- 1Graduate Program in Complexity Sciences, Autonomous University of México City, México City, Mexico
- 2Immunology Department, National Institute of Cardiology ‘Ignacio Chávez’, México City, Mexico
- 3Computational Genomics Division, National Institute of Genomic Medicine, México City, Mexico
- 4Center for Complexity Sciences, Universidad Nacional Autónoma de México, México City, Mexico
Cardiovascular diseases stand as a prominent global cause of mortality, their intricate origins often entwined with comorbidities and multimorbid conditions. Acknowledging the pivotal roles of age, sex, and social determinants of health in shaping the onset and progression of these diseases, our study delves into the nuanced interplay between life-stage, socioeconomic status, and comorbidity patterns within cardiovascular diseases. Leveraging data from a cross-sectional survey encompassing Mexican adults, we unearth a robust association between these variables and the prevalence of comorbidities linked to cardiovascular conditions. To foster a comprehensive understanding of multimorbidity patterns across diverse life-stages, we scrutinize an extensive dataset comprising 47,377 cases diagnosed with cardiovascular ailments at Mexico’s national reference hospital. Extracting sociodemographic details, primary diagnoses prompting hospitalization, and additional conditions identified through ICD-10 codes, we unveil subtle yet significant associations and discuss pertinent specific cases. Our results underscore a noteworthy trend: younger patients of lower socioeconomic status exhibit a heightened likelihood of cardiovascular comorbidities compared to their older counterparts with a higher socioeconomic status. By empowering clinicians to discern non-evident comorbidities, our study aims to refine therapeutic designs. These findings offer profound insights into the intricate interplay among life-stage, socioeconomic status, and comorbidity patterns within cardiovascular diseases. Armed with data-supported approaches that account for these factors, clinical practices stand to be enhanced, and public health policies informed, ultimately advancing the prevention and management of cardiovascular disease in Mexico.
1 Introduction
Cardiovascular diseases in diferent life-stages constitute big challenges for health systems worldwide. Most of these conditions may be related to ageing, sex and socioeconomic status (SES) and are also strongly associated with the particular complex patient’s needs, social determinants and quality of healthcare (2, 1). Patients may complicate in their evolution by multiple cooccuring medical conditions or comorbidities making more challenging to care for them (2). To account for this, the term comorbidity was coined to represent the occurrence of other medical conditions in addition to an index condition of interest (3).
Such comorbidity relationships occur whenever two or more diseases are present in the same individual more often than by chance alone (4, 5). Multimorbidity is associated with the risk of premature death, loss of functional capacity, depression, complex drug regimes, psychological distress, declined quality of life, increase in hospitalizations and decreased productivity. This is also linked to economic burden for health-care systems and society (7, 6).
There is evidence linking comorbidity with social determinants of health (SDHs) such as cultural issues, social support, housing, demographic environment and SES. Together, these factors, make a source of complexity that creates potential vulnerability for someone facing it, many of them in disavantage for being in socioeconomically deprived areas. The association between SES and prevalence of multimorbidity has been recently established (8, 11, 10, 9, 12). Also, these complex phenomena have profound implications for the delivery of high quality care of chronic health conditions and remark the necesity of complex interventions to tackle multimorbidity (2, 13–15).
Likewise, the non-random co-occurrence of certain diseases differs in different life-stages: the prevalence of multimorbidity increases with age, 60% of the events are reported among 65–74 year olds higher than the prevalence of each individual disease (16, 17). Also, the sex is increasingly perceived as a key determinant of multimorbidity in cardiovascular disease (CVD). Although it has been seen as a predominantly men disease, due to men’s higher absolute risk compared with women, the relative risk in women of CVD morbidity and mortality is higher increased by some medical factors (diabetes, hypertension, hypercholesterolemia, obesity, chronic kidney, rheumatoid arthritis and other inflammatory joint diseases) (18, 19). Moreover, serious multiple chronic conditions are more common in older women (rather than 65) and may limit treatment alternatives (10, 20, 21).
Multimorbidity presents many challenges, which may at times seem overwhelming. In such a scenario, evidence-based treatment guidelines, designed for single diseases, may lead to serious therapeutic conflicts (1). To circumvent such limitations, a personalized approach to medicine may benefit from the inclusion of ideas from a somewhat recent field of research, generally known as systems biology or network medicine –when applied to humans–. This approach offers the potential to decipher and understand the relationships between comorbidities at a much deeper level by considering coordinated instances (systems) rather than single conditions. A theoretical framework of diseasome has indicated that most human diseases are interdependent. These concept lead to anoher called the human disease network (HDN), a graph in which two diseases are connected if they have in common some biological, genetic, metabolic o even socioeconomic element (1).
The present study is aimed to analyze the patterns of cardiovascular-associated multimorbidity stratified by life-stages, sex and socioeconomic status. Studying such patterns at a large scale will be useful, both to discover trends helpful for public health care planning, as well as to serve as additional clues to understand the complex interaction between genetic/molecular, clinic and social/environmental conditionants of cardiovascular diseases in the different age/sex/SES.
Here, we will expand upon the outcomes derived from various comorbidity networks under consideration. These networks were constructed based on previously outlined criteria, taking into account structural characteristics arising from relationships between pairs of diseases. The focus will be on mutual information (MI) shared by diseases (depending on the frequency of their joint presence, as we will see later), acting as an indicator of co-occurrence between two conditions. This approach offers an additional perspective for discerning comorbidity patterns across diverse networks.
To pinpoint comorbidities potentially linked to sex and/or SES within each network, we will employ the Page Rank Score (PRS), a network indicator of overall influence. This scoring system enables the numerical identification of diseases with greater relevance within each network (1). By factoring in MI between pairs of diseases, the PRS enhances precision, providing richer insights into comorbidity and multimorbidity phenomena in individuals.
The main reserach question that we will aboard in this work is then How does the interplay between life-stage and socioeconomic status influence the comorbidity patterns in cardiovascular diseases among the Mexican metropolitan population, and what are the subtle associations and differences in comorbidity prevalence across age groups and socioeconomic strata?
2 Materials and methods
2.1 Data acquisition (electronic health records)
The National Institute of Cardiology ’Ignacio Chávez’ (NICICH), one of Mexico’s National Institutes of Health, is the reference hospital for specialized cardiovascular care in Mexico. The NICICH it is also a third level hospital receiving in-patients with related ailments such as metabolic, inflammatory and systemic diseases, whose treatment may involve immunology, rheumatology, nephrology, and similar specialities in addition to cardiology-related treatments (1).
In this work we used the NICICH Electronic Health Record (EHR) database entries as recorded between January 1, 2011 and June 31, 2019. The EHR-database contains information on socioeconomic factors as well as the main clinical diagnosis that led to hospitalization, it also reports other diseases, disorders, conditions or health problems that the individuals may present. The SES as recorded in the institutional file is a well-defined construct that involves the weighting of variables related to education, employment status, family monetary income, access to public services (water, electricity, drainage) and housing conditions (rural or urban). The EHR management procedures of the institution are set to provide up to five main comorbidities. International Classification of Diseases, tenth revision (ICD-10) was used to identify and clasificate them. The full set of hospital discharged patients, with all types of diagnostics, age, sex and SES were considered in the time period under study, with the exception of those with incomplete information or erroneous coding. The study population included 47, 377 discharged cases. The cardiovascular comorbidities assessed included any disease registered in each case (see Figure 1).
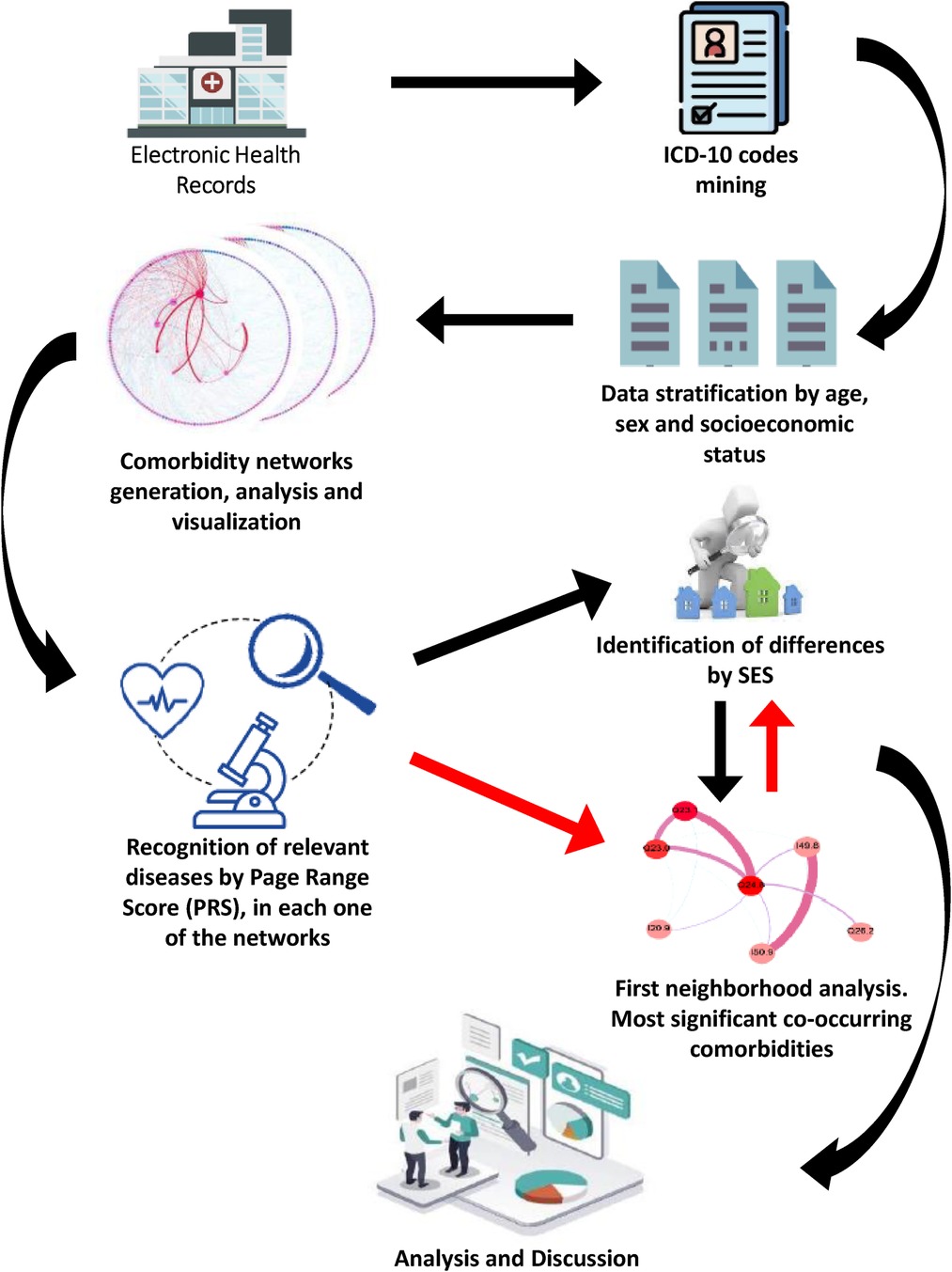
Figure 1. Flow chart of study. The figure represents the general workflow of this study from data collection (EHR mining), data pre-processing and processing, analytics, results and discussion.
2.2 Data processing (ICD-10 coding)
Once EHR data has been pre-processed to tabular format, disease and comorbidity relationships could be investigated. Mining, processing and cross-transforming ICD-10 data were performed using the icd (v. 4.0.9) R library (22) (https://www.rdocumentation.org/packages/icd/versions/4.0.9). While ICD codes are increasingly becoming useful tools in the clinical and basic research arenas, their use is not free of caveats and limitations (for a brief dicsussion of some of these in the context of current norms, please refer to the relevant paragrpahs in the discussion section).
2.3 Statistical analysis
A database of 47, 377 electronic health records (EHRs) was used as this study corpus. Analysis was stratified by age and sex group. Descriptive statistics were used to summarize overall information. The chronic conditions with the highest prevalence, stratified by SES, and the number of chronic conditions associated with each disease were computed.
