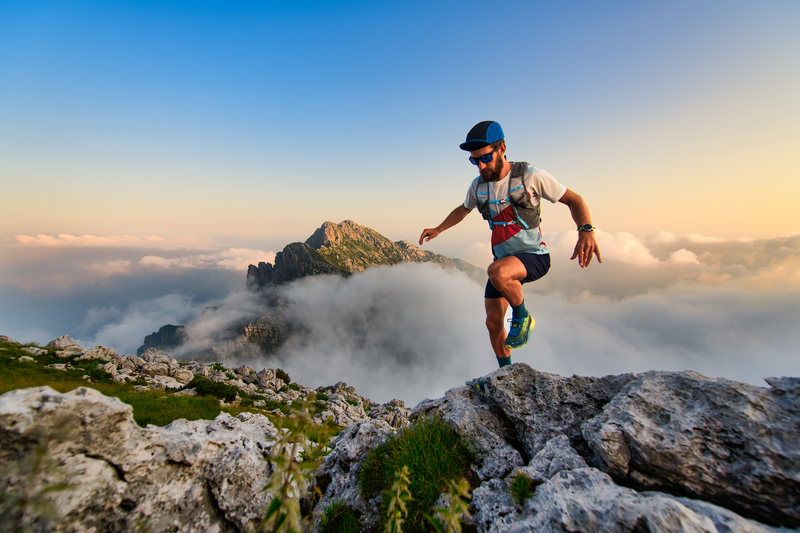
94% of researchers rate our articles as excellent or good
Learn more about the work of our research integrity team to safeguard the quality of each article we publish.
Find out more
REVIEW article
Front. Cardiovasc. Med. , 12 April 2024
Sec. Cardioneurology
Volume 11 - 2024 | https://doi.org/10.3389/fcvm.2024.1265089
Deep brain stimulation (DBS) is an interdisciplinary and reversible therapy that uses high-frequency electrical stimulation to correct aberrant neural pathways in motor and cognitive neurological disorders. However, the high frequency of the waves used in DBS can interfere with electrical recording devices (e.g., electrocardiogram, electroencephalogram, cardiac monitor), creating artifacts that hinder their interpretation. The compatibility of DBS with these devices varies and depends on factors such as the underlying disease and the configuration of the neurostimulator. In emergencies where obtaining an electrocardiogram is crucial, the need for more consensus on reducing electrical artifacts in patients with DBS becomes a significant challenge. Various strategies have been proposed to attenuate the artifact generated by DBS, such as changing the DBS configuration from monopolar to bipolar, temporarily deactivating DBS during electrocardiographic recording, applying frequency filters both lower and higher than those used by DBS, and using non-standard leads. However, the inexperience of medical personnel, variability in DBS models, or the lack of a controller at the time of approach limit the application of these strategies. Current evidence on their reproducibility and efficacy is limited. Due to the growing elderly population and the rising utilization of DBS, it is imperative to create electrocardiographic methods that are easily accessible and reproducible for general physicians and emergency services.
Deep brain stimulation (DBS) is an interdisciplinary and reversible therapy that delivers high-frequency electrical stimulation to specific brain sites through implanted electrodes to correct aberrant neural pathways underlying motor and cognitive neurological disorders (1).
Although initially used for the treatment of essential tremor and Parkinson's disease (PD), the use of DBS has expanded to the treatment of other refractory movement disorders (dystonia, tics) (2–4), psychiatric disorders (major depressive disorder, obsessive-compulsive disorder, addictions) (5–7), and more recently, other neurodegenerative diseases such as Alzheimer's disease (8).
The high-frequency waves used in neurostimulation range from 100 to 200 Hz, which can interfere with electrical recording devices (e.g., electrocardiogram, electroencephalogram, cardiac monitors), creating artifacts that limit or impede their interpretation. The variability in compatibility between DBS and these devices depends on both modifiable and non-modifiable factors of both systems (9, 10). However, there is no consensus on strategies to reduce the electrical artifacts created by DBS in patients with movement disorders, which becomes particularly relevant in emergencies where the acquisition of an electrocardiogram (ECG) is imperative.
An increasingly aging population, the growing number of people with movement disorders, and the increased use of DBS will inevitably lead primary care physicians to encounter patients with some form of neurostimulation. Given the lack of knowledge about DBS among the non-specialized medical community and the absence of protocols for its electrocardiographic approach, this review provides a compilation of its functioning, the electrocardiographic artifacts it produces, and the existing strategies to reduce them.
Neurodegenerative diseases are the leading cause of disability-adjusted life years and the second leading cause of mortality worldwide (11, 12). PD is the second most common neurodegenerative disease globally, second only to Alzheimer's disease, affecting 2%–3% of the population over 65 years old (13). The worldwide prevalence of PD is estimated to be between 100 and 300 per 100,000 individuals (14), and in Mexico, this disease affects 1.6%–2.3% of the population over 65 years old, a figure similar to that reported in the rest of Latin America (15). The incidence of PD has dramatically increased in the past three decades (16, 17). From 1990 to 2016, the number of people worldwide with PD doubled to 6 million, a number projected to double again by the year 2040 (17, 18), making PD the fastest-growing neurological disorder in the world (16, 19), a phenomenon that some authors have referred to as the Parkinson's pandemic. Although the cause of PD cannot be attributed in 90% of cases (20), increased longevity in the population and expanded diagnostic strategies partially explain this growth phenomenon. Genetic predisposition and environmental exposure contribute significantly to the increasing global incidence (21), estimated to be 108–202 per 100,000 individuals over 65 years old in the United States (22) and approximately 10.2 per 100,000 individuals in Mexico (23). Incidence and prevalence are 1.5–2 times higher in men (14).
Only 2% of eligible PD patients are estimated to receive DBS treatment (24, 25), indicating that DBS is an underutilized therapeutic option.
National registries on DBS placement are limited; however, as of 2021, it was estimated that over 200,000 patients with movement disorders had been treated with DBS devices worldwide, which continues to increase each year (26).
Cardiovascular diseases represent one of the leading causes of morbidity and mortality in patients with PD (27–29). The association between PD and acute myocardial infarction has been inconsistent, with some studies demonstrating that individuals with PD have a significantly higher risk of developing acute myocardial infarction than the general population (30–33). In contrast, others have not reported significant differences (34). Both conditions share pathophysiological processes and an age-related increase in incidence (31, 33). On the other hand, some cardiovascular risk factors have even been identified as protective against developing PD (35–38).
Other diseases, such as heart failure, autonomic dysfunction, arrhythmias, and conduction disorders, are particularly prevalent in individuals with PD (39–44). Some reports have linked PD to prolonged QT interval and the development of malignant arrhythmias, likely secondary to autonomic dysfunction and pharmacological treatment, closely related to the risk of sudden death, estimated to occur in 3%–4% of PD patients (45–48).
Diabetes mellitus and systemic arterial hypertension are particularly prevalent comorbidities in individuals with PD (30, 49, 50). Studies conducted in Mexico highlight a high prevalence of cardiometabolic diseases and elevated cardiovascular risk among patients with PD (51, 52). Furthermore, individuals with diabetes mellitus have up to a 23% higher likelihood of developing PD (53).
