- 1School of Public Health (Shenzhen), Sun Yat-sen University, Shenzhen, China
- 2Guangdong Provincial Key Laboratory of Diabetology, Guangzhou Key Laboratory of Mechanistic and Translational Obesity Research, The Third Affiliated Hospital of Sun Yat-sen University, Guangzhou, China
Background: Cardiovascular disease (CVD) is an escalating global health crisis, contributing significantly to worldwide mortality and morbidity. Dyslipidemia stands as a critical risk factor for CVD. Vascular endothelial growth factor A (VEGFA) is pivotal in angiogenesis and represents a clinical target for CVD intervention. However, the impact of genetic modulation of VEGFA on lipid levels and the subsequent risk of cardiovascular events remains unclear.
Methods: We used LDpred2 to calculate genetic scores for lipid levels based on VEGFA variation, serving as instrumental variables to simulate the effect of VEGFA inhibitors. We then assessed the associations between genetic risk for lipid levels and CVD risk by conducting One-sample Mendelian randomization.
Results: Our results indicated that low-density lipoprotein cholesterol [LDL-C; odds ratio (OR) = 1.09, 95% CI: 1.06–1.11], remnant cholesterol (RC; OR = 1.24, 95% CI: 1.13–1.36), and triglycerides (TG; OR = 1.14, 95% CI: 1.07–1.22) were positively associated with the incidence of CVD. In contrast, high-density lipoprotein cholesterol (HDL-C) was inversely associated with the incidence of CVD (OR = 0.80, 95% CI: 0.76–0.86). When considering the genetic score for LDL-C constructed based on VEGFA, the group with a high genetic score demonstrated an elevated CVD risk (OR = 1.11, 95% CI: 1.04–1.19) compared to those with a low genetic score. Notably, One-sample Mendelian randomization results provided evidence of a causal relationship between LDL-C and CVD (p = 8.4×10−3) when using genetic variation in VEGFA as an instrumental variable.
Conclusions: Genetic variation mimicking the effect of VEGFA inhibition, which lowers LDL-C levels, was causally associated with a reduced risk of cardiovascular events. These findings offer insight into the potential therapeutic relevance of modulating VEGFA-mediated lipid changes in the prevention and management of CVD.
1. Introduction
Cardiovascular disease (CVD) persists as the leading cause of mortality worldwide, exerting a substantial toll on public health and healthcare expenditures (1). Among the myriad risk factors associated with CVD, dyslipidemia stands out as one of the most prevalent. Prolonged exposure to elevated levels of low-density lipoprotein cholesterol (LDL-C) has been shown to escalate the relative risk of CVD mortality by 50%–80% (2). Individuals characterized by primary low high-density lipoprotein cholesterol (HDL-C) are at an elevated risk of developing CVD compared to those exhibiting optimal lipid profiles (3). Notably, low-density lipoprotein-triglyceride (LDL-TG) presents itself as a potential marker for perturbed residual lipoprotein metabolism, significantly correlated with an increased risk of CVD (4). Furthermore, extant research underscores a causal relationship between lipid metabolism-related parameters and CVD (5, 6).
However, the association between various lipid constituents and the risk of CVD continues to exhibit variability due to variances in study populations. For instance, large-scale prospective cohort studies have illuminated that heightened levels of traditional lipid metrics, including total cholesterol (TC), LDL-C, and triglycerides (TG), in conjunction with diminished HDL-C levels, are connected to an augmented risk of CVD (7, 8). Nevertheless, the relationships between TC, HDL-C, LDL-C and the risk of mortality from CVD do not exhibit consistent trends and may manifest a “U” or “J” pattern (9, 10). A Swedish cohort study has even unveiled an association between elevated TC and LDL-C levels and a reduced risk of atrial fibrillation (11). Furthermore, multiple studies have challenged the notion that high LDL-C invariably leads to CVD (12–14). Discrepancies in these findings may be attributed to population-specific variations in lipid profiles across different regions. Moreover, investigations in developed nations have encountered challenges in discerning the adverse effects of small increases in lipid levels on CVD incidence. Consequently, further research is imperative to elucidate the factors contributing to lipid dysregulation and the onset of CVD. Additionally, exploring the potential therapeutic applications of these factors assumes paramount importance.
