- 1Department of Diagnostic and Interventional Radiology, Heart Center Leipzig, Leipzig, Germany
- 2Medical Faculty, University of Leipzig, Leipzig, Germany
- 3Department of Cardiology, Heart Center Leipzig, University of Leipzig, Leipzig, Germany
- 4Department of Cardiac Surgery, Heart Center Leipzig, University of Leipzig, Leipzig, Germany
- 5Helios Health Institute, Leipzig, Germany
- 6Siemens Healthcare GmbH, Erlangen, Germany
- 7Institute for Medical Informatics, Statistics and Epidemiology (IMISE), University of Leipzig, Leipzig, Germany
Objective: To compare machine learning (ML)-based CT-derived fractional flow reserve (CT-FFR) in patients before transcatheter aortic valve replacement (TAVR) by observers with differing training and to assess influencing factors.
Background: Coronary computed tomography angiography (cCTA) can effectively exclude CAD, e.g. prior to TAVR, but remains limited by its specificity. CT-FFR may mitigate this limitation also in patients prior to TAVR. While a high reliability of CT-FFR is presumed, little is known about the reproducibility of ML-based CT-FFR.
Methods: Consecutive patients with obstructive CAD on cCTA were evaluated with ML-based CT-FFR by two observers. Categorization into hemodynamically significant CAD was compared against invasive coronary angiography. The influence of image quality and coronary artery calcium score (CAC) was examined.
Results: CT-FFR was successfully performed on 214/272 examinations by both observers. The median difference of CT-FFR between both observers was −0.05(−0.12-0.02) (p < 0.001). Differences showed an inverse correlation to the absolute CT-FFR values. Categorization into CAD was different in 37/214 examinations, resulting in net recategorization of Δ13 (13/214) examinations and a difference in accuracy of Δ6.1%. On patient level, correlation of absolute and categorized values was substantial (0.567 and 0.570, p < 0.001). Categorization into CAD showed no correlation to image quality or CAC (p > 0.13).
Conclusion: Differences between CT-FFR values increased in values below the cut-off, having little clinical impact. Categorization into CAD differed in several patients, but ultimately only had a moderate influence on diagnostic accuracy. This was independent of image quality or CAC.
1. Introduction
Patients evaluated to be treated with transcatheter aortic valve replacement (TAVR) are generally elderly and have a high prevalence of coronary artery disease (CAD) (1–3). CAD is recommended to be excluded and if needed to be treated before the procedure (3–7). Coronary computed tomography angiography (cCTA) is the first line diagnostic tool for the exclusion of CAD in other patient groups (7) and its high negative predictive value (NPV) is known to be preserved also in patient before TAVR (3, 6, 8). Thus, its use is increasingly recognized as part of the standard CT evaluation protocol for TAVR-planning (3–8). However, cCTA remains limited by its low specificity, particularly in this patient group. CT-derived fractional flow reserve (CT-FFR) has been described as a promising tool to mitigate this limitation by non-invasively predicting hemodynamic relevance (9–11) also in patients prior to TAVR (12–16).
Machine learning (ML)-based CT-FFR is a computationally less demanding approach, which makes on-site computation of CT-FFR feasible on standard workstations and is known to correlate well with the more conventional computational fluid dynamics (CFD) approach (17). As opposed to the commercial off-site approaches, where the exact segmentation process is unknown, the user himself performs the segmentation in ML-based CT-FFR. While a high reliability of segmentation is presumed, the significance of the segmentation process and observer experience on the reliability of CT-FFR has not been well examined (18, 19), with no systematic analysis as of now.
In this study, we systematically compared the ML-based CT-FFR measurements carried out by two observers with differing expertise, on segment, vessel and patient level in a large group of patients before TAVR. Furthermore, we analyzed the frequency of conflicting categorizations and the influence of image quality and coronary artery calcium score (CAC).
2. Material and methods
2.1. Study design and patient population
The patient population and study design have previously been reported on (8, 13). In short, consecutive examinations with retrospectively ECG-gated CT for TAVR-planning over a period of 7 months were screened. Only patients having undergone invasive coronary angiography (ICA) within 3 months of CT were considered for the current analysis. Of the 388 patients, 272 had at least one coronary stenosis (≥50%) on cCTA being of interest for CT-FFR evaluation (Figure 1).
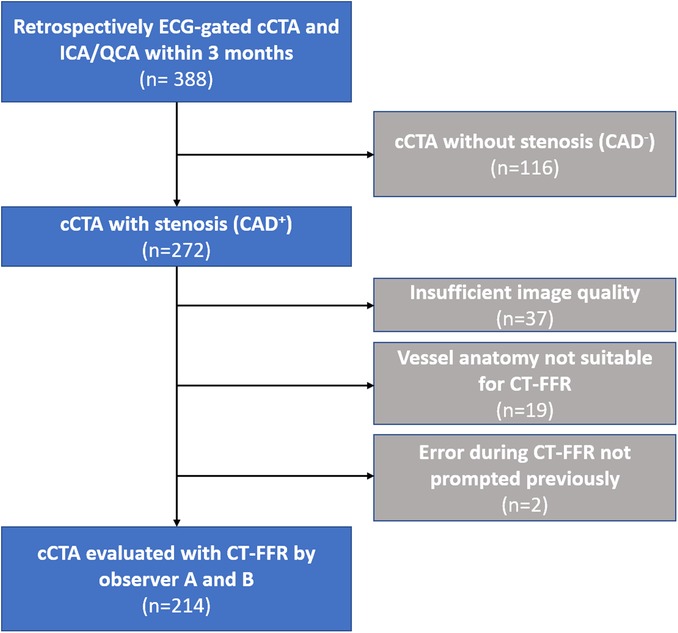
Figure 1. Flowchart of the study population. Flowchart of the study population and reasons for exclusion. CAD, coronary artery disease; cCTA, coronary computed tomography angiography; CT-FFR, CT-derived-fractional-flow-reserve; ECG, electrocardiogram; ICA, invasive coronary angiography; QCA, quantitative coronary angiography.
2.2. CT acquisition
The scan protocol has previously been described in detail (8). Briefly, a retrospectively ECG-gated helical CT of the heart was performed from caudal to cranial, immediately followed by a high-pitch helical CT in the opposite direction for depiction of the aorta and iliofemoral access route using a single bolus of 70 ml iodinated contrast medium. All patients were examined with the same scanner (Somatom Definition Flash; Siemens). No beta blockers or nitrates were given. The ECG-gated scan of the heart was used for computation of the ML-based CT-FFR.