2.3.1 Cohort stratification
For the purpose of statistical and network analysis, patients were stratified based on age and sex. The age groups were defined as follows: the 0–20 years old age bracket has 9,782 individuals (20.65%) of which 4,921 are women and 4,861 men; for the 21–40 years old range there were 6,939 individuals (14.65%) split into 3,593 women and 3,346 men; the 41–60 year old range included 13,690 persons (28.90%) with 5,095 women and 8,595 men; 14,537 (30.68%) individuals conformed the 61–80 years old group with 5,695 women and 8,842 men; lastly the 81 years and older group had 2,429 (5.13%) registered patients 1,187 women and 1,242 men. These strata were used to build the different comorbidity networks that will be presented and discussed later.
2.3.2 Cardiovascular comorbidity network (CVCnetworks)
Electronic health records data was processed using in-house developed code (in the R programming language) for the design and analysis of comorbidity networks as previously reported (1). Programming code for this study is available in the following public access repository: https://github.com/CSB-IG/Comorbidity_Networks. Once the mining of the medical cases was carried out, a set of undirected networks (one network for each age/sex/SES bracket combination, see Subsection 2.4), was built based on the significant co-ocurrent diseases coded according to ICD-10 codes.
Briefly, the origin and destination nodes in these networks are diseases as identified with their respective ICD-10 codes. Subsequently, a link was drawn between these nodes, as long as at least these diseases co-occurr in the same person within this group more often than by chance alone (hypergeometric test, with a False Discovery Rate (FDR) multiple testing correction FDR < 0.05). The strength of the comorbidity association was determined by using the MI calculated for each pair of diseases in the CVCnetworks by using a custom made script (available at https://github.com/CSB-IG/ICD_Comorbidity/blob/main/Disc_Mut_Info.py) based on the mutual_info_score function of the sklearn.metrics Python package.
2.4 Network statistics and visualization
In network theory, one of the parameters used to evaluate the connections in the graph is the degree centrality (DC), the total number of links on a node or the sum of the frequencies of the interactions. The degree distribution of a disease is the number of ICD-10 codes associated with that disease. Aside from the node degree, a relevant centrality measure is the PRS (23) that captures the relative influence of a given node in the context of network communication. The Network Analyzer plugin (24) in the Cytoscape open source network analysis suite was used to explore and visualize the network (25) and also the CytoNCA package was used to calculate further network centrality measures (26).
Betweenness centrality (BC) measure is used to assess the relevance of a given condition in the context of a node’s influence in global network information flow. Weighted network analytics, PRS calculations and visualization were performed by using Gephi (27). In brief, MI will be used to assess the strength of comorbidity relations (i.e., a higher MI value represents a stronger comorbidity association between two diseased conditions). PRS on the other hand will be used to assess the relevance of a given disease in the context of the comorbidity network given its vicinity (i.e., a higher PRS value represents a higher potential to become a multimorbid condition). In the context of this vicinity, we will often refer to the set of diseases directly connected to a given disease as their comorbidity nearest neighbors (CNNs).
In this study, a double-circle layout visualization was implemented, where nodes were arranged according to their PRS in a counter-clockwise direction, and the top 10 highest ranking diseases were placed in the center of the graph. Nodes were colored on a gradient scale from red (higher closeness centrality) to blue (lower closeness centrality). Additionally, the node size was determined based on their Betweenness Centrality measure, where larger nodes indicated a higher value of Betweenness Centrality.
3 Results
3.1 Cardiovascular comorbidity networks general results
Comorbidity networks were built for the specific age/sex/SES as previosuly described and a general topological analysis was conducted prior to a detailed analysis of each network. Table 1 present the main topological features of these networks. By examining the connectivity and structural patterns, significant relationships can be identified, which will be discussed later (A set of tables containing the full connectivity informations for all the networks can be found in the Supplementary Materials).
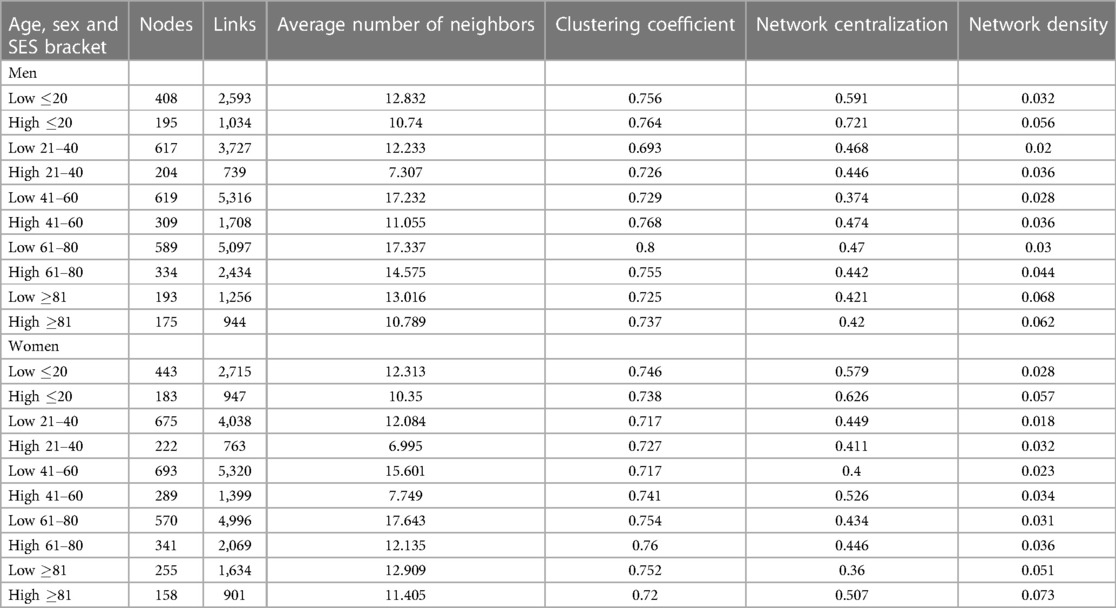
Table 1. Topological characteristics of comorbidity networks by age, sex and socioeconomic status (low or high).
The analysis of the various networks shown in Table 1 revealed that, overall, individuals with low SES exhibited a higher diversity of diseases, reflected in the larger number of nodes, often double or more compared to high SES networks. This phenomenon is attributed to health inequalities arising from constraints faced by this population, making them more susceptible to developing diseases not prevalent in high SES individuals or manifesting and being treated differently due to varying access to necessary resources, ranging from adequate nutrition to healthcare access.
The clustering coefficient showed notable uniformity across all networks, with the network corresponding to men aged 61–80 with low SES exhibiting the highest clustering level. This suggests a higher likelihood of individuals in this network easily manifesting any disease within the network from an initial disease. While this observation doesn’t sharply differentiate from other networks, it raises concerns about disease interactions and, consequently, treatment concerning pharmacological interactions.
The higher prevalence of disease diversity in low SES may be associated with the greater density observed in high SES networks. This increased density implies more interconnections among all diseases in high SES networks. However, this doesn’t necessarily indicate a higher propensity for comorbidity in high SES individuals, as evidenced by a considerably higher number of connections in low SES, particularly among men over 80.
Furthermore, finding a higher network centralization in graphs for the age range of 0–20 years, with even greater centralization in high SES, may result from comorbidities influenced by factors related to birth. Additionally, there is a lower disease diversity in high SES, while the higher number of diseases in low SES diversifies the conditions centralizing comorbidity relationships.
The average number of comorbidities, measured through the average neighbors in various networks, tends to be higher in older ages compared to young individuals. This trend is more pronounced in men than women. Notably, the decrease in the average number of comorbidities in the population over 80 contradicts existing literature.
A closer analysis of the different CVC networks reveal that there are some pairs of diseases that are prevalent as the most relevant comorbidities in more than one group of age/sex/SES. Some of the most relevant of these pairs of diseases are shown in Figure 2. Understanding disease pairs that transcend demographic (e.g., age/sex/SES) boundaries may help us to provide a holistic view of health challenges and opportunities for intervention. It may contribute to more effective public health strategies and policies that consider the interconnected nature of diseases across diverse populations. Let us examine some of the implications.
3.2 Cardiovascular comorbidity networks based on socioeconomic status in men and women aged 20 years or younger
Upon visual inspection of the distinct networks depicted in Figure 3, discernible structural differences in their connectivity patterns come to light. A more nuanced examination of the network statistics could provide additional insights into shared features and commonalities. For example, Table 2 highlights the top 5 diseases with the highest comorbidity burden, as indicated by their respective PRS within the specified network.
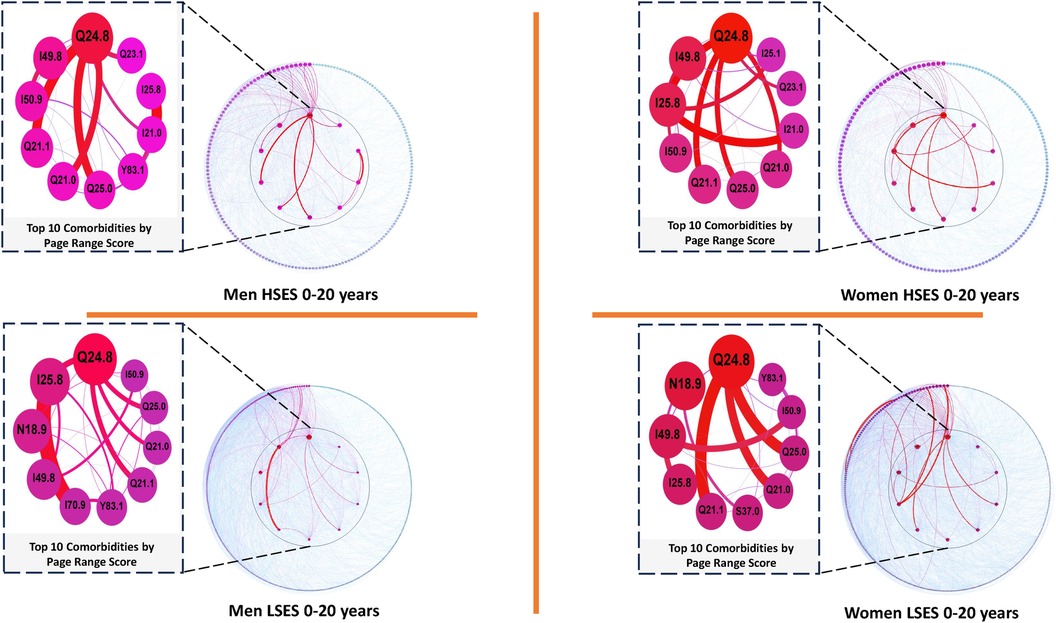
Figure 3. Comorbidity networks for patients aged 0–20 years old, for both sexes in the low (LSES) and high (HSES) socioeconomic status. Nodes are ordered according with their Page Rank Score (PRS). High PRS nodes appear in the center. Size and color intensity (red implies higher values, blue lower values) of the nodes is also given by the PRS as a measure of relative importance in the network. Size and color of the edges represent the mutual information weight among disease pairs.

Table 2. Top 5 diseases with a higher comorbidity burden in networks for men and women patients of low and high SES aged 20 years old or less as well as their PRS value.
Several commonalities emerge among these highly comorbid diseases. Regardless of sex or SES, the notable presence of Other specified congenital malformations of the heart (Q24.8) and Generalized and unspecified atherosclerosis (I70.9) is observed. Additionally, Other forms of chronic ischemic heart disease (I25.8) appears in three out of four networks, except for men with high SES, where it ranks 9th according to its PRS. A similar scenario unfolds for Atrial septal defect, which moves to the 7th rank in men of low SES. Notably, most highly prevalent and comorbid diseases in this age group exhibit a strong genetic risk component, likely explaining their consistently high rankings across all four networks, irrespective of sex or SES.
Equally noteworthy, albeit for divergent reasons, are instances such as Unspecified cardiac insufficiency (I50.9), which holds a high rank solely among individuals of both sexes with high SES, and Chronic kidney disease, unspecified (N18.9), appearing exclusively in the top 5 for men and women of low SES. The association of unspecified chronic disease with low SES in children and young adults (up to 20 years old) suggests a probable link to environmental factors. Consequently, we opted to investigate its network neighborhood. Intriguingly, robust comorbidity relationships with M32.1 Systemic lupus erythematosus with organ or system involvement were identified in networks corresponding to different age/sex/SES categories.
