three levels of DLIR. In the future, we plan to perform further studies on the comprehensive comparison of all levels of ASIR-V and DLIR algorithms on patients after PCI. Finally, we noticed that all the misdiagnosed 23 cases had overestimation on the degree of luminal stenosis. Further study should establish subgroup analysis to find out the dominant factors for the overestimation.
Conclusion
In summary, as the more advanced reconstruction methods, DLIR and SSF2 could further significantly reduce image noise and cardiac motion artifacts, respectively, compared with ASIR-V and SSF1 reconstruction algorithms. The combination of DLIR and SSF2 in CCTA for patients with PCI could effectively reduce the radiation and contrast medium dose while improving the quality of CCTA images and providing high diagnostic efficiency for ISR.
Data availability statement
The data that support the findings of this study are available, requests to access the datasets should be directed to WW at 244171013@qq.com.
Ethics statement
The studies involving humans were approved by The First Affiliated Hospital of Zhengzhou University. The studies were conducted in accordance with the local legislation and institutional requirements. The participants provided their written informed consent to participate in this study. Written informed consent was obtained from the individual(s) for the publication of any potentially identifiable images or data included in this article.
Author contributions
WW: Writing – original draft. HZ: Data curation, Writing – review & editing. YW: Data curation, Writing – review & editing. XM: Writing – review & editing. JH: Data curation, Writing – review & editing. LR: Data curation, Writing – review & editing. JL: Data curation, Writing – review & editing. LW: Data curation, Writing – review & editing. YZ: Data curation, Writing – review & editing.
Funding
The authors declare financial support was received for the research, authorship, and/or publication of this article. This project is funded by study was supported by Key R&D projects in Henan Province (231111313100).
Conflict of interest
The authors declare that the research was conducted in the absence of any commercial or financial relationships that could be construed as a potential conflict of interest.
Publisher's note
All claims expressed in this article are solely those of the authors and do not necessarily represent those of their affiliated organizations, or those of the publisher, the editors and the reviewers. Any product that may be evaluated in this article, or claim that may be made by its manufacturer, is not guaranteed or endorsed by the publisher.
Abbreviations
CAD, cardiovascular diseases; PCI, percutaneous coronary intervention; ISR, in-stent restenosis; CCTA, coronary computed tomography angiography; HD, high definition; Hi-Res, high resolution; SSF2, SnapShot Freeze 2; DLIR, deep learning image reconstruction; ASIR-V, volume-based adaptive statistical iterative reconstruction; SSF1, SnapShot Freeze 1; CAG, coronary angiography; MPR, maximum intensity projection; VR, volume rendering; MIP, maximum intensity projection; CPR, curved planar reformat; ROI, region of interest; SD, standard dose; LD, low dose; LMA, left main artery; LAD, left ascending artery; LCX, left circumflex artery; RCA, right coronary artery; AO, aortic root; D1, diagonal branches; SNR, signal-to-noise ratio; CNR, contrast-to-noise ratio; PPV, positive predictive value; NPV, negative predictive value; CTDIvol, CT dose index; DLP, dose length product; ED, effective dose; BMI, body mass index.