Cardiometabolic diseases prevail among individuals with movement disorders receiving DBS treatment (54). Studies that have retrospectively analyzed the main reasons for emergency department visits in DBS patients have focused solely on those related to the neurostimulation device itself (55–57). However, it has been recognized that age and comorbidities, particularly coronary artery disease, directly influence readmission rates and hospitalization time in these patients (58, 59). The mortality rate in PD patients treated with DBS is 8.2%–21.4% at five years (60–62) and 23%–30.4% at ten years (54, 62), with acute myocardial infarction being the second or third leading cause of death in these patients (54, 60, 63).
Neurostimulation is the nervous system's electrical, selective, and reversible modulation through invasive and noninvasive techniques. There are different types of neurostimulation based on the neuroanatomical area they modulate. DBS is characterized by delivering electrical stimuli to the basal ganglia. These stimuli are generated by an implantable pulse generator (IPG) and delivered through extension wires connected to electrodes (64). Most IPGs have a lithium battery with a lifespan of 7–10 years. However, recently rechargeable systems and even those powered by sources such as thoracic movement during the respiratory cycle have been implemented (65). These devices are coated with titanium and are subcutaneously implanted, usually in the infraclavicular area. The placement of electrodes in the exact neuroanatomical area is done through stereotactic surgery, and each electrode is constructed of platinum-iridium, which provides excellent conductivity and minimal toxicity (66).
On the other hand, the extension wires are subcutaneously placed in the lateral portion of the neck and are made of a nickel alloy covered with a polyurethane sheath (66). At their ends, each electrode has one or more contacts from which the impulses emerge outward, and the spatial arrangement of these contacts determines the shape and extent of the generated electric field (66, 67). The electrical impulses are short (60–450 μs), have a frequency ranging from 100 to 200 Hz, and amplitude between 2.0–5.0 mV (67, 68), parameters comparable to those used in conventional pacemakers. DBS placement is generally bilateral, although, in some patients, unilateral neurostimulation is sufficient (67, 68).
DBS models exhibit heterogeneity, yet they share common characteristics that allow for modification in configuration or deactivation through an external controller. The two principal configurations of neurostimulation, monopolar and bipolar, govern the flow of electrical current within the implanted electrode and the implanted pulse generator (IPG). In the monopolar configuration, the current emanates from one or several contacts of the implanted electrode (cathode) to the IPG (anode), generating a broader electromagnetic field (EF). In contrast, bipolar stimulation involves the simultaneous activation of two electrode contacts, with one serving as the anode and the other as the cathode. This configuration confines the electrical current to a more localized area.
The distinction between bipolar and monopolar configurations extends to their impact on the Volume of Tissue Activated (VTA). Computational modeling indicates distinct spatial distributions of stimulation effects. Monopolar configurations tend to produce a more extensive VTA, whereas bipolar configurations, activating two electrode contacts concurrently, result in a more focused and intricate pattern of neural modulation.
Furthermore, it is important to note that DBS leads can function interchangeably as cathodes or anodes, providing versatility in the modulation of neural activity. This flexibility extends to the choice between voltage-based and current-based stimulation. In voltage-based stimulation, the device sets a specific power level, allowing for adaptability but requiring careful consideration of individual variability. On the other hand, current-based stimulation maintains a consistent current output, ensuring a more predictable and controlled delivery of electrical stimulation (69). The versatile selection in stimulation configuration enriches the adaptability of DBS interventions, enabling tailored approaches that align with patient-specific needs and response profiles. This flexibility empowers customized strategies in DBS interventions, catering to the unique requirements and responses of individual patients (69–72).
The effects of DBS are immediate, reversible, and pleiotropic, as they depend on factors specific to the electrical system of the IPG and the intrinsic characteristics of the stimulated tissue (e.g., types of ion channels, myelin content, orientation of nerve fibers), which means that the effects on one specific brain area may not apply to another (1). Although the exact DBS mechanism of action remains unclear, in the context of PD various theories have been proposed, including the prevention and modification of abnormal neural pathways' propagation, neurochemical modification of synapses, and the neuronal microenvironment (1, 73–77). The beneficial effect of DBS is likely a combination of several elements.
The control of voluntary movements is a process that occurs at cortical and subcortical levels, where the basal ganglia play an essential role in the initiation and cognitive modulation, dependent on the balance between glutamatergic excitatory pathways and GABAergic inhibitory pathways (78–82). This balance is disrupted in movement disorders. In PD, the loss of dopamine in the substantia nigra to the striatum causes overactivation of the direct excitatory pathway through the internal globus pallidus and inhibition of the indirect path through the external globus pallidus and the subthalamic nucleus, resulting in a more significant inhibition of the thalamus and its cortical inputs, leading to the akinesia and rigidity characteristic of PD (67, 82–84). DBS therapy aims to silence the pathologically hyperactive pathways through internal globus pallidus or subthalamic nucleus stimulation (68). Different therapeutic targets are preferred for treating other movement disorders, such as the ventral intermediate nucleus of the thalamus for essential tremor, the most common movement disorder in the world (84).
Although initially conceived as a panacea for movement disorders, the most significant benefit of DBS appears reserved for individuals with a specific phenotype of PD (Table 1). While there is no defined age range, DBS is recommended for patients younger than 70 years (85–87). Generally, monopolar stimulation is preferred over bipolar stimulation, as it requires a lower stimulation intensity to achieve the same clinical effects. This is often attributed to the more diffuse electric field in monopolar setups. The broader distribution of current allows for a greater volume of neural tissue to be influenced, requiring less current density at any given point to produce the desired effects (66). Nevertheless, the choice between monopolar and bipolar stimulation isn't solely determined by the required stimulation intensity. Side-effect thresholds, referring to the point at which unwanted effects or discomfort occur, differ between the two montages. While the more focused electric field in bipolar setups may have a higher risk of localized side effects due to the higher current density, this could also mean a more precise and controlled modulation of the desired area (66).
Table 1. Indications and contraindications for deep brain stimulation (DBS) placement in patients with Parkinson's disease (PD) (64, 86).
The presence of a cardiac pacemaker had previously been considered a contraindication for DBS implantation (85, 88). However, some case series have reported that the coexistence of both devices in the same patient does not pose a greater risk, with the bipolar configuration being preferred over the monopolar to avoid interference (88, 89). The main adverse effects of DBS include dysarthria, balance impairment, visual disturbances, tonic contractions, and behavioral changes such as mania, apathy, and severe depression (67, 90). Considering these adverse effects, the variability in response, and the inherent risks of surgical intervention, DBS is reserved for patients with a specific profile of PD.
The VTA and the extent of the EF generated during neurostimulation are crucial when considering DBS as a potential artifact for other electrical recording devices. In the monopolar configuration, the EF generated spans an area from the implanted electrode to the IPG, reaching the precordial region, creating electrical artifacts and interference with other stimulation and recording devices located in the same anatomical area (e.g., pacemakers, ECG, cardiac monitors) (9, 10) (Figure 1).
Figure 1. Left image: in the monopolar configuration, the generated electric field exhibits a greater extension and reaches the precordium, overlapping with the recording area of the electrocardiogram (arrangement of leads I, II, and III is represented by Einthoven's triangle). Right image: The electrode generates an eccentric electric field, from the cathode to the anode.