It is pertinent to note that the documented presence of what has been termed lupus nephritis in children is well-established (29–31, 28). Notably, lupus nephritis can be specifically reported using the ICD-10 code M32.14 Glomerular disease in systemic lupus erythematosus, rather than the more general code M32.1. Nevertheless, the occurrence of juvenile systemic lupus erythematosus (JSLE) has been reported as a more active disease in children and young adults, characterized by faster progression and worse outcomes, including progressive chronic kidney disease, compared to its adult-onset counterpart, leading to poorer long-term survival. Studies indicate that lupus nephritis may affect up to 50%–75% of all children with JSLE. Consequently, analyzing the comorbidity landscapes associated with either concurrent N18.9 and M32.1 (or M32.14) may offer valuable insights for determining optimal diagnostic and therapeutic strategies to enhance patient outcomes.
3.3 Cardiovascular comorbidity networks based on socioeconomic status in men and women aged 21–40 years
Examination of comorbidity networks for individuals aged 21–40 years, encompassing both sexes and SES, reveals similar trends in highly comorbid conditions as observed in children and young adults (aged 0–21 years). Noteworthy diseases, including Other specified cardiac arrhythmias (I49.8), Other forms of chronic ischemic heart disease (I25.8), Chronic kidney disease, unspecified (N18.9), and Other specified congenital malformations of the heart (Q24.8), consistently rank among the top 5 conditions with high PRS in their respective networks, irrespective of sex or SES (see Table 3 and Figure 4).

Table 3. Top 5 diseases with a higher comorbidity burden in networks for men and women patients of low and high SES aged 21 through 40 years old as well as their PRS value.
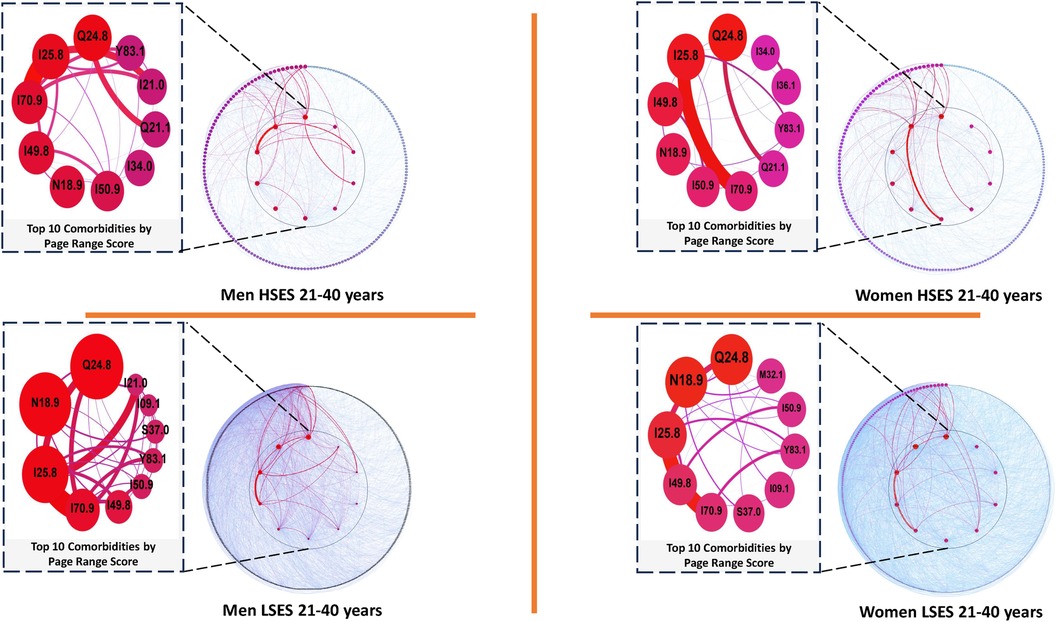
Figure 4. Comorbidity networks for patients aged 21–40 years old, for both sexes in the low (LSES) and high (HSES) socioeconomic status. Visualization parameters are as in Figure 3.
It is evident that, up to this age bracket, the most highly morbid conditions are largely shared across different SES. Notably, Other and unspecified atherosclerosis (I70.9), which does not appear in the top 5 for women with high SES in Table 3, is nonetheless ranked 6th in that particular subgroup.
3.4 Cardiovascular comorbidity networks based on socioeconomic status in men and women aged 41–60 years
In examining the networks for the current age range (depicted in Figure 5), notable diseases consistently rank among the top five in each graph, as outlined in Table 4. Other forms of chronic ischemic heart disease (I25.8), Other specified cardiac arrhythmias (I49.8), Other specified congenital malformations of the heart (Q24.8), and Unspecified atherosclerosis (I70.9) persist in both men and women across all SES. Conversely, Unspecified heart failure (I50.9) exclusively appears in the high SES group, while Unspecified chronic kidney disease (N18.9) emerges solely among the five most relevant diseases in the low SES group. Consequently, a more in-depth analysis of these latter two diseases was undertaken.
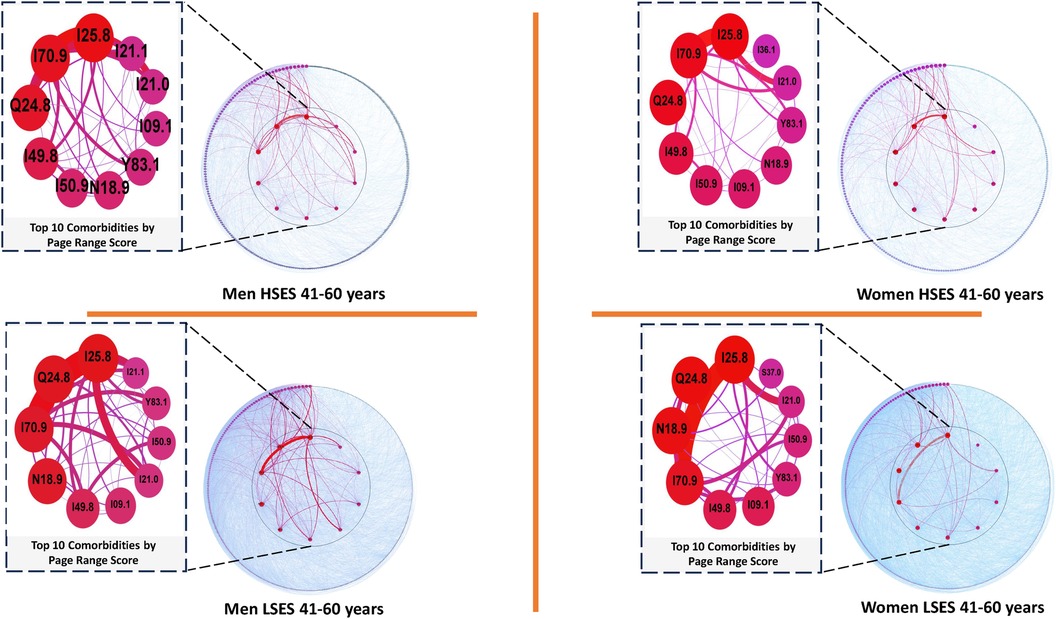
Figure 5. Comorbidity networks for patients aged 41–60 years old, for both sexes in the low (LSES) and high (HSES) socioeconomic status. Visualization parameters are as in Figure 3.

Table 4. Top 5 diseases with a higher comorbidity burden in networks for men and women patients of low and high SES aged 41 through 60 years old as well as their PRS value.
Regarding Unspecified heart failure (I50.9) in the low SES group, it maintains a substantial position, ranking eighth among both men and women based on their PRS. In contrast, Unspecified chronic kidney disease (N18.9) continues to feature prominently in the high SES group, ranking sixth among men and seventh among women according to their PRS.
As no discernible differences were observed in SES based on the PRS, a first-neighbors analysis was conducted on the diseases listed in Table 4. This analysis considered the MI between pairs of diseases and examined the relationships forming between the diseases mentioned in the table. Notably, the relationship between Unspecified atherosclerosis (I70.9) and Unspecified chronic kidney disease (N18.9), sharing an MI of 0.018057, exhibited a distinction with respect to SES in women networks.
3.5 Cardiovascular comorbidity networks based on socioeconomic status in men and women aged 61–80 years
In this population, Table 5 highlights consistent representation of the same diseases among the top positions in all graphs, including Other forms of chronic ischemic heart disease (I25.8), Unspecified atherosclerosis (I70.9), Other specified congenital malformations of the heart (Q24.8), and Other specified cardiac arrhythmias (I49.8). Notably, Unspecified chronic kidney disease (N18.9) ranks sixth for high-SES men. Similarly, Unspecified rheumatic diseases of endocardial valve (I09.1) appears in sixth place for low-SES men and women across both strata.

Table 5. Top 5 diseases with a higher comorbidity burden in networks for men and women patients of low and high SES aged 61 through 80 years old as well as their PRS value.
In the analysis of nearest neighbors, it was found that Other specified congenital malformations of the heart (Q24.8), consistently positioned in the networks from early stages of life, is linked to Unspecified rheumatic diseases of endocardial valve (I09.1) exclusively in low-SES men, sharing an MI of 0.015235 and occupying the fifty-second position among the relationships in this population. This phenomenon appears solely in this age range and in low-SES men (see Figure 6). For women, the relationship between Other specified congenital malformations of the heart (Q24.8) and Unspecified rheumatic diseases of endocardial valve (I09.1), absent in the present age range, is evident between 21 and 60 years old, exclusively in the low-SES group.
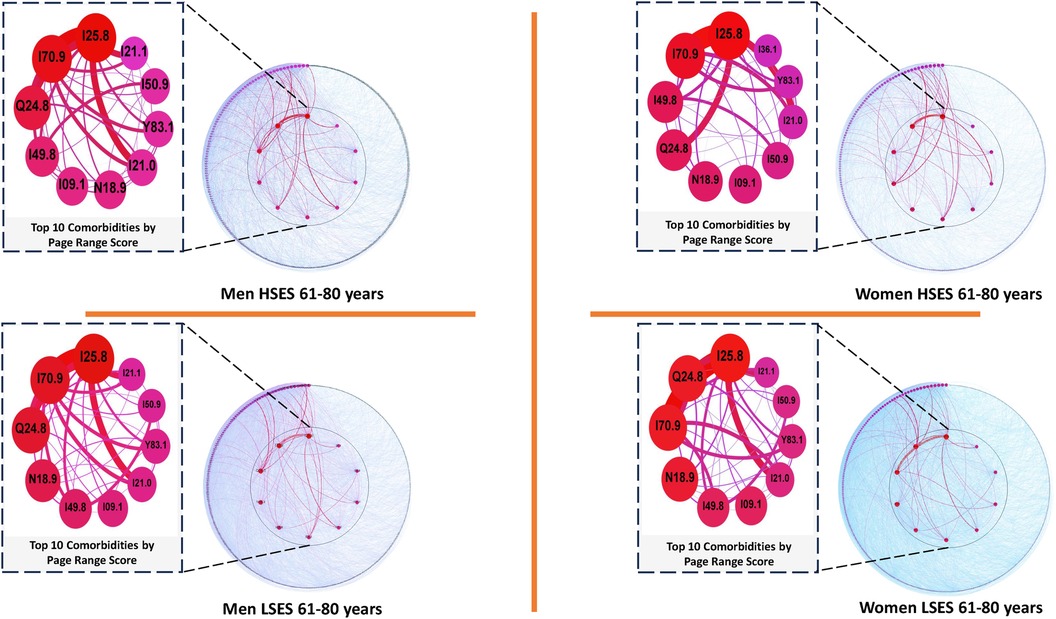
Figure 6. Comorbidity networks for patients aged 61–80 years old, for both sexes in the low (LSES) and high (HSES) socioeconomic status. Visualization parameters are as in Figure 3.
3.6 Cardiovascular comorbidity networks based on socioeconomic status in men and women aged 80 and older
In the population aged 80 and older, Table 6 reveals a consistent top three diseases across both sexes and SES (see Figure 7): Other forms of chronic ischemic heart disease (I25.8), Unspecified atherosclerosis (I70.9), and Other specified cardiac arrhythmias (I49.8). These conditions maintain their prominence throughout the lifespan of the study population, alongside Unspecified heart failure (I50.9) and Unspecified chronic kidney disease (N18.9).
