- 1Dongzhimen Hospital, Beijing University of Chinese Medicine, Beijing, China
- 2Institute of Cardiovascular Diseases, Beijing University of Chinese Medicine, Beijing, China
Background: The number of models developed for predicting major adverse cardiovascular events (MACE) in patients undergoing percutaneous coronary intervention (PCI) is increasing, but the performance of these models is unknown. The purpose of this systematic review is to evaluate, describe, and compare existing models and analyze the factors that can predict outcomes.
Methods: We adhered to the Preferred Reporting Items for Systematic Reviews and Meta-Analyses (PRISMA) 2020 during the execution of this review. Databases including Embase, PubMed, The Cochrane Library, Web of Science, CNKI, Wanfang Data, VIP, and SINOMED were comprehensively searched for identifying studies published from 1977 to 19 May 2023. Model development studies specifically designed for assessing the occurrence of MACE after PCI with or without external validation were included. Bias and transparency were evaluated by the Prediction Model Risk Of Bias Assessment Tool (PROBAST) and Transparent Reporting of a multivariate Individual Prognosis Or Diagnosis (TRIPOD) statement. The key findings were narratively summarized and presented in tables.
Results: A total of 5,234 articles were retrieved, and after thorough screening, 23 studies that met the predefined inclusion criteria were ultimately included. The models were mainly constructed using data from individuals diagnosed with ST-segment elevation myocardial infarction (STEMI). The discrimination of the models, as measured by the area under the curve (AUC) or C-index, varied between 0.638 and 0.96. The commonly used predictor variables include LVEF, age, Killip classification, diabetes, and various others. All models were determined to have a high risk of bias, and their adherence to the TRIPOD items was reported to be over 60%.
Conclusion: The existing models show some predictive ability, but all have a high risk of bias due to methodological shortcomings. This suggests that investigators should follow guidelines to develop high-quality models for better clinical service and dissemination.
Systematic Review Registration: https://www.crd.york.ac.uk/PROSPERO/display_record.php?RecordID=400835, Identifier CRD42023400835.
Introduction
As global populations continue to grow and age, cardiovascular diseases, particularly coronary artery disease (CAD), have emerged as significant contributors to both mortality and disability (1). Since its first introduction in 1977, percutaneous coronary intervention (PCI) techniques have seen rapid advancements. Presently, PCI is recommended for various scenarios including single-vessel disease accompanied by symptoms and ischemia, as well as early invasive treatment for acute coronary syndrome (ACS), specifically in high-risk patients (2). Despite successful revascularization, patients still face an incidence rate of approximately 20% (3) for cardiovascular events or deaths, primarily occurring within the first year after PCI (4). This has a profound impact on their prognosis and quality of life.
Major adverse cardiovascular events (MACE), which are typically defined as a combination of cardiovascular mortality, non-fatal myocardial infarction, and non-fatal stroke (3-point MACE), represent a frequently utilized outcome in cardiovascular research. When hospitalization for heart failure is also considered, it is referred to as the 4-point MACE (5). In certain studies, the scope of the MACE definition may extend to encompass additional events such as unplanned coronary revascularization, hospitalization for chest pain, arrhythmia, all-cause mortality, and others (6, 7).
Numerous studies are dedicated to pinpointing predictive factors associated with MACE occurrence. Some modifiable clinical parameters and laboratory markers have garnered attention. Indicators such as increased neutrophil-to-lymphocyte ratio (8), elevated Lp(a) level combined with heightened hs-CRP (9), the monocyte to high-density lipoprotein ratio, and Gensini score (10) are acknowledged as potential predictors of MACE in individuals undergoing PCI.
Models combined with multiple predictors may assist in identifying high-risk populations. Several predicting models (11–14) have been developed, manifesting as risk score systems or nomograms, to forecast 30-day MACE, 1-year MACE, or longer-term outcomes following PCI. However, the model performance of these models remains uncertain. The purpose of this study is to identify, describe, and appraise existing models used to predict MACE among post-PCI patients.
Materials and methods
This study was conducted in adherence to the guidelines provided by the Preferred Reporting Items for Systematic reviews and Meta-analysis (PRISMA) 2020 (15). The study was registered at PROSPERO with CRD42023400835. Since that this review comprises published studies and publicly available data, ethical approval is not deemed necessary.
Eligibility criteria
All model development studies, whether validated or not, whether the patient underwent elective or emergency PCI, are encompassed within the scope of this review. These models should have postoperative MACE as their designated outcome, and they are eligible for inclusion irrespective of the duration of follow-up. It is imperative that these studies report on the performance of the models, including but not limited to discrimination and calibration.
Studies will be excluded if they fall within the following categories: (1) Conference abstracts, editorials, expert views, notes, or letters; (2) Review or meta-analysis articles; (3) Full-text articles were not available; (4) Studies that developed CPMs exclusively for specific populations, such as patients with diabetes, chronic kidney disease, atrial fibrillation, or the elderly or women; (5) Studies that applied an existing model to a new domain or evaluated the performance of known models adding one or more new predictors; (6) Studies focused on comparing models rather than assessing their predictive capability for MACE; and (7) External validation articles lacking corresponding development data.
Search strategy
We conducted searches across the following databases: Embase, PubMed, The Cochrane Library, Web of Science, CNKI, Wanfang Data, VIP and SINOMED on 19 May 2023. All studies published from 1977 in which year the first coronary intervention was reported up until the date of literature retrieval are entirely considered. In addition, we supplemented our initial search by manually reviewing the reference lists of identified studies, aiming to minimize the possibility of missing relevant data. The search strategy takes a combination of subject words (MeSH, ENTREE, and others) and free-text terms related to PCI, CPM, and MACE. The search terms encompass a range of expressions including “Percutaneous Coronary Intervention,” “Coronary Revascularization,” “major adverse cardiovascular event,” “cardiovascular outcome,” “MACE,” “prediction model,” “risk stratification,” “risk score,” and others. Endnote X9 software (Thomson Reuters, Philadelphia, Pennsylvania, USA) was used for document management and duplicate removal. In cases where multiple reports are derived from the same population, only the most recent study will be included. Detailed search strategies are provided in Supplementary Appendix S1.
