- Department of Clinical Epidemiology and Evidence-Based Medicine, The First Hospital of China Medical University, Shenyang, China
Purpose: We aimed to establish and authenticate a clinical prognostic nomogram for predicting long-term Major Adverse Cardiovascular Events (MACEs) among high-risk patients who have undergone Percutaneous Coronary Intervention (PCI) in county-level health service.
Patients and methods: This prospective study included Acute Coronary Syndrome (ACS) patients treated with PCI at six county-level hospitals between September 2018 and August 2019, selected from both the original training set and external validation set. Least Absolute Shrinkage and Selection Operator (LASSO) regression techniques and logistic regression were used to assess potential risk factors and construct a risk predictive nomogram. Additionally, the potential non-linear relationships between continuous variables were tested using Restricted Cubic Splines (RCS). The performance of the nomogram was evaluated based on the Receiver Operating Characteristic (ROC) curve analysis, Calibration Curve, Decision Curve Analysis (DCA), and Clinical Impact Curve (CIC).
Results: The original training set and external validation set comprised 520 and 1,061 patients, respectively. The final nomogram was developed using nine clinical variables: Age, Killip functional classification III-IV, Hypertension, Hyperhomocysteinemia, Heart failure, Number of stents, Multivessel disease, Low-density Lipoprotein Cholesterol, and Left Ventricular Ejection Fraction. The AUC of the nomogram was 0.79 and 0.75 in the training set and external validation set, respectively. The DCA and CIC validated the clinical value of the constructed prognostic nomogram.
Conclusion: We developed and validated a prognostic nomogram for predicting the probability of 3-year MACEs in ACS patients who underwent PCI at county-level hospitals. The nomogram could provide a precise risk assessment for secondary prevention in ACS patients receiving PCI.
1. Introduction
Coronary Heart Disease (CHD) is a major contributor to global health and socioeconomic burdens, especially with the rising age of the world population. As an alarming emergence, CHD is a global public health issue posing a severe threat to human health (1). Despite an increase in the overall CHD burden, age-adjusted CHD mortality is decreasing in developed countries (2, 3). Low- and Middle-Income Countries (LMIC) face a substantial cardiovascular disease burden as urbanization and societal transformation escalate. According to the Report on Cardiovascular Health and Diseases in China data from 2019, rural and urban areas experienced a significant burden of mortality due to cardiovascular disease (CVD), with CVD accounting for 46.74% and 44.26% of all deaths in these respective regions (4). Furthermore, CHD severity appears to follow a reverse socioeconomic gradient in developing nations (5). A prospective analytical study conducted in India discovered notable socioeconomic disparities in access to primary and secondary prevention for Acute Coronary Syndrome (ACS) management (6).
As a life-threatening symptom of coronary heart disease, ACS includes ST-segment Elevation Myocardial Infarction (STEMI), Non-ST-segment Elevation Myocardial Infarction (NSTEMI), and Unstable Angina (UA) (7, 8). A combination of oral medications and interventional procedures are commonly used to manage patients with ACS (9, 10). Percutaneous Coronary Intervention (PCI), which involves balloon dilatation and stenting, is the preferred method of reperfusion therapy for ACS patients (10–12). Despite undergoing PCI, a subset of ACS patients continue to experience Major Adverse Cardiovascular Events (MACEs), including non-fatal myocardial infarction, non-fatal ischemic stroke, death, and bleeding events (13).
Several cardiovascular disease risk and prognosis assessment tools have been established in different populations to guide clinical practice (14–16). Unfortunately, these risk scores are limited by their short follow-up durations and may not accurately reflect the prognosis of ACS patients, particularly in economically disadvantaged regions where long-term risk stratification for post-PCI ACS patients is unclear. Furthermore, the Human Development Index (HDI) is strongly associated with the CHD prevalence in developing countries, and the current allocation of healthcare resources to county-level hospitals in LMIC is relatively low. Therefore, risk assessment for long-term postoperative surveillance should be simple and suitable for remote follow-up to ensure the continued enhancement of quality healthcare services (17, 18).
Consequently, our study aimed to validate a prognostic model capable of predicting the likelihood of experiencing MACEs in ACS patients who underwent PCI at a county-level hospitals. We employed a multicenter external validation strategy to serve as a reference for the swift screening of high-risk individuals and early clinical intervention. Moreover, our study aimed to foster the advancement of quality healthcare in regions with limited healthcare resources.
2. Material and methods
2.1. Study population and design
From September 2018 to August 2019, a prospective nested case-control study was undertaken on a primary cohort of ACS patients enrolled for PCI at six county-level hospitals within Liaoning Province, China. Across all study centers, 1,795 individuals were diagnosed with ACS and treated with PCI based on their clinical conditions. A total of 1,741 patients were followed up from recruitment to August 2022, and 3.0% of cases (n = 54) were removed due to incomplete clinical information and exclusion criteria. A total of 1,581 patients were finally analyzed after three years of follow-up. The study enrolment and results are shown in Supplementary Figure S2. The original training set used for the prognostic evaluation was constructed using three centers (n = 520) that were similar to one another in terms of spatial and socioeconomic features (Supplementary Figure S1). Additionally, the expected sample size was calculated based on 20 events per variable (EPV), and the model was prospectively evaluated using three other cohorts (n = 1,061).The case group consisted of 143 ACS patients who experienced a major adverse cardiovascular event during the follow-up period from September 2018 to August 2019. On the other hand, the control group included patients who did not experience an adverse cardiovascular event until the end of the follow-up period.
The following were the inclusion criteria: (1) Patients with ACS who met the Chinese Medical Association's guidelines for diagnosis and treatment of acute coronary syndromes (supplementary methods contain definitions for each subset of ACS); (2) Three years following PCI, patients with complete revascularization. The exclusion criteria were as follows: (1) Patients with hematologic disease, multiple organ failure, or a cancer diagnosis; (2) Patients with significant comorbidity, trauma, or surgery; (3) Patients with incomplete hospitalisation registration information that cannot be followed up; (4) Patients who died within 30 days.
The study was approved by the institutional review board of the First Hospital of China Medical University [approval number: (2019) 189]. Written informed consent was obtained from all surviving participants or the next of kin who provided information about the deceased participants.
The study adheres to the Transparent Reporting of a Multivariable Prediction Model for Individual Prognosis or Diagnosis (TRIPOD) reporting guidelines (19).
2.2. Clinical endpoint and definitions
The primary endpoint of this study was MACEs, defined as a composite of stroke, heart failure, target lesion revascularization, recurrent myocardial infarction, and all-cause death.