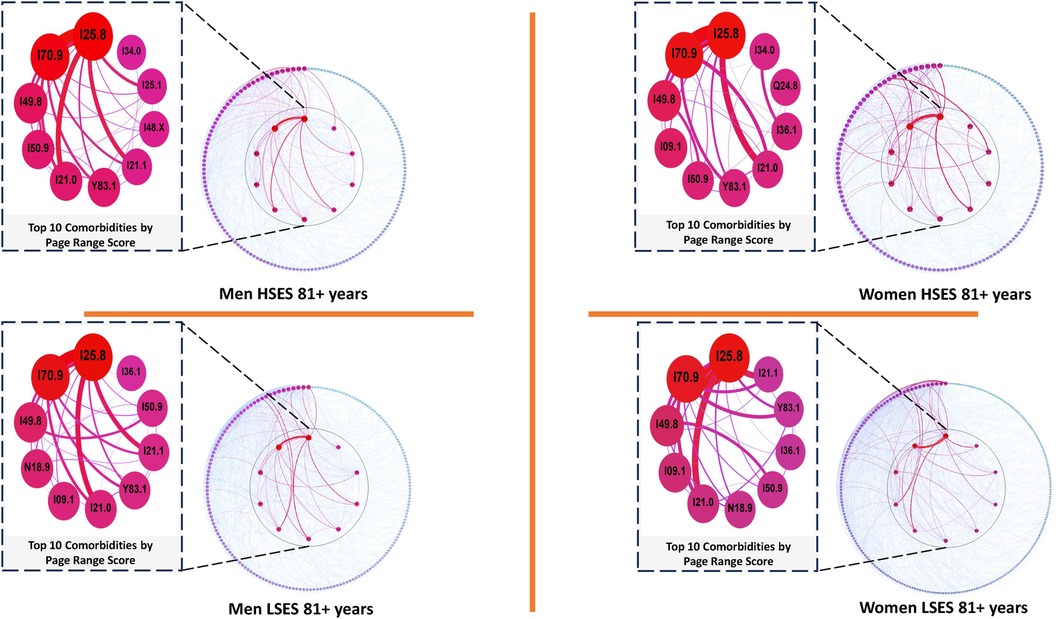
Figure 7. Comorbidity networks for patients aged 81 years and older, for both sexes in the low (LSES) and high (HSES) socioeconomic status. Visualization parameters are as in Figure 3.

Table 6. Top 5 diseases with a higher comorbidity burden in networks for men and women patients of low and high SES aged 80 years and older as well as their PRS value.
Regarding the latter two conditions, it is noteworthy that Unspecified heart failure (I50.9), absent among the top five diseases in the low SES group, ranks ninth for men and seventh for women in this stratum. On the other hand, Unspecified chronic kidney disease (N18.9), exclusive to men in the low SES group, is positioned nineteenth for men in the high SES group. For women, it appears sixth in the low SES group and twelfth in the high SES group according to their PRS.
As for the remaining two diseases, they exhibit a relationship that is exclusive to women in the low SES group within the present age range. In this co-occurrence, the pair ranks ninety-sixth out of 1,634 disease pairs, with a MI of 0.004605. It is noteworthy that, at the individual level, Acute transmural myocardial infarction of anterior wall (I21.0), which, according to the IPR analysis, is absent in women of high SES and men of low SES, takes the seventh place in the former case and the sixth place in the latter. Conversely, Unspecified rheumatic diseases of endocardium valve (I09.1) holds the thirteenth place of relevance for men of high SES.
The relationship between Acute transmural myocardial infarction of anterior wall (I21.0) and Unspecified rheumatic diseases of endocardium valve (I09.1), observed in previous age ranges, is limited to the low SES group. Specifically, it appears only between the ages of 61 and 80 for men and from 41 up to the present age range for women.
4 Discussion
In this section, we will delve deeper into the outcomes derived from the various comorbidity networks, which were constructed based on the previously described criteria considering the structural characteristics arising from relationships between pairs of diseases. The analysis incorporates mutual information as an indicator of co-occurrence between two diseases, offering a supplementary perspective for discerning comorbidity patterns within these networks.
Shared pairs of diseases across several ge/sex/SAS strata (recall Figure 2) may provide information in the following dimensions:
• Cross-sectional patterns: Identifying disease pairs across different demographic groups can highlight patterns that persist across various segments of the population. This suggests that certain diseases may interact or co-occur consistently, irrespective of age, sex, or SES.
• Public health implications: Disease pairs present in multiple demographic groups may have broader public health implications. Understanding these commonalities can guide healthcare policies, resource allocation, and preventive measures that are applicable to a diverse range of individuals.
• Targeted interventions: Recognizing consistent disease pairs allows for the development of targeted interventions that address shared risk factors or mechanisms. It enables healthcare providers to implement more effective and tailored strategies for prevention, early detection, and management.
• Complex interactions: The presence of shared disease pairs across demographic groups may indicate complex interactions between diseases that go beyond individual factors. Investigating these interactions can provide insights into the underlying mechanisms and pathways leading to comorbidities.
• Longitudinal health monitoring: Tracking disease pairs across different life stages and demographics contributes to a more comprehensive understanding of health trajectories. It facilitates the identification of critical points where interventions can be most impactful in preventing the development of severe comorbidities.
• Reducing health disparities: Recognizing common disease pairs across diverse populations helps in addressing health disparities. It allows for a more equitable distribution of healthcare resources and interventions, aiming to reduce the disproportionate burden of certain diseases on specific demographic groups.
• Research and policy focus: Disease pairs that persist across age, sex, and SES groups may warrant further research attention and policy focus. They can serve as indicators for the need to explore underlying determinants, social factors, and genetic influences contributing to the observed patterns.
The identification of comorbidities potentially associated with sex and/or SES in each network involved the utilization of the Page Rank Score. This numerical measure allows us to pinpoint diseases with greater relevance within each network (1). The PRS enhances precision by considering the MI between pairs of diseases, thereby providing more detailed insights into comorbidity and multimorbidity phenomena in individuals.
4.1 Comorbidity networks: general observations
In examining the disparities across the analyzed networks, a noteworthy observation emerges: individuals with low SES generally exhibit a greater diversity of diseases, often double or more, compared to their high-SES counterparts. This pattern suggests that individuals with low SES face health inequalities, making them more susceptible to a broader spectrum of diseases. These diseases may either not occur in high-SES individuals or manifest differently, influenced by varying access to essential resources for their care—ranging from nutrition to healthcare services (34, 33, 32).
Moreover, the heightened diversity of diseases in the low-SES group may be linked to the greater density observed in high-SES networks. This increased density results in more connections between all diseases in high-SES networks. However, despite the higher number of connections, individuals with low SES exhibit a significantly higher number of comorbidity relationships, as evident from Table 1.
The finding of greater network centralization, particularly in the age range of 0–20 years—accentuated in high SES during these ages—may be attributed to specific comorbidities mediated by factors related to birth. This narrower diversity of diseases in high SES during these ages suggests distinct comorbidity patterns. Additionally, the greater number of diseases in low SES contributes to diversifying the conditions centralizing comorbidity relationships. Factors unique to low SES, such as overcrowding, nutrition, and structural conditions during infancy, expose individuals to different health challenges, potentially leading to varied patterns of comorbidity and multimorbidity in the short or long term (5, 36, 35).
Similarly, our analysis revealed that the average number of comorbidities, measured by the average number of neighbors in the different networks, is higher in older age groups compared to younger individuals (refer to Table 1). However, this trend is more pronounced in men than in women, as indicated by our results. Notably, in the population aged 80 and older, a decrease in average comorbidities is observed, which contradicts the prevailing literature on multimorbidity phenomena (37, 39, 38).
Among the most prevalent and clinically relevant conditions in various age groups, we consistently find Chronic kidney disease, unspecified (N18.9), Other specified congenital malformations of the heart (Q24.8), Other specified forms of chronic ischemic heart disease (I25.8), Heart failure, unspecified (I50.9), Unspecified atherosclerosis (I70.9), and Other specified cardiac arrhythmias (I49.8). This prevalence may stem from the interconnected nature of these conditions within the network, where several relationships involve equally significant diseases, influencing various physiological processes (1). However, it is crucial to note that the relevance of some of these conditions within the network may diminish or be absent in certain age groups, contingent on the SES of the patients, as we will discuss later.
This observation that younger patients of low socioeconomic status are more likely to have comorbidities than older subjects of higher SES raises relevant questions. Some of these issues may be related to limited access to healthcare, social determinants of health in early life, nutrition and lifestile factors, environmental factors, educational attainment and healthcare utilization patterns, among other constraints (41, 40). Since individuals with lower SES often face barriers in accessing healthcare services, including preventive care and early diagnosis, this may result in undiagnosed or untreated health conditions, contributing to the development of comorbidities. Also, early childhood experiences and SDHs, such as nutrition, access to quality education, and living conditions, significantly influence health outcomes later in life (42, 43). Younger patients from low SES backgrounds may have experienced adverse childhood conditions that contribute to the development of health issues and comorbidities. Younger individuals with lower SES may have limited access to healthy food options, leading to dietary habits that increase the risk of conditions such as obesity, diabetes, and cardiovascular diseases (44, 45). Living in socioeconomically disadvantaged neighborhoods can expose individuals to environmental factors that contribute to poor health outcomes. Environmental stressors, pollution, and lack of recreational spaces may impact the overall health of younger individuals from low SES backgrounds (46).
In summary, a complex interplay of socioeconomic, environmental, and lifestyle factors contributes to the observation that younger patients of low SES are more likely to have comorbidities. These factors highlight the importance of addressing social determinants of health and implementing interventions that promote health equity and access to comprehensive healthcare services for all individuals, regardless of socioeconomic status. Let us examine these complex comorbidity patterns in more detail.
4.2 Comorbidity networks in individuals aged 0–20 years
In this initial age range, our analysis of the PRS initially highlighted two diseases that could be associated with low SES. First, Unspecified heart failure (I50.9), listed only among the top five in high SES according to Table 2, was revealed through a deeper analysis to maintain a prominent position in low SES, ranking among the top ten most important. This suggests that it is not exclusive to the high SES population (47).
In contrast to Unspecified heart failure (I50.9), Chronic kidney disease unspecified (N18.9) predominantly affects men with low SES according to our results. There is evidence linking low SES to a predisposition to chronic diseases, including Chronic kidney disease unspecified (N18.9), either directly or as a consequence of preceding chronic diseases, with social determinants of health playing a crucial role (48, 49). While further investigation is necessary, factors such as education may be related, suggesting that in this age range, the influence of this factor could stem from the family nucleus where infants and adolescents develop (51, 50). Additionally, habits related to nutrition and lack of physical activity can impact the development of conditions closely related to N18.9, such as obesity (52, 53), a significant health issue in Mexico from an early age (54, 55).
It is worth noting that the presence of Chronic Kidney Disease unspecified (N18.9) in the early years of life is related to congenital malformations and glomerulopathies as the main known causes (57, 56). These conditions may be linked to factors inherent to urbanization, overpopulation, and hygiene, which can negatively impact certain biological processes and increase the risk of developing these diseases (58).
After the above and understanding that being Chronic Kidney Disease unspecified (N18.9) a condition that mostly affects low-SES men in this age range, its comorbidities will also may be specific to this population. Therefore, through an analysis of its first neighbors, we decided to examine its relationship with Systemic Lupus Erythematosus with organ or system involvement (M32.1), since they have a somehow direct relationship (59) and, in turn, it is also a disease that we can more commonly find in low-SES men (60, 61) according to our findings.
Given that Chronic Kidney Disease unspecified (N18.9) predominantly affects low-SES men in this age range, its comorbidities are likely specific to this population. Therefore, through an analysis of its first neighbors, we decided to explore its relationship with Systemic Lupus Erythematosus with organ or system involvement (M32.1), as they have a somewhat direct relationship (59). Moreover, it is also a disease more commonly found in low-SES men (60, 61) according to our findings.
Regarding this pair of diseases, lupus erythematosus tends to affect various vital organs, and although less frequent in children, it is more severe than in adults, with kidney disease present in 50%–90% of patients. Therefore, the close relationship between these conditions within the network is not surprising. The association of M32.1 with low SES may be attributed to the condition’s multifactorial nature, involving genetic and environmental factors. Recurrent infections are also known risk factors for triggering the onset of the disease, with these types of infections more prevalent in families where young children live with school-aged children. Such situations are characteristic of overcrowded environments where multiple families coexist, a scenario more common among individuals with low SES. Premature or low birth weight babies, found more frequently in low-SES settings, are also significant factors in recurrent infections (62).
Conversely, in women, Chronic Kidney Disease unspecified (N18.9) does not exhibit different impacts by SES, according to our data. This suggests that differences in its occurrence by SES may be less pronounced in this population, although further research is needed to confirm this. Additionally, the relationship with Systemic Lupus Erythematosus with organ or system involvement (M32.1) is present irrespective of SES. This may be related to the fact that women are more predisposed to developing M32.1. However, it’s essential to consider that both diseases are linked to cardiovascular system issues, influenced by biological and lifestyle factors, aligning with the context of the data from which these networks were modeled. Therefore, other associated variables need to be considered to ascertain the significance of SES in the concurrent occurrence of these conditions in men.