It should be noted that vectors from the electrical artifact resulting from monopolar stimulation can be observed continuously throughout the body's electric field. This is consistent with the more diffuse electric field generated by monopolar setups, where the current flows from a single electrode to a reference point. Moreover, the generation of an observable artifact by monopolar stimulation can be attributed to the distinct voltage decay characteristics between monopolar and bipolar configurations (91). Maxwell's equations describe the fundamental principles governing electromagnetic fields, providing insights into how electric fields propagate and decay in different configurations (92). However, in a bipolar configuration, the EF is more circumscribed and rarely reaches the precordial area, generating significantly fewer artifacts and interference (93) (Figure 2).
Figure 2. Left image: in the bipolar configuration, the electric field is confined to a more circumscribed area and rarely reaches the precordium. Right image: There is simultaneous activation of two electrode contacts, with one serving as an anode and the other as a cathode.
Artifact resulting from a bipolar montage typically resides below the noise floor in peripheral recording electrodes, such as EKG leads. This implies that the artifact generated by bipolar stimulation is less prominent or more challenging to detect in recordings from peripheral electrodes. The specific spatial characteristics of the bipolar configuration might contribute to a reduced impact on distant recording sites (94).
As mentioned before, Maxwell's equations provide a mathematical description of how the electric field produced by different electrode configurations (e.g., monopolar, or bipolar) will propagate through the body but also can be applied to analyze the voltage decay (how the electric field strength diminishes with distance from the stimulation source). This provides a theoretical foundation for understanding the generation, propagation, and decay of electric fields, and their principles are essential for comprehending the biophysics of electrical stimulation and the resulting artifacts in the body's electric field. Applying these principles allows researchers and clinicians to optimize stimulation parameters and electrode configurations for specific applications.
Other factors directly influencing the quality of recordings are the stimulation voltage and the underlying disease (93). The infraclavicular position, whether left or right, does not show differences in artifact generation (93).
Under standard settings, modern electrocardiograms can record frequencies between 0.05–150 Hz, including the impulses generated by DBS (100–200 Hz). Capturing these frequencies during an ECG or long-term recordings (e.g., Holter monitor) can generate artifacts during the recording, mimicking abnormal rhythms (e.g., atrial fibrillation, atrial flutter) and even making interpretation impossible (9, 68, 93–98) (Figure 3). This problem becomes particularly relevant in a cardiovascular emergency, where obtaining an ECG is imperative.
Figure 3. Twelve-lead electrocardiograms in a patient with deep brain stimulation (DBS) in monopolar configuration at 159 Hz: Top left image: monopolar at 100 Hz, an artifact affecting all leads is observable, with V2-V4 showing more pronounced effects. Top right image: monopolar with a 150 Hz filter, as the filter is close to the DBS stimulation frequency, the artifact becomes markedly more conspicuous. Bottom left image: Monopolar with a 20 Hz filter, significant reduction of artifact is noted, albeit slightly discernible in V2 and V3. Bottom right image: Switch to the bipolar configuration at 100 Hz, complete disappearance of the DBS artifact is achieved, rendering the electrocardiogram indistinguishable from that of an individual without DBS. ECG: Electrocardiogram, DBS: Deep Brain Stimulation.
Multiple authors have emphasized the importance of enhancing the medical community's knowledge in managing DBS, addressing the challenges involved in its handling, and establishing a protocol for electrocardiographic approaches (10, 93, 99, 100).
Several studies and case reports have demonstrated the effectiveness of various electrocardiographic strategies to improve the quality of electrocardiographic recordings in the presence of artifacts caused by deep brain stimulation (DBS).
I. Changing the DBS configuration
For the reasons described above, switching the device to a bipolar configuration significantly reduces the artifact produced by monopolar DBS, allowing for interpretable electrocardiographic recordings in most cases (68, 93).
II. Temporarily deactivating DBS during electrocardiographic recording
Temporary deactivation of DBS is the definitive intervention to eliminate the electrocardiographic artifact. This approach can be done using the device controller or by placing a magnet over the generator pulse implant for <5 s. Deactivating one of the devices can significantly attenuate the artifact when the patient has bilateral stimulation (68). However, this strategy does not appear to be superior to changing the configuration, as total deactivation, albeit momentary, results in the return of abnormal movements, leading to motion artifacts in the ECG. The resting tremor frequency in Parkinson's disease is 4–6 Hz (100), which can mimic abnormal rhythms and limit interpretability (10, 101–103.) In a study of 100 patients with Parkinson's disease, Hwang et al. described the presence of various electrocardiographic artifacts, with 78% showing baseline variations and 11% exhibiting abnormal rhythms similar to atrial flutter/fibrillation (104). Different techniques have been described to attenuate this artifact in patients with movement disorders, such as electrode placement on limb roots (99). However, further studies are needed to evaluate their effectiveness during electrocardiographic recording in patients with DBS.
III. Application of frequency filters
EKG machines incorporate robust filtering mechanisms to isolate and record the electrical activity of the heart. These filters typically include low-pass filters to eliminate high-frequency noise and high-pass filters to attenuate low-frequency interference. Traditional EKG devices allow operators to manually adjust filters based on specific needs. However, it's important to note that some EKG models have fixed filters that cannot be manually changed. The unique challenges posed by DBS artifacts require a specialized approach beyond standard recommendations by organizations like the American Heart Association (AHA) (105). Recent studies highlight effective cutoff frequencies for DBS artifact reduction. For instance, implementing a low-frequency cutoff filter around 40 Hz has proven effective in mitigating artifacts related to the average frequency of DBS pulses (100–200 Hz) (68). Additionally, applying filters above the DBS pulse range (300–512 Hz) has shown significant artifact reduction (98) (Figure 4). A cutoff filter that attenuates artifacts from abnormal movements has not been determined.
IV. Use of non-standard leads for electrocardiographic recording
Figure 4. Comparative analysis of suggested filters and filters standardized by the American heart association (AHA) (105) in a bode diagram on their effect on the detection of heart electrical activity concerning the artifact generated by the standard stimulation frequency used in DBS treatment for Parkinson's disease (100-200 Hz).
Using alternative leads to the classic 12 leads described by Wilson can help capture cardiac vectors without overlapping with the EF of monopolar DBS configuration. Mruk et al. demonstrated that using Nehb-Spöri leads (Figure 5) significantly reduces the artifact caused by the return of abnormal movements after deactivating monopolar DBS (94), likely attributed to the exclusion of peripheral leads, which are more susceptible to motion artifacts. A reported case by Steltzer et al. described a significant reduction of DBS artifacts when using devices with less than 12 leads (KardiaMobile 6l, Apple Watch) (98). It is possible that other non-standard leads (e.g., right-sided, posterior, Lewis, Medrano leads) could improve the quality of electrocardiographic recordings in these patients.