Selection process
The eligibility of studies was assessed independently (YW and SL) with a third investigator (XW) available to resolve any disagreements. Initially, articles seemingly unrelated to the intended research objectives were excluded based on the screening of titles and abstracts. Then, the studies meeting the criteria were included after a comprehensive full-text reading.
Data extraction
As the Critical Appraisal and Data Extraction for Systematic Reviews of Prediction Modelling Studies (CHARMS) Checklist (16) suggests, descriptive tables were used to extract information that encompasses the following items: (1) characteristics of studies (e.g., the authorship, publication year, country, study design, participants, outcome definition); (2) characteristics of the models (e.g., the predictors, sample size, algorithms used to select the predictors, model development method, internal validation method, model evaluation metrics such as the area under the curve(AUC)/C-index for discrimination, calibration, sensitivity, specificity; details of dealing missing data; Model presentation). Data were extracted independently by two viewers (YD and WD), and the viewers cross-checked the data before analysis.
Quality assessment
The assessment of the risk of bias and applicability was performed independently by the reviewers (WD and DW) using the PROBAST tool (17), which consists of four domains: participants, predictors, outcome, and analysis. To support the evaluation, a set of 20 questions (Supplementary Appendix S2) was answered with “yes,” “probably yes,” “no,” “probably no,” or “no information.”
Data synthesis and analysis
The key findings were summarized in a narrative manner and presented in a tabular format or graphs. In addition, we analyzed the adherence to the TRIPOD (18) statement of each study. The 22 items (Supplementary Appendix S3) covered a range of aspects including the title, abstract, methods, results, and other information. No qualitative analysis was performed in this study.
Results
Study selection
The initial search identified 5,234 potentially relevant articles, and an additional one was added through reference citation (19). After removing 945 duplicates, 4,820 articles remained. Subsequently, we screened the titles and abstracts, resulting in the exclusion of 4,146 studies that did not meet the inclusion criteria. In total, 114 articles were reviewed in full text. Following this comprehensive evaluation, we identified 23 studies for the final analysis. Among them, 11 studies were published in Chinese (20–30), while the remaining 12 studies were published in English (11–13, 31–39). The selection process is visually depicted in Figure 1.
Study and model characteristics
A total of 23 studies developed 28 new models for predicting MACE. Each study presented at least one model. Notably, four studies (24, 26, 32, 34) created multiple predictive models, incorporating distinct variables. The models sharing identical predictor variables and stemming from the same study population, yet predicting MACE at varying time intervals, were categorized as one model. A total of 12 studies addressed prognostic models for patients with ST-segment elevation myocardial infarction (STEMI) (20, 26–29, 32–34, 36–39). Six studies were centered on patients with myocardial infarction (MI) (11, 12, 21, 22, 24, 35). Meanwhile, three studies specifically focused on patients with acute coronary syndrome (13, 23, 25). Notably, Grayson et al. (31) recruited patients undergoing PCI across a spectrum of conditions including stable angina, unstable angina, acute myocardial infarction (AMI), and cardiogenic shock. He (30) did not explicitly mention the diagnostic information of the patients.
The prognostic models were mostly developed within 3 years (n = 19, 82.6%). Among the 23 studies, 20 were retrospective cohort studies, while two were prospective studies (13, 37), and one was a nested case–control study (12). Approximately 91.3% of the studies (n = 21) were constructed using Chinese populations, while one study (31) was based on England individuals, and another study (37) was based on Spain individuals. The models developed in the eight studies (21, 24, 25, 30, 33, 37, 39) did not undergo either internal or external validation. Three studies performed external validation by enrolling patients at different times (11, 31, 35). The study sample sizes ranged from 124 (22) to 23,718 (35). Events were reported in 21 studies (91.3%), ranging from 17 (34) to 2,615 (35). The main characteristics of the included studies are summarized in Table 1.
Model development and performance
All models employed regression analysis, with 15 studies (12, 20–25, 27, 28, 30, 31, 34–36, 38) using logistic regression, while eight studies (11, 13, 26, 29, 32, 33, 37, 39) using Cox regression. The models were ultimately presented in various forms, such as formulas (22, 30, 31), risk scores (35, 37), nomograms (12, 13, 20, 21, 25–29, 36, 38, 39), or combinations thereof. Detailed information regarding the modeling methods, variable selecting methods, calibration method, and model presentation can be found in Table 2. The predicted outcomes spanned from in-hospital MACE (20, 21, 25, 27, 31, 35, 36) to MACE occurring post-discharge, with follow-up periods extending up to a maximum of 5 years (11). In terms of calibration, one study (29) reported Hosmer–Lemeshow test results, while six studies (11, 13, 20, 25, 28, 32, 35) provided calibration curves. In addition, nine studies (12, 21, 26, 27, 30, 31, 34, 36, 38) reported both calibration curves and test results. A total of 7 studies (22–24, 30, 33, 37, 39) did not report calibration information. The predictors and definitions of MACE across these studies were outlined in Supplementary Table S1.
Discrimination, assessed by the area under the curve or C-index, stands as the most critical metric for evaluating model predictive performance. With the exception of one study (37), 22 studies reported model discrimination ranging from 0.638 to 0.96 (Figure 2). The AUC values for the models constructed for STEMI patients, AMI/MI patients, and ACS patients ranged from 0.666 to 0.96 (Figure 2A), 0.638 to 0.872 (Figure 2B), and 0.712 to 0.854 (Figure 2B), respectively. In the training set, the AUC values ranged from 0.72 to 0.94 (Figure 2C).