2.3. Data collection and follow-up
The data collected in our study were obtained from scans at various hospitals, and not all participating hospitals were connected to a common electronic health record (EHR) system. Our research team prioritized data privacy and security. We ensured adherence to ethical and legal standards during our data extraction procedures while also implementing robust quality control measures to uphold accuracy and consistency.
Baseline characteristics (patient demographic data, medical history, preoperative clinical characteristics, coronary angiography features, laboratory indicators, echocardiography indices, and medication use during hospitalization) of the training set and external validation set are shown in Table 1.
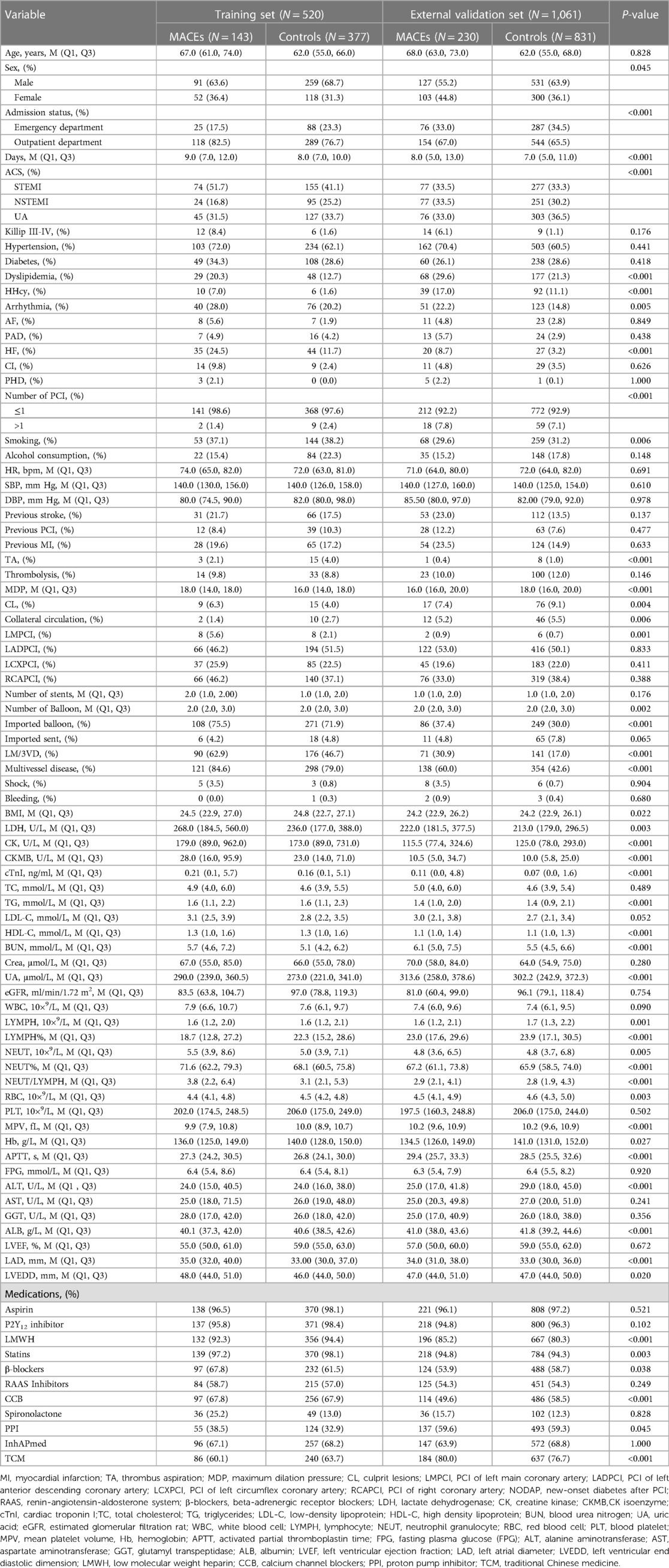
Table 1. Demographic and clinical characteristics of ACS patients in the training and external validation set.
Through telephone (to the patients themselves or their relatives), patients were followed up in the first, second, and third years post-PCI, with no further follow-up if a death event was recorded. During follow-up, medication use, daily behavioral habits, and outcome events, including all-cause death, target lesion revascularization, recurrent myocardial infarction, stroke, heart failure, rehospitalization for cardiac reasons, and major bleeding events, were all recorded. The general condition of the patients and the medications taken during the follow-up period are depicted in Supplementary Table S3.
2.4. Statistical analysis
Normally distributed variables were summarized as means and standard deviations, medians and interquartile ranges represented skewed distributional data, and frequencies or proportions were used to describe categorical variables. The t-test, the rank-sum test, and the χ2 test were applied to compare continuous variables of normal distribution, continuous variables of non-normal distribution, and categorical variables, respectively.
As candidate predictors, 97 clinical features with at least 70% data completeness were evaluated. For the missing values, multiple imputation was performed using random forest. The most useful predictors were filtered using the Least Absolute Shrinkage and Selection Operator (LASSO) regression, which was additionally augmented with 10-fold cross-validation for internal validation, incorporating penalty parameter tuning based on minimum criteria and 1 standard error (SE) of the minimum criteria. The detailed description of the LASSO model is shown in Section 1.3 of the Supplementary Material. The most predictive covariates were selected by lambda.1se. The predictor factors discovered by the LASSO regression analysis were incorporated using the multivariate logistic regression model. Subsequently, the predictor variables that consistently achieved statistical significance were used to generate the risk score and were represented by the nomogram.
Furthermore, Restricted Cubic Splines (RCS) were used to analyze the association of continuous variables with MACEs incidence among ACS patients post-PCI. The reference values (OR = 1) were set at the 10th percentiles, and four knots were placed at the 5th, 35th, 65th, and 95th percentiles of the distribution, respectively. Following that, continuous variables that reported non-linear association were transformed based on RCS and clinical experience to develop an improved predictive model. All statistical analyses were performed using SPSS (version 26.0) and R software (Version R-4.1.3).
3. Results
3.1. Characteristics of patients and outcome
After excluding those who were lost to follow-up or had missing data, the study comprised 1,581 ACS patients who underwent PCI. The training and validation sets included 520 and 1,061 patients, respectively. The baseline and follow-up characteristics of patients in the training and validation sets are respectively displayed in Table 1 and Supplementary Table S3. During follow-up, MACEs were detected in 143 (27.5%) but not in 377 training data set instances and in 230 (21.7%) but not in 831 validation set cases. The distribution of clinical outcomes in ACS patients with different subtypes of coronary artery disease in the training and external validation sets is shown in Supplementary Tables S1,S2.