4.3 Comorbidity networks in individuals aged 21–40 years
In the networks specific to individuals aged 21–40 years, we observe that although the same diseases maintain their top positions according to their Importance Page Rank, the relationships some diseases have with their first neighbors vary. An illustrative example is the case of Other specified congenital malformations of the heart (Q24.8), which, exclusively in low SES for both men and women, retains a first-neighbor relationship with Chronic kidney disease, unspecified (N18.9) and Other forms of chronic ischemic heart disease (I25.8), a relationship suggested in previous literature (64, 63).
The multifactorial etiology of Q24.8 as a congenital malformation implies potential influences from genetic and maternal factors during pregnancy, including maternal health conditions such as diabetes, hypertension, and obesity (67, 65, 66, 68, 69). On the other hand, N18.9 and I25.8 share common factors associated with chronic diseases, such as unhealthy diets and sedentary lifestyles, believed to be more prevalent in low SES populations (70–72).
Thus, the shared social determinants of these three diseases in low SES, including the presence of unhealthy habits, a family history of chronic diseases, and limited access to healthcare, could contribute to their co-occurrence in this population. While further research is necessary to confirm this relationship, the current study highlights the significant association between the concurrent occurrence of these diseases and the mutual information they share in their respective networks.
A more specific and noteworthy case pertains to the association between Chronic Kidney Disease, unspecified (N18.9) and Other specified chronic ischemic heart disease (I25.8), observed exclusively in the men network of low SES individuals within this age range. This relationship is characterized by a significant MI score of 0.021196, indicative of its clinical relevance as a comorbidity in this population (74, 73). The co-occurrence of these two diseases is anticipated due to the well-established predisposition of kidney disease to cardiovascular conditions, with I25.8 being a notable example. Furthermore, the incidence of I25.8 is known to be age- and sex-related, with a lower likelihood of development among women of childbearing age due to the protective effect of sex hormones (75). In this context, an intermediate disease, Other specified congenital malformations of the heart (Q24.8), could partially explain the observed association between N18.9 and I25.8. However, additional research is necessary to confirm this hypothesis. Notably, the exclusive appearance of this comorbidity in the low SES men network is likely linked to shared structural and lifestyle factors discussed earlier.
4.4 Comorbidity networks in individuals aged 41–60 years
The most relevant conditions within the networks for high SES remain consistent in the top five positions for both men and women. There are striking similarities in the lower SES, where Chronic kidney disease, unspecified (N18.9) replaces Heart failure, unspecified (I50.9). Nevertheless, the latter remains among the top ten most important conditions (i.e., high Page Rank Score) according to our results. Hence, among the most significant differences observed in this age range, it is notable that men, irrespective of SES, exhibit a first-neighbor relationship among all diseases included in the top five networks for men in this age range (see Table 4). In contrast, women, as per our data, manifest different configurations contingent on their SES. Notably, only a direct relationship between Atherosclerosis, unspecified (I70.9) and Chronic kidney disease, unspecified (N18.9) is evident in women of high SES. This pairing becomes noteworthy because it is the sole age range where a discrepancy surfaces concerning sex and SES. It suggests that solely women of high SES exhibit this comorbidity at earlier ages than those of low SES (76), unlike men who experience this comorbidity in both SES.
Regarding this pair of diseases, it is established that Chronic kidney disease (N18.9) tends to foster the development of cardiovascular diseases, including Atherosclerosis (I70.9), due to deficiencies intrinsic to renal deterioration and its association with the cardiovascular system. This association becomes more prominent in advanced stages of renal disease (78, 77), underscoring the close relationship between both conditions. The differentiated appearance in women based on SES, affecting initially women of high SES, is a counterintuitive phenomenon. Typically, chronic diseases are anticipated to emerge earlier or with more substantial impact in low SES populations due to the interplay of various factors inherent in low SES (81, 82, 80, 79). Further research is warranted to comprehensively understand this phenomenon.
4.5 Comorbidity networks in individuals aged 61–80 years old
Regarding this age range, in general, the diseases that occupy the top positions in each network remain constant, according to their PRS, presenting some changes in terms of their level of relevance according to Table 5. That being said, we analyzed how the most relevant diseases were organized with others, present in the aforementioned table with the highest PRS, which showed us that Rheumatic diseases of endocardial valve unspecified (I09.1) only appears significantly connected to Other specified congenital malformations of heart (Q24.8) in low SES men (83, 84). While this association may be expected given that cardiac malformations could contribute to the development of I09.1, the exclusive appearance of this relationship in men of low SES is noteworthy.
In addition to the presence of a cardiac malformation, other risk factors that are associated with I09.1, such as poor oral health and hygiene (85, 86) or injectable drug use (87), may increase the likelihood of co-occurrence between these diseases in individuals of low SES. Studies have linked poor dental hygiene to low SES, which may result from limited access to education or health services (88, 90, 89). Likewise, low SES has been associated with higher drug consumption, possibly due to factors such as education, family background, place of residence, and social relationships (91). Injectable drug use has been specifically linked to poverty and unemployment, although this relationship requires further investigation (92).
As can be seen in the previous paragraph, the joint presence of the aforementioned conditions, despite being influenced by biological factors, the weight in the occurrence of Unspecified rheumatic diseases of endocardial valve (I09.1) may be falling on the conditions that people go through throughout their development, giving rise to generating comprehensive interventions in the treatment and care of patients with congenital malformations. These interventions should not only focus on medical treatment, but also on the care of vulnerable groups, in order to reduce the gaps in inequality that could be influencing why both conditions affect more people with low SES (84, 93).
4.6 Comorbidity networks in individuals aged 80 years and older
In this population, we found that despite the fact that the most relevant diseases in the different networks remain constant, the relationships they form between them can be different. An example of this is the one presented by Acute transmural anterior wall myocardial infarction (I21.0) and Unspecified rheumatic valve diseases (I09.1), as they only showed a relationship in low-SES women in this age range (95, 94, 93).
Regarding this pair, the literature generally indicates that Acute transmural anterior wall myocardial infarction (I21.0) is a rare or infrequent complication in patients with Unspecified rheumatic valve diseases (I09.1), occurring in the acute phase of the disease, where coronary embolism is related to bacterial endocarditis, thus causing an acute myocardial infarction (96). This helps us to confirm that there may be biological processes involved in the co-occurrence of these two conditions, but leaves their relationship with low SES up in the air, so it is necessary to continue investigating this topic and also to analyze why it appears more frequently in women since, as mentioned above (97), both diseases have a strong and well-positioned relationship according to our results. This could become more important if we take into account that mortality from Acute transmural anterior wall myocardial infarction (I21.0) increases directly with people’s age (98), and according to our results, both are very well-positioned diseases in the network of women over 80 years old according to their PRS (Table 6).
4.7 Cardiovascular comorbidity in the context of social determinants of health
Social determinants of health are the conditions in which people are born, grow, live, work, and age, and they play a crucial role in shaping health outcomes. These determinants are influenced by the distribution of money, power, and resources at global, national, and local levels. The main SDHs include socioeconomic stutus, education, employment and working conditions, social support networks, healthcare access and quality, physical environment, social and economic policies, cultural and social norms, early childhood experiences and behavioral factors. Understanding and addressing these social determinants are essential for developing effective public health policies and interventions aimed at improving overall health and reducing health disparities (99).
In the present context, our findings just presented point out to some general trends. More specific issues may be found by using the comorbidity network to navigate on local hospital EHRs that include personal patient data. Since that data is to be protected by confidentiality and ethical constraints here we will focus on the general associations. For instance, notable associations with age include congenital heart malformations in younger age groups and an increased prevalence of conditions like chronic kidney disease and atherosclerosis in older age groups. Distinct patterns are observed in men and women, such as the relationship between chronic kidney disease and atherosclerosis being significant in women but more nuanced in men. Differences in disease associations are identified based on SES, highlighting conditions more prevalent in low SES populations, such as chronic kidney disease and rheumatic diseases. In terms of multifactor risks, study delves into the intersectionality of age, sex, and SES, examining how these factors collectively shape cardiovascular comorbidity patterns.
Our findings reveal nuanced relationships, such as the appearance of specific comorbidities in women of high SES at earlier ages, emphasizing the need for a comprehensive understanding of social determinants’ interplay. The identified comorbidity patterns offer insights into potential interventions that consider the social determinants of health. Addressing structural factors like oral health, hygiene, and drug use may be crucial for managing specific comorbidities associated with low SES. Comprehensive interventions focusing on vulnerable groups, especially those with congenital malformations or several risk-related SDHs, are proposed to reduce health inequalities. The study underscores the necessity for continued research to deepen the understanding of the observed relationships, especially those involving age, sex, and SES.
It is relevant however to point out that in this work, statistically significant associations are presented, but no causal or mechanistic explanations have been developed. Rather our study aims to be a starting point to study these, as well as a tool to inform hospital management and public health officials to help them in planning and policy development if possible.
4.8 Summary of findings
In what follows we will summarize the more relevant, general results observed by examining the comorbidity and multimorbidity patterns. These global (in the context of our analyzed populations) trends may help contextualize the highly variable landscape of cardiovascular comorbidity presented in this study and available at the Supplementary materials (i.e., the whole set of CVC networks).
• Comorbidity networks in people aged 0–20 years old
• Unspecified Heart Failure (I50.9)
• Initially associated with high SES but remains important in low SES.
• Indicates it is not exclusive to high SES populations.
• Chronic Kidney Disease Unspecified (N18.9)
• Primarily affects low-SES men.
• Linked to low SES through factors like education, nutrition, and lifestyle.
• Relationship with Systemic Lupus Erythematosus (M32.1) explored.
• Chronic Kidney Disease (N18.9) and Systemic Lupus Erythematosus (M32.1)
• Lupus affects vital organs, often co-occurs with kidney disease.
• Shared risk factors include infections, crowded living conditions, and low SES.
• Comorbidity networks in people aged 21–40 years old
• Other Specified Congenital Malformations of the Heart (Q24.8)
• Connected to Chronic Kidney Disease Unspecified (N18.9) and Other Forms of Chronic Ischemic Heart Disease (I25.8).
• Shared social determinants, including unhealthy habits and limited healthcare access.
• Chronic Kidney Disease (N18.9) and Other Forms of Chronic Ischemic Heart Disease (I25.8)
• Specific to low-SES men.
• Reflects the known association between kidney disease and cardiovascular conditions.
• Comorbidity networks in people aged 41–60 years old
• Chronic Kidney Disease Unspecified (N18.9)
• Replaces Unspecified Heart Failure (I50.9) in high SES.
• Key comorbidity with Atherosclerosis (I70.9) in high-SES women.
• Suggests SES-related differences in disease patterns.
• Comorbidity networks in people aged 61–80 years old
• Rheumatic Diseases of Endocardial Valve Unspecified (I09.1)
• Connected to Other Specified Congenital Malformations of the Heart (Q24.8) in low-SES men.
• Factors include cardiac malformations, poor oral health, and injectable drug use.
• Comorbidity networks in people aged 80 years and older
• Acute Transmural Anterior Wall Myocardial Infarction (I21.0) and Unspecified Rheumatic Valve Diseases (I09.1)
• Relationship observed in low-SES women.
• Rare complication, biological processes involved.
• Need for further investigation into SES-related and gender-related aspects.
4.9 Relation to other studies
Mapping the comorbidity and multimorbidity landscape of cardiovascular diseases has been an issue of interest in the international medical and biomedical research community for some time. Different approaches parallel and complementary to what we have just presented have been developed. These efforts span from the highly specific to the very broad approaches. One quite relevant example of the latter is MorbiNet, a Spanish study that analyzes a very large population consisting of 3,135,948 adult people in Catalonia, Spain. This work also mined EHRs but is focused exclusively in the relationship between common chronic conditions and type 2 diabetes (100). MorbiNet, as the present work is a network-based approach; there the authors build networks from odds-ratio estimates adjusted by age and sex and considered connections those conditions with odds ratios larger than 1.2 and Bonferroni-adjusted logistic regression p-values less than 1E-5. This work reports that the more connected conditions in T2DM undirected network were: complicated hypertension and atherosclerosis/peripheral vascular disease, cholecystitis/cholelithiasis, retinopathy and peripheral neuritis/neuropathy. The strongest associations from T2DM directed networks were to retinopathy (OR: 23.8), glomerulonephritis/nephrosis (OR: 3.4), peripheral neuritis/neuropathy (OR: 2.7) and pancreas cancer (OR: 2.4). Though their methods are in some sense similar to ours, there are some noticeable differences. Perhaps the most evident is that due to the large scale of their study they focus on common chronic diseases, somehow regardless of the outcomes and mainly related to one (admittedly extremely important) condition, type 2 diabetes. Also, their networks are unweighted, meaning that every comorbidity relationship above the significance threshold contributes to the comorbidity landcape on a similar fashion, whereas in our case, every comorbidity relationship is characterized by a mutual information value representing the relative strength of this association.