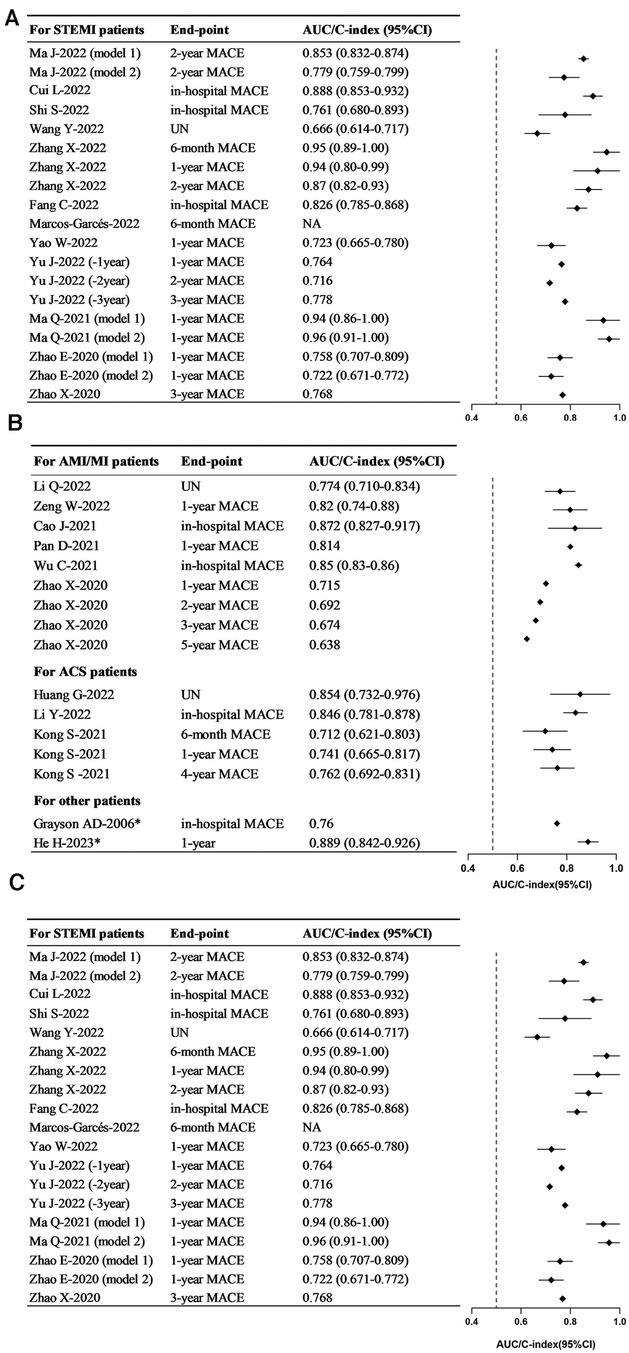
Figure 2. Forest plots in the discrimination of models. (A) Forest plot for the training set. (B) Forest plot for the training set—continued. (C) Forest plot for the validating set. STEMI, ST-segment elevation myocardial infarction; AMI, acute myocardial infarction; MI, myocardial infarction; ACS, acute coronary syndrome; MACE, major adverse cardiovascular events.
Predictor variables
The predictors were consistently standardized across studies before analysis. In various studies, the same indicators may be measured by different methods or at different time points. Some indicators, although different, share close clinical significance. In these cases, these indicators were analyzed collectively. For instance, terms such as “age” and “advanced age” were standardized as “age.” Similarly, variations such as “male,” “female,” “sex,” “female gender,” and “female sex” were standardized as “gender.” Expressions such as “Diabetes mellitus,” “diabetes,” “history of diabetes,” and “history of Diabetes mellitus (DM)” were all standardized as “diabetes.” Likewise, terms such as “Killip grade II–IV,” “Killip grade ≥ 3,” “Killip class,” “the Killip classification,” and “Killip's classification > I” were standardized as “Killip classification.” Similarly, expressions such as “left ventricular ejection fraction(LVEF),” “Low LVEF,” “ejection fraction at admission,” “CMR-LVEF <40%,” and “EF” were all standardized as “LVEF.” Terms such as “smoking history” and “smoking” were both standardized as “smoking history.” Terms such as “peak cTnI,” “cTnI,” “ TnI,” and “hypersensitive troponin T” were all harmonized to “cTnI”; “ high-sensitivity C-reactive protein (hs-CRP),” “C-reactive protein (CRP),” “hs-CRP > 10 mg/L,” and “hs-CRP level” were combined as “CRP/hs-CRP”; “N-terminal pro-B-type natriuretic peptide (NT-proBNP),” “baseline NT-proBNP,” “B-type natriuretic peptide (BNP),” and “BNP level” were combined as “BNP/NT-proBNP.” In addition, “creatinine” and “Scr” were both standardized as “Cr” and jointly analyzed alongside “estimated glomerular filtration rate eGFR.” The predictors applied in prediction models were multifarious covering demographic indicators, medical history, medications usage, lesion locations, PCI procedures, radiological indicators, and serological biomarkers. Most predictors appeared only once. In the same research where multiple models were constructed, the predictors were not counted repeatedly. The 10 most frequently occurring predictor variables in this review were as follows (Figure 3): LVEF (14/23), age (12/23), Killip classification (8/23), diabetes (7/23), Cr/eGFR (7/23), BNP/NT-proBNP (6/23), gender (5/23), cTnI (5/23), smoking (4/23), hypertension (4/23), and CRP/hs-CRP (4/23).
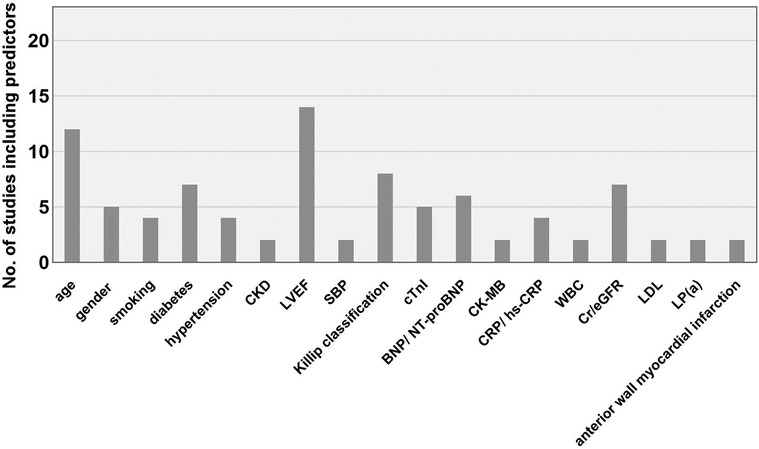
Figure 3. Main predictors of the included models. CKD, chronic kidney disease; LVEF, left ventricular ejection fraction; SBP, systolic blood pressure; BNP, brain natriuretic peptide; NT-proBNP, N-terminal pro-B-type natriuretic peptide; CK-MB, creatine kinase-MB; CRP, C-reactive protein; hs-CRP, high-sensitivity C-reactive protein; WBC, white blood cell count; LDL, low-density lipoprotein; eGFR, estimated glomerular filtration rate; Lp(a), lipoprotein(a).