Figure 5. Nehb-Spöri leads, with limb leads (R.A., LA, and L.L.) placed on the right parasternal line at the level of the second intercostal space (A), left axillary posterior line at the level of the scapular apex (D), and left midclavicular line at the fifth intercostal space (J), respectively, thus reducing artifact caused by distal movement in Parkinson's disease after deactivation of deep brain stimulation (DBS). Parasternal Line (PSL), intercostal space (ICS), Left Axillary Posterior line (LAP), Left midclavicular line (LMC).
Although effective, these approaches are not always reproducible. Changing the polarity and deactivating DBS are strategies that require medical personnel who are familiar with manipulating neurostimulation devices. Modifying the neurostimulator is not applicable in all contexts, whether due to lack of experience or unavailability of the controller at the time of medical intervention. Variability among DBS models can affect the operator's ability to deactivate or modify the device's configuration.
While the application of frequency filters to eliminate artifacts induced by DBS has demonstrated effectiveness in specific case reports, there is a dearth of evidence comparing it to alternative strategies, and its efficacy compared to different DBS configurations still needs to be verified. Furthermore, the ability to manually manipulate filters in traditional EKG equipment confers a level of adaptability that proves invaluable in the landscape of electrocardiographic approach in DBS patients. In contrast, EKG models with fixed filters mandate adherence to predetermined settings, emphasizing the critical importance of judicious equipment selection for DBS studies. Filtering capabilities wield direct influence over the accuracy with which neural signals are captured and analyzed.
While electrocardiographic recordings with non-standard leads provide an alternative perspective to the classic 12 leads, they limit the comprehensive electrocardiographic analysis of the heart and the application of standardized measures for detecting atrial and ventricular enlargements.
Some case reports have highlighted the challenges in managing DBS devices during cardiovascular emergencies, where obtaining an electrocardiogram is imperative, leading to delays in diagnosis and treatment (93, 99).
It is generally recommended that patients undergoing DBS treatment have an electrocardiogram and cardiovascular assessment as future reference (89).
Portable cardiac monitoring devices (e.g., smartwatches) can identify abnormal rhythms and perform single-lead electrocardiographic recordings, which have shown effectiveness in patients with DBS (96). These observations encourage the implementation of such devices in patients receiving neurostimulation.
Although a cutoff filter to reduce abnormal movement artifacts has not been determined, recent technological advances have enabled the development of signal-filtering techniques for the electrical signal of the heart. These techniques have successfully reduced various types of noise in electrocardiograms, such as electromyographic noise. This progress allows for the acquisition of cleaner and clearer electrocardiographic signals, facilitating their accurate interpretation (106).
Recently, programming modalities for DBS have been investigated to allow neurologists or neurosurgeons to make remote and real-time adjustments to the neurostimulator's configuration, enabling necessary modifications when electrocardiographic assessment is required (105, 107). Additionally, some designs of electrodes with multiple contacts have demonstrated improved control over the VTA and generated EF New designs of miniature DBS generators would allow placement in the patient's skull, limiting EF projection towards the precordium during monopolar DBS activation (108, 109).
Current strategies, although effective, are not replicable in all contexts. This issue, raised in an increasingly aging society where DBS is a widely used therapy, calls for electrocardiographic approach strategies in these patients that are affordable and reproducible for primary care physicians and emergency services.
CS-F: Funding acquisition, Investigation, Project administration, Supervision, Visualization, Writing – original draft, Writing – review & editing. LG-G: Funding acquisition, Investigation, Project administration, Supervision, Visualization, Writing – original draft, Writing – review & editing. AH-D: Funding acquisition, Investigation, Project administration, Supervision, Visualization, Writing – original draft, Writing – review & editing. JO-H: Writing – review & editing. MR-M: Formal Analysis, Writing – review & editing. AC-A: Formal Analysis, Writing – review & editing. AH-M: Formal Analysis, Writing – review & editing. MR-V: Formal Analysis, Validation, Writing – review & editing.
The author(s) declare financial support was received for the research, authorship, and/or publication of this article.
Ignacio Chávez National Institute of Cardiology supported open access funding for this article.
All the authors are supported by the National Institute of Cardiology Ignacio Chavez and the National Institute of Neurology Manuel Velasco Suarez.
The authors declare that the research was conducted in the absence of any commercial or financial relationships that could be construed as a potential conflict of interest.
The author(s) declared that they were an editorial board member of Frontiers, at the time of submission. This had no impact on the peer review process and the final decision.
All claims expressed in this article are solely those of the authors and do not necessarily represent those of their affiliated organizations, or those of the publisher, the editors and the reviewers. Any product that may be evaluated in this article, or claim that may be made by its manufacturer, is not guaranteed or endorsed by the publisher.
1. Jakobs M, Fomenko A, Lozano AM, Kiening KL. Cellular, molecular, and clinical mechanisms of action of deep brain stimulation—a systematic review on established indications and outlook on future developments. EMBO Mol Med. (2019) 11(4):e99575. doi: 10.15252/emmm.201809575
2. Fan H, Zheng Z, Yin Z, Zhang J, Lu G. Deep brain stimulation treating dystonia: a systematic review of targets, body distributions, and etiology classifications. Front Hum Neurosci. (2021) 15:695. doi: 10.3389/fnhum.2021.00695
3. Chandra V, Hilliard JD, Foote KD. Deep brain stimulation for the treatment of tremor. J Neurol Sci. (2022) 435:120190. doi: 10.1016/j.jns.2022.120190
4. Muller-Vahl KR. Deep brain stimulation in tourette syndrome: the known and the unknown. J Neurol Neurosurg Psychiatr. (2019) 90:1076–7. doi: 10.1136/jnnp-2019-321008