2.3. cCTA and CT-FFR analysis
Coronary arteries were analyzed morphologically by segment according to the 18-segment model (20). When a stenosis of ≥50% diameter was identified on cCTA, CT-FFR values were obtained approximately 2 cm distal to the stenosis (21). The standard of reference was ICA with quantitative coronary analysis (QCA) with the same threshold and ≥70% for a secondary evaluation.
ML-based CT-FFR (cFFR version 3.2.0, Siemens; not commercially available) was performed by observer B on all examinations previously analyzed by observer A (13). The ML-based prototype used for this study has been described in detail before (13, 17, 22). The computationally less demanding process enabled on-site computation on a desktop workstation.
Per-segment interpretations were combined to form per-vessel and per-patient ratings, considering the respective worst segment (highest grade of stenosis; lowest CT-FFR value). CT-FFR values of ≤0.80 were considered as hemodynamically significant CAD (CADf+) (23).
Both observers received the same instructions for segmentation and measurement of CT-FFR. Observer A had received several weeks of training in coronary artery imaging, including formal reading of cCTA and case discussions with correlation to ICA. Observer B only received comprehensive instructions on coronary artery segmentation and handling of the CT-FFR prototype at hand. Both measurements were taken within 18 months. The methods adopted for this study comply with the Guidelines for Reporting Reliability and Agreement Studies (GRRAS) (24).
2.4. Statistical analysis
Continuous variables are presented as median and [interquartile range (IQR)]. Differences between the two observers in CT-FFR values and evaluation times were assessed using the Wilcoxon signed-rank test. Interobserver agreement for CT-FFR values was evaluated using intra-class correlation (ICC) type ICC (1, 3) according to the convention proposed by Shrout and Fleiss (25). For interpretation of ICC coefficients, we followed the guidelines given by Cicchetti, which identify values <0.5 as a poor, 0.5–0.75 as moderate, 0.75–0.9 as a good, and >0.9 as an excellent correlation (26). Interobserver agreement with respect to categorization into hemodynamically significant CAD according to CT-FFR was assessed using Cohen's kappa and interpreted as proposed by Landis and Koch, which classifies correlation as follows: <0.2 slight, 0.2–0.4 fair, 0.4–0.6 moderate, 0.6–0.8 substantial, >0.8 almost perfect (27). Correlation between CT-FFR differences and covariates was calculated using Spearman's rank correlation (quantitative image quality measures and calcium burden) or Kendall's rank correlation (qualitative image quality). Correlation between mismatched coronary artery disease categorization and covariates was determined using the point-biserial correlation (quantitative image quality and CAC) or rank-biserial correlation (qualitative image quality). A p value of <0.05 was considered statistically significant. Statistical analysis was performed using R (version 4.1.2; R Foundation for Statistical Computing, Vienna, Austria).
3. Results
3.1. (Re)evaluation with ML-based CT-FFR
In total, 214 of the 272 examinations with signs of CAD on cCTA were successfully evaluated with ML-based CT-FFR by observer A and B (Figure 1). Two patients could not be reevaluated by observer B, because of an error with the prototype not prompted earlier. Reasons for initial exclusion were insufficient or borderline image quality hindering continuous segmentation of the coronary tree, and anatomical variants outside the model boundaries of the CT-FFR prototype (13). Evaluation time was significantly lower for observer A (observer A: 24 (18–32) min; observer B: 28 (22–35) min; p < 0.001).
Of the included patients 90 (42.1%) were female and the mean body mass index was 29.2 ± 5.5 kg/m2.
3.2. Differences in CT-FFR values
CT-FFR values were significantly different between observer A and B with the largest median differences on patient level (n = 214; −0.05[−0.12–0.02]; p < 0.001). Median differences on vessel level were also significantly different between the observers (left anterior descending artery [LAD] >left circumflex artery [LCX] >right coronary artery [RCA]). The LM had a much lower number of stenoses (n = 13) and thus could not be considered for analyses (Table 1).
Patients recategorized as false negative (FN) from true positive (TP) by either observer showed CT-FFR values closest to the cut-off of ≤0.80 (observer A: n = 17, 0.85 [0.83–0.87]; observer B: n = 28, 0.84 [0.82–0.89]). The distribution of CT-FFR values of both observers is shown in Figure 2. Observer A measured more outliers, particularly in the RCA and LCX. Discrepancies of CT-FFR values between the observers were smaller for high CT-FFR values, and larger for low values (Figure 3).
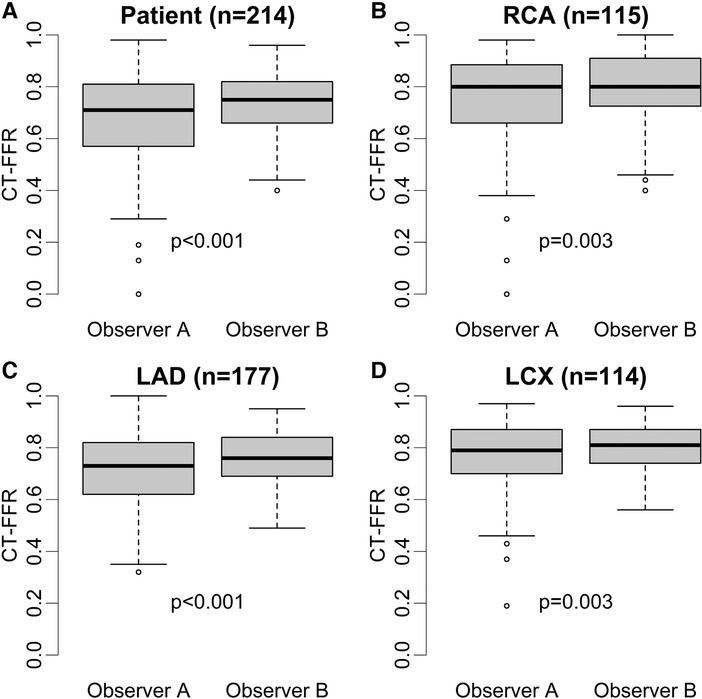
Figure 2. Distribution of absolute CT-FFR values. Box plots of CT-FFR values measured by two observers on patient (A) and vessel level (B–D). Observer B measured higher median CT-FFR values with a smaller interquartile range on patient and vessel level (p ≤ 0.003) (A–D). The difference between both observers were larger on patient level (A) compared to vessel level (patient: 0.05> RCA: 0.05; LAD: 0.04; CX: 0.04) (B–D). Observer A shows more outliers, especially in RCA and LCX (B,C). CT-FFR, CT-derived fractional flow reserve; LAD, left anterior descending artery; LCX, left circumflex artery; RCA, right coronary artery.