Risk of bias and applicability assessment
According to the PROBAST assessment results, it was determined that all studies had a high risk of bias (Figure 4) owing to deficiencies in their study design, execution, and analysis. The participants' domain mostly consisted of data (n = 20, 86.9%) derived from retrospective cohort studies or registry data that were not originally collected for the specific purpose of developing predictive models. A total of 16 studies directly excluded cases with incomplete data or subgroups that might have an impact on predictive outcomes. This could potentially introduce higher risk of bias. In the predictors' domain, none of the studies provided any information regarding the use of a blinding method. Consequently, they all received a response of “no information” when addressing the signaling question “Were predictor assessments made without knowledge of outcome data?” In the results' domain, a predominant issue emerged: the inappropriate definition of outcomes in all studies. The outcome definition did not exclude predictor variables in five studies (20, 21, 24, 25, 30), for instance, heart failure was included in the outcome, while LVEF or NT-proBNP were used as predictors. Moreover, certain studies (20, 23, 25, 27) aimed to predict in-hospital MACE, yet they were unable to specify the timing of parameter collection. This could result in an improper time interval between the outcomes. The analysis domain is a high-risk area for bias, primarily stemming from several factors. These include sample sizes that were insufficient to fulfill the requirement of having events per variable (EPV) of ≥20 (15/23, 65.2%), the inappropriate conversion of continuous variables into categorical variables (16/23, 69.6%), the improper handling of missing data (18/23, 78.3%), and the absence of calibration reporting (6/23, 26.1%), among other factors. Predictor selection was predominantly based on univariable analysis (12, 22, 24, 26–28, 30, 37); however, it is advisable to avoid using this method. All the studies included in this review had a low risk of applicability due to the primary focus on evaluating models and identifying potential prognostic factors, with less emphasis on participant and outcome heterogeneity. The PROBAST results for each study are shown in Supplementary Table S2.
Transparent reporting assessment
The study's adherence to transparent reporting of a multivariable prediction model for individual prognosis or diagnosis (TRIPOD) items reflects the completeness of reporting the included CPM studies. In this review, the median adherence rate stands at 53%, with an interquartile range (IQR) varying from 30.4% to 100% (Figure 5).
Those poorly reported items were mainly concentrated within the Methods section. The omission of blinding was a significant issue in this review since neither the evaluation of outcomes (Item 6b) nor the evaluation of predictors (Item 7b) provided any details regarding blinding procedures or measures to prevent bias. Despite the provision of sample sizes, researchers never clarified how the study size was determined (Item 8). Moreover, no studies explained how they deal with predictors (Item 10a). Five items had adherence rates below 50%. Only two studies (2/23, 8.7%) reported on risk groups (32, 35) (Item 11) and participant selection flowcharts (35, 37) (Item 13a). Furthermore, only three studies (3/23, 13.0%) reported relevant treatment (12, 25, 28) (Item 5c). In addition, seven studies (7/23, 30.4%) adhered to the recommendation citing existing models when introducing the medical background in the Introduction section (11–13, 29, 31, 32, 35) (Item 3a). Nine studies (11–13, 32–34, 36, 37, 39) (9/23, 39.1%) provided supplementary information (Item 21). Four items were incompletely reported (50%–80% adherence). The eligibility criteria (Item 5b) were addressed in 18 studies (18/23, 78.3%). Ten studies (13/23, 56.5%) described how to use the model (Item 15ba). Fourteen studies (14/23, 60.9%) detailed the definition of the predictor (Item 7a). The funding information (Item 22) was reported in 18 out of 23 articles (78.3%) where this item was suitable. All studies completely described the following nine items (100% adherence). In the Abstract, all studies provided ample information (Item 2). Within the Methods section, the description of the study design (Item 4a) and outcome definition (Item 6a) were all presented. Studies also efficiently described the characteristics of the participants (Item 13b), model presentation (Item 15a), and performance measures (Item 16) in the Results section. Meanwhile, the Discussion section of all studies were fully organized around the study limitations (Item 18), overall interpretation (Item 19a), and potential clinical application (Item 20). The articles included in this review had 80% compliance with the remaining TRIPOD items (Supplementary Figure S1).
Discussion
Recently, there has been a noticeable increase in the number of prognostic model development studies that specifically focus on post-PCI patients. These studies frequently center on endpoints such as mortality (40–42), hemorrhage, renal injury, and atrial fibrillation (43–45). Another important endpoint that has garnered significant interest among researchers is MACE. The application of clinical prediction models can provide valuable information to patients and families and assist healthcare professionals in allocating hospital resources, potentially contributing to the improvement of healthcare quality. In addition, prediction models may aid in the clinical trial design, identifying patients with the required risk characteristics, thereby enhancing statistical power or reducing sample size and costs (46).
This systematic review provides an overview of the current landscape of models designed to predict MACE after PCI. In total, 23 articles were included in this study, presenting a total of 28 proposed models. The discriminatory power of these models varied, spanning from 0.638 to 0.96, where 66.7% of the models achieved a discrimination value exceeding 0.75. In terms of follow-up time, the AUC range for models predicting in-hospital MACE is 0.76–0.888, while the AUC range for the six models predicting 1-year MACE is 0.715–0.96. It is evident that the models explored in our study demonstrated commendable predictive powers in identifying high-risk patients.
The best-performing model (34) in this study achieved an AUC of 0.94 in the training set and an AUC of 0.90 in the validation set. This outstanding performance might be attributed to the model's reliance on a wide array of quantitative imaging parameters. Currently, polygenic risk scores, proteomics, lipidomics (47–49), and other data are gradually being introduced to predict cardiovascular events. Clearly, clinical predictive models based on easily accessible traditional risk factors may be more straightforward to apply and generalize. Collecting and utilizing such data may involve higher costs but hold the potential to improve model performance. Artificial intelligence (AI) and machine learning algorithms, including techniques such as Extreme Gradient Boosting, the Gaussian mixture model, Decision Tree, and Random Forest (50–53) have become increasingly prevalent in addressing such challenges. Machine learning with its remarkable capability to analyze extensive volumes of intricate data (54) holds tremendous potential for enhancing predictive performance and should see wider adoption in the medical field.