5. Gadot R, Najera R, Hirani S, Anand A, Storch E, Goodman WK, et al. Efficacy of deep brain stimulation for treatment-resistant obsessive-compulsive disorder: systematic review and meta-analysis. J Neurol Neurosurg Psychiatry. (2022) 93:1166–73. doi: 10.1136/jnnp-2021-328738
6. Dougherty DD. Deep brain stimulation: clinical applications. Psychiatr Clin North Am. (2018) 41:385–94. doi: 10.1016/j.psc.2018.04.004
7. Bilge MT, Gosai AK, Widge AS. Deep brain stimulation in psychiatry: mechanisms, models, and next-generation therapies. Psychiatr Clin North Am. (2018) 41:373–83. doi: 10.1016/j.psc.2018.04.003
8. Ríos AS, Oxenford S, Neudorfer C, Butenko K, Li N, Rajamani N, et al. Optimal deep brain stimulation sites and networks for stimulation of the fornix in Alzheimer’s disease. Nat Commun. (2022) 13:1–14. doi: 10.1038/s41467-021-27699-2
9. Constantoyannis C, Heilbron B, Honey CR. Electrocardiogram artifacts caused by deep brain stimulation. Can J Neurol Sci. (2004) 31:343–6. doi: 10.1017/S0317167100003425
10. Frysinger RC, Quigg M, Elias WJ. Bipolar deep brain stimulation permits routine EKG, EEG, and polysomnography. Neurology. (2006) 66:268–70. doi: 10.1212/01.wnl.0000194272.79084.7e
11. GBD 2016 Neurology Collaborators. Global, regional, and national burden of neurological disorders, 1990–2016: a systematic analysis for the global burden of disease study 2016. Lancet Neurol. (2019) 18:459–80. doi: 10.1016/S1474-4422(18)30499-X
12. Murray CJ, Vos T, Lozano R, Naghavi M, Flaxman AD, Michaud C, et al. Disability-adjusted life years (DALYs) for 291 diseases and injuries in 21 regions, 1990–2010: a systematic analysis for the global burden of disease study 2010. Lancet. (2012) 380:2197–223. doi: 10.1016/S0140-6736(12)61689-4
13. Poewe W, Seppi K, Tanner CM, Halliday GM, Brundin P, Volkmann J, et al. Parkinson Disease. Nat Rev Dis Primers. (2017) 3:17013. doi: 10.1038/nrdp.2017.13
14. Pringsheim T, Jette N, Frolkis A, Steeves TD. The prevalence of Parkinson’s disease: a systematic review and meta-analysis. Mov Disord. (2014) 29:1583–90. doi: 10.1002/mds.25945
15. Llibre-Guerra JJ, Prina M, Sosa AL, Acosta D, Jimenez-Velazquez IZ, Guerra M, et al. Prevalence of parkinsonism and Parkinson disease in urban and rural populations from Latin America: a community-based study. Lancet Reg Health Am. (2022) 7:100136. doi: 10.1016/j.lana.2021.100136
16. Savica R, Grossardt BR, Bower JH, Ahlskog JE, Rocca WA. Time trends in the incidence of Parkinson’s disease. JAMA Neurol. (2016) 73:981–9. doi: 10.1001/jamaneurol.2016.0947
17. GBD 2015 Neurological Disorders Collaborator Group. Global, regional, and national burden of neurological disorders during 1990–2015: a systematic analysis for the global burden of disease study 2015. Lancet Neurol. (2017) 16:877–97. doi: 10.1016/S1474-4422(17)30299-5
18. Dorsey ER, Bloem BR. The Parkinson pandemic-A call to action. JAMA Neurol. (2018) 75:9–10. doi: 10.1001/jamaneurol.2017.3299
19. Dorsey ER, Sherer T, Okun MS, Bloem BR. The emerging evidence of the Parkinson’s pandemic. J Parkinsons Dis. (2018) 8:S3–8. doi: 10.3233/JPD-181474
20. Klein C, Westenberger A. Genetics of Parkinson’s disease. Cold Spring Harb Perspect Med. (2012) 2:a008888. doi: 10.1101/cshperspect.a008888
21. Ascherio A, Schwarzschild MA. The epidemiology of Parkinson’s disease: risk factors and prevention. Lancet Neurol. (2016) 15:1257–72. doi: 10.1016/S1474-4422(16)30230-7
22. Willis AW, Roberts E, Beck JC, Fiske B, Ross W, Savica R, et al. Incidence of Parkinson’s disease in North America. NPJ Parkinsons Dis. (2022) 8:1–7. doi: 10.1038/s41531-021-00272-w
23. Rodríguez-Violante M, Velásquez-Pérez L, Cervantes-Arriaga A. Incidence rates of Parkinson’s disease in Mexico: analysis of 2014–2017 statistics. Rev Mex Neuroci. (2019) 20:136–40. doi: 10.24875/RMN.M19000043
24. Kestenbaum M, Ford B, Louis ED. Estimating the proportion of essential tremor and Parkinson’s disease patients undergoing deep brain stimulation surgery: five-year data from Columbia University Medical Center (2009–2014). Mov Disord Clin Pract. (2015) 2:384–7. doi: 10.1002/mdc3.12185
25. Wagle Shukla A, Deeb W, Patel B, Ramirez-Zamora A. Is deep brain stimulation therapy underutilized for movement disorders? Expert Rev Neurother. (2018) 18:899–901. doi: 10.1080/14737175.2018.1538791
26. Vedam-Mai V, Deisseroth K, Giordano J, Lazaro-Munoz G, Chiong W, Suthana N, et al. Proceedings of the eighth annual deep brain stimulation think tank: advances in optogenetics, ethical issues affecting DBS research, neuromodulatory approaches for depression, adaptive neurostimulation, and emerging DBS technologies. Front Hum Neurosci. (2021) 15:169. doi: 10.3389/fnhum.2021.645172
27. Scorza FA, Fiorini AC, Scorza CA, Finsterer J. Cardiac abnormalities in Parkinson’s disease and parkinsonism. J Clin Neurosci. (2018) 53:1–5. doi: 10.1016/j.jocn.2018.01.012
28. Hobson P, Meara J. Mortality and quality of death certification in a cohort of patients with Parkinson’s disease and matched controls in North Wales, UK at 18 years: a community-based cohort study. BMJ Open. (2018) 8:e018969. doi: 10.1136/bmjopen-2017-018969
29. Pennington S, Snell K, Lee M, Walker R. The cause of death in idiopathic Parkinson’s disease. Parkinsonism Relat Disord. (2010) 16:434–7. doi: 10.1016/j.parkreldis.2010.04.010
30. Sheen SH, Hong JB, Kim H, Kim J, Han IB, Sohn S. The relationship between Parkinson’s disease and acute myocardial infarction in Korea: a nationwide longitudinal cohort study. J Korean Neurosurg Soc. (2022) 65:507–13. doi: 10.3340/jkns.2021.0195
31. Liang HW, Huang YP, Pan SL. Parkinson’s disease and risk of acute myocardial infarction: a population-based, propensity score-matched, longitudinal follow-up study. Am Heart J. (2015) 169:508–14. doi: 10.1016/j.ahj.2014.11.018
32. Park JH, Kim DH, Park YG, Kwon DY, Choi M, Jung JH, et al. Association of Parkinson’s disease with risk of cardiovascular disease and all-cause mortality: a nationwide, population-based cohort study. Circulation. (2020) 141:1205–7. doi: 10.1161/CIRCULATIONAHA.119.044948
33. Hong CT, Hu HH, Chan L, Bai CH. Prevalent cerebrovascular and cardiovascular disease in people with Parkinson’s disease: a meta-analysis. Clin Epidemiol. (2018) 10:1147–59. doi: 10.2147/CLEP.S163493
34. Alves M, Caldeira D, Ferro JM, Ferreira JJ. Does Parkinson’s disease increase the risk of cardiovascular events? A systematic review and meta-analysis. Eur J Neurol. (2020) 27:288–96. doi: 10.1111/ene.14076
35. Sundbøll J, Szépligeti SK, Szentkúti P, Adelborg K, Horváth-Puhó E, Pedersen L, et al. Risk of Parkinson’s disease and secondary parkinsonism in myocardial infarction survivors. J Am Heart Assoc. (2022) 11:e022768. doi: 10.1161/JAHA.121.022768