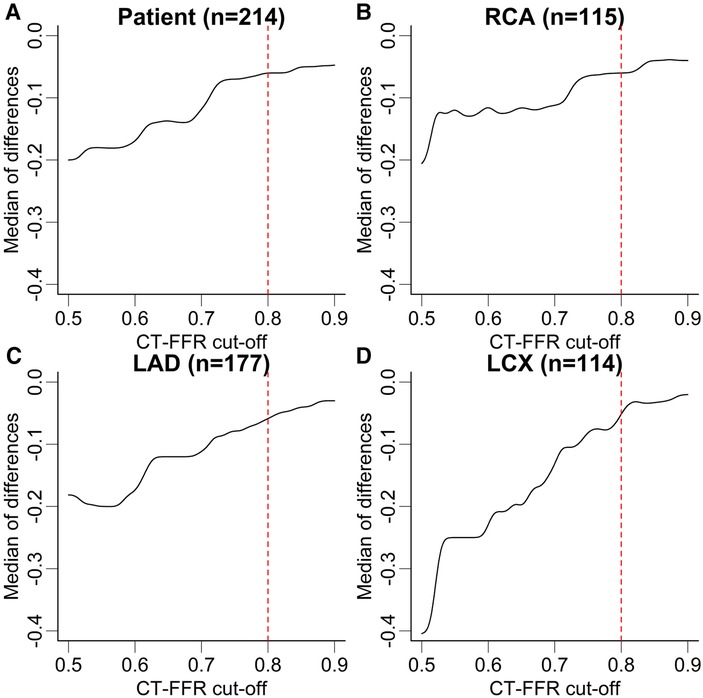
Figure 3. Median difference of CT-FFR values in dependence of absolute values. Median difference of CT-FFR values at different cut-offs between both observers at patient (A) and vessel level (B–D). The median difference of CT-FFR values is higher for low absolute values and lower for high values. Note, there is no discrete cut-off for all levels of observation, but differences are higher below the clinical cut-off CT-FFR ≤0.80. The dashed red lines correspond to the CT-FFR cut-off used to characterize hemodynamically significant CAD. The lines of the graph have been smoothed with a Gaussian filter to help avoid over-interpretation of small steps. CT-FFR, CT-derived fractional flow reserve; LAD, left anterior descending artery; LCX, left circumflex artery; RCA, right coronary artery.
3.3. Interobserver variability
Analysis on patient level showed fair-good agreement between both observers (ICC coefficient: 0.567; p < 0.001). On vessel level, correlation between both observers was fair-good in the RCA and LAD. Agreement of measured values in the LCX was fair (Table 1).
Categorization into hemodynamically significant CAD according to CT-FFR correlated between both observers. On patient level, interobserver agreement was moderate-substantial (Cohen's kappa: 0.570; p < 0.001). Correlation in the RCA was moderate-substantial, in the LAD fair, and in the LCX fair (Table 2).
Observer B recategorized 14/214 patients from negative to positive and 23 patients from positive to negative, with 13 recategorizations being incorrect in regard to the standard of reference, resulting in a difference of diagnostic accuracy of Δ−6.07%. Specificity and negative predictive value (NPV) on patient level were decreased by Δ−1.82% and Δ−12.84%, respectively. On patient and vessel level, more recategorizations occurred from positive to negative than vice versa (Table 3). Gross frequency of different categorizations on vessel level was LCX: 38.6% >LAD: 23.2% >RCA: 20.9%, resulting in a net difference of Δ−3.34% in accuracy. On vessel level, specificity was slightly higher for observer B (Δ+0.73%), while other test metrics were slightly lower (Table 3). There was no discernable trend towards a differing frequency in discrepant categorizations on segment level, e.g., in the distal segments. The gross rate of discrepant categorizations into CADf+ or CADf−on segment level is shown in Appendix 1. On segment level the difference in accuracy was Δ+1.46%.
3.4. Standard of reference and diagnostic performance
Overall, observer B rated fewer stenoses CADf+ on patient level, resulting in a lower specificity, NPV and diagnostic accuracy compared to observer A (Table 3). If the ICA cut-off were changed to ≥70% diameter lumen narrowing, the overall differences between observer A and B became much smaller (specificity: Δ+2.08 vs. Δ−1.82; NPV: Δ−6.56 vs. Δ−12.84; accuracy: Δ−1.40 vs. Δ−6.07) (Table 3).
3.5. Influence of image quality and coronary artery calcifications
Absolute CT-FFR values did not correlate with quantitative image quality (CNR, HU). CT-FFR values correlated weakly with qualitative image quality on patient level and in the RCA (patient: r = −0.116; RCA: r = −0.16; p < 0.03). CT-FFR values correlated weakly with CAC on patient level and in the LAD (patient: r = 0.18; LAD: r = 0.206; p < 0.009).
Categorization into CAD was independent of quantitative or qualitative image quality and of CAC (Table 4).
4. Discussion
Interobserver variability of ML-based CT-FFR has not been studied extensively on a large patient cohort with a high prevalence of CAD. This study on patients before TAVR was carried out by observers with differing levels of experience and rendered somewhat different results. This led to occasional differences in categorization of hemodynamically significant CAD and moderate changes in diagnostic performance. Recategorization of patients was independent of image quality or CAC.
Absolute values of CT-FFR showed significant differences between the observers from 0.03 to 0.04 on vessel and 0.05 on patient level (Table 1). This led to occasional recategorizations into hemodynamically significant CAD when CT-FFR values fell close to the cut-off [grey zone 0.75–0.80 (28)] and likely was the most relevant reason for differences in diagnostic performance between both observers. No observer was clearly superior to the other, with observer A having higher diagnostic accuracy on patient and vessel level and observer B performing slightly better on segment level. The difference in measured values between the observers was lower for high CT-FFR values and much larger for low values. There is no clear cut-off for all levels of observation, but differences are higher below the clinical cut-off CT-FFR ≤0.80 (Figure 3). A possible explanation for this observation could be that segmentation of larger vessel lumina is easier and thus more reproducible; while the opposite is true for small vessels. This is consistent with our observation of the smallest vessel, the LCX, having the weakest interobserver agreement. The higher discrepancy of small values is of little concern, as values far below the common cut-off (0.80 or 0.75) are of little to no significance for clinical decision-making (21, 29). Absolute CT-FFR values measured by the more experienced observer (observer A) had higher variance compared to observer B (Figure 2). A possible reason for this may be a more conservative segmentation of the contrasted lumen, while observer B may have tried to extrapolate the lumen in the presence of blooming artifacts at heavily calcified lesions in a more generic way (30).