This review has found that the most common variables for predicting post-PCI MACE include LVEF, age, Killip classification, diabetes, Cr, BNP, gender, troponin, smoking, hypertension, and CRP. These variables encompass unmodifiable factors such as demographic data like age and gender. The included studies consistently indicate that older age is associated with worse outcomes. The odds ratio (OR) for individuals over 60 years of age is 1.212 times higher than for those under 60 years (25), while individuals aged 80 and above have a higher risk of MACE compared with those aged 70–79 (31). Several studies suggest that female patients generally have worse prognoses, although Ma et al.'s study (26) arrived at the opposite conclusion. This could be attributed to the study's male-to-female patient ratio, which was 3.5 times higher, with no adjustment made during the analysis. In the models developed for STEMI patients, the top three variables are LVEF, Killip classification, and age. For AMI patients, the most common variables include LVEF, diabetes, and age, while in ACS patients, the primary variables are LVEF, age, and BNP. There is a consistent demonstration of the value of LVEF and age for adverse outcomes of PCI patients. When analyzing these predictive factors collectively, it cannot be overlooked that the Killip classification assesses cardiac function in cases of AMI, but it is not applicable to patients with unstable angina within the ACS population. Variables assessing kidney function, such as a history of CKD, SCr, and eGFR, were also included in multiple studies. This emphasizes the need for clinical attention to both cardiac and renal function to identify individuals at risk of adverse outcomes early. In recent years, several studies have revealed the connection between inflammation and coronary heart disease. Inflammatory-related variables such as NLR, CRP, and hs-CRP were also incorporated into multiple models. Residual inflammatory risk (RIR), defined as when plasma LDL-C levels are below 1.8 mmol/L and plasma hs-CRP levels are ≥2 mg/L (55), was employed in a model constructed to predict in-hospital MACE in AMI patients after PCI (21). The model achieved an AUC value of 0.82, indicating a close correlation between RIR and recurrent cardiovascular events.
However, the reporting is not rigorous enough, and all studies were judged to be at high risk of bias. The primary factors contributing to this included retrospective study designs, the absence of blinding during the assessment of predictors or outcomes, unjustified categorization and definition of outcomes, failure to circumvent univariate analysis when screening variables, insufficient sample size for EPV, improper internal validation methods, lack of external validation, and improper handling of missing data. The PROBAST, released in 2019 (17), serves as a pivotal tool for evaluating clinical predictive modeling during systematic reviews. Intriguingly, despite 22 out of 23 articles in this review being published post-2019, they still exhibited methodological shortcomings across various facets. To enhance the quality of clinical predictive modeling and maximize the value of such models in clinical applications, it is advisable for researchers to acquaint themselves with the PROBAST tool at an early stage to minimize possible biases in the study design or data sources.
Potential clinical applications
Although these models demonstrate good predictive performance, all studies were at high overall risk of bias. This review reveals that there is insufficient evidence to apply any of these models in clinical practice. However, clinical practitioners can pay closer attention to the common variables in the models and intervene appropriately with modifiable risk factors, which may help reduce the incidence of MACE.
Strength and limitations
This study marks a notable effort in directing our attention towards predicting models for MACE in post-PCI patients. We adopted convincing tools such as PROBAST and TRIPOD in this systematic review to provide more informative results. Certain procedural characteristics, such as access site, vessel dilation, type of PCI, initial and final TIMI flow grade, stent type (drug-eluting stents or bare metal stents), and stent length, can reflect the contemporariness of the treatment and indicate the complexity of the medical condition (56). They can also serve as a basis for identifying high-risk individuals. However, these factors have not been sufficiently considered in the current models. Future researchers should give greater consideration to these aspects in their studies. In addition, the application of a language filter limiting studies to Chinese and English during literature screening may result in the omission of valuable data and insights published in other languages. This could introduce some and potentially impact the conclusions of this systematic review. Due to the limited number of relevant studies, we incorporated prediction models designed for patients undergoing PCI in all clinical settings. Nevertheless, it is essential to recognize that patient prognosis and risk factors exhibit variations in different clinical settings. Moreover, these studies vary in their definitions of MACE and the duration of follow-up. Although we made efforts to describe them categorically, the inherent heterogeneity makes further quantitative analysis challenging.
Conclusion
In summary, despite the rising number of modeling studies, the practicality of many models remains uncertain due to a lack of external validation and methodological shortcomings. It is imperative for researchers to adhere to guidelines to enhance study designs and construct models with high clinical applicability using proper internal validation and external methods.
Data availability statement
The datasets used and/or analyzed during this study are available from the corresponding author on reasonable request.
Author contributions
XW is the guarantor. WQD conceived the study and designed the search strategy. DYW provided methodological guidance. YDW and SJL were responsible for screening and data extraction. WQD, YKD, and DYW conducted quality assessments and data analysis. XW resolved any disagreements that arose throughout the process. WQD drafted the manuscript, which was subsequently revised by DYW and approved by all authors.
Funding
The author(s) declare financial support was received for the research, authorship, and/or publication of this article.
This study was supported by Special Funds for Basic Research Operating Expenses of Central Universities (2022-JYB-JBZR-033).
Conflict of interest
The authors declare that the research was conducted in the absence of any commercial or financial relationships that could be construed as a potential conflict of interest.
Publisher's note
All claims expressed in this article are solely those of the authors and do not necessarily represent those of their affiliated organizations, or those of the publisher, the editors and the reviewers. Any product that may be evaluated in this article, or claim that may be made by its manufacturer, is not guaranteed or endorsed by the publisher.