36. Rozani V, Gurevich T, Giladi N, El-Ad B, Tsamir J, Hemo B, et al. Higher serum cholesterol and decreased Parkinson’s disease risk: a statin-free cohort study. Mov Disord. (2018) 33:1298–305. doi: 10.1002/mds.27413
37. Hurh K, Park M, Jang SI, Park EC, Jang SY. Association between serum lipid levels over time and risk of Parkinson’s disease. Sci Rep. (2022) 12:1–9. doi: 10.1038/s41598-022-25180-8
38. Vikdahl M, Bäckman L, Johansson I, Forsgren L, Håglin L. Cardiovascular risk factors and the risk of Parkinson’s disease. Eur J Clin Nutr. (2015) 69:729–33. doi: 10.1038/ejcn.2014.259
39. Piqueras-Flores J, López-García A, Moreno-Reig Á, González-Martínez A, Hernández-González A, Vaamonde-Gamo J, et al. Structural and functional alterations of the heart in Parkinson’s disease. Neurol Res. (2018) 40:53–61. doi: 10.1080/01616412.2017.1390933
40. Chen Z, Li G, Liu J. Autonomic dysfunction in Parkinson’s disease: implications for pathophysiology, diagnosis, and treatment. Neurobiol Dis. (2020) 134:104708. doi: 10.1016/j.nbd.2020.104708
41. Liepelt-Scarfone I, Pilotto A, Müller K, Bormann C, Gauss K, Wurster I, et al. Autonomic dysfunction in subjects at high risk for Parkinson’s disease. J Neurol. (2015) 262:2643–52. doi: 10.1007/s00415-015-7888-z
42. Arnao V, Cinturino A, Valentino F, Perini V, Mastrilli S, Bellavia G, et al. In patients with Parkinson disease, autonomic symptoms are frequent and associated with other non-motor symptoms. Clin Auton Res. (2015) 25:301–7. doi: 10.1007/s10286-015-0306-x
43. Merola A, Romagnolo A, Comi C, Rosso M, Artusi CA, Zibetti M, et al. Prevalence and burden of dysautonomia in advanced Parkinson’s disease. Mov Disord. (2017) 32:796–7. doi: 10.1002/mds.26970
44. Kim JS, Lee SH, Oh YS, Park JW, An JY, Park SK, et al. Cardiovascular autonomic dysfunction in mild and advanced Parkinson’s disease. J Mov Disord. (2016) 9:97–103. doi: 10.14802/jmd.16001
45. Scorza FA, Guimarães-Marques M, Nejm M, de Almeida ACG, Scorza CA, Fiorini AC, et al. Sudden unexpected death in Parkinson’s disease: insights from clinical practice. Clinics. (2022) 77:100001. doi: 10.6061/clinics/2022/e3387
46. Bitner A, Zalewski P, Klawe JJ, Newton JL. Drug interactions in Parkinson’s disease: safety of pharmacotherapy for arterial hypertension. Drugs Real World Outcomes. (2015) 2:1–12. doi: 10.1007/s40801-015-0008-7
47. Scorza FA, do Carmo AC, Fiorini AC, Nejm MB, Scorza CA, Finsterer J, et al. Sudden unexpected death in Parkinson’s disease (SUDPAR): a review of publications since the decade of the brain. Clinics. (2017) 72:649–51. doi: 10.6061/clinics/2017(11)01
48. Porta OA, Ochoa CG. ¿Riesgo de muerte súbita en la enfermedad de Parkinson? Neurología Argentina. (2014) 6:114–6. doi: 10.1016/j.neuarg.2014.02.006
49. Santiago JA, Bottero V, Potashkin JA. Biological and clinical implications of comorbidities in Parkinson’s disease. Front Aging Neurosci. (2017) 9:330. doi: 10.3389/fnagi.2017.00330
50. Wang X, Zeng F, Jin WS, Zhu C, Wang QH, Bu XL, et al. Comorbidity burden of patients with Parkinson’s disease and parkinsonism between 2003 and 2012: a multicentre, nationwide, retrospective study in China. Sci Rep. (2017) 7(1):13331. doi: 10.1038/s41598-017-13706-
51. Cervantes-Arriaga A, Esquivel-Zapata Ó, Escobar-Valdivia E, García-Romero D, Alcocer-Salas Á, Rodríguez-Violante M. Asociación entre comorbilidades cardiometabólicas y enfermedad de Parkinson en población mexicana. Gac Med Mex. (2021) 157:280–5. doi: 10.24875/GMM.21000294
52. Flores-Alfaro F, Torres SAC, Cerda-Contreras C, Martínez-Roque DG, Soto-Rincón CA, Góngora-Rivera F, et al. High cardiovascular risk in patients with Parkinson’s disease: a pilot study from northeastern méxico. Parkinsonism Relat Disord. (2020) 79:e43. doi: 10.1016/j.parkreldis.2020.06.175
53. Yang YW, Hsieh TF, Li CI, Liu CS, Lin WY, Chiang JH, et al. Increased risk of Parkinson’s disease with diabetes mellitus in a population-based study. Medicine (Baltimore). (2017) 96:e5921. doi: 10.1097/MD.0000000000005921
54. Kim A, Yang HJ, Kwon JH, Kim MH, Lee J, Jeon B. Mortality of deep brain stimulation and risk factors in patients with Parkinson’s disease: a national cohort study in Korea. J Korean Med Sci. (2023) 38:e10. doi: 10.3346/jkms.2023.38.e10
55. Resnick AS, Foote KD, Rodriguez RL, Malaty IA, Moll JL, Carden DL, et al. The number and nature of emergency department encounters in patients with deep brain stimulators. J Neurol. (2010) 257:122–31. doi: 10.1007/s00415-009-5343-8
56. Morishita T, Foote KD, Burdick AP, Katayama Y, Yamamoto T, Frucht SJ, et al. Identification and management of deep brain stimulation intra- and postoperative urgencies and emergencies. Parkinsonism Relat Disord. (2010) 16:153–62. doi: 10.1016/j.parkreldis.2009.10.003
57. Cossu G, Sensi M. Deep brain stimulation emergencies: how the new technologies could modify the current scenario. Curr Neurol Neurosci Rep. (2017) 17:56. doi: 10.1007/s11910-017-0761-y
58. Rumalla K, Smith KA, Follett KA, Nazzaro JM, Arnold PM. Rates, causes, risk factors, and outcomes of readmission following deep brain stimulation for movement disorders: analysis of the U.S. Nationwide readmissions database. Clin Neurol Neurosurg. (2018) 171:129–34. doi: 10.1016/j.clineuro.2018.06.013
59. Mikos A, Pavon J, Bowers D, Foote KD, Resnick AS, Fernandez HH, et al. Factors related to extended hospital stays following deep brain stimulation for Parkinson’s disease. Parkinsonism Relat Disord. (2010) 16:324–8. doi: 10.1016/j.parkreldis.2010.02.002
60. Rocha S, Monteiro A, Linhares P, Chamadoira C, Basto MA, Reis C, et al. Long-term mortality analysis in Parkinson’s disease treated with deep brain stimulation. Parkinsons Dis. (2014) 2014:639502. doi: 10.1155/2014/639502
61. Ryu HS, Kim MS, You S, Kim MJ, Kim YJ, Kim J, et al. Mortality of advanced Parkinson’s disease patients treated with deep brain stimulation surgery. J Neurol Sci. (2016) 369:230–5. doi: 10.1016/j.jns.2016.08.041
62. Bang Henriksen M, Johnsen EL, Sunde N, Vase A, Gjelstrup MC, Østergaard K. Surviving 10 years with deep brain stimulation for Parkinson’s disease—a follow-up of 79 patients. Eur J Neurol. (2016) 23:53–61. doi: 10.1111/ene.12614
63. Weaver FM, Stroupe KT, Smith B, Gonzalez B, Huo Z, Cao L, et al. Survival in patients with Parkinson’s disease after deep brain stimulation or medical management. Mov Disord. (2017) 32:1756–63. doi: 10.1002/mds.27235
64. Fariba KA, Gupta V. Deep brain stimulation. In: StatPearls [Internet]. Treasure Island (FL): StatPearls Publishing; 2024 Jan (2023). PMID: 32496727. Available at: https://www.ncbi.nlm.nih.gov/books/NBK557847/.