Though not exactly a comorbidity analysis, the framework to study cardiovascular diseases from the standpoint of network science as presented by Lee and coworkers is worth mentioning (101). There, the authors establish a set of basic network theory principles that allowed them to look up to disease-disease interactions, uncovering disease mechanisms, and even allow for clinical risk stratification and biomarker discovery. A simialr approach is sketched by Benincassa and collaborators (102) though the scope is more limited to uncover disease modules.
A hybrid network analytics/classical epidemiology approach is presented by Haug et al. (103). They analyzed multimorbidity patterns, representing groups of included or excluded diseases, delineated the health states of patients in a population-wide analysis spanning 17 years and encompassing 9,000,000 patient histories of hospital diagnoses (a data set provided by the Austrian Federal Ministry for Health, covering all approx. 45,000,000 hospital stays of about 9,000,000 individuals in Austria during the 17 years from 1997 to 2014.). These patterns encapsulate the evolving health trajectories of patients, wherein new diagnoses acquired over time alter their health states. Their study assesses age- and sex-specific risks for patients to acquire specific sets of diseases in the future based on their current health state. The population studied is characterized by 132 distinct multimorbidity patterns. Among elderly patients, three groups of multimorbidity patterns are identified, each associated with low (yearly in-hospital mortality of 0.2%–0.3%), medium (0.3%–1%), and high in-hospital mortality (2%–11%). Combinations of diseases that significantly elevate the risk of transitioning into high-mortality health states in later life are identified. For instance, in men (women) aged 50–59 with diagnoses of diabetes and hypertension, the risk of entering the high-mortality region within one year is elevated by a factor of 1.96 ± 0.11 (2.60 x00B1; 0.18) compared to all patients of the same age and sex, respectively. This risk increases further to a factor of 2.09 ± 0.12 (3.04 ± 0.18) if they are additionally diagnosed with metabolic disorders. This study is simialr to ours in the sense that was not limited for particular diagnosis (though only considered 1,074 codes from A00 to N99, grouped into 131 blocks as defined by the WHO, which excludes congenital diseases that are quite relevant for children and young individuals) and it was based on mining ICD-10 codes from the EHRs. Their emphasis however, is different from ours since thay are more interested in patient trajectories which describe the health state of this patient at different points in time, rather than in general trends useful for hospital management.
4.10 Limitations of the present study
This study utilizes ICD-10 codes to document and classify disease conditions. It is important to note that the use of ICD-10 codes in research presents challenges and limitations, as the system was primarily developed for hospital administration and cost-estimation purposes, rather than as a controlled vocabulary for standardized clinical reporting or epidemiological research (1). Concerns about the suitability of ICD codes for other secondary purposes, such as research or policy interventions, have been raised due to coding errors found in patient data by some authors (105, 106, 104, 107).
The validity of ICD codes to identify specific conditions depends on the extent to which the condition contributes to health service use, as well as the time, place, and method of data collection (108). Diagnostic accuracy tests of ICD-10 codes have been conducted to evaluate features such as sensitivity, specificity, positive predictive values (PPV), and negative predictive values (NPV) for specific major diagnoses, major procedures, minor procedures, ambulatory diagnoses, co-existing conditions, and death status. These studies have generally found good-to-excellent coding quality for ICD-10 codes in these areas (1).
Given these considerations, when using these codes for clinical purposes, careful evaluation is necessary since the actual subjects of interest may not be accurately defined. This may be critical in the assessment of chronic conditions. Moreover, ICD codes perform better with sets of diseases enriched for frequent, well-known conditions.
It is noteworthy that in the specific case of Electronic Health Records in the NICICH, the administrative database coding, archiving, and retrieval procedures have been certified and validated by the World Health Organization (WHO) through the local ‘Collaborating Center for WHO International Classification Schemes - Mexico Chapter’ (CEMECE, for its Spanish acronym). These procedures are in agreement with ISO 9001:2000, ISO/IEC 27001 certifications, and with the Official Mexican Norm (NOM for its Spanish acronym): NOM-004-SSA3-2012 (1).
5 Concluding remarks
In conclusion, the analysis of comorbidity networks across different age groups and socioeconomic status reveals interesting patterns in disease co-occurrence. There are consistent associations between certain diseases, and these associations may vary based on age and SES. Moreover, the presence of certain comorbidities differ between men and women and across different age and SES, as expected.
Some diseases, such as chronic kidney disease and specific cardiac conditions, consistently appear among the most relevant comorbidities across age groups and SES. Additionally, the study highlights specific associations, such as the relationship between unspecified heart failure, chronic kidney disease, and systemic lupus erythematosus with organ involvement, which may have implications for diagnostic and therapeutic strategies.
Notably, the findings also suggest that individuals with low SES tend to exhibit a greater diversity of diseases, potentially indicating disparities in health outcomes and access to healthcare resources. The importance of social determinants of health in shaping comorbidity patterns is evident, emphasizing the need for comprehensive interventions that address not only medical aspects but also social and environmental factors.
Overall, this study provides valuable insights into the complex landscape of comorbidities, shedding light on how age, sex, and SES contribute to the interconnected web of diseases. Further research and ongoing investigation are crucial to deepen our understanding of these relationships and inform more targeted and effective approaches to healthcare and disease prevention.
Data availability statement
The data analyzed in this study is subject to the following licenses/restrictions: Data was taken from annonymized Electronic Health Records from the National Institute of Cardiology Ignacio Chavez. Data summaries are available upon request. Requests to access these datasets should be directed tobWlyZXlhLm1hcnRpbmV6QGNhcmRpb2xvZ2lhLm9yZy5teA==.
Author contributions
EHL conceived the project, EHL and MMG directed and supervised the project, MMG and EHL designed and develop the computational strategy, EHL, MMG, FRA and HACA implemented the code and database search procedures, MMG, HACA, FRA and EHL conducted the calculations and validation, MMG, HACA, FRA and EHL analysed the results. MMG and EHL wrote the manuscript. All authors contributed to the article and approved the submitted version.
Acknowledgments
The authors want to thank Rosalinda Altamira-Mendoza and Lucía Ríos-Núñez from the Deputy Direction for Planning at the National Institute of Cardiology ’Ignacio Chavez’ for their helpful advice on EHR data management. This work was supported by federal funding from the National Institute of Genomic Medicine (Mexico).
Conflict of interest
The authors declare that the research was conducted in the absence of any commercial or financial relationships that could be construed as a potential conflict of interest.
Publisher's note
All claims expressed in this article are solely those of the authors and do not necessarily represent those of their affiliated organizations, or those of the publisher, the editors and the reviewers. Any product that may be evaluated in this article, or claim that may be made by its manufacturer, is not guaranteed or endorsed by the publisher.
Supplementary material
The Supplementary Material for this article can be found online at: https://www.frontiersin.org/articles/10.3389/fcvm.2024.1215458/full#supplementary-material
References
1. Cruz-Ávila HA, Vallejo M, Martínez-García M, Hernández-Lemus E. Comorbidity networks in cardiovascular diseases. Front Physiol. (2020) 11:1009. doi: 10.3389/fphys.2020.01009
2. Safford MM, Allison JJ, Kiefe CI. Patient complexity: more than comorbidity. the vector model of complexity. J Gen Intern Med. (2007) 22:382–90. doi: 10.1007/s11606-007-0307-0
3. Ng SK, Holden L, Sun J. Identifying comorbidity patterns of health conditions via cluster analysis of pairwise concordance statistics. Stat Med. (2012) 31:3393–405. doi: 10.1002/sim.5426
4. Faner R, Cruz T, López-Giraldo A, Agustí A. Network medicine, multimorbidity, the lung in the elderly. Eur Respir J. (2014) 44:775–88. doi: 10.1183/09031936.00078714
5. Violan C, Foguet-Boreu Q, Flores-Mateo G, Salisbury C, Blom J, Freitag M, et al. Prevalence, determinants, patterns of multimorbidity in primary care: a systematic review of observational studies. PLoS One. (2014) 9:e102149. doi: 10.1371/journal.pone.0102149
6. Birke H, Jacobsen R, Jønsson AB, Guassora ADK, Walther M, Saxild T, et al. A complex intervention for multimorbidity in primary care: a feasibility study. J Comorb. (2020) 10:2235042X20935312. doi: 10.1177/2235042X20935312
7. Wang L, Cocker F, Kilpatrick M, Otahal P, Si L, Palmer AJ, et al. The associations of multimorbidity with health-related productivity loss in a large and diverse public sector setting: a cross-sectional survey. J Occup Environ Med. (2018) 60:528–35. doi: 10.1097/JOM.0000000000001243
8. Barnett K, Mercer SW, Norbury M, Watt G, Wyke S, Guthrie B. Epidemiology of multimorbidity and implications for health care, research, and medical education: a cross-sectional study. Lancet. (2012) 380:37–43. doi: 10.1016/S0140-6736(12)60240-2
9. Biswas T, Townsend N, Islam MS, Islam MR, Gupta RD, Das SK, et al. Association between socioeconomic status and prevalence of non-communicable diseases risk factors and comorbidities in Bangladesh: findings from a nationwide cross-sectional survey. BMJ Open. (2019) 9:e025538. doi: 10.1136/bmjopen-2018-025538
10. Carvalho JN, Roncalli ÂG, Cancela MC, Souza DLB. Prevalence of multimorbidity in the Brazilian adult population according to socioeconomic and demographic characteristics. PLoS One. (2017) 12:e0174322. doi: 10.1371/journal.pone.0174322
11. Jensen NL, Pedersen HS, Vestergaard M, Mercer SW, Glümer C, Prior A. The impact of socioeconomic status and multimorbidity on mortality: a population-based cohort study. Clin Epidemiol. (2017) 9:279. doi: 10.2147/CLEP.S129415
12. Mair FS, Jani BD. Emerging trends and future research on the role of socioeconomic status in chronic illness and multimorbidity. Lancet Public Health. (2020) 5:e128–9. doi: 10.1016/S2468-2667(20)30001-3
13. Landi F, Onder G, Cesari M, Barillaro C, Lattanzio F, Carbonin PU, et al. Comorbidity and social factors predicted hospitalization in frail elderly patients. J Clin Epidemiol. (2004) 57:832–6. doi: 10.1016/j.jclinepi.2004.01.013
14. Low LL, Wah W, Ng MJ, Tan SY, Liu N, Lee KH. Housing as a social determinant of health in Singapore and its association with readmission risk and increased utilization of hospital services. Front Public Health. (2016) 4:109. doi: 10.3389/fpubh.2016.00109
15. Vinjerui KH, Boeckxstaens P, Douglas KA, Sund ER. Prevalence of multimorbidity with frailty and associations with socioeconomic position in an adult population: findings from the cross-sectional HUNT Study in Norway. BMJ Open. (2020) 10:e035070. doi: 10.1136/bmjopen-2019-035070
16. Formiga F, Ferrer A, Sanz H, Marengoni A, Alburquerque J, Pujol R, et al. Patterns of comorbidity and multimorbidity in the oldest old: the Octabaix study. Eur J Intern Med. (2013) 24:40–4. doi: 10.1016/j.ejim.2012.11.003
17. Vetrano DL, Palmer K, Marengoni A, Marzetti E, Lattanzio F, Roller-Wirnsberger R, et al. Frailty and multimorbidity: a systematic review and meta-analysis. J Gerontol Ser A. (2019) 74:659–66. doi: 10.1093/gerona/gly110
18. Möller-Leimkühler AM. Gender differences in cardiovascular disease and comorbid depression. Dialogues Clin Neurosci. (2007) 9:71. doi: 10.31887/DCNS.2007.9.1/ammoeller
19. Nurmohamed MT, Heslinga M, Kitas GD. Cardiovascular comorbidity in rheumatic diseases. Nat Rev Rheumatol. (2015) 11:693–704. doi: 10.1038/nrrheum.2015.112
20. King DE, Xiang J, Pilkerton CS. Multimorbidity trends in united states adults, 1988–2014. J Am Board Fam Med. (2018) 31:503–13. doi: 10.3122/jabfm.2018.04.180008
21. Roman Lay AA, Ferreira do Nascimento C, Caba Burgos F, Larraín Huerta Ad.C, Rivera Zeballos RE, Pantoja Silva V, et al. Gender differences between multimorbidity and all-cause mortality among older adults. Curr Gerontol Geriatr Res. (2020) 2020:1–7. doi: 10.1155/2020/7816785
23. Brin S, Page L. The anatomy of a large-scale hypertextual web search engine. Comput Netw ISDN Syst. (1998) 30:107–17. doi: 10.1016/S0169-7552(98)00110-X
24. Doncheva NT, Assenov Y, Domingues FS, Albrecht M. Topological analysis and interactive visualization of biological networks and protein structures. Nat Protoc. (2012) 7:670. doi: 10.1038/nprot.2012.004
25. Kohl M, Wiese S, Warscheid B. Cytoscape: software for visualization and analysis of biological networks. In: Data Mining in Proteomics. Springer (2011). p. 291–303.