Supplementary material
The Supplementary Material for this article can be found online at: https://www.frontiersin.org/articles/10.3389/fcvm.2023.1287434/full#supplementary-material
References
1. Roth GA, Mensah GA, Johnson CO, Addolorato G, Ammirati E, Baddour LM, et al. Global burden of cardiovascular diseases and risk factors, 1990–2019: update from the GBD 2019 study. J Am Coll Cardiol. (2020) 76(25):2982–3021. doi: 10.1016/j.jacc.2020.11.010
2. Bhatt DL. Percutaneous coronary intervention in 2018. JAMA. (2018) 319(20):2127–8. doi: 10.1001/jama.2018.5281
3. Fanaroff AC, Zakroysky P, Wojdyla D, Kaltenbach LA, Sherwood MW, Roe MT, et al. Relationship between operator volume and long-term outcomes after percutaneous coronary intervention. Circulation. (2019) 139(4):458–72. doi: 10.1161/circulationaha.117.033325
4. Madhavan MV, Kirtane AJ, Redfors B, Généreux P, Ben-Yehuda O, Palmerini T, et al. Stent-related adverse events >1 year after percutaneous coronary intervention. J Am Coll Cardiol. (2020) 75(6):590–604. doi: 10.1016/j.jacc.2019.11.058
5. Peng ZY, Yang CT, Kuo S, Wu CH, Lin WH, Ou HT. Restricted mean survival time analysis to estimate Sglt2i-associated heterogeneous treatment effects on primary and secondary prevention of cardiorenal outcomes in patients with type 2 diabetes in Taiwan. JAMA Netw Open. (2022) 5(12):e2246928. doi: 10.1001/jamanetworkopen.2022.46928
6. Chen MY, Rochitte CE, Arbab-Zadeh A, Dewey M, George RT, Miller JM, et al. Prognostic value of combined Ct angiography and myocardial perfusion imaging versus invasive coronary angiography and nuclear stress perfusion imaging in the prediction of major adverse cardiovascular events: the Core320 multicenter study. Radiology. (2017) 284(1):55–65. doi: 10.1148/radiol.2017161565
7. Cheng L, Rong J, Zhuo X, Gao K, Meng Z, Wen X, et al. Prognostic value of malnutrition using geriatric nutritional risk index in patients with coronary chronic total occlusion after percutaneous coronary intervention. Clin Nutr. (2021) 40(6):4171–9. doi: 10.1016/j.clnu.2021.01.042
8. Wada H, Dohi T, Miyauchi K, Shitara J, Endo H, Doi S, et al. Pre-procedural neutrophil-to-lymphocyte ratio and long-term cardiac outcomes after percutaneous coronary intervention for stable coronary artery disease. Atherosclerosis. (2017) 265:35–40. doi: 10.1016/j.atherosclerosis.2017.08.007
9. Yuan D, Wang P, Jia S, Zhang C, Zhu P, Jiang L, et al. Lipoprotein(a), high-sensitivity C-reactive protein, and cardiovascular risk in patients undergoing percutaneous coronary intervention. Atherosclerosis. (2022) 363:109–16. doi: 10.1016/j.atherosclerosis.2022.10.013
10. Yu R, Hou R, Wang T, Li T, Han H, An J. Correlation between monocyte to high-density lipoprotein ratio and major adverse cardiovascular events in patients with acute coronary syndrome after percutaneous coronary intervention. Pak J Med Sci. (2021) 37(3):885–9. doi: 10.12669/pjms.37.3.3469
11. Zhao X, Liu C, Zhou P, Sheng Z, Li J, Zhou J, et al. Estimation of major adverse cardiovascular events in patients with myocardial infarction undergoing primary percutaneous coronary intervention: a risk prediction score model from a derivation and validation study. Front Cardiovasc Med. (2020) 7:603621. doi: 10.3389/fcvm.2020.603621
12. Pan D, Xiao S, Hu Y, Pan Q, Wu Q, Wang X, et al. Clinical nomogram to predict major adverse cardiac events in acute myocardial infarction patients within 1 year of percutaneous coronary intervention. Cardiovasc Ther. (2021) 2021:3758320. doi: 10.1155/2021/3758320
13. Kong S, Chen C, Zheng G, Yao H, Li J, Ye H, et al. A prognostic nomogram for long-term major adverse cardiovascular events in patients with acute coronary syndrome after percutaneous coronary intervention. BMC Cardiovasc Disord. (2021) 21(1):253. doi: 10.1186/s12872-021-02051-0
14. Mrdovic I, Savic L, Krljanac G, Asanin M, Perunicic J, Lasica R, et al. Predicting 30-day major adverse cardiovascular events after primary percutaneous coronary intervention. The risk-PCI score. Int J Cardiol. (2013) 162(3):220–7. doi: 10.1016/j.ijcard.2011.05.071
15. Page MJ, Moher D, Bossuyt PM, Boutron I, Hoffmann TC, Mulrow CD, et al. Prisma 2020 explanation and elaboration: updated guidance and exemplars for reporting systematic reviews. Br Med J. (2021) 372:n160. doi: 10.1136/bmj.n160
16. Moons KG, de Groot JA, Bouwmeester W, Vergouwe Y, Mallett S, Altman DG, et al. Critical appraisal and data extraction for systematic reviews of prediction modelling studies: the charms checklist. PLoS Med. (2014) 11(10):e1001744. doi: 10.1371/journal.pmed.1001744
17. Wolff RF, Moons KGM, Riley RD, Whiting PF, Westwood M, Collins GS, et al. PROBAST a tool to assess the risk of bias and applicability of prediction model studies. Ann Intern Med. (2019) 170(1):51–8. doi: 10.7326/m18-1376
18. Collins GS, Reitsma JB, Altman DG, Moons KG. Transparent reporting of a multivariable prediction model for individual prognosis or diagnosis (tripod): the tripod statement. Br Med J. (2015) 350:g7594. doi: 10.1136/bmj.g7594
19. D'Ascenzo F, De Filippo O, Gallone G, Mittone G, Deriu MA, Iannaccone M, et al. Machine learning-based prediction of adverse events following an acute coronary syndrome (praise): a modelling study of pooled datasets. Lancet. (2021) 397(10270):199–207. doi: 10.1016/S0140-6736(20)32519-8
20. Cui L, Dong S, Li C, Yu H, Han Y, Song H, et al. Construction of nomogram model to predict the risk of major 356 adverse cardiovascular events in patients with St-segment elevation myocardial infarction after primary percutaneous coronary intervention. J Chin Pract Diag Ther. (2021) 35(4):367–71. doi: 10.13507/j.issn.1674-3474.2021.04.011