3.2. Predictor selection
The LASSO regression included 97 factors evaluated at admission and follow-up (Table 1 and Supplementary Table S3). Twenty-one variables (Age, Killip Functional Classification III-IV (Killip III-IV), Hypertension, dyslipidemia, Hyperhomocysteinemia (HHcy), Heart Failure (HF), Chronotropic Incompetence (CI), Pulmonary Heart Disease (PHD), Number of stents, Multivessel disease, New-Onset Diabetes after PCI (NODAP), Aspartate Aminotransferase (AST), Low-Density Lipoprotein Cholesterol (LDL-C), estimated Glomerular Filtration Rate (eGFR), Total Cholesterol (TC), Blood Urea Nitrogen (BUN), Absolute Neutrophil Count (ANC), Left Ventricular Ejection Fraction (LVEF), Left Atrial Diameter (LAD), Maximum Dilation Pressure (MDP), P2Y12 Receptor Antagonist (P2Y12-RA) use after PCI were found to continue to be significant predictors of MACEs (Supplementary Figure S3).
3.3. Association between continuous variables and predicted outcomes
The correlation between continuous variables selected through LASSO regression and the anticipated outcome was analyzed before developing the prognostic program. We employed restricted cubic splines (RCS) to graphically represent non-linear associations (Supplementary Table S4). In both the training and external validation sets, the variable LVEF showed a non-linear association with the predicted outcome MACEs (Supplementary Figure S4).
3.4. Development of the multivariate prognostic nomogram
According to the univariate and multivariate logistic regression analysis (P < 0.05) (Table 2), 9 out of 21 prospective clinical factors were independently statistically significant predictors of MACEs in the training set and were incorporated in the prognostic nomogram (Figure 1). These variables included Age (OR, 1.08; 95% CI, 1.06–1.11; P < 0.001), Killip III-IV (OR, 4.65; 95% CI, 1.43–15.14; P = 0.011), Hypertension (OR, 1.81; 95% CI, 1.11–2.97; P = 0.018), HHcy (OR, 4.99; 95% CI, 1.46–17.04; P = 0.010), HF (OR, 1.83; 95% CI, 1.02–3.26; P = 0.042), Number of stents (OR, 1.40; 95% CI, 1.09–1.80; P = 0.008), Multivessel disease (OR, 1.78; 95% CI, 1.13–2.83; P = 0.014), LDLC (OR, 1.54; 95% CI, 1.22–1.93; P < 0.001), and LVEF (OR, 0.26; 95% CI, 0.13–0.49; P < 0.001). A straight line drawn from the point axis upward connected each predictor in a prognostic nomogram to a specific point. The “Total Points” axis was used to display the sum of scores for each variable. The plotted “Total Points” axis was subsequently connected directly to the probability axis by a vertical line to determine the probability of MACEs.
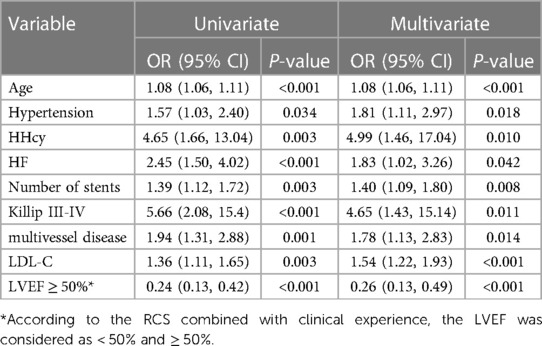
Table 2. Univariate and multivariable logistics regression analysis of predictive variables in the training set.
3.5. Performance of the prognostic nomogram
The Area Under the ROC Curve (AUC) for the model in the training set was 0.79 (95% CI 0.75–0.84), which showed excellent discrimination compared to the GRACE score (Supplementary Figure S5a). The model's cutoff value was 0.30, and based on the optimal cutoff points, the sensitivity and specificity were 76.90% and 69.50%, respectively. The calibration plot for the risk of MACEs demonstrated a substantial agreement between nomogram prediction and actual observation (Supplementary Figure S6a).
3.6. Validation of the prognostic nomogram
In the validation set, the nomogram also demonstrated superior ability in predicting the risk of MACEs after PCI compared to the GRACE score. According to the ROC curve, the AUC value of the model was 0.75 (95% CI, 0.71–0.78) (Supplementary Figure S5b), and with these results, the validation set likewise confirmed the nomogram's favorable calibration (Supplementary Figure S6b).
3.7. Clinical utility
Furthermore, the clinical validity of the nomogram regarding its clinical utility was evaluated using a Decision Curve Analysis (DCA) and Clinical Impact Curve (CIC) (Supplementary Figures S7,S8). The DCA showed that the decision curve of the model is located above the None and All lines when the threshold probability of MACEs post-PCI in ACS patients was between 0.08 and 0.98, implying that, within that reasonable level, the prediction model delivered a significant net benefit. In other words, using the prognostic nomogram to predict postoperative MACEs in ACS patients was more favorable than the all-patient tactics or the no-patient tactics. In addition, when compared to the GRACE score, the prognostic nomogram showed a larger range of thresholds as well as greater net benefit over much of the threshold range, indicating that it is a substantially superior scoring system. The CIC shows the clinical validity of the prognostic nomogram. When the threshold probability was greater than the 65% predictive score probability value, the prognostic nomogram determined that the population at high risk for MACEs was highly matched to the population that actually experienced MACEs, confirming the high clinical effectiveness of the prognostic nomogram. In conclusion, these findings supported the clinical applicability and accuracy of the nomogram in predicting the probability of MACEs in ACS patients post-PCI.
4. Discussion
Based on the increased CHD incidence, its health and economic burdens have increased considerably (20). Although the prognosis of CHD patients has improved due to expanded PCI use, estimating the long-term risk of MACEs among ACS patients post-PCI is frequently still required due to residual cardiovascular risk (21, 22). Atherosclerosis is a chronic disease process that dynamically develops in the cardiovascular setting (23). Several factors related to inflammation, the immune system, and metabolic disorders arising from genetic, environmental, and behavioral drivers accelerate the progression of atherosclerosis, which subsequently contributes to the development of ACS (24–29). Thus, rather than any single factor, the onset and progression of ACS and its residual risk are influenced by the interaction of multisystemic factors.