Correlation of CT-FFR values in RCA and LAD and on patient level was moderate or borderline-good. Regardless, overall correlation in our patient cohort was lower than that reported in other patient groups. Ko et al. reported a median difference of 0.03 on patient level vs. 0.05 in this study. Studies with more experienced observers reported good-to-excellent interobserver agreement (18, 19, 31). Observers with less training showed higher discrepancies and moderate agreement (32, 33). The studies consisted of much younger patient groups (60.0 ± 8.5 years; 64.6 ± 8.9 years; 61.8 ± 10.2 years; 62.7 ± 8.9 years vs. 78.9 ± 9.7 years) with fewer stenoses per patient [0.53; 1.47; 0.33; 1.22 (stenosed vessels) vs. 1.6 stenoses per patient] (18, 19, 32, 34). Median evaluation time was vastly different (Ko et al.: 27 min; Donnelly et al.: 9 min; Yang et al.: 50 min; Ihdayhid et al.: 24–38 min; current study: 24 and 28 min). In addition to the lower experience of the observers, the most likely cause for the weaker interobserver agreement in our study lies in the much different and more challenging patient group of patients prior TAVR with more frequent and perhaps higher grade and frequently calcified stenoses. Overall, more experienced observers had better agreement, while less experience only had moderate agreement between the observers (18, 32, 33). This is a direct result of differences in lumen segmentation by the user himself in ML-based CT-FFR. Resulting differences are thus no different from the reproducibility of other techniques e.g., of cCTA with good interobserver and intraobserver agreement between trained observers, and moderate agreement between untrained observers (35, 36), or even ICA interpretation (37). Other CT-FFR solutions, namely the only commercially available and FDA approved CFD-based technique has not publish data concerning observer experience and reliability. Overall, observer experience seems to have a large influence on reliable CAD diagnosis. Standardized training and certification may likely improve reliability of ML-based CT-FFR further.
Interobserver agreement of categorization of patients into hemodynamically significant CAD was similar to the correlation of absolute values with moderate and sometimes moderate-to-good correlation for the RCA, LAD and patient level. This may be reassuring as in clinical decision making most commonly a discreet cut-off is used. Despite the agreement between the observers not being optimal, it can be considered fair, taking into account the observer's differing experience (Table 2). Notably, the LCX had the lowest correlation of absolute values and lowest agreement between categorized values. Although there is no definitive answer for this observation, the LCX is generally the smallest vessel with relatively few and short segments and the second highest rate of motion during the cardiac cycle (38). This may contribute to motion- and step-artefacts consequently decreasing diagnostic performance (39) and ultimately making segmentation the most challenging in this vessel.
Observer B showed lower diagnostic performance on patient level, with especially lower sensitivity (Δ−10.58%). However, on vessel level, specificity of observer B was higher (Δ+0.73%) (Table 3). A possible explanation may be a different, more conservative segmentation approach for the less experienced observer B, e.g., when encountering artifacts. The much lower NPV on all levels of observation might be caused by observer B's lack of clinical experience, possibly leading to a generic extrapolation of the lumen in calcified lesions and failure to differentiate plaque from artifact and vice versa. Thus, more hemodynamically relevant stenoses were missed (higher false-negative count). However, it must be kept in mind that the standard of reference in this study was anatomical (ICA with QCA). The hemodynamic significance of stenosis in ICA, especially in the context of aortic valve stenosis (AS) and subsequent left ventricular (LV) hypertrophy, is unclear. Because CT-FFR is derived from the vessel cross-section and dependent on specific vessel anatomy and LV mass, AS may influence this technique and generate different values than in patients without AS and none of the resulting adaptations.
The change in diagnostic performance and specificity (Δ−1.82%) on patient level is dependent on the standard of reference. The very conservative cut-off of ≥50% for QCA might not be optimal for clinical decision-making, as it likely includes many stenoses without hemodynamic relevance. Meanwhile, CT-FFR may have classified these as not hemodynamically relevant causing false-negative categorizations. A higher ICA cut-off (e.g., QCA ≥70%) would lead to fewer false-negative categorizations by CT-FFR. Changing the standard of reference to this more stringent cut-off would decrease sensitivity, potentially increase specificity and may decrease the differences in diagnostic performance between both observers (accuracy: Δ−6.07–Δ−1.40; Table 3).
Overall, observer A likely evaluated stenoses more strictly, which explains the higher sensitivity. More clinical experience is the most probable reason for the better performance on the clinically relevant levels of observation, namely patient and vessel level. Minute differences in segmentation of the lumen may lead to different categorization into CAD whenever values fall close to the cut-off. Notably, specificity remains very similar between the observers. This can be explained by many true-negative categorizations of values relatively clearly above the cut-off. The patients that are categorized as false-negative presented CT-FFR values closer to the grey zone (0.75–0.80) than other patients (Observer A: 0.85; Observer B: 0.84). These borderline cases are prone to recategorization between both observers. As many recategorizations are correct in regard to ICA and cancel each other out, their influence on diagnostic performance is much smaller than their number leads to believe. This is supported by the number of differing CAD categorizations being larger than the actual change in diagnostic performance (recategorizations: 37/214; accuracy: Δ−6.07).
Image quality and calcium burden may interfere with the correct assessment of coronary arteries. However, the categorization into hemodynamically relevant CAD with CT-FFR was independent of CAC and image quality, which is encouraging for the use of CT-FFR in the group of patients prior to TAVR. Small, likely not clinically relevant correlations of absolute CT-FFR values and image quality and CAC were noted. Considering the number of tests performed, these findings should not be overestimated. Our findings with little to no influence of CAC on CT-FFR are consistent with the literature, with only Tesche et al. finding a degrading effect CAC on CT-FFR with very high scores (18, 19, 30, 39, 40), even though also patients with much higher CAC were included in our study. The virtual lack of correlation of CT-FFR values to image quality suggests that once a certain threshold of image quality is reached, CT-FFR may be expected to be performed reliably. Even a new deep learning algorithm for the improvement of image quality was not able to increase diagnostic performance of CT-FFR further (30).
Patients prior to TAVR assessed with ML-based CT-FFR by two observers with differing experience were sometimes categorized differently into having hemodynamically relevant CAD or not. This was independent of image quality or CAC. This can easily be understood if values fall close to the cut-off and CT-FFR is only measured at a single point in a fixed distance distal to the stenoses (Figure 4). However, hemodynamical implications of luminal narrowing can manifest distal to that point of measurement (21). On the other hand, diffuse arteriosclerosis without a distinct stenosis may have a cumulative effect (41) additive to or independent of the stenosis measured. Instead of a single measurement with a fixed cut-off, a relative decrease of CT-FFR values along the coronary tree could perhaps prove more representative for the global hemodynamic situation (21, 41–44) of the coronary arterial vasculature.