26. Tang Y, Li M, Wang J, Pan Y, Wu F-X. CytoNCA: a cytoscape plugin for centrality analysis and evaluation of protein interaction networks. Biosystems. (2015) 127:67–72. doi: 10.1016/j.biosystems.2014.11.005
27. Bastian M, Heymann S, Jacomy M. Gephi: an open source software for exploring and manipulating networks. In: Proceedings of the International AAAI Conference on Web and Social Media. (2009). Vol. 3, p. 361–362.
28. Niaudet P. Treatment of lupus nephritis in children. Pediatr Nephrol. (2000) 14:158–66. doi: 10.1007/s004670050034
29. Oni L, Wright RD, Marks S, Beresford MW, Tullus K. Kidney outcomes for children with lupus nephritis. Pediatr Nephrol. (2021) 36:1377–85. doi: 10.1007/s00467-020-04686-1
30. Perfumo F, Martini A. Lupus nephritis in children. Lupus. (2005) 14:83–8. doi: 10.1191/0961203305lu2065oa
31. Yang L-Y, Chen W-P, Lin C-Y. Lupus nephritis in children—a review of 167 patients. Pediatrics. (1994) 94:335–40. doi: 10.1542/peds.94.3.335
32. Boutayeb A, Boutayeb S, Boutayeb W. Multi-morbidity of non communicable diseases and equity in WHO Eastern Mediterranean countries. Int J Equity Health. (2013) 12:1–13. doi: 10.1186/1475-9276-12-60
33. Roberts K, Rao D, Bennett T, Loukine L, Jayaraman G. Prevalence and patterns of chronic disease multimorbidity and associated determinants in Canada. Health Promot Chron Dis Prev Can Res Policy Pract. (2015) 35:87. doi: 10.24095/hpcdp.35.6.01
34. Uijen AA, van de Lisdonk EH. Multimorbidity in primary care: prevalence and trend over the last 20 years. Eur J Gen Pract. (2008) 14:28–32. doi: 10.1080/13814780802436093
35. Amell A, Roso-Llorach A, Palomero L, Cuadras D, Galván-Femenía I, Serra-Musach J, et al. Disease networks identify specific conditions and pleiotropy influencing multimorbidity in the general population. Sci Rep. (2018) 8:15970. doi: 10.1038/s41598-018-34361-3
36. Malecki SL, Van Mil S, Graffi J, Breetvelt E, Corral M, Boot E, et al. A genetic model for multimorbidity in young adults. Genet Med. (2020) 22:132–41. doi: 10.1038/s41436-019-0603-1
37. Foscolou A, Chrysohoou C, Dimitriadis K, Masoura K, Vogiatzi G, Gkotzamanis V, et al. The association of healthy aging with multimorbidity: IKARIA study. Nutrients. (2021) 13:1386. doi: 10.3390/nu13041386
38. Moin JS, Moineddin R, Upshur REG. Measuring the association between marginalization and multimorbidity in Ontario, Canada: a cross-sectional study. J Comorb. (2018) 8:2235042X18814939. doi: 10.1177/2235042X18814939
39. Ryan B, Allen B, Zwarenstein M, Stewart M, Glazier R, Fortin M, et al. Multimorbidity and mortality in Ontario, Canada: a population-based retrospective cohort study. J Comorb. (2020) 10:2235042X20950598. doi: 10.1177/2235042X20950598
40. Haider A, Bengs S, Luu J, Osto E, Siller-Matula JM, Muka T, et al. Sex and gender in cardiovascular medicine: presentation and outcomes of acute coronary syndrome. Eur Heart J. (2020) 41:1328–36. doi: 10.1093/eurheartj/ehz898
41. Kristensen PL, Wedderkopp N, Møller NC, Andersen LB, Bai CN, Froberg K. Tracking and prevalence of cardiovascular disease risk factors across socio-economic classes: a longitudinal substudy of the European Youth Heart Study. BMC Public Health. (2006) 6:1–9. doi: 10.1186/1471-2458-6-20
42. Mannoh I, Hussien M, Commodore-Mensah Y, Michos ED. Impact of social determinants of health on cardiovascular disease prevention. Curr Opin Cardiol. (2021) 36:572–9. doi: 10.1097/HCO.0000000000000893
43. Suglia SF, Campo RA, Brown AG, Stoney C, Boyce CA, Appleton AA, et al. Social determinants of cardiovascular health: early life adversity as a contributor to disparities in cardiovascular diseases. J Pediatr. (2020) 219:267–73. doi: 10.1016/j.jpeds.2019.12.063
44. Lloyd-Jones DM, Ning H, Labarthe D, Brewer L, Sharma G, Rosamond W, et al. Status of cardiovascular health in US adults and children using the American Heart Association’s new “Life’s Essential 8” metrics: prevalence estimates from the National Health and Nutrition Examination Survey (NHANES), 2013 through 2018. Circulation. (2022) 146:822–35. doi: 10.1161/CIRCULATIONAHA.122.060911
45. Parcha V, Patel N, Kalra R, Arora G, Arora P. Prevalence, awareness, treatment, and poor control of hypertension among young American adults: race-stratified analysis of the national health and nutrition examination survey. In: Mayo Clinic Proceedings. Elsevier (2020). Vol. 95. p. 1390–1403.
46. Najman JM, Wang W, Plotnikova M, Mamun AA, McIntyre D, Williams GM, et al. Poverty over the early life course and young adult cardio-metabolic risk. Int J Public Health. (2020) 65:759–68. doi: 10.1007/s00038-020-01423-1
47. Ramsay SE, Whincup PH, Papacosta O, Morris RW, Lennon LT, Goya Wannamethee S. Inequalities in heart failure in older men: prospective associations between socioeconomic measures and heart failure incidence in a 10-year follow-up study. Eur Heart J. (2014) 35:442–7. doi: 10.1093/eurheartj/eht449
48. Marinovich S, Lavorato C, Rosa-Diez G, Bisigniano L, Fernández V, Hansen-Krogh D. The lack of income is associated with reduced survival in chronic haemodialysis. Nefrología (English Edition). (2012) 32:79–88. doi: 10.3265/Nefrologia.pre2011.Nov.11110
49. Novick TK, Rizzolo K, Cervantes L. COVID-19 and kidney disease disparities in the United States. Adv Chronic Kidney Dis. (2020) 27:427–33. doi: 10.1053/j.ackd.2020.06.005
50. Crews DC, Gutiérrez OM, Fedewa SA, Luthi J-C, Shoham D, Judd SE, et al. Low income, community poverty and risk of end stage renal disease. BMC Nephrol. (2014) 15:1–9. doi: 10.1186/1471-2369-15-192
51. Thio CH, Vart P, Kieneker LM, Snieder H, Gansevoort RT, Bültmann U. Educational level and risk of chronic kidney disease: longitudinal data from the PREVEND study. Nephrol Dial Transplant. (2020) 35:1211–8. doi: 10.1093/ndt/gfy361
52. Lakkis JI, Weir MR. Obesity and kidney disease. Prog Cardiovasc Dis. (2018) 61:157–67. doi: 10.1016/j.pcad.2018.07.005
53. Singh AK, Kari JA. Metabolic syndrome and chronic kidney disease. Curr Opin Nephrol Hypertens. (2013) 22:198–203. doi: 10.1097/MNH.0b013e32835dda78
54. Pérez-Herrera A, Cruz-López M. Childhood obesity: current situation in Mexico. Nutr Hosp. (2019) 36:463–9. doi: 10.20960/nh.2116
55. Turnbull B, Gordon SF, Martínez-Andrade GO, González-Unzaga M. Childhood obesity in Mexico: a critical analysis of the environmental factors, behaviours and discourses contributing to the epidemic. Health Psychol Open. (2019) 6:2055102919849406. doi: 10.1177/2055102919849406
56. Eales JM, Jiang X, Xu X, Saluja S, Akbarov A, Cano-Gamez E, et al. Uncovering genetic mechanisms of hypertension through multi-omic analysis of the kidney. Nat Genet. (2021) 53:630–7. doi: 10.1038/s41588-021-00835-w
57. Elhassan EA, Murray SL, Connaughton DM, Kennedy C, Cormican S, Cowhig C, et al. The utility of a genetic kidney disease clinic employing a broad range of genomic testing platforms: experience of the Irish Kidney Gene Project. J Nephrol. (2022) 35:1655–65. doi: 10.1007/s40620-021-01236-2
58. Skrzypczyk P, Okarska-Napierała M, Stelmaszczyk-Emmel A, Górska E, Pańczyk-Tomaszewska M. Renalase in children with chronic kidney disease. Biomarkers. (2019) 24:638–44. doi: 10.1080/1354750X.2019.1642957
59. Wakiguchi H, Takei S, Kubota T, Miyazono A, Kawano Y. Treatable renal disease in children with silent lupus nephritis detected by baseline biopsy: association with serum C3 levels. Clin Rheumatol. (2017) 36:433–7. doi: 10.1007/s10067-016-3491-7
60. Contreras GN, Lenz O, Pardo V, Borja E, Cely CM, Iqbal K, et al. Outcomes in African Americans and Hispanics with lupus nephritis. Kidney Int. (2006) 69(10):1846–51. doi: 10.1038/sj.ki.5000243
61. Mok M, Li W. Do asian patients have worse lupus? Lupus. (2010) 19:1384–90. doi: 10.1177/0961203310375832
62. Barber MR, Drenkard C, Falasinnu T, Hoi A, Mak A, Kow NY, et al. Global epidemiology of systemic lupus erythematosus. Nat Rev Rheumatol. (2021) 17:515–32. doi: 10.1038/s41584-021-00668-1
63. Dimopoulos K, Diller G-P, Koltsida E, Pijuan-Domenech A, Papadopoulou SA, Babu-Narayan SV, et al. Prevalence, predictors, and prognostic value of renal dysfunction in adults with congenital heart disease. Circulation. (2008) 117:2320–8. doi: 10.1161/CIRCULATIONAHA.107.734921
64. Noori NM, Sadeghi S, Shahramian I, Keshavarz K. Urine β 2-microglobolin in the patients with congenital heart disease. Int Cardiovasc Res J. (2013) 7:62–6.24757623
65. Bateman BT, Huybrechts KF, Fischer MA, Seely EW, Ecker JL, Oberg AS, et al. Chronic hypertension in pregnancy and the risk of congenital malformations: a cohort study. Am J Obstet Gynecol. (2015) 212(3):337.e1–14. doi: 10.1016/j.ajog.2014.09.031
66. Blomberg M, Källén B. Maternal obesity and morbid obesity: the risk for birth defects in the offspring. Birth Defects Res Part A Clin Mol Teratol. (2009) 88(1):35–40. doi: 10.1002/bdra.20620
67. Chou H-H, Chiou M, Liang F-W, Chen L-H, Lu T-H, Li C-Y. Association of maternal chronic disease with risk of congenital heart disease in offspring. Can Med Assoc J. (2016) 188:E438–46. doi: 10.1503/cmaj.160061
68. Lisowski LA, Verheijen PM, Copel J, Kleinman CS, Wassink S, Visser GHA, et al. Congenital heart disease in pregnancies complicated by maternal diabetes mellitus. Herz. (2009) 35:19–26. doi: 10.1007/s00059-010-3244-3
69. van der Bom T, Zomer AC, Zwinderman AH, Meijboom FJ, Bouma BJ, Mulder BJ. The changing epidemiology of congenital heart disease. Nat Rev Cardiol. (2011) 8:50–60. doi: 10.1038/nrcardio.2010.166
70. Shoham DA, Vupputuri SX, Kaufman JS, Kshirsagar AV, Roux AVD, Coresh J, et al. Kidney disease and the cumulative burden of life course socioeconomic conditions: the Atherosclerosis Risk in Communities (ARIC) study. Soc Sci Med. (2008) 67(8):1311–20. doi: 10.1016/j.socscimed.2008.06.007