21. Cao J, Zhang L, Zhan L, Ma L. Predictive value of residual inflammation risk-based nomogram model for major adverse cardiovascular events in acute myocardial infarction patients after percutaneous coronary intervention. J Third Mil Med Univ. (2021) 43(18):1821–30. doi: 10.16016/j.1000-5404.202103184
22. Zeng W, Wu A, Jiang J, Chen R, Zhang R, Wang C. Influencing factors and predictive modeling of major cardiovascular events occurring 1 year after PCI in patients with acute myocardial infarction. Chin J Gerontol. (2022) 42(20):4905–8. doi: 10.3969/j.issn.1005-9202.2022.20.001
23. Huang G, Zheng X, Chen S. Major cardiovascular events in patients with acute coronary syndrome: construction of clinical prediction model based on platelet to lymphocyte ratio. S China J Cardiovasc Dis. (2022) 28(5):411–6. doi: 10.3969/j.issn.1007-9688.2022.05.06
24. Li Q, Tan X, Jiang W, Yuan M, Ni H, Wang Y, et al. Predictive model for long-term major adverse cardiovascular events in patients with acute myocardial infarction undergoing percutaneous coronary intervention. Chin Gen Pract. (2022) 25(24):2965–74. doi: 10.12114/j.issn.1007-9572.2022.0237
25. Li Y, Liang L, Chen B, Wang Y. Construction of an in-hospital mace attack risk prediction model for patients with acute coronary syndrome after percutaneous coronary intervention. Chin Heart J. (2022) 34(5):531–6. doi: 10.12125/j.chj.202203019
26. Ma J, Liu J, Bai G, Liu X, Li Y. Construction of a predictive model based on plasma Dpp4 activity to assess the 2-year risk of MACEs events in STEMI patients after primary PCI. Med J W China. (2022) 34(3):396–401. doi: 10.3969/j.issn.1672-3511.2022.03.015
27. Shi S, Hou W, Li Z, Jia X, Ren Z. Predictive value of inflammatory factors for in-hospital major 380 adverse cardiovascular events in patients with acute ST-segment elevation myocardial infarction after percutaneous coronary intervention. Chin Heart J. (2022) 34(04):422–7. doi: 10.12125/j.chj.202110010
28. Wang Y, Yan W, Xu Y, Gu Y, Zhang M, Yang X, Song Y, et al. Risk factors of major adverse cardiovascular events and establishment of nomogram model after percutaneous coronary intervention. Chin J Evid Bases Cardiovasc Med. (2022) 14(11):1386–90. doi: 10.3969/j.issn.1674-4055.2022.11.23
29. Zhang X, Ding Y, Yao Y, Gu Y, Zhang X. Establishment and validation of prognostic risk nomogram model among patients with premature ST-segment elevation myocardial infarction. J Nanjing Med Univ. (2022) 42(11):1539–46, 52. doi: 10.7655/nydxbns20221106
30. He H. Construction and analysis of risk model for major adverse cardiovascular events in 238 patients after percutaneous coronary intervention. Chin J Clin. (2023) 51(04):409–11. doi: 10.3969/j.issn.2095-8552.2023.04.010
31. Grayson AD, Moore RK, Jackson M, Rathore S, Sastry S, Gray TP, et al. Multivariate prediction of major adverse cardiac events after 9,914 percutaneous coronary interventions in the north west of England. Heart. (2006) 92(5):658–63. doi: 10.1136/hrt.2005.066415
32. Zhao EF, Xie H, Zhang YS. A nomogram based on apelin-12 for the prediction of major adverse cardiovascular events after percutaneous coronary intervention among patients with ST-segment elevation myocardial infarction. Cardiovasc Ther. (2020) 2020:9416803. doi: 10.1155/2020/9416803
33. Zhao X, Wang Y, Liu C, Zhou P, Sheng Z, Li J, et al. Prognostic value of total bilirubin in patients with ST-segment elevation acute myocardial infarction undergoing primary coronary intervention. Front Cardiovasc Med. (2020) 7:615254. doi: 10.3389/fcvm.2020.615254
34. Ma Q, Ma Y, Wang X, Li S, Yu T, Duan W, et al. A radiomic nomogram for prediction of major adverse cardiac events in ST-segment elevation myocardial infarction. Eur Radiol. (2021) 31(2):1140–50. doi: 10.1007/s00330-020-07176-y
35. Wu C, Huo X, Liu J, Zhang L, Bai X, Hu S, et al. Development and validation of a risk prediction model for in-hospital major cardiovascular events in patients hospitalised for acute myocardial infarction. BMJ Open. (2021) 11(5):e042506. doi: 10.1136/bmjopen-2020-042506
36. Fang C, Chen Z, Zhang J, Jin X, Yang M. Construction and evaluation of nomogram model for individualized prediction of risk of major adverse cardiovascular events during hospitalization after percutaneous coronary intervention in patients with acute ST-segment elevation myocardial infarction. Front Cardiovasc Med. (2022) 9:1050785. doi: 10.3389/fcvm.2022.1050785
37. Marcos-Garcés V, Perez N, Gavara J, Lopez-Lereu MP, Monmeneu JV, Rios-Navarro C, et al. Risk score for early risk prediction by cardiac magnetic resonance after acute myocardial infarction. Int J Cardiol. (2022) 349:150–4. doi: 10.1016/j.ijcard.2021.11.050
38. Yao WS, Li J. Risk factors and prediction nomogram model for 1-year readmission for major adverse cardiovascular events in patients with STEMI after PCI. Clin Appl Thromb Hemost. (2022) 28:10760296221137847. doi: 10.1177/10760296221137847
39. Yu J, Liu Y, Peng W, Xu Z. Serum vcam-1 and icam-1 measurement assists for mace risk estimation in ST-segment elevation myocardial infarction patients. J Clin Lab Anal. (2022) 36(10):e24685. doi: 10.1002/jcla.24685
40. Zhang J, Zheng YY, Wu TT, Ma X, Ma YT, Xie X, et al. Blood routine test parameters score, a novel predictor of adverse outcomes of coronary artery disease patients with or without diabetes who underwent percutaneous coronary intervention: a retrospective cohort study. ACS Omega. (2021) 6(48):32508–16. doi: 10.1021/acsomega.1c03990