The vascular endothelial growth factors (VEGFs), a family of secreted signaling polypeptides, play critical roles in stimulating angiogenesis, promoting lymphopoiesis, regulating inflammation, and modulating lipid metabolism. VEGFA, the most potent angiogenic-stimulant member of the VEGF superfamily, which can be produced by a variety of cell types, including endothelial cells, platelets, macrophages, and tumor cells, has gained significant attention for its crucial role in dynamic homeostasis and pathological processes (15, 16). VEGFA stimulates endothelial cell mitosis and migration, enhances microvascular permeability, and promotes angiogenesis by interacting with VEGF receptors (VEGFRs) that belong to the tyrosine kinase receptor family (17–19). While it is true that other VEGF family members, such as VEGFB, VEGFC, and VEGFD, also contribute to the regulation of angiogenesis, lymphangiogenesis, and lipid metabolism (20–24), it's important to emphasize that VEGFA remains a crucial focus of research in the context of cardiovascular diseases and lipid regulation. It is reported that the function of VEGFB in improving metabolic dysfunction and inducing the browning of white adipose tissue is also dependent on VEGFA (25). VEGFA can enhance plasma lipids by inhibiting lipoprotein lipase activity (26), and the bioavailability of VEGFA is related to intestinal chylomicron absorption (27). Furthermore, VEGFA has promising potential as a therapeutic target for CVD. For instance, a novel mRNA-based drug AZD8601, functioning as a “secreted protein” to deliver VEGFA, has been developed to promote vascular regeneration and treat heart failure (28). In addition, sequential VEGFA/S1p administration with engineered bone marrow (BM) cells improves vascularization and reduces unfavorable cardiac remodeling following myocardial infarction in mice (29). However, the effect of regulating lipid levels by inhibiting VEGFA on the reduction of cardiovascular event risk remains unclear.
A shared genetic regulation mechanism may exist between VEGFA and cholesterol homeostasis molecules since a common variant highly associated with plasma VEGFA levels also contributes to the variation of both LDL-C and HDL-C (30). VEGFA expression can be promoted by oxidized low-density lipoprotein (ox-LDL), thereby inducing endothelial dysfunction in human aortic endothelial cells (31). VEGFA can influence the change of lipoprotein profiles and increase the proportion of triglyceride in large very low-density lipoprotein (VLDL) particles (26). Although a Mendelian randomization study did not provide substantial evidence to support the positive effect of VEGF on ischemic heart disease (IHD), it cannot eliminate the possibility that some specific types of VEGF might still have a role in the pathology (32). Angiogenesis, mediated by VEGFA, may be involved in plaque instability and thromboembolic events (33). Moreover, VEGFA levels are elevated in the serum and plasma of coronary artery disease (CAD) patients (34). Therefore, an accurate assessment of the role of VEGFA in CVD is crucial for the precise prevention and management of these diseases. Targeting VEGFA in lipid metabolism could be an effective strategy for reducing the risk of cardiovascular events. Our study seeks to leverage individual genetic data to investigate the influence of genetic variation within the VEGFA gene on changes in lipid levels and its subsequent impact on susceptibility to CVD. This will provide valuable epidemiological evidence to support the implementation of precision medicine in the context of CVD.
2. Materials and methods
2.1. Study population
The study cohort comprised 502,469 participants, including 71,318 individuals with cardiovascular events, who were sourced from the UK Biobank (https://www.ukbiobank.ac.uk). The UK Biobank is a comprehensive biomedical database and research resource that provides in-depth phenotypic and genomic data. Participants aged between 40 and 70 years were recruited between 2006 and 2010 from 22 assessment centers in the UK. Data on the participants’ health and genetic information were obtained through questionnaires, interviews, physical measurements, and biospecimen analysis, with all participants providing written informed consent.
2.2. Genetic data
The genetic data of the study cohort underwent single nucleotide polymorphism (SNP) genotyping, imputation, and quality control by the UK Biobank team. The first 50,000 participants were genotyped using the Affymetrix UK BiLEVE Axiom chip (35), while the remaining participants were genotyped using the Affymetrix UKB Axiom array (36). Genetic imputation utilized a combined panel of UK10K and 1,000 Genomes phase 3 reference panels. Further information on these processes is available at: http://www.ukbiobank.ac.uk/scientists-3/genetic-data/.
2.3. Assessment of CVD
Individuals who have reported experiencing a heart attack, angina, ischemic stroke, or transient ischemic attack (UKB codes 1075, 1074, 1082, 1583 in field 20002; codes 1, 2, 3 in field 6150), undergone cardiovascular procedures (UKB codes 1070, 1071, 1105, 1109, 1095, and 1514 in field 20004), Hospital Episode Statistics database and records of cardiovascular procedures in hospitals (OPCS-4 codes K40-K46, K47.1, K49-K50, K75) and participants who received a hospital diagnosis of CVD (ICD-10 codes G45, I20-I25, I63-I64 or the corresponding ICD-9 codes 410-414, 434, 436 and 42979).
2.4. Genetic instruments
LDpred2 method (37), which used summary statistics and a linkage disequilibrium matrix, was utilized to calculate genetic scores (38). This method integrates prior information and genotype data, enhancing the precision of genetic risk prediction. In the analysis of large-scale genetic data, we utilized the R packages “bigstatsr” (https://privefl.github.io/bigstatsr/) and “bigsnpr” (https://privefl.github.io/bigsnpr/).