Several risk and prognosis assessment tools for cardiovascular diseases have been developed to guide clinical practice by identifying individuals at increased risk for MACEs across various populations (30–33). These tools, along with their corresponding scores, can inform clinical decisions for secondary prevention by identifying high-risk patients who might require ancillary clinical assistance and resources. However, in addition to some of them requiring complex completion procedures, the clinical validity of these scores is limited. Furthermore, different studies have revealed discrepancies in prognostic judgments for the above scores. For example, GRACE, TIMI, Zwolle, and CADILLAC scores were employed to analyze the 5-year prognosis of STEMI patients post-PCI. Kozieradzka et al. found that for predicting all-cause mortality, the CADILLAC model had the lowest discrimination (14). After comparing the prognostic accuracy of six scoring models for three-year mortality in STEMI patients, Jarkovsky et al. discovered that longer follow-up periods could best be predicted by GRACE (15). Scruth et al., on the other hand, concluded that CADILLAC and TIMI scores were better predictors of major cardiac events at one year (16).
Several currently popular models used to evaluate the risk of cardiovascular disease may not have been adequately optimized for long-term prognosis and the consistent use of scoring by clinicians over time. These models exhibit varying outcomes and pose significant decision-making risks in real-world clinical settings, particularly among patients with acute coronary syndrome undergoing percutaneous coronary intervention at county-level hospitals. Consequently, the present study aimed to integrate routinely available characteristics during PCI treatment and follow-up in multicenter county-level hospitals to develop a nomogram for predicting the occurrence of MACEs after PCI in ACS patients. The population included in this study was ACS patients treated with PCI from 2018 to 2019, which is closer to the real world than previously registered scores, and the nomogram modelling demonstrated more accurate predictive efficacy than the conventional Grace scoring system. Consequently, our nomogram can serve as a valuable adjunct to existing risk scores.
Previous research has linked the clinical profile of ACS patients at admission to prognosis, with age, cardiac insufficiency, and blood pressure clinically recognized as independent markers of poor prognosis following PCI in ACS patients (34–53).
In addition to being the most commonly used measure of left ventricular systolic function, Left Ventricular Ejection Fraction (LVEF), as measured by transthoracic echocardiography, is one of the strongest predictors of MACEs occurrence post-PCI in patients with coronary artery disease. At admission, the LVEF determines the extent of the decline in the left ventricle's systolic function. Individuals are more likely to experience severe cardiovascular endpoint events due to the long-term decline in cardiac output caused by myocardial infarction. According to the American Society of Echocardiography and the European Society of Cardiovascular Imaging, specific thresholds of 52% and 54% for men and women, respectively, define an increased risk of left ventricular dysfunction and early death (54). Tajstra et al. demonstrated that in ischemic heart failure (LVEF ≤ 35%), patients with chronic, completely occlusive lesions had a worse long-term prognosis (55). An observational study of 230,464 cases from the British Cardiovascular Intervention Society angioplasty database revealed that compared to patients with preserved ejection fraction (50%), patients with moderately impaired LV ejection fraction (30%–49%) had a threefold increase in 30-day post-PCI mortality (56). As revealed by a study summarizing five randomized clinical trials, patients with reduced ejection fraction (<40%) or median ejection fraction (40%–49%) had an increased risk of all-cause mortality, cardiac death, and a composite risk of cardiac death in the context of coronary artery disease treated with clinically indicated PCI (57). In contrast, recent cohort studies have shown inconsistent findings, with ejection fraction below 60% or over 65% being associated with an increased mortality risk. These findings contradict the previous consensus that associated an increased mortality risk only with severely reduced ejection fraction (58). Furthermore, an Australian study discovered that at higher ejection fraction levels, women had a greater risk of death (59). Since these studies were conducted on the general population undergoing echocardiography, the association between LVEF and adverse outcomes in coronary artery disease patients following PCI remains unclear. Herein, we attempted to examine the connection between LVEF and the risk of MACEs in ACS patients undergoing PCI in a large observational cohort of county-level multicenter hospitals. Using restricted cubic splines, we evaluated a non-linear relationship between LVEF and adverse cardiovascular events. We discovered that compared with patients with preserved ejection fraction (>50%), patients with reduced ejection fraction (≤50%) had a higher incidence of post-PCI MACEs. This result is consistent with the findings of a prior study, which indicated that when LVEF was evaluated using cardiac magnetic resonance, the TIMI risk score had an improved capacity to predict all-cause mortality, reinfarction, and new-onset congestive heart failure within a year following infarction (60). In addition, to minimize the confounding effect of different assessment times, we completed all echocardiographic assessments during hospitalization in our study. Images were not evaluated by a central laboratory in our study, which may introduce subjectivity in the interpretation of echocardiographic data. However, our goal was to reflect true clinical practice, in which ultrasound assessments are performed by a variety of health care providers.
Additionally, the multivessel disease was found to be an independent predictor of MACEs in patients post-PCI (60, 61). An increased number of diseased vessels indicates more extensive and complex coronary lesions, necessitating more stents, balloons, and other interventional devices used during PCI, which substantially increase the risk of damage to coronary vessels and cardiomyocytes, and in turn leading to thrombosis and microcirculatory disorders, consequently increasing the risk of post-PCI adverse events (42, 53, 62, 63).
The other independent factor in predicting adverse events in ACS patients was serum homocysteine (Hcy) levels. Homocysteine-induced endothelial dysfunction, lipid peroxidation, and peroxisome proliferator-activated receptor inactivation may enhance smooth muscle cell proliferation, extracellular matrix production, and MACE risk after PCI (64). Secondary prevention using folic acid and other B vitamins failed to prevent or minimize cardiovascular events in some randomized controlled studies, despite a connection between raised plasma HCY levels and late cardiac events and poor prognosis in CAD patients (65–70). Thus, more clinical trials are needed to demonstrate the predictive usefulness of HCY levels on coronary atherosclerosis development, late stent failure, and long term clinical outcomes.
Following the adjustment for other clinical traits in the study's participants, we also observed that LDL-C levels remained associated with the risk of adverse events in PCI recipients. This finding stresses the importance of appropriate risk reduction methods as it underlines the large residual risk in these patients despite successful revascularization. Furthermore, a multicenter study investigating the association between LDL-C and long-term cardiovascular events post-PCI linked higher LDL-C levels with an increased risk of late cardiovascular events (71). Therefore, prompt initiation of intensive statin therapy may provide early clinical benefit after ACS, and long-term adherence to optimal lipid-lowering therapy may effectively reduce long-term cardiovascular events post-PCI (65).