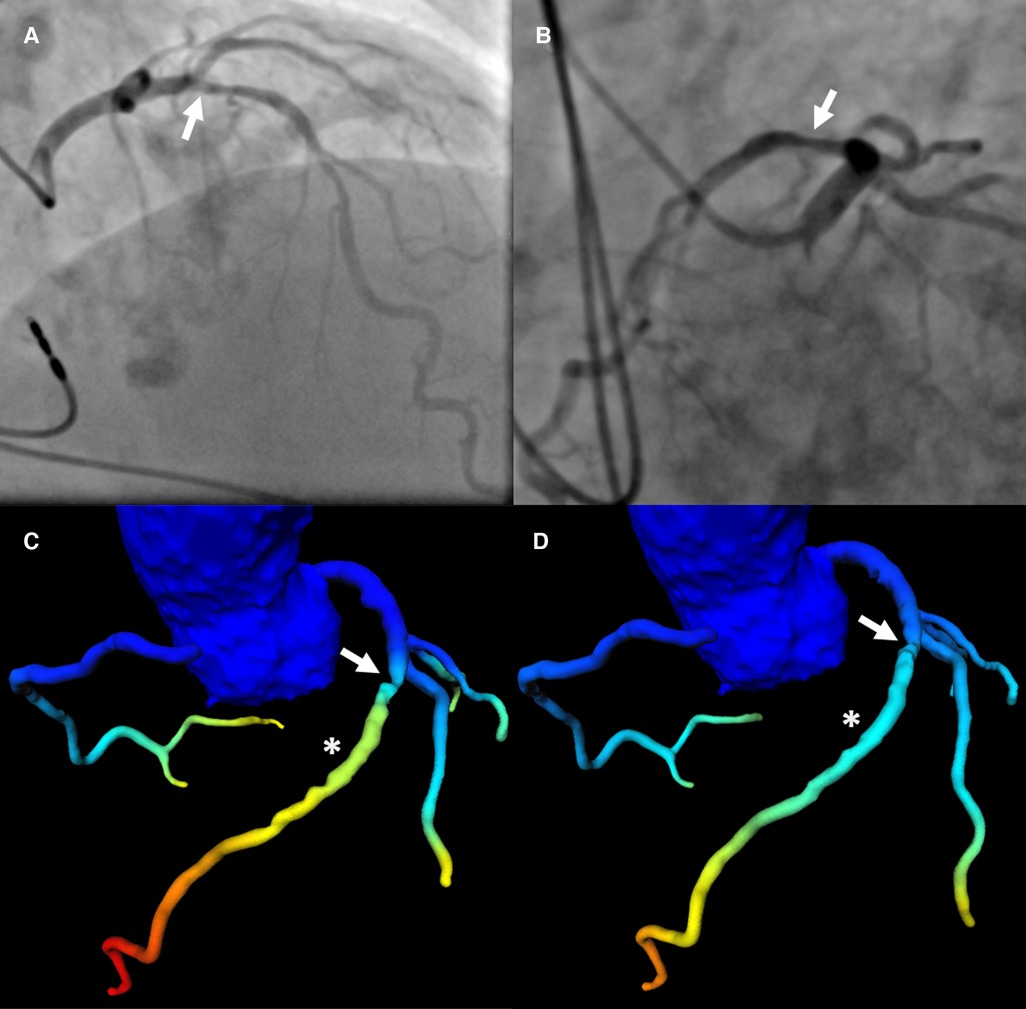
Figure 4. Patient with severe LAD stenosis and discrepant categorization according to CT-FFR values. Patient with severe stenosis (arrow in a-d) in the middle LAD (S7) on ICA (QCA: 78%) (A,B) and results of CT-FFR of observer A (C) and observer B (D) CT-FFR values were taken approximately 2 cm distal to the stenosis (asterisk in C,D). The CT-FFR value measured by observer A was 0.79, indicating hemodynamically significant CAD (C), the value measured by observer B was 0.86, indicating non-significant CAD (D) The threshold for hemodynamically significant CAD was ≤0.80. CAD, coronary artery disease; CT-FFR, CT-derived fractional flow reserve; ICA, invasive coronary angiography; LAD, left anterior descending artery; QCA, quantitative coronary analysis.
4.1. Limitations
Several important limitations to our study must be noted. First, the standard of reference in this study was morphological, not functional with the conservative cut-off of ≥50% diameter on QCA. We explored how discrepancies with a more stringent cut-off would change. But ultimately, a functional standard of reference like invasive FFR would be desirable, particularly in the patient group before TAVR with hemodynamical changes, likely also in coronary artery physiology. Independently of the applied standard, the observed differences in CT-FFR values between the observers are real and likely to be similar in practical application and should be considered whenever performing CT-FFR for clinical decision making. Furthermore, patients before TAVR generally have severe AS with subsequent LV-hypertrophy. As ML-based CT-FFR also considers LV-mass in addition to vessel cross section and specific vessel anatomy for its computation, AS and underling secondary changes may influence computed CT-FFR values. Though different, clinical experience of both observer A and B was limited and may not reflect clinical practice at academic centers with dedicated experts performing such analysis, it can be assumed that expert observers are more consistent (18, 19). However, so far no data is available about the segmentation process of the commercially available off-site solution. Furthermore, the limited experience of the observers likely amplified the differences in read values, perhaps even allowing for a better evaluation of the potential disturbing factors of image quality and CAC.
5. Conclusion
Measurement of ML-based CT-FFR in patients prior to TAVR by observers with different clinical experience lead to discrepancies in CT-FFR values and CAD categorization, with larger discrepancies in low values and smaller discrepancies in high values. This caused a moderate difference in diagnostic accuracy. Image quality and CAC appear not to influence categorization according to CT-FFR. It seems advisable for segmentation to be performed by expert observers, particularly when values around the “grey zone” are to be expected.
Data availability statement
The raw data supporting the conclusions of this article will be made available by the authors, without undue reservation.
Ethics statement
The studies involving humans were approved by the local ethics committee of the University of Leipzig (reference number: 435/18-ek). The study was conducted in accordance with the local legislation and institutional requirements. The ethics committee/institutional review board waived the requirement of written informed consent for participation from the participants or the participants’ legal guardians/next of kin because of the retrospective design and no identifiable data was being used.