References
1. Benjamin EJ, Blaha MJ, Chiuve SE, Cushman M, Das SR, Deo R, et al. Heart disease and stroke statistics-2017 update: a report from the American Heart Association. Circulation. (2017) 135(10):e146–603. doi: 10.1161/CIR.0000000000000485
2. Moussa ID, Mohananey D, Saucedo J, Stone GW, Yeh RW, Kennedy KF, et al. Trends and outcomes of restenosis after coronary stent implantation in the United States. J Am Coll Cardiol. (2020) 76(13):1521–31. doi: 10.1016/j.jacc.2020.08.002
3. Shlofmitz E, Iantorno M, Waksman R. Restenosis of drug-eluting stents: a new classification system based on disease mechanism to guide treatment and state-of-the-art review. Circ Cardiovasc Interv. (2019) 12(8):e007023. doi: 10.1161/CIRCINTERVENTIONS.118.007023
4. Katz G, Harchandani B, Shah B. Drug-eluting stents: the past, present, and future. Curr Atheroscler Rep. (2015) 17(3):485. doi: 10.1007/s11883-014-0485-2
5. Tesche C, De Cecco CN, Vliegenthart R, Duguay TM, Stubenrauch AC, Rosenberg RD, et al. Coronary CT angiography-derived quantitative markers for predicting in-stent restenosis. J Cardiovasc Comput Tomogr. (2016) 10(5):377–83. doi: 10.1016/j.jcct.2016.07.005
6. van Driest FY, Bijns CM, van der Geest RJ, Broersen A, Dijkstra J, Scholte A, et al. Utilizing (serial) coronary computed tomography angiography (CCTA) to predict plaque progression and major adverse cardiac events (MACE): results, merits and challenges. Eur Radiol. (2022) 32(5):3408–22. doi: 10.1007/s00330-021-08393-9
7. Benz DC, Fuchs TA, Gräni C, Studer Bruengger AA, Clerc OF, Mikulicic F, et al. Head-to-head comparison of adaptive statistical and model-based iterative reconstruction algorithms for submillisievert coronary CT angiography. Eur Heart J Cardiovasc Imaging. (2018) 19(2):193–8. doi: 10.1093/ehjci/jex008
8. Chen Y, Liu Z, Li M, Yu Y, Jia Y, Ma G, et al. Reducing both radiation and contrast doses in coronary CT angiography in lean patients on a 16-cm wide-detector CT using 70 kVp and ASiR-V algorithm, in comparison with the conventional 100-kVp protocol. Eur Radiol. (2019) 29(6):3036–43. doi: 10.1007/s00330-018-5837-9
9. Chartrand G, Cheng PM, Vorontsov E, Drozdzal M, Turcotte S, Pal CJ, et al. Deep learning: a primer for radiologists. Radiographics. (2017) 37(7):2113–31. doi: 10.1148/rg.2017170077
10. Greffier J, Hamard A, Pereira F, Barrau C, Pasquier H, Beregi JP, et al. Image quality and dose reduction opportunity of deep learning image reconstruction algorithm for CT: a phantom study. Eur Radiol. (2020) 30(7):3951–9. doi: 10.1007/s00330-020-06724-w
11. Jiang B, Li N, Shi X, Zhang S, Li J, de Bock GH, et al. Deep learning reconstruction shows better lung nodule detection for ultra-low-dose chest CT. Radiology. (2022) 303(1):202–12. doi: 10.1148/radiol.210551
12. Li W, Diao K, Wen Y, Shuai T, You Y, Zhao J, et al. High-strength deep learning image reconstruction in coronary CT angiography at 70-kVp tube voltage significantly improves image quality and reduces both radiation and contrast doses. Eur Radiol. (2022) 32(5):2912–20. doi: 10.1007/s00330-021-08424-5
13. Wang H, Wang R, Li Y, Zhou Z, Gao Y, Bo K, et al. Assessment of image quality of coronary computed tomography angiography in obese patients by comparing deep learning image reconstruction with adaptive statistical iterative reconstruction Veo. J Comput Assist Tomogr. (2022) 46(1):34–40. doi: 10.1097/RCT.0000000000001252
14. Li Y, Yu M, Li W, Lu Z, Wei M, Zhang J. Third generation dual-source CT enables accurate diagnosis of coronary restenosis in all size stents with low radiation dose and preserved image quality. Eur Radiol. (2018) 28(6):2647–54. doi: 10.1007/s00330-017-5256-3
15. Wang M, Fan J, Shi X, Qin L, Yan F, Yang W. A deep-learning reconstruction algorithm that improves the image quality of low-tube-voltage coronary CT angiography. Eur J Radiol. (2022) 146:110070. doi: 10.1016/j.ejrad.2021.110070
16. Higaki T, Nakamura Y, Zhou J, Yu Z, Nemoto T, Tatsugami F, et al. Deep learning reconstruction at CT: phantom study of the image characteristics. Acad Radiol. (2020) 27(1):82–7. doi: 10.1016/j.acra.2019.09.008
17. Tatsugami F, Higaki T, Nakamura Y, Yu Z, Zhou J, Lu Y, et al. Deep learning-based image restoration algorithm for coronary CT angiography. Eur Radiol. (2019) 29(10):5322–9. doi: 10.1007/s00330-019-06183-y