65. Elsanadidy E, Mosa IM, Hou B, Schmid T, El-Kady MF, Khan RS, et al. Self-sustainable intermittent deep brain stimulator. Cell Rep Phys Sci. (2022) 3:101099. doi: 10.1016/j.xcrp.2022.101099
66. Krauss JK, Lipsman N, Aziz T, Boutet A, Brown P, Chang JW, et al. Technology of deep brain stimulation: current status and future directions. Nat Rev Neurol. (2020) 17:75–87. doi: 10.1038/s41582-020-00426-z
67. Hariz M, Blomstedt P. Deep brain stimulation for Parkinson’s disease. J Intern Med. (2022) 292:764–78. doi: 10.1111/joim.13541
68. Guinand A, Noble S, Frei A, Renard J, Tramer MR, Burri H. Extra-cardiac stimulators: what do cardiologists need to know? Europace. (2016) 18:1299–307. doi: 10.1093/europace/euv453
69. Gilbert Z, Mason X, Sebastian R, Tang AM, Martin Del Campo-Vera R, Chen KH, et al. A review of neurophysiological effects and efficiency of waveform parameters in deep brain stimulation. Clin Neurophysiol. (2023) 152:93–111. doi: 10.1016/j.clinph.2023.04.007
70. Butson CR, Cooper SE, Henderson JM, McIntyre CC. Patient-specific analysis of the volume of tissue activated during deep brain stimulation. NeuroImage. (2007) 34:661–70. doi: 10.1016/j.neuroimage.2006.09.034
71. Deli G, Balas I, Nagy F, Balazs E, Janszky J, Komoly S, et al. Comparison of the efficacy of unipolar and bipolar electrode configuration during subthalamic deep brain stimulation. Parkinsonism Relat Disord. (2011) 17:50–4. doi: 10.1016/j.parkreldis.2010.10.012
72. Volkmann J, Moro E, Pahwa R. Basic algorithms for the programming of deep brain stimulation in Parkinson’s disease. Mov Disord. (2006) 21(Suppl 14):S284–89. doi: 10.1002/mds.20961
73. Sotiropoulos SN, Steinmetz PN. Assessing the direct effects of deep brain stimulation using embedded axon models. J Neural Eng. (2007) 4:107–19. doi: 10.1088/1741-2560/4/2/011
74. Carron R, Chaillet A, Filipchuk A, Pasillas-Lépine W, Hammond C. Closing the loop of deep brain stimulation. Front Syst Neurosci. (2013) 7:Q20. doi: 10.3389/fnsys.2013.00110
75. Alosaimi F, Boonstra JT, Tan S, Temel Y, Jahanshahi A. The role of neurotransmitter systems in mediating deep brain stimulation effects in Parkinson’s disease. Front Neurosci. (2022) 16:998932. doi: 10.3389/fnins.2022.998932
76. Musacchio T, Rebenstorff M, Fluri F, Brotchie JM, Volkmann J, Koprich JB, et al. Subthalamic nucleus deep brain stimulation is neuroprotective in the A53T α-synuclein Parkinson’s disease rat model. Ann Neurol. (2017) 81:825–36. doi: 10.1002/ana.24947
77. Smith GS, Mills KA, Pontone GM, Anderson WS, Perepezko KM, Brasic J, et al. Effect of STN DBS on vesicular monoamine transporter 2 and glucose metabolism in Parkinson’s disease. Parkinsonism Relat Disord. (2019) 64:235–41. doi: 10.1016/j.parkreldis.2019.04.006
78. Florio TM, Scarnati E, Rosa I, Di Censo D, Ranieri B, Cimini A, et al. The basal ganglia: more than just a switching device. CNS Neurosci Ther. (2018) 24:677–84. doi: 10.1111/cns.12987
79. Nelson AB, Kreitzer AC. Reassessing models of basal ganglia function and dysfunction. Annu Rev Neurosci. (2014) 37:117–35. doi: 10.1146/annurev-neuro-071013-013916
80. Chakravarthy VS, Joseph D, Bapi RS. What do the basal ganglia do? A modeling perspective. Biol Cybern. (2010) 103:237–53. doi: 10.1007/s00422-010-0401-y
81. Yin HH, Knowlton BJ. The role of the basal ganglia in habit formation. Nat Rev Neurosci. (2006) 7:464–76. doi: 10.1038/nrn1919
82. Obeso JA, Rodríguez-Oroz MC, Benitez-Temino B, Blesa FJ, Guridi J, Marin C, et al. Functional organization of the basal ganglia: therapeutic implications for Parkinson’s disease. Mov Disord. (2008) 23:S548–59. doi: 10.1002/mds.22062
83. Stoessl AJ, Lehericy S, Strafella AP. Imaging insights into basal ganglia function, Parkinson’s disease, and dystonia. Lancet. (2014) 384:532–44. doi: 10.1016/S0140-6736(14)60041-6
84. Eisinger RS, Cernera S, Gittis A, Gunduz A, Okun MS. A review of basal ganglia circuits and physiology: application to deep brain stimulation. Parkinsonism Relat Disord. (2019) 59:9–20. doi: 10.1016/j.parkreldis.2019.01.009