Author contributions
RG: Conceptualization, Data curation, Formal analysis, Investigation, Methodology, Supervision, Writing – original draft, Writing – review & editing. AS: Conceptualization, Data curation, Formal analysis, Investigation, Writing – original draft, Writing – review & editing. KP: Data curation, Investigation, Writing – review & editing. PS: Conceptualization, Data curation, Investigation, Methodology, Writing – review & editing. NM: Investigation, Writing – review & editing. HH: Investigation, Validation, Writing – review & editing. LH: Investigation, Writing – review & editing. KR: Investigation, Writing – review & editing. SD: Investigation, Writing – review & editing. SL: Investigation, Writing – review & editing. TN: Investigation, Writing – review & editing. PK: Investigation, Writing – review & editing. CK: Investigation, Writing – review & editing. CL: Investigation, Writing – review & editing. SE: Investigation, Writing – review & editing. MB: Writing – review & editing. HT: Investigation, Writing – review & editing. CP: Methodology, Software, Writing – review & editing. MA-W: Data curation, Resources, Validation, Writing – review & editing. MH: Formal analysis, Writing – original draft, Writing – review & editing. MG: Conceptualization, Funding acquisition, Methodology, Project administration, Resources, Software, Supervision, Visualization, Writing – review & editing.
Funding
The author(s) declare financial support was received for the research, authorship, and/or publication of this article.
We acknowledge support from Leipzig University for Open Access Publishing.
Conflict of interest
CP was employed by Siemens Healthcare GmbH.
The remaining authors declare that the research was conducted in the absence of any commercial or financial relationships that could be construed as a potential conflict of interest.
The reviewer JU declared a past co-authorship with the author RFG to the handling editor.
Publisher's note
All claims expressed in this article are solely those of the authors and do not necessarily represent those of their affiliated organizations, or those of the publisher, the editors and the reviewers. Any product that may be evaluated in this article, or claim that may be made by its manufacturer, is not guaranteed or endorsed by the publisher.
References
1. Mack MJ, Leon MB, Thourani VH, Makkar R, Kodali SK, Russo M, et al. Transcatheter aortic-valve replacement with a balloon-expandable valve in low-risk patients. N Engl J Med. (2019) 380:1695–705. doi: 10.1056/NEJMOA1814052
2. Popma JJ, Deeb GM, Yakubov SJ, Mumtaz M, Gada H, O’Hair D, et al. Transcatheter aortic-valve replacement with a self-expanding valve in low-risk patients. N Engl J Med. (2019) 380:1706–15. doi: 10.1056/NEJMOA1816885
3. Francone M, Budde RPJ, Bremerich J, Dacher JN, Loewe C, Wolf F, et al. CT and MR imaging prior to transcatheter aortic valve implantation: standardisation of scanning protocols, measurements and reporting—a consensus document by the European society of cardiovascular radiology (ESCR). Eur Radiol. (2020) 30:2627–50. doi: 10.1007/S00330-019-06357-8
4. Otto CM, Nishimura RA, Bonow RO, Carabello BA, Erwin JP, Gentile F, et al. 2020 ACC/AHA guideline for the management of patients with valvular heart disease: a report of the American college of cardiology/American heart association joint committee on clinical practice guidelines. J Am Coll Cardiol. (2021) 77:e25–e197. doi: 10.1016/J.JACC.2020.11.018
5. Grover FL, Vemulapalli S, Carroll JD, Edwards FH, Mack MJ, Thourani VH, et al. 2016 annual report of the society of thoracic surgeons/American college of cardiology transcatheter valve therapy registry. J Am Coll Cardiol. (2017) 69:1215–30. doi: 10.1016/J.JACC.2016.11.033
6. Blanke P, Weir-McCall JR, Achenbach S, Delgado V, Hausleiter J, Jilaihawi H, et al. Computed tomography imaging in the context of transcatheter aortic valve implantation (TAVI)/transcatheter aortic valve replacement (TAVR): an expert consensus document of the society of cardiovascular computed tomography. JACC Cardiovasc Imaging. (2019) 12:1–24. doi: 10.1016/J.JCMG.2018.12.003
7. Vahanian A, Beyersdorf F, Praz F, Milojevic M, Baldus S, Bauersachs J, et al. 2021 ESC/EACTS guidelines for the management of valvular heart disease. Eur Heart J. (2022) 43:561–632. doi: 10.1093/EURHEARTJ/EHAB395
8. Gohmann RF, Lauten P, Seitz P, Krieghoff C, Lücke C, Gottschling S, et al. Combined coronary CT-angiography and TAVI-planning: a contrast-neutral routine approach for ruling-out significant coronary artery disease. J Clin Med. (2020) 9:1623. doi: 10.3390/jcm9061623
9. Patel MR, Nørgaard BL, Fairbairn TA, Nieman K, Akasaka T, Berman DS, et al. 1-year impact on medical practice and clinical outcomes of FFRCT: the ADVANCE registry. JACC Cardiovasc Imaging. (2020) 13:97–105. doi: 10.1016/J.JCMG.2019.03.003
10. Fairbairn TA, Nieman K, Akasaka T, Nørgaard BL, Berman DS, Raff G, et al. Real-world clinical utility and impact on clinical decision-making of coronary computed tomography angiography-derived fractional flow reserve: lessons from the ADVANCE registry. Eur Heart J. (2018) 39:3701–11. doi: 10.1093/EURHEARTJ/EHY530
11. Nørgaard BL, Leipsic J, Gaur S, Seneviratne S, Ko BS, Ito H, et al. Diagnostic performance of noninvasive fractional flow reserve derived from coronary computed tomography angiography in suspected coronary artery disease the NXT trial (analysis of coronary blood flow using CT angiography: next steps). JACC. (2014) 63:1145–55. doi: 10.1016/j.jacc.2013.11.043
12. Gutberlet M, Krieghoff C, Gohmann R. Werden die karten der CT-koronarangiographie mit der FFR CT neu gemischt? Herz. (2020) 45:431–40. doi: 10.1007/s00059-020-04944-w
13. Gohmann RF, Pawelka K, Seitz P, Majunke N, Heiser L, Renatus K, et al. Combined cCTA and TAVR planning for ruling out significant CAD: added value of ML-based CT-FFR. JACC Cardiovasc Imaging. (2022) 15:476–86. doi: 10.1016/J.JCMG.2021.09.013