Notably, due to urbanization, significant geographical disparities were observed in the course and prognosis of ACS patients in developing nations, with provincial hospital patients being younger and having lower fatality rates than those treated in district hospitals (72, 73). Furthermore, the Prospective Urban Rural Epidemiologic (PURE) study revealed that underprivileged populations in low-income countries faced challenges regarding access to primary and secondary prevention (5). As a quantitative tool for evaluating clinical risk and benefit, our nomogram has demonstrated strong predictive performance, enabling clinicians to promptly identify patients in need of active attention and frequent follow-up. This allows for early prognostic risk assessment, the development of personalized treatment plans, and the establishment of follow-up schedules to effectively guide patient management. Early detection and diagnosis of MACEs after PCI in patients with ACS can be achieved by predicting their increased risk during follow-up. This can be done through intensive electrocardiogram monitoring in community healthcare units. Implementing this approach can help ensure consistent and standardized preventive treatment for high-risk populations in remote areas. Additionally, it can address the challenges of limited access to hospitals and high hospitalization costs for low-income populations.
5. Limitations
First, this study created a clinical prediction model by examining independent risk factors for long-term MACEs in PCI patients. Thus, the chosen indicators primarily comprise those commonly employed in clinical contexts.
Second, the study exclusively assessed the prognostic accuracy of the predictive nomogram in ACS patients undergoing PCI. More research is needed to determine whether the predictive nomogram exerts a similar clinical effect on ACS patients undergoing alternative therapies, such as coronary artery bypass graft.
Third, due to the low reliability of telephone follow-up, the study has not distinguished between cardiovascular and non-cardiovascular as well as cancer-specific causes of mortality.
Fourth, since the study was conducted across multiple centers, there were variations in surgical equipment and physician experience. As a result, the PCI outcomes were inevitably impacted, potentially affecting the prognosis of patients. Therefore, the findings of this investigation could be compromised.
Fifth, this study has not directly compared patient outcomes with those of patients treated at other hospital levels. Therefore, the findings are exclusive to the county-level hospital environment.
6. Conclusion
In summary, our nomogram aims to provide novel perspectives for county-level post-PCI rehabilitation programs in LMIC, and consequently lower the incidence of severe adverse cardiovascular events in post-PCI patients.
Data availability statement
The raw data supporting the conclusions of this article will be made available by the authors, without undue reservation.
Ethics statement
The studies involving humans were approved by Institutional Review Board of the First Hospital of China Medical University. The studies were conducted in accordance with the local legislation and institutional requirements. The participants provided their written informed consent to participate in this study.
Author contributions
YL: Formal Analysis, Methodology, Writing – original draft, Writing – review & editing. YW: Investigation, Writing – review & editing. BZ: Conceptualization, Writing – review & editing.
Funding
The author(s) declare that no financial support was received for the research, authorship, and/or publication of this article.
Acknowledgments
We thank all subjects and colleagues for participating in the study.
Conflict of interest
The authors declare that the research was conducted in the absence of any commercial or financial relationships that could be construed as a potential conflict of interest.
Publisher's note
All claims expressed in this article are solely those of the authors and do not necessarily represent those of their affiliated organizations, or those of the publisher, the editors and the reviewers. Any product that may be evaluated in this article, or claim that may be made by its manufacturer, is not guaranteed or endorsed by the publisher.
Supplementary material
The Supplementary Material for this article can be found online at: https://www.frontiersin.org/articles/10.3389/fcvm.2023.1297527/full#supplementary-material
References
1. GBD 2019 Diseases and Injuries Collaborators. Global burden of 369 diseases and injuries in 204 countries and territories, 1990–2019: a systematic analysis for the global burden of disease study 2019. Lancet. (2020) 396(10258):1204–22. doi: 10.1016/s0140-6736(20)30925-9
2. O’Flaherty M, Allender S, Taylor R, Stevenson C, Peeters A, Capewell S. The decline in coronary heart disease mortality is slowing in young adults (Australia 1976–2006): a time trend analysis. Int J Cardiol. (2012) 158(2):193–8. doi: 10.1016/j.ijcard.2011.01.016
3. Ananth CV, Rutherford C, Rosenfeld EB, Brandt JS, Graham H, Kostis WJ, et al. Epidemiologic trends and risk factors associated with the decline in mortality from coronary heart disease in the United States, 1990–2019. Am Heart J. (2023) 263:46–55. doi: 10.1016/j.ahj.2023.05.006
4. Writing Committee of the Report on Cardiovascular Health and Diseases in China. Report on cardiovascular health and diseases in China 2021: an updated summary. Biomed Environ Sci. (2022) 35(7):573–603. doi: 10.3967/bes2022.079
5. Rosengren A, Smyth A, Rangarajan S, Ramasundarahettige C, Bangdiwala SI, AlHabib KF, et al. Socioeconomic status and risk of cardiovascular disease in 20 low-income, middle-income, and high-income countries: the prospective urban rural epidemiologic (pure) study. Lancet Glob Health. (2019) 7(6):e748–60. doi: 10.1016/s2214-109x(19)30045-2
6. Xavier D, Pais P, Devereaux PJ, Xie C, Prabhakaran D, Reddy KS, et al. Treatment and outcomes of acute coronary syndromes in India (create): a prospective analysis of registry data. Lancet. (2008) 371(9622):1435–42. doi: 10.1016/s0140-6736(08)60623-6