41. Liu Q, Zhang Y, Zhang PQ, Zhang JB, Cao XJ, He SS, et al. Both baseline Selvester QRS score and change in QRS score predict prognosis in patients with acute ST-segment elevation myocardial infarction after percutaneous coronary intervention. Coron Artery Dis. (2020) 31(5):403–10. doi: 10.1097/mca.0000000000000869
42. Damman P, Kuijt WJ, Woudstra P, Haeck JDE, Koch KT, Gu YLL, et al. Multiple biomarkers at admission are associated with angiographic, electrocardiographic, and imaging cardiovascular mechanistic markers of outcomes in patients undergoing primary percutaneous coronary intervention for acute ST-elevation myocardial infarction. Am Heart J. (2012) 163(5):783–9. doi: 10.1016/j.ahj.2012.01.004
43. Shoji S, Kohsaka S, Kumamaru H, Nishimura S, Ishii H, Amano T, et al. Risk prediction models in patients undergoing percutaneous coronary intervention: a collaborative analysis from a Japanese administrative dataset and nationwide academic procedure registry. Int J Cardiol. (2023) 370:90–7. doi: 10.1016/j.ijcard.2022.10.144
44. Niimi N, Shiraishi Y, Sawano M, Ikemura N, Inohara T, Ueda I, et al. Machine learning models for prediction of adverse events after percutaneous coronary intervention. Sci Rep. (2022) 12(1):6262. doi: 10.1038/s41598-022-10346-1
45. Aittokallio J, Kauko A, Vaura F, Salomaa V, Kiviniemi T, Schnabel RB, et al. Polygenic risk scores for predicting adverse outcomes after coronary revascularization. Am J Cardiol. (2022) 167:9–14. doi: 10.1016/j.amjcard.2021.11.046
46. Hizoh I, Domokos D, Banhegyi G, Becker D, Merkely B, Ruzsa Z. Mortality prediction algorithms for patients undergoing primary percutaneous coronary intervention. J Thorac Dis. (2020) 12(4):1706–20. doi: 10.21037/jtd.2019.12.83
47. Lu X, Liu Z, Cui Q, Liu F, Li J, Niu X, et al. A polygenic risk score improves risk stratification of coronary artery disease: a large-scale prospective Chinese cohort study. Eur Heart J. (2022) 43(18):1702–11. doi: 10.1093/eurheartj/ehac093
48. Helgason H, Eiriksdottir T, Ulfarsson MO, Choudhary A, Lund SH, Ivarsdottir EV, et al. Evaluation of large-scale proteomics for prediction of cardiovascular events. JAMA. (2023) 330(8):725–35. doi: 10.1001/jama.2023.13258
49. Nurmohamed NS, Kraaijenhof JM, Mayr M, Nicholls SJ, Koenig W, Catapano AL, et al. Proteomics and lipidomics in atherosclerotic cardiovascular disease risk prediction. Eur Heart J. (2023) 44(18):1594–607. doi: 10.1093/eurheartj/ehad161
50. Katsuki M, Matsumori Y, Kawamura S, Kashiwagi K, Koh A, Tachikawa S, et al. Developing an artificial intelligence-based headache diagnostic model from a clinic patients’ dataset. Headache. (2023) 63(8):1097–108. doi: 10.1111/head.14611
51. Carvantes-Barrera A, Díaz-González L, Rosales-Rivera M, Chávez-Almazán LA. Risk factors associated with COVID-19 lethality: a machine learning approach using Mexico database. J Med Syst. (2023) 47(1):90. doi: 10.1007/s10916-023-01979-4
52. Ito T, Morooka H, Takahashi H, Fujii H, Iwaki M, Hayashi H, et al. Identification of clinical phenotypes associated with poor prognosis in patients with nonalcoholic fatty liver disease via unsupervised machine learning. J Gastroenterol Hepatol. (2023) 38(10):1832–39. doi: 10.1111/jgh.16326
53. Han S, Sohn TJ, Ng BP, Park C. Predicting unplanned readmission due to cardiovascular disease in hospitalized patients with cancer: a machine learning approach. Sci Rep. (2023) 13(1):13491. doi: 10.1038/s41598-023-40552-4
54. Obermeyer Z, Emanuel EJ. Predicting the future—big data, machine learning, and clinical medicine. N Engl J Med. (2016) 375(13):1216–9. doi: 10.1056/NEJMp1606181
55. Ridker PM. Clinician’s guide to reducing inflammation to reduce atherothrombotic risk: JACC review topic of the week. J Am Coll Cardiol. (2018) 72(25):3320–31. doi: 10.1016/j.jacc.2018.06.082
56. Szabo D, Szabo A, Magyar L, Banhegyi G, Kugler S, Pinter A, et al. Admission lactate level and the grace 2.0 score are independent and additive predictors of 30-day mortality of STEMI patients treated with primary PCI-results of a real-world registry. PLoS One. (2022) 17(11):e0277785. doi: 10.1371/journal.pone.0277785
Keywords: percutaneous coronary intervention, major adverse cardiovascular events, clinical predictive models, prognosis, systematic review
Citation: Deng W, Wang D, Wan Y, Lai S, Ding Y and Wang X (2024) Prediction models for major adverse cardiovascular events after percutaneous coronary intervention: a systematic review. Front. Cardiovasc. Med. 10:1287434. doi: 10.3389/fcvm.2023.1287434
Received: 1 September 2023; Accepted: 18 December 2023;
Published: 8 January 2024.
Edited by:
Tommaso Gori, Johannes Gutenberg University Mainz, GermanyReviewed by:
Anastasios Apostolos, National and Kapodistrian University of Athens, GreeceZoltan Ruzsa, University of Szeged, Hungary
© 2024 Deng, Wang, Wan, Lai, Ding and Wang. This is an open-access article distributed under the terms of the Creative Commons Attribution License (CC BY). The use, distribution or reproduction in other forums is permitted, provided the original author(s) and the copyright owner(s) are credited and that the original publication in this journal is cited, in accordance with accepted academic practice. No use, distribution or reproduction is permitted which does not comply with these terms.
*Correspondence: Xian Wang d3g2NTA1MTVAMTI2LmNvbQ==
†These authors have contributed equally to this work and share first authorship