14. Brown AJ, Michail M, Ihdayhid A-R, Comella A, Thakur U, Cameron JD, et al. Circulation: cardiovascular interventions feasibility and validity of computed tomography-derived fractional flow reserve in patients with severe aortic stenosis the CAST-FFR study. Circ Cardiovasc Interv. (2021) 14:9586. doi: 10.1161/CIRCINTERVENTIONS.120.009586
15. Hamdan A, Wellnhofer E, Konen E, Kelle S, Goitein O, Andrada B, et al. Coronary CT angiography for the detection of coronary artery stenosis in patients referred for transcatheter aortic valve replacement. J Cardiovasc Comput Tomogr. (2015) 9:31–41. doi: 10.1016/j.jcct.2014.11.008
16. Peper J, Becker LM, van den Berg H, Bor WL, Brouwer J, Nijenhuis VJ, et al. Diagnostic performance of CCTA and CT-FFR for the detection of CAD in TAVR work-up. JACC Cardiovasc Interv. (2022) 15:1140–9. doi: 10.1016/J.JCIN.2022.03.025
17. Coenen A, Kim Y-H, Kruk M, Tesche C, de Geer J, Kurata A, et al. Diagnostic accuracy of a machine-learning approach to coronary computed tomographic angiography–based fractional flow reserve. Circ Cardiovasc Imaging. (2018) 11:1–11. doi: 10.1161/CIRCIMAGING.117.007217
18. Donnelly PM, Kolossváry M, Karády J, Ball PA, Kelly S, Fitzsimons D, et al. Experience with an on-site coronary computed tomography-derived fractional flow reserve algorithm for the assessment of intermediate coronary stenoses. Am J Cardiol. (2018) 121:9–13. doi: 10.1016/J.AMJCARD.2017.09.018
19. Yang DH, Kim YH, Roh JH, Kang JW, Ahn JM, Kweon J, et al. Diagnostic performance of on-site CT-derived fractional flow reserve versus CT perfusion. Eur Heart J Cardiovasc Imaging. (2017) 18:432–40. doi: 10.1093/ehjci/jew094
20. Leipsic J, Abbara S, Achenbach S, Cury R, Earls JP, Mancini GJ, et al. SCCT guidelines for the interpretation and reporting of coronary CT angiography: a report of the society of cardiovascular computed tomography guidelines committee. J Cardiovasc Comput Tomogr. (2014) 8:342–58. doi: 10.1016/j.jcct.2014.07.003
21. Nørgaard BL, Fairbairn TA, Safian RD, Rabbat MG, Ko B, Jensen JM, et al. Coronary CT angiography-derived fractional flow reserve testing in patients with stable coronary artery disease: recommendations on interpretation and reporting. Radiol Cardiothorac Imaging. (2019) 1:e190050. doi: 10.1148/ryct.2019190050
22. Itu L, Rapaka S, Passerini T, Georgescu B, Schwemmer C, Schoebinger M, et al. A machine-learning approach for computation of fractional flow reserve from coronary computed tomography. J Appl Physiol. (2016) 121:42–52. doi: 10.1152/japplphysiol.00752.2015.-Frac
23. Chinnaiyan KM, Akasaka T, Amano T, Bax JJ, Blanke P, De Bruyne B, et al. Rationale, design and goals of the HeartFlow assessing diagnostic value of non-invasive FFRCT in coronary care (ADVANCE) registry. J Cardiovasc Comput Tomogr. (2017) 11:62–7. doi: 10.1016/J.JCCT.2016.12.002
24. Kottner J, Audigé L, Brorson S, Donner A, Gajewski BJ, Hróbjartsson A, et al. Guidelines for reporting reliability and agreement studies (GRRAS) were proposed. J Clin Epidemiol. (2011) 64:96–106. doi: 10.1016/J.JCLINEPI.2010.03.002
25. Shrout PE, Fleiss JL. Intraclass correlations: uses in assessing rater reliability. Psychol Bull. (1979) 86:420–8. doi: 10.1037//0033-2909.86.2.420
26. Cicchetti DV. Guidelines, criteria, and rules of thumb for evaluating normed and standardized assessment instruments in psychology. Psychol Assess. (1994) 6:284–90. doi: 10.1037/1040-3590.6.4.284
27. Landis JR, Koch GG. The measurement of observer agreement for categorical data. Biometrics. (1977) 33:159. doi: 10.2307/2529310
28. Tang CX, Liu CY, Lu MJ, Schoepf UJ, Tesche C, Bayer RR, et al. CT FFR for ischemia-specific CAD with a new computational fluid dynamics algorithm: a Chinese multicenter study. JACC Cardiovasc Imaging. (2020) 13:980–90. doi: 10.1016/j.jcmg.2019.06.018
29. Brandt V, Schoepf UJ, Aquino GJ, Bekeredjian R, Varga-Szemes A, Emrich T, et al. Impact of machine-learning-based coronary computed tomography angiography-derived fractional flow reserve on decision-making in patients with severe aortic stenosis undergoing transcatheter aortic valve replacement. Eur Radiol. (2022) 32:6008–16. doi: 10.1007/S00330-022-08758-8
30. van Hamersvelt RW, Voskuil M, de Jong PA, Willemink MJ, Išgum I, Leiner T. Diagnostic performance of on-site coronary CT angiography–derived fractional flow reserve based on patient-specific lumped parameter models. Radiol Cardiothorac Imaging. (2019) 1:1–9. doi: 10.1148/ryct.2019190036
31. Fujii Y, Kitagawa T, Ikenaga H, Tatsugami F, Awai K, Nakano Y. The reliability and utility of on-site CT-derived fractional flow reserve (FFR) based on fluid structure interactions: comparison with FFRCT based on computational fluid dynamics, invasive FFR, and resting full-cycle ratio. Heart Vessels. (2023) 38(9):1095–107. doi: 10.1007/S00380-023-02265-6
32. Ihdayhid AR, Sakaguchi T, Kerrisk B, Hislop-Jambrich J, Fujisawa Y, Nerlekar N, et al. Influence of operator expertise and coronary luminal segmentation technique on diagnostic performance, precision and reproducibility of reduced-order CT-derived fractional flow reserve technique. J Cardiovasc Comput Tomogr. (2020) 14:356–62. doi: 10.1016/J.JCCT.2019.11.014
33. Kumamaru KK, Angel E, Sommer KN, Iyer V, Wilson MF, Agrawal N, et al. Inter- and intraoperator variability in measurement of on-site CT-derived fractional flow reserve based on structural and fluid analysis: a comprehensive analysis. Radiol Cardiothorac Imaging. (2019) 1:1–7. doi: 10.1148/RYCT.2019180012
34. Ko BS, Cameron JD, Munnur RK, Wong DTL, Fujisawa Y, Sakaguchi T, et al. Noninvasive CT-derived FFR based on structural and fluid analysis: a comparison with invasive FFR for detection of functionally significant stenosis. JACC Cardiovasc Imaging. (2017) 10:663–73. doi: 10.1016/J.JCMG.2016.07.005