71. Stengel B, Tarver-Carr ME, Powe NR, Eberhardt MS, Brancati FL. Lifestyle factors, obesity and the risk of chronic kidney disease. Epidemiology. (2003) 14:479–87. doi: 10.1097/01.EDE.0000071413.55296.c4
72. Varela MM-S, Nøhr EA, Llopis-González A, Andersen AN, Olsen J. Socio-occupational status and congenital anomalies. Eur J Public Health. (2009) 19:161–7. doi: 10.1093/eurpub/ckp003
73. Angelantonio ED, Chowdhury R, Sarwar N, Aspelund T, Danesh J, Gudnason VG. Chronic kidney disease and risk of major cardiovascular disease and non-vascular mortality: prospective population based cohort study. BMJ. (2010) 341:1–7. doi: 10.1136/bmj.c4986
74. Keller T, Messow C-M, Lubos E, Nicaud V, Wild PS, Rupprecht HJ, et al. Cystatin C and cardiovascular mortality in patients with coronary artery disease and normal or mildly reduced kidney function: results from the AtheroGene study. Eur Heart J. (2009) 30(3):314–20. doi: 10.1093/eurheartj/ehn598
75. Forsberg P-O, Ohlsson H, Sundquist K. Causal nature of neighborhood deprivation on individual risk of coronary heart disease or ischemic stroke: a prospective national Swedish co-relative control study in men and women. Health Place. (2018) 50:1–5. doi: 10.1016/j.healthplace.2017.12.006
76. Wang D, Jackson EA, Karvonen-Gutierrez CA, Elliott MR, Harlow SD, Hood MM, et al. Healthy lifestyle during the midlife is prospectively associated with less subclinical carotid atherosclerosis: the study of women’s health across the nation. J Am Heart Assoc Cardiovasc Cerebrovasc Dis. (2018) 7:1–13. doi: 10.1161/JAHA.118.010405
77. Marco GSD, Reuter S, Kentrup D, Ting L, Ting L, Grabner A, et al. Cardioprotective effect of calcineurin inhibition in an animal model of renal disease. Eur Heart J. (2011) 32(15):1935–45. doi: 10.1093/eurheartj/ehq436
78. Rogacev KS, Seiler S, Zawada AM, Reichart B, Herath E, Roth D, et al. CD14++CD16+ monocytes and cardiovascular outcome in patients with chronic kidney disease. Eur Heart J. (2011) 32(1):84–92. doi: 10.1093/eurheartj/ehq371
79. Chat V, Wu F, Demmer RT, Parvez F, Ahmed A, Eunus M, et al. Association between number of children and carotid intima-media thickness in Bangladesh. PLoS One. (2018) 13:1–11. doi: 10.1371/journal.pone.0208148
80. Dragano N, Verde PE, Moebus S, Stang A, Schmermund A, Roggenbuck U, et al. Subclinical coronary atherosclerosis is more pronounced in men and women with lower socio-economic status: associations in a population-based study coronary atherosclerosis and social status. Eur J Prev Cardiol. (2007) 14:568–74. doi: 10.1097/HJR.0b013e32804955c4
81. Guimaraes JMN, Clarke PJ, Tate DG, Coeli CM, Griep RH, de Jesus Fonseca M, et al. Social mobility and subclinical atherosclerosis in a middle-income country: association of intra- and inter-generational social mobility with carotid intima-media thickness in the Brazilian longitudinal study of adult health (ELSA-Brasil). Soc Sci Med. (2016) 169:9–17. doi: 10.1016/j.socscimed.2016.09.021
82. Lynch JW, Kaplan GA, Salonen RM, Salonen JT. Socioeconomic status and progression of carotid atherosclerosis. prospective evidence from the Kuopio Ischemic Heart Disease Risk Factor Study. Arterioscler Thromb Vasc Biol. (1997) 17(3):513–9. doi: 10.1161/01.ATV.17.3.513
84. Okello E, Kakande B, Sebatta ES, Kayima J, Kuteesa MO, Mutatina B, et al. Socioeconomic and environmental risk factors among rheumatic heart disease patients in Uganda. PLoS One. (2012) 7:1–6. doi: 10.1371/journal.pone.0043917
85. Ahola K, Saarinen AJ, Kuuliala A, Leirisalo-Repo M, Murtomaa HT, Meurman JH. Impact of rheumatic diseases on oral health and quality of life. Oral Dis. (2015) 21(3):342–8. doi: 10.1111/odi.12285
86. Helenius LMJ, Meurman JH, Helenius IJ, Kari K, Hietanen J, Suuronen R, et al. Oral and salivary parameters in patients with rheumatic diseases. Acta Odontol Scand. (2005) 63:284–93. doi: 10.1080/00016350510020043
87. Montastruc F, Montastruc G, Vigreux P, Bruneval P, Guilbeau-Frugier C, Cron C, et al. Valvular heart disease in a patient taking 3,4-methylenedioxymethamphetamine (MDMA, ‘Ecstasy’). Br J Clin Pharmacol. (2012) 74(3):547–8. doi: 10.1111/j.1365-2125.2012.04252.x
88. Manhães ALD, Costa AJL. Access to and utilization of dental services in the State of Rio de Janeiro, Brazil: an exploratory study based on the 1998 National Household Sample Survey. Cad Saude Publica. (2008) 24(1):207–18. doi: 10.1590/S0102-311X2008000100021
89. Manski RJ, Macek MD, Moeller JF. Private dental coverage: who has it and how does it influence dental visits and expenditures? J Am Dent Assoc. (2002) 133(11):1551–9. doi: 10.14219/jada.archive.2002.0087
90. Manski RJ, Moeller JF, Chen H, Clair PAS, Schimmel JA, Pepper JV. Wealth effect and dental care utilization in the united states. J Public Health Dent. (2012) 72(3):179–89. doi: 10.1111/j.1752-7325.2012.00312.x
91. Ghosh A, Simon KI, Sommers BD. The effect of health insurance on prescription drug use among low-income adults:evidence from recent medicaid expansions. J Health Econ. (2019) 63:64–80. doi: 10.1016/j.jhealeco.2018.11.002
92. Spencer S. Lessons from the pure study. Glob Cardiol Sci Pract. (2014) 2014:379–81. doi: 10.5339/gcsp.2014.52
93. Peláez-Ballestas I, Granados Y, Quintana RM, Loyola-Sanchez A, Julián-Santiago F, Rosillo C, et al. Epidemiology and socioeconomic impact of the rheumatic diseases on indigenous people: an invisible syndemic public health problem. Ann Rheum Dis. (2018) 77:1397–404. doi: 10.1136/annrheumdis-2018-213625
94. Grande G, Romppel M. Gender differences in recovery goals in patients after acute myocardial infarction. J Cardiopulm Rehabil Prev. (2011) 31:164–72. doi: 10.1097/HCR.0b013e31820333c8
95. Joynt KE, Mega JL, O’Donoghue ML. Difference or disparity: will big data improve our understanding of sex and cardiovascular disease? Circ Cardiovasc Qual Outcomes. (2015) 8:S52–5. doi: 10.1161/CIRCOUTCOMES.115.001701
96. Leitman M, Dreznik Y, Tyomkin V, Fuchs TT, Krakover R, Vered Z. Vegetation size in patients with infective endocarditis. Eur Heart J Cardiovasc Imaging. (2012) 13(4):330–8. doi: 10.1093/ejechocard/jer253
97. Sambola A, Fernandez-Hidalgo N, Almirante B, Roca I, Gonzalez-Alujas T, Serra B, et al. Sex differences in native-valve infective endocarditis in a single tertiary-care hospital. Am J Cardiol. (2010) 106(1):92–8. doi: 10.1016/j.amjcard.2010.02.019
98. Codreanu I, Pegg TJ, Selvanayagam JB, Robson MD, Rider OJ, Dasanu CA, et al. Normal values of regional and global myocardial wall motion in young and elderly individuals using navigator gated tissue phase mapping. Age. (2013) 36:231–41. doi: 10.1007/s11357-013-9535-x
99. Martínez-García M, Salinas-Ortega M, Estrada-Arriaga I, Hernández-Lemus E, García-Herrera R, Vallejo M. A systematic approach to analyze the social determinants of cardiovascular disease. PLoS One. (2018) 13:e0190960. doi: 10.1371/journal.pone.0190960
100. Aguado A, Moratalla-Navarro F, López-Simarro F, Moreno V. Morbinet: multimorbidity networks in adult general population. analysis of type 2 diabetes mellitus comorbidity. Sci Rep. (2020) 10:2416. doi: 10.1038/s41598-020-59336-1
101. Lee LY, Pandey AK, Maron BA, Loscalzo J. Network medicine in cardiovascular research. Cardiovasc Res. (2021) 117:2186–202. doi: 10.1093/cvr/cvaa321
102. Benincasa G, Marfella R, Della Mura N, Schiano C, Napoli C. Strengths and opportunities of network medicine in cardiovascular diseases. Circ J. (2020) 84:144–52. doi: 10.1253/circj.CJ-19-0879
103. Haug N, Deischinger C, Gyimesi M, Kautzky-Willer A, Thurner S, Klimek P. High-risk multimorbidity patterns on the road to cardiovascular mortality. BMC Med. (2020) 18:1–12. doi: 10.1186/s12916-020-1508-1
104. Amy Y, Quan H, McRae A, Wagner GO, Hill MD, Coutts SB. Moderate sensitivity and high specificity of emergency department administrative data for transient ischemic attacks. BMC Health Serv Res. (2017) 17:666. doi: 10.1186/s12913-017-2612-6
105. Delgado RP, Zavalaga LFL, Morales EAC, Garcia LL. Concordancia entre el diagnóstico médico y la codificación de informática, considerando el cie-10, en la consulta externa de pediatria en el hospital nacional cayetano heredia, lima-perú. Rev Méd Herediana. (2005) 16:239–45.
106. Henderson T, Shepheard J, Sundararajan V. Quality of diagnosis and procedure coding in ICD-10 administrative data. Med Care. (2006) 44:1011–9. doi: 10.1097/01.mlr.0000228018.48783.34
107. Peng M, Lee S, D’Souza AG, Doktorchik CT, Quan H. Development and validation of data quality rules in administrative health data using association rule mining. BMC Med Inform Decis Mak. (2020) 20:1–10. doi: 10.1186/s12911-020-1089-0
Keywords: comorbidities, cardiovascular diseases, human disease network, diseasome, socioeconomic status, health care
Citation: Cruz-Ávila HA, Ramírez-Alatriste F, Martínez-García M and Hernández-Lemus E (2024) Comorbidity patterns in cardiovascular diseases: the role of life-stage and socioeconomic status. Front. Cardiovasc. Med. 11:1215458. doi: 10.3389/fcvm.2024.1215458
Received: 2 May 2023; Accepted: 29 January 2024;
Published: 13 February 2024.
Edited by:
Pietro Amedeo Modesti, University of Florence, ItalyReviewed by:
Shelly R McFarlane, University of the West Indies, JamaicaHani Aiash, Upstate Medical University, United States
© 2024 Cruz-Ávila, Ramírez-Alatriste, Martínez-García and Hernández-Lemus. This is an open-access article distributed under the terms of the Creative Commons Attribution License (CC BY). The use, distribution or reproduction in other forums is permitted, provided the original author(s) and the copyright owner(s) are credited and that the original publication in this journal is cited, in accordance with accepted academic practice. No use, distribution or reproduction is permitted which does not comply with these terms.
*Correspondence: Mireya Martínez-García bWlyZXlhLm1hcnRpbmV6QGNhcmRpb2xvZ2lhLm9yZy5teA== Enrique Hernández-Lemus ZWhlcm5hbmRlekBpbm1lZ2VuLmdvYi5teA==