85. Andros L. La estimulación cerebral profunda en la enfermedad de Parkinson. Rev Médico Científ. (2015) 28:24–35.
86. Munhoz RP, Picillo M, Fox SH, Bruno V, Panisset M, Honey CR, et al. Eligibility criteria for deep brain stimulation in Parkinson’s disease, tremor, and dystonia. Can J Neurol Sci. (2016) 43:462–71. doi: 10.1017/cjn.2016.35
87. Marin-Medina DS, Quintero-Moreno JF, Valencia-Vásquez A, Duque-Salazar C, Gil-Restrepo AF, Castaño-Montoya JP, et al. Estimulación cerebral profunda en enfermedad de Parkinson. Iatreia. (2018) 31:262–73. doi: 10.17533/udea.iatreia.v31n3a04
88. Capelle HH, Simpson RK Jr, Kronenbuerger M, Michaelsen J, Tronnier V, Krauss JK. Long-term deep brain stimulation in elderly patients with cardiac pacemakers. J Neurosurg. (2005) 102:53–9. doi: 10.3171/jns.2005.102.1.0053
89. Sharma M, Talbott D, Deogaonkar M. Interaction between cardiac pacemakers and deep brain stimulation pulse generators: technical considerations. Basal Ganglia. (2016) 6:19–22. doi: 10.1016/j.baga.2015.11.001
90. Koeglsperger T, Palleis C, Hell F, Mehrkens JH, Bötzel K. Deep brain stimulation programming for movement disorders: current concepts and evidence-based strategies. Front Neurol. (2019) 10:410. doi: 10.3389/fneur.2019.00410
91. Miocinovic S, Lempka SF, Russo GS, Maks CB, Butson CR, Sakaie KE, et al. Experimental and theoretical characterization of the voltage distribution generated by deep brain stimulation. Exp Neurol. (2009) 216:166–76. doi: 10.1016/j.expneurol.2008.11.024
92. Gratiy SL, Halnes G, Denman D, Hawrylycz MJ, Koch C, Einevoll GT, et al. From Maxwell’s equations to the theory of current-source density analysis. Eur J Neurosci. (2017) 45:1013–23. doi: 10.1111/ejn.13534
93. Mruk M, Stroop R, Boergel J, Lang NM, Nakamura M, Lehrke R, et al. Neurostimulator-induced ECG artifacts: a systematic analysis. Clin Neurol Neurosurg. (2021) 203:106557. doi: 10.1016/j.clineuro.2021.106557
94. Chen Y, Ma B, Hao H, Li L. Removal of electrocardiogram artifacts from local field potentials recorded by sensing-enabled neurostimulator. Front Neurosci. (2021) 15:637274. doi: 10.3389/fnins.2021.637274
95. Arafat T, Miron G, Strauss I, Fahoum F. Electrodiagnostic artifacts due to neurostimulation devices for drug-resistant epilepsy. Epilepsy Behav Rep. (2022) 20:100566. doi: 10.1016/j.ebr.2022.100566
96. Simma L, Fontana M, Schmitt-Mechelke T. Resuscitation of a patient with an implanted electronic device. Ann Emerg Med. (2018) 71:467–9. doi: 10.1016/j.annemergmed.2017.07.009
97. Kooger BL, Jaspers GJ, van Hunnik A, Delhaas T, Vanagt WY. Deep brain stimulator-induced flutter-like artefact on holter recording. Eur Heart J. (2017) 38:61. doi: 10.1093/eurheartj/ehv751
98. Stelzer MA, Padanilam MS, Miller JJ, Foreman J, Golden K, Ahmed AS. Identifying and troubleshooting low-frequency artifacts mimicking atrial flutter caused by deep brain stimulator. Heart Rhythm Case Rep. (2021) 7:683. doi: 10.1016/j.hrcr.2021.07.001
99. Mindermann T, Maurer D. Delayed diagnosis of myocardial infarction due to deep brain stimulation. Acta Neurochir. (2013) 155:1679–80. doi: 10.1007/s00701-013-1702-4
100. Martin WA, Camenzind E, Burkhard PR. ECG Artifact due to deep brain stimulation. Lancet. (2003) 361:1431. doi: 10.1016/S0140-6736(03)13136-4
101. Lee HJ, Lee WW, Kim SK, Park H, Jeon HS, Kim HB, et al. Tremor frequency characteristics in Parkinson’s disease under resting-state and stress-state conditions. J Neurol Sci. (2016) 362:272–7. doi: 10.1016/j.jns.2016.01.058
102. Pérez-Riera AR, Barbosa-Barros R, Daminello-Raimundo R, de Abreu LC. Main artifacts in electrocardiography. Ann Noninvasive Electrocardiol. (2018) 23:e12483. doi: 10.1111/anec.12483
103. Sawhney V, Campbell N. Parkinson’s disease and pseudo-ventricular tachycardia. QJM. (2014) 107:775. doi: 10.1093/qjmed/hcu024
104. Hwang WJ, Chen JY, Sung PS, Lee JC. Parkinsonian tremor-induced electrocardiographic artifacts mimicking atrial flutter/fibrillation or ventricular tachycardia. Int J Cardiol. (2014) 173:597–600. doi: 10.1016/j.ijcard.2014.03.090
105. Kligfield P, Gettes LS, Bailey JJ, Childers R, Deal BJ, Hancock EW, et al. Recommendations for the standardization and interpretation of the electrocardiogram. Circulation. (2007) 115:1306–24. doi: 10.1161/CIRCULATIONAHA.106.180200
106. Nie P, Zhang J, Yang X, Shao Y, Zhang X, Liu W, et al. Remote programming in patients with Parkinson’s disease after deep brain stimulation: safe, effective, and economical. Front Neurol. (2022) 13:821. doi: 10.3389/fneur.2022.821
107. Pintér D, Járdaházi E, Janszky J, Kovács N. Potential clinical and economic benefits of remote deep brain stimulation programming. Sci Rep. (2022) 12:1–10. doi: 10.1038/s41598-022-22206-z
108. Frey J, Cagle J, Johnson KA, Wong JK, Hilliard JD, Butson CR, et al. Past, present, and future of deep brain stimulation: hardware, software, imaging, physiology and novel approaches. Front Neurol. (2022) 13:343. doi: 10.3389/fneur.2022.825178
Keywords: electrocardiography, electrophysiology, frequency filters, artifact, deep brain stimulation, movement disorders, Parkinson’s disease, neurostimulation
Citation: Sierra-Fernández CR, Garnica-Geronimo LR, Huipe-Dimas A, Ortega-Hernandez JA, Ruiz-Mafud MA, Cervantes-Arriaga A, Hernández-Medrano AJ and Rodríguez-Violante M (2024) Electrocardiographic approach strategies in patients with Parkinson disease treated with deep brain stimulation. Front. Cardiovasc. Med. 11:1265089. doi: 10.3389/fcvm.2024.1265089
Received: 21 July 2023; Accepted: 19 March 2024;
Published: 12 April 2024.
Edited by:
Constantina Aggeli, National and Kapodistrian University of Athens, GreeceReviewed by:
Robert LeMoyne, Northern Arizona University, United States© 2024 Sierra-Fernández, Garnica-Geronimo, Huipe-Dimas, Ortega-Hernandez, Ruiz-Mafud, Cervantes-Arriaga, Hernández-Medrano and Rodríguez-Violante. This is an open-access article distributed under the terms of the Creative Commons Attribution License (CC BY). The use, distribution or reproduction in other forums is permitted, provided the original author(s) and the copyright owner(s) are credited and that the original publication in this journal is cited, in accordance with accepted academic practice. No use, distribution or reproduction is permitted which does not comply with these terms.
*Correspondence: Luis Rodrigo Garnica-Geronimo cm9kcmlnby5nYXJuaWNhLWdlcm9uaW1vQG91dGxvb2suY29t
†These authors have contributed equally to this work and share first authorship
Abbreviations DBS, deep brain stimulation; EF, electromagnetic field; ECG, electrocardiogram; IPG, implantable pulse generator; PD, Parkinson’s disease; VTA, volume of tissue activated.
‡ORCID Amin Cervantes-Arriaga orcid.org/0000-0002-3935-9278
Disclaimer: All claims expressed in this article are solely those of the authors and do not necessarily represent those of their affiliated organizations, or those of the publisher, the editors and the reviewers. Any product that may be evaluated in this article or claim that may be made by its manufacturer is not guaranteed or endorsed by the publisher.
Research integrity at Frontiers
Learn more about the work of our research integrity team to safeguard the quality of each article we publish.