35. Nicol ED, Stirrup J, Roughton M, Padley SPG, Rubens MB. 64-channel cardiac computed tomography: intraobserver and interobserver variability (part 1): coronary angiography. J Comput Assist Tomogr. (2009) 33:161–8. doi: 10.1097/RCT.0B013E31817C423E
36. Kerl JM, Schoepf UJ, Bauer RW, Tekin T, Costello P, Vogl TJ, et al. 64-slice multidetector-row computed tomography in the diagnosis of coronary artery disease: interobserver agreement among radiologists with varied levels of experience on a per-patient and per-segment basis. J Thorac Imaging. (2012) 27:29–35. doi: 10.1097/RTI.0B013E3181F82805
37. Murphy ML, Galbraith JE, de Soyza N. The reliability of coronary angiogram interpretation: an angiographic-pathologic correlation with a comparison of radiographic views. Am Heart J. (1979) 97:578–84. doi: 10.1016/0002-8703(79)90184-4
38. Achenbach S, Ropers D, Holle J, Muschiol G, Daniel WC, Moshage W. In-plane coronary arterial motion velocity: measurement with electron-beam CT. Radiology. (2000) 216:457–63. doi: 10.1148/RADIOLOGY.216.2.R00AU19457
39. Leipsic J, Yang TH, Thompson A, Koo BK, John Mancini GB, Taylor C, et al. CT angiography (CTA) and diagnostic performance of noninvasive fractional flow reserve: results from the determination of fractional flow reserve by anatomic CTA (DeFACTO) study. Am J Roentgenol. (2014) 202:989–94. doi: 10.2214/AJR.13.11441
40. Tesche C, Otani K, De Cecco CN, Coenen A, De Geer J, Kruk M, et al. Influence of coronary calcium on diagnostic performance of machine learning CT-FFR: results from MACHINE registry. JACC Cardiovasc Imaging. (2020) 13:760–70. doi: 10.1016/J.JCMG.2019.06.027
41. Takagi H, Ishikawa Y, Orii M, Ota H, Niiyama M, Tanaka R, et al. Optimized interpretation of fractional flow reserve derived from computed tomography: comparison of three interpretation methods. J Cardiovasc Comput Tomogr. (2019) 13:134–41. doi: 10.1016/J.JCCT.2018.10.027
42. Cami E, Tagami T, Raff G, Fonte TA, Renard B, Gallagher MJ, et al. Assessment of lesion-specific ischemia using fractional flow reserve (FFR) profiles derived from coronary computed tomography angiography (FFRCT) and invasive pressure measurements (FFRINV): importance of the site of measurement and implications for patient referral for invasive coronary angiography and percutaneous coronary intervention. J Cardiovasc Comput Tomogr. (2018) 12:480–92. doi: 10.1016/J.JCCT.2018.09.003
43. Gohmann RF, Seitz P, Pawelka K, Majunke N, Schug A, Heiser L, et al. Clinical medicine combined coronary CT-angiography and TAVI planning: utility of CT-FFR in patients with morphologically ruled-out obstructive coronary artery disease. J Clin Med. (2022) 11:1331–44. doi: 10.3390/jcm11051331
44. Iwasaki K, Kusachi S. Coronary pressure measurement based decision making for percutaneous coronary intervention. Curr Cardiol Rev. (2009) 5:323. doi: 10.2174/157340309789317832
Appendix
Keywords: aortic stenosis, computed tomography coronary angiography, coronary angiography, coronary artery disease, transcatheter aortic valve implantation, diagnostic accuracy, machine learning, computed tomography fractional flow reserve
Citation: Gohmann RF, Schug A, Pawelka K, Seitz P, Majunke N, El Hadi H, Heiser L, Renatus K, Desch S, Leontyev S, Noack T, Kiefer P, Krieghoff C, Lücke C, Ebel S, Borger MA, Thiele H, Panknin C, Abdel-Wahab M, Horn M and Gutberlet M (2023) Interrater variability of ML-based CT-FFR during TAVR-planning: influence of image quality and coronary artery calcifications. Front. Cardiovasc. Med. 10:1301619. doi: 10.3389/fcvm.2023.1301619
Received: 25 September 2023; Accepted: 13 November 2023;
Published: 21 December 2023.
Edited by:
Gaoyang Li, Tohoku University, JapanReviewed by:
Boyan Mao, Beijing University of Chinese Medicine, ChinaJohannes Uhlig, University Medical Center Goettingen, Germany
© 2023 Gohmann, Schug, Pawelka, Seitz, Majunke, El Hadi, Heiser, Renatus, Desch, Leontyev, Noack, Kiefer, Krieghoff, Lücke, Ebel, Borger, Thiele, Panknin, Abdel-Wahab, Horn and Gutberlet. This is an open-access article distributed under the terms of the Creative Commons Attribution License (CC BY). The use, distribution or reproduction in other forums is permitted, provided the original author(s) and the copyright owner(s) are credited and that the original publication in this journal is cited, in accordance with accepted academic practice. No use, distribution or reproduction is permitted which does not comply with these terms.
*Correspondence: Robin F. Gohmann cm9iaW4uZ29obWFubkBnbXguZGU=
†These authors have contributed equally to this work and share first authorship
‡These authors have contributed equally to this work and share senior authorship
Abbreviations: AS, aortic valve stenosis; CAC, coronary artery calcium score; CAD, coronary artery disease; CAD−, negative for coronary artery disease; CADf−, negative for hemodynamically significant coronary artery disease; CADf+, negative for hemodynamically significant coronary artery disease; cCTA, coronary CT-angiography; CFD, computational fluid dynamics; CI, confidence interval; CNR, contrast to noise ratio; CT-FFR, CT-derived fractional flow reserve; FN, false negative; FP, false positive; HU, Hounsfield unit; ICA, invasive coronary angiography; ICC, intra-class correlation coefficient; LAD, left anterior descending artery; LCX, circumflex artery; LM, left main coronary artery; LV, left ventricular; ML, machine learning; MM, mismatched coronary artery disease categorizations; NN, remained negative; NP, negative to positive; NPV, negative predictive value; PN, positive to negative; PP, remained positive; PPV, positive predictive value; QCA, quantitative coronary angiography; RCA, right coronary artery; SCCT, Society of Cardiovascular Computed Tomography#; Sen, sensitivity; Spe, specificity; TAVR, transcatheter aortic valve replacement; TP, true positive.