7. Fuster V, Kovacic JC. Acute coronary syndromes: pathology, diagnosis, genetics, prevention, and treatment. Circ Res. (2014) 114(12):1847–51. doi: 10.1161/circresaha.114.302806
8. Kumar A, Cannon CP. Acute coronary syndromes: diagnosis and management, part I. Mayo Clin Proc. (2009) 84(10):917–38. doi: 10.1016/s0025-6196(11)60509-0
9. Bhatt DL, Lopes RD, Harrington RA. Diagnosis and treatment of acute coronary syndromes: a review. JAMA. (2022) 327(7):662–75. doi: 10.1001/jama.2022.0358
10. Kamran H, Jneid H, Kayani WT, Virani SS, Levine GN, Nambi V, et al. Oral antiplatelet therapy after acute coronary syndrome: a review. JAMA. (2021) 325(15):1545–55. doi: 10.1001/jama.2021.0716
11. Hoole SP, Bambrough P. Recent advances in percutaneous coronary intervention. Heart. (2020) 106(18):1380–6. doi: 10.1136/heartjnl-2019-315707
12. Chacko L, Howard JP, Rajkumar C, Nowbar AN, Kane C, Mahdi D, et al. Effects of percutaneous coronary intervention on death and myocardial infarction stratified by stable and unstable coronary artery disease: a meta-analysis of randomized controlled trials. Circ Cardiovasc Qual Outcomes. (2020) 13(2):e006363. doi: 10.1161/circoutcomes.119.006363
13. Zhang S, Wang W, Sawhney JPS, Krittayaphong R, Kim HS, Nhan VT, et al. Antithrombotic management and long-term outcomes following percutaneous coronary intervention for acute coronary syndrome in Asia. Int J Cardiol. (2020) 310:16–22. doi: 10.1016/j.ijcard.2020.01.008
14. Kozieradzka A, Kamiński KA, Maciorkowska D, Olszewska M, Dobrzycki S, Nowak K, et al. Grace, timi, zwolle and cadillac risk scores–do they predict 5-year outcomes after st-elevation myocardial infarction treated invasively? Int J Cardiol. (2011) 148(1):70–5. doi: 10.1016/j.ijcard.2009.10.026
15. Littnerova S, Kala P, Jarkovsky J, Kubkova L, Prymusova K, Kubena P, et al. Grace score among six risk scoring systems (cadillac, pami, timi, dynamic timi, zwolle) demonstrated the best predictive value for prediction of long-term mortality in patients with st-elevation myocardial infarction. PLoS One. (2015) 10(4):e0123215. doi: 10.1371/journal.pone.0123215
16. Scruth EA, Cheng E, Worrall-Carter L. Risk score comparison of outcomes in patients presenting with st-elevation myocardial infarction treated with percutaneous coronary intervention. Eur J Cardiovasc Nurs. (2013) 12(4):330–6. doi: 10.1177/1474515112449412
17. Zhu KF, Wang YM, Zhu JZ, Zhou QY, Wang NF. National prevalence of coronary heart disease and its relationship with human development index: a systematic review. Eur J Prev Cardiol. (2016) 23(5):530–43. doi: 10.1177/2047487315587402
18. Zhong Q, Gao Y, Zheng X, Chen J, Masoudi FA, Lu Y, et al. Geographic variation in process and outcomes of care for patients with acute myocardial infarction in China from 2001 to 2015. JAMA Netw Open. (2020) 3(10):e2021182. doi: 10.1001/jamanetworkopen.2020.21182
19. Collins GS, Reitsma JB, Altman DG, Moons KG. Transparent reporting of a multivariable prediction model for individual prognosis or diagnosis (tripod): the tripod statement. Ann Intern Med. (2015) 162(1):55–63. doi: 10.7326/m14-0697
20. Zheng X, Curtis JP, Hu S, Wang Y, Yang Y, Masoudi FA, et al. Coronary catheterization and percutaneous coronary intervention in China: 10-year results from the China peace-retrospective cathpci study. JAMA Intern Med. (2016) 176(4):512–21. doi: 10.1001/jamainternmed.2016.0166
21. Ajala ON, Everett BM. Targeting inflammation to reduce residual cardiovascular risk. Curr Atheroscler Rep. (2020) 22(11):66. doi: 10.1007/s11883-020-00883-3
22. Hoogeveen RC, Ballantyne CM. Residual cardiovascular risk at low ldl: remnants, lipoprotein(a), and inflammation. Clin Chem. (2021) 67(1):143–53. doi: 10.1093/clinchem/hvaa252
23. Libby P, Buring JE, Badimon L, Hansson GK, Deanfield J, Bittencourt MS, et al. Atherosclerosis. Nat Rev Dis Primers. (2019) 5(1):56. doi: 10.1038/s41572-019-0106-z
24. Wolf D, Ley K. Immunity and inflammation in atherosclerosis. Circ Res. (2019) 124(2):315–27. doi: 10.1161/circresaha.118.313591
25. Poznyak A, Grechko AV, Poggio P, Myasoedova VA, Alfieri V, Orekhov AN. The diabetes mellitus-atherosclerosis connection: the role of lipid and glucose metabolism and chronic inflammation. Int J Mol Sci. (2020) 21(5):1835. doi: 10.3390/ijms21051835
26. Ridker PM, Everett BM, Thuren T, MacFadyen JG, Chang WH, Ballantyne C, et al. Antiinflammatory therapy with canakinumab for atherosclerotic disease. N Engl J Med. (2017) 377(12):1119–31. doi: 10.1056/NEJMoa1707914
27. Gallone G, Baldetti L, Pagnesi M, Latib A, Colombo A, Libby P, et al. Medical therapy for long-term prevention of atherothrombosis following an acute coronary syndrome: JACC state-of-the-art review. J Am Coll Cardiol. (2018) 72(23 Pt A):2886–903. doi: 10.1016/j.jacc.2018.09.052
28. Eikelboom JW, Connolly SJ, Bosch J, Dagenais GR, Hart RG, Shestakovska O, et al. Rivaroxaban with or without aspirin in stable cardiovascular disease. N Engl J Med. (2017) 377(14):1319–30. doi: 10.1056/NEJMoa1709118
29. Mauri L, Kereiakes DJ, Yeh RW, Driscoll-Shempp P, Cutlip DE, Steg PG, et al. Twelve or 30 months of dual antiplatelet therapy after drug-eluting stents. N Engl J Med. (2014) 371(23):2155–66. doi: 10.1056/NEJMoa1409312
30. Fox KA, Dabbous OH, Goldberg RJ, Pieper KS, Eagle KA, Van de Werf F, et al. Prediction of risk of death and myocardial infarction in the six months after presentation with acute coronary syndrome: prospective multinational observational study (grace). Br Med J. (2006) 333(7578):1091. doi: 10.1136/bmj.38985.646481.55
31. Halkin A, Singh M, Nikolsky E, Grines CL, Tcheng JE, Garcia E, et al. Prediction of mortality after primary percutaneous coronary intervention for acute myocardial infarction: the cadillac risk score. J Am Coll Cardiol. (2005) 45(9):1397–405. doi: 10.1016/j.jacc.2005.01.041
32. Antman EM, Cohen M, Bernink PJ, McCabe CH, Horacek T, Papuchis G, et al. The timi risk score for unstable angina/non-st elevation mi: a method for prognostication and therapeutic decision making. JAMA. (2000) 284(7):835–42. doi: 10.1001/jama.284.7.835