18. Akagi M, Nakamura Y, Higaki T, Narita K, Honda Y, Zhou J, et al. Deep learning reconstruction improves image quality of abdominal ultra-high-resolution CT. Eur Radiol. (2019) 29(11):6163–71. doi: 10.1007/s00330-019-06170-3
19. Benz DC, Benetos G, Rampidis G, von Felten E, Bakula A, Sustar A, et al. Validation of deep-learning image reconstruction for coronary computed tomography angiography: impact on noise, image quality and diagnostic accuracy. J Cardiovasc Comput Tomogr. (2020) 14(5):444–51. doi: 10.1016/j.jcct.2020.01.002
20. Jensen CT, Liu X, Tamm EP, Chandler AG, Sun J, Morani AC, et al. Image quality assessment of abdominal CT by use of new deep learning image reconstruction: initial experience. AJR Am J Roentgenol. (2020) 215(1):50–7. doi: 10.2214/AJR.19.22332
21. Sun J, Li H, Li J, Cao Y, Zhou Z, Li M, et al. Performance evaluation of using shorter contrast injection and 70 kVp with deep learning image reconstruction for reduced contrast medium dose and radiation dose in coronary CT angiography for children: a pilot study. Quant Imaging Med Surg. (2021) 11(9):4162–71. doi: 10.21037/qims-20-1159
22. Byrne RA, Joner M, Kastrati A. Stent thrombosis and restenosis: what have we learned and where are we going? The Andreas Grüntzig Lecture ESC 2014. Eur Heart J. (2015) 36(47):3320–31. doi: 10.1093/eurheartj/ehv511
23. Li J, Guo MT, Yang X, Gao F, Li N, Huang MG. The usefulness of subtraction coronary computed tomography angiography for in-stent restenosis assessment of patients with CoCr stent using 320-row area detector CT. Medicine (Baltimore). (2021) 100(51):e28345. doi: 10.1097/MD.0000000000028345
24. Andreini D, Pontone G, Mushtaq S, Conte E, Guglielmo M, Mancini ME, et al. Diagnostic accuracy of coronary CT angiography performed in 100 consecutive patients with coronary stents using a whole-organ high-definition CT scanner. Int J Cardiol. (2019) 274:382–7. doi: 10.1016/j.ijcard.2018.09.010
25. Tang CX, Guo BJ, Schoepf JU, Bayer RR 2nd, Liu CY, Qiao HY, et al. Feasibility and prognostic role of machine learning-based FFR(CT) in patients with stent implantation. Eur Radiol. (2021) 31(9):6592–604. doi: 10.1007/s00330-021-07922-w
26. Qin B, Li Z, Zhou H, Liu Y, Wu H, Wang Z. The predictive value of the perivascular adipose tissue CT fat attenuation index for coronary in-stent restenosis. Front Cardiovasc Med. (2022) 9:822308. doi: 10.3389/fcvm.2022.822308
27. Eckert J, Renczes-Janetzko P, Schmidt M, Magedanz A, Voigtländer T, Schmermund A. Coronary CT angiography (CCTA) using third-generation dual-source CT for ruling out in-stent restenosis. Clin Res Cardiol. (2019) 108(4):402–10. doi: 10.1007/s00392-018-1369-1
28. Serruys PW, Kotoku N, Nørgaard BL, Garg S, Nieman K, Dweck MR, et al. Computed tomographic angiography in coronary artery disease. EuroIntervention. (2023) 18:e1307–27. doi: 10.4244/EIJ-D-22-00776
Keywords: coronary CT angiography, percutaneous coronary intervention, in-stent restenosis, deep learning image reconstruction algorithm, SnapShot Freeze 2
Citation: Wu W, Zhan H, Wang Y, Ma X, Hou J, Ren L, Liu J, Wang L and Zhang Y (2024) Improving image quality and in-stent restenosis diagnosis with high-resolution “double-low” coronary CT angiography in patients after percutaneous coronary intervention. Front. Cardiovasc. Med. 11: 1330824. doi: 10.3389/fcvm.2024.1330824
Received: 31 October 2023; Accepted: 20 June 2024;
Published: 23 July 2024.
Edited by:
Edoardo Conte, University of Milan, ItalyReviewed by:
Georgios Benetos, National and Kapodistrian University of Athens, GreeceMarta Belmonte, OLV Aalst, Belgium
© 2024 Wu, Zhan, Wang, Ma, Hou, Ren, Liu, Wang and Zhang. This is an open-access article distributed under the terms of the Creative Commons Attribution License (CC BY). The use, distribution or reproduction in other forums is permitted, provided the original author(s) and the copyright owner(s) are credited and that the original publication in this journal is cited, in accordance with accepted academic practice. No use, distribution or reproduction is permitted which does not comply with these terms.
*Correspondence: Yonggao Zhang, zyg015780@126.com