33. De Luca G, Suryapranata H, van ‘t Hof AW, de Boer MJ, Hoorntje JC, Dambrink JH, et al. Prognostic assessment of patients with acute myocardial infarction treated with primary angioplasty: implications for early discharge. Circulation. (2004) 109(22):2737–43. doi: 10.1161/01.Cir.0000131765.73959.87
34. Kong S, Chen C, Zheng G, Yao H, Li J, Ye H, et al. A prognostic nomogram for long-term major adverse cardiovascular events in patients with acute coronary syndrome after percutaneous coronary intervention. BMC Cardiovasc Disord. (2021) 21(1):253. doi: 10.1186/s12872-021-02051-0
35. Pedersen F, Butrymovich V, Kelbæk H, Wachtell K, Helqvist S, Kastrup J, et al. Short- and long-term cause of death in patients treated with primary Pci for stemi. J Am Coll Cardiol. (2014) 64(20):2101–8. doi: 10.1016/j.jacc.2014.08.037
36. Yamaji K, Shiomi H, Morimoto T, Nakatsuma K, Toyota T, Ono K, et al. Effects of age and sex on clinical outcomes after percutaneous coronary intervention relative to coronary artery bypass grafting in patients with triple-vessel coronary artery disease. Circulation. (2016) 133(19):1878–91. doi: 10.1161/circulationaha.115.020955
37. Giustino G, Redfors B, Brener SJ, Kirtane AJ, Généreux P, Maehara A, et al. Correlates and prognostic impact of new-onset heart failure after st-segment elevation myocardial infarction treated with primary percutaneous coronary intervention: insights from the infuse-ami trial. Eur Heart J Acute Cardiovasc Care. (2018) 7(4):339–47. doi: 10.1177/2048872617719649
38. Hwang D, Lee JM, Yang S, Chang M, Zhang J, Choi KH, et al. Role of post-stent physiological assessment in a risk prediction model after coronary stent implantation. JACC Cardiovasc Interv. (2020) 13(14):1639–50. doi: 10.1016/j.jcin.2020.04.041
39. Qin Y, Wei X, Han H, Wen Y, Gu K, Ruan Y, et al. Association between age and readmission after percutaneous coronary intervention for acute myocardial infarction. Heart. (2020) 106(20):1595–603. doi: 10.1136/heartjnl-2019-316103
40. Ye Z, Xu Y, Tang L, Wu M, Wu B, Zhu T, et al. Predicting long-term prognosis after percutaneous coronary intervention in patients with new onset st-elevation myocardial infarction: development and external validation of a nomogram model. Cardiovasc Diabetol. (2023) 22(1):87. doi: 10.1186/s12933-023-01820-9
41. Fang C, Chen Z, Zhang J, Jin X, Yang M. Construction and evaluation of nomogram model for individualized prediction of risk of Major adverse cardiovascular events during hospitalization after percutaneous coronary intervention in patients with acute st-segment elevation myocardial infarction. Front Cardiovasc Med. (2022) 9:1050785. doi: 10.3389/fcvm.2022.1050785
42. Liu HW, Han YL, Jin QM, Wang XZ, Ma YY, Wang G, et al. One-year outcomes in patients with st-segment elevation myocardial infarction caused by unprotected left main coronary artery occlusion treated by primary percutaneous coronary intervention. Chin Med J. (2018) 131(12):1412–9. doi: 10.4103/0366-6999.233948
43. Qin Z, Du Y, Zhou Q, Lu X, Luo L, Zhang Z, et al. Nt-Probnp and major adverse cardiovascular events in patients with st-segment elevation myocardial infarction who received primary percutaneous coronary intervention: a prospective cohort study. Cardiol Res Pract. (2021) 2021:9943668. doi: 10.1155/2021/9943668
44. Song JJ, Liu YP, Wang WY, Yang J, Wen J, Chen J, et al. Development and validation of a nomogram predicting one-year mortality in patients undergoing percutaneous coronary intervention. J Geriatr Cardiol. (2022) 19(12):960–9. doi: 10.11909/j.issn.1671-5411.2022.12.003
45. Song J, Liu Y, Wang W, Chen J, Yang J, Wen J, et al. A nomogram predicting 30-day mortality in patients undergoing percutaneous coronary intervention. Front Cardiovasc Med. (2022) 9:897020. doi: 10.3389/fcvm.2022.897020
46. Hua C, Tian H, Wang Y, Zheng J, Liu P, Zhang B, et al. Development and validation of a nomogram for predicting mortality in patients with atrial fibrillation and acute coronary syndrome who underwent percutaneous coronary intervention in a Chinese multicenter cohort. Appl Bionics Biomech. (2022) 2022:2586400. doi: 10.1155/2022/2586400
47. Bo X, Liu Y, Yang M, Lu Z, Zhao Y, Chen L. Development and validation of a nomogram of in-hospital major adverse cardiovascular and cerebrovascular events in patients with acute coronary syndrome. Front Cardiovasc Med. (2021) 8:699023. doi: 10.3389/fcvm.2021.699023
48. Zhao E, Xie H, Zhang Y. A nomogram based on apelin-12 for the prediction of major adverse cardiovascular events after percutaneous coronary intervention among patients with st-segment elevation myocardial infarction. Cardiovasc Ther. (2020) 2020:9416803. doi: 10.1155/2020/9416803
49. Wang Y, Wang W, Jia S, Gao M, Zheng S, Wang J, et al. Development of a nomogram for the prediction of in-hospital mortality in patients with acute st-elevation myocardial infarction after primary percutaneous coronary intervention: a multicentre, retrospective, observational study in Hebei province, China. BMJ Open. (2022) 12(2):e056101. doi: 10.1136/bmjopen-2021-056101
50. Pan D, Xiao S, Hu Y, Pan Q, Wu Q, Wang X, et al. Clinical nomogram to predict major adverse cardiac events in acute myocardial infarction patients within 1 year of percutaneous coronary intervention. Cardiovasc Ther. (2021) 2021:3758320. doi: 10.1155/2021/3758320
51. Zheng YY, Wu TT, Gao Y, Guo QQ, Ma YY, Zhang JC, et al. A novel abc score predicts mortality in non-st-segment elevation acute coronary syndrome patients who underwent percutaneous coronary intervention. Thromb Haemost. (2021) 121(3):297–308. doi: 10.1055/s-0040-1718411
52. Zhao X, Yuan X, Zhong Y, Wang G. Risk factor analysis and construction of a prognostic model after percutaneous coronary intervention in patients with chronic total occlusion. Comput Math Methods Med. (2022) 2022:9902380. doi: 10.1155/2022/9902380
53. Li M, Hou J, Gu X, Weng R, Zhong Z, Liu S. Incidence and risk factors of in-stent restenosis after percutaneous coronary intervention in patients from southern China. Eur J Med Res. (2022) 27(1):12. doi: 10.1186/s40001-022-00640-z
54. Lang RM, Badano LP, Mor-Avi V, Afilalo J, Armstrong A, Ernande L, et al. Recommendations for cardiac chamber quantification by echocardiography in adults: an update from the American society of echocardiography and the European association of cardiovascular imaging. Eur Heart J Cardiovasc Imaging. (2015) 16(3):233–70. doi: 10.1093/ehjci/jev014
55. Tajstra M, Pyka Ł, Gorol J, Pres D, Gierlotka M, Gadula-Gacek E, et al. Impact of chronic total occlusion of the coronary artery on long-term prognosis in patients with ischemic systolic heart failure: insights from the commit-Hf registry. JACC Cardiovasc Interv. (2016) 9(17):1790–7. doi: 10.1016/j.jcin.2016.06.007
56. Almudarra SS, Gale CP, Baxter PD, Fleming SJ, Brogan RA, Ludman PF, et al. Comparative outcomes after unprotected left main stem percutaneous coronary intervention: a national linked cohort study of 5,065 acute and elective cases from the bcis registry (British cardiovascular intervention society). JACC Cardiovasc Interv. (2014) 7(7):717–30. doi: 10.1016/j.jcin.2014.03.005
57. Siontis GC, Branca M, Serruys P, Silber S, Räber L, Pilgrim T, et al. Impact of left ventricular function on clinical outcomes among patients with coronary artery disease. Eur J Prev Cardiol. (2019) 26(12):1273–84. doi: 10.1177/2047487319841939
58. Wehner GJ, Jing L, Haggerty CM, Suever JD, Leader JB, Hartzel DN, et al. Routinely reported ejection fraction and mortality in clinical practice: where does the nadir of risk lie? Eur Heart J. (2020) 41(12):1249–57. doi: 10.1093/eurheartj/ehz550
59. Stewart S, Playford D, Scalia GM, Currie P, Celermajer DS, Prior D, et al. Ejection fraction and mortality: a nationwide register-based cohort study of 499,153 women and men. Eur J Heart Fail. (2021) 23(3):406–16. doi: 10.1002/ejhf.2047
60. Eitel I, de Waha S, Wöhrle J, Fuernau G, Lurz P, Pauschinger M, et al. Comprehensive prognosis assessment by Cmr imaging after st-segment elevation myocardial infarction. J Am Coll Cardiol. (2014) 64(12):1217–26. doi: 10.1016/j.jacc.2014.06.1194
61. Liu KL, Lin SM, Chang CH, Chen YC, Chu PH. Plasma angiopoietin-1 level, left ventricular ejection fraction, and multivessel disease predict development of 1-year major adverse cardiovascular events in patients with acute st elevation myocardial infarction - a pilot study. Int J Cardiol. (2015) 182:155–60. doi: 10.1016/j.ijcard.2014.12.172
62. Cho YK, Nam CW. Percutaneous coronary intervention in patients with multi-vessel coronary artery disease: a focus on physiology. Korean J Intern Med. (2018) 33(5):851–9. doi: 10.3904/kjim.2018.006
63. Denktas AE, Paniagua D, Jneid H. Coronary physiology assessment for the diagnosis and treatment of stable ischemic heart disease. Curr Atheroscler Rep. (2016) 18(10):62. doi: 10.1007/s11883-016-0613-2
64. Schnyder G, Flammer Y, Roffi M, Pin R, Hess OM. Plasma homocysteine levels and late outcome after coronary angioplasty. J Am Coll Cardiol. (2002) 40(10):1769–76. doi: 10.1016/s0735-1097(02)02481-6
65. Koskinas KC, Mach F, Räber L. Lipid-lowering therapy and percutaneous coronary interventions. EuroIntervention. (2021) 16(17):1389–403. doi: 10.4244/eij-d-20-00999
66. Fu Z, Qian G, Xue H, Guo J, Chen L, Yang X, et al. Hyperhomocysteinemia is an independent predictor of long-term clinical outcomes in Chinese octogenarians with acute coronary syndrome. Clin Interv Aging. (2015) 10:1467–74. doi: 10.2147/cia.S91652
67. Yeh JK, Chen CC, Hsieh MJ, Tsai ML, Yang CH, Chen DY, et al. Impact of homocysteine level on long-term cardiovascular outcomes in patients after coronary artery stenting. J Atheroscler Thromb. (2017) 24(7):696–705. doi: 10.5551/jat.36434
68. Guo J, Gao Y, Ahmed M, Dong P, Gao Y, Gong Z, et al. Serum homocysteine level predictive capability for severity of restenosis post percutaneous coronary intervention. Front Pharmacol. (2022) 13:816059. doi: 10.3389/fphar.2022.816059
69. Lonn E, Yusuf S, Arnold MJ, Sheridan P, Pogue J, Micks M, et al. Homocysteine lowering with folic acid and B vitamins in vascular disease. N Engl J Med. (2006) 354(15):1567–77. doi: 10.1056/NEJMoa060900
70. Bønaa KH, Njølstad I, Ueland PM, Schirmer H, Tverdal A, Steigen T, et al. Homocysteine lowering and cardiovascular events after acute myocardial infarction. N Engl J Med. (2006) 354(15):1578–88. doi: 10.1056/NEJMoa055227
71. Sud M, Han L, Koh M, Abdel-Qadir H, Austin PC, Farkouh ME, et al. Low-density lipoprotein cholesterol and adverse cardiovascular events after percutaneous coronary intervention. J Am Coll Cardiol. (2020) 76(12):1440–50. doi: 10.1016/j.jacc.2020.07.033
72. Xu H, Yang Y, Wang C, Yang J, Li W, Zhang X, et al. Association of hospital-level differences in care with outcomes among patients with acute st-segment elevation myocardial infarction in China. JAMA Netw Open. (2020) 3(10):e2021677. doi: 10.1001/jamanetworkopen.2020.21677
Keywords: ACS, PCI, MACEs, predictive nomogram, county (district) medical center
Citation: Lu Y, Wang Y and Zhou B (2023) Predicting long-term prognosis after percutaneous coronary intervention in patients with acute coronary syndromes: a prospective nested case-control analysis for county-level health services. Front. Cardiovasc. Med. 10:1297527. doi: 10.3389/fcvm.2023.1297527
Received: 20 September 2023; Accepted: 21 November 2023;
Published: 4 December 2023.
Edited by:
Artur Dziewierz, Jagiellonian University Medical College, PolandReviewed by:
Michal Terlecki, Jagiellonian University Medical College, PolandKayode O. Kuku, National Heart, Lung, and Blood Institute (NIH), United States
© 2023 Lu, Wang and Zhou. This is an open-access article distributed under the terms of the Creative Commons Attribution License (CC BY). The use, distribution or reproduction in other forums is permitted, provided the original author(s) and the copyright owner(s) are credited and that the original publication in this journal is cited, in accordance with accepted academic practice. No use, distribution or reproduction is permitted which does not comply with these terms.
*Correspondence: Bo Zhou ripple_zhou@126.com