Firstly, we sourced 7,114 SNPs from the VEGFA gene in the NCBI database (https://www.ncbi.nlm.nih.gov/snp/?term=VEGFA) and cross-referenced them with the SNPs derived from the GWAS meta-analysis data concerning HDL-C, LDL-C, remnant cholesterol (RC), total cholesterol (TC), and triglycerides (TG) from the Global Lipids Genetics Consortium (GLGC) (http://csg.sph.umich.edu/willer/public/lipids2013/) (39). We selected the variants that displayed association with the five cholesterol levels (Hardy-Weinberg Equilibrium (HWE) p-value >1 × 10−6 and minor allele frequency (MAF) >0.01) for further analysis (Supplementary Figure 1). The preliminary analysis of genetic variants in VEGFA associated with the levels of these five lipids is presented in Supplementary Tables 1–S5. Subsequently, we utilized LDpred2 to calculate the genetic risk score (GRS) for each participant within the UK Biobank population based on the selected SNPs from each lipid GWAS dataset. The participants were categorized into three groups based on the tertile of their GRS for each of the five lipids (Supplementary Figure 2). Finally, GRS calculated based on VEGFA variation was used to evaluate the association between lipid levels and CVD risk.
2.5. One-sample Mendelian randomization
One-sample Mendelian randomization (MR) was employed to elucidate the potential causal associations between lipid levels based on VEGFA GRS and the risk of CVD. MR is a robust statistical methodology that leverages genetic variation as an instrumental variable to estimate the impact of specific exposure or risk factors on clinically relevant outcomes (40). The framework of MR is illustrated in Supplementary Figure 3. We adopted the Two-stage least squares (TSLS) to perform Mendelian randomization inference in the UK Biobank population. In the first stage, we conducted a regression analysis, wherein the exposure of interest (lipid level) was regressed against the instrumental variable (VEGFA variation). This initial stage provided us with the estimated effect of these genetic variants on the exposure, effectively serving as an instrumental variable estimation. In the subsequent second stage, we performed another regression analysis, focusing on the outcome of interest (CVD). We regressed the outcome on the predicted value of the exposure (lipid level), which was derived from the first-stage analysis. The regression coefficient obtained from this stage represented the causal estimate, shedding light on the potential impact of lipid levels on CVD risk, guided by the genetic instrumental variable. A range of covariates for adjustment, including age, sex, ethnicity, body mass index (BMI), cholesterol-lowering medicine, genotyping batch, and PC1-PC10, were made to control for potential confounding factors and enhance the robustness of our causal inference.
2.6. Statistical analysis
The statistical analyses were conducted using SAS version 9.4 and R version 4.1.1. Baseline characteristics were reported as means and standard deviations for continuous variables and percentages for categorical variables. Logistic regression was employed to investigate the association between phenotypic lipid profiles and the incidence of CVD and to explore the relationship between lipid levels determined by VEGFA genetic variations and CVD risk. The covariates adjusted for in our analyses included age, sex, ethnicity, assessment center, Townsend index, alcohol frequency, smoking status, BMI, and cholesterol-lowering medication. Odds ratios (ORs) with 95% confidence intervals (CIs) were reported to present the results. We applied the Benjaminiand-Hochberg (BH) p-value correction to account for multiple testing (41). Statistical significance was considered at a 2-sided α threshold of p < 0.05.
3. Results
3.1. Participants characteristics
Baseline demographics and characteristics from 502,469 participants [229,117 women (54.41%); mean (SD) age, 56.63 (8.09) years] were analyzed and summarized in Table 1. Among them, 71,318 individuals (14.19%) had pre-existing CVD. The mean (SD) of BMI was 27.43 (4.80) kg/m2. The participants’ mean (SD) lipid levels were as follows: LDL-C, 3.56 (0.87) mmol/L; HDL-C, 1.45 (0.38) mmol/L; RC, 1.55 (0.42) mmol/L; TC, 4.56 (0.94) mmol/L; and TG, 1.30 (0.57) mmol/L.
3.2. Associations between phenotypic lipid profiles and CVD
Our observational study in UK Biobank (Table 2) showed that LDL-C, RC, and TG levels were positively associated with the incidence of CVD (OR = 1.09, 95% CI: 1.06–1.11; OR = 1.24, 95% CI: 1.13–1.36; OR = 1.14, 95% CI: 1.07–1.22, respectively Conversely, HDL-C emerged as a protective factor against CVD (OR = 0.80, 95% CI: 0.76–0.86). However, no significant association was found between TC levels and the incidence of CVD in UK Biobank participants (OR = 1.04, 95% CI: 1.00–1.09). The significance of the findings remained unaltered after the application of multiple corrections for the p-values. Additionally, after adjusting for the effects of known pathogenic FH variants within the LDLR, APOB, and PCSK9 genes (42), the observational findings remained consistent (Supplementary Table 6).
3.3. VEGFA GRS and cardiovascular events
GRS was calculated based on VEGFA variants to reflect LDL-C and TC levels. Subsequently, we assessed the relationship between GRS and cardiovascular risks. Compared with the high VEGFA score, the lower VEGFA score showed an 11% (OR = 1.11, 95% CI: 1.04–1.19) reduced risk of CVD, p = 3.00×10−4 (Figure 1A); likewise, the lower VEGFA score for TC was associated with a 12% (OR = 1.12, 95% CI: 1.05–1.20) decreased the risk of CVD, p = 6.00×10−4 (Figure 1B). A dose-response stratified analysis showed a stepwise decrease in LDL-C and TC levels (calculated based on VEGFA variants) with a corresponding decrease in the risk of CVD (Figures 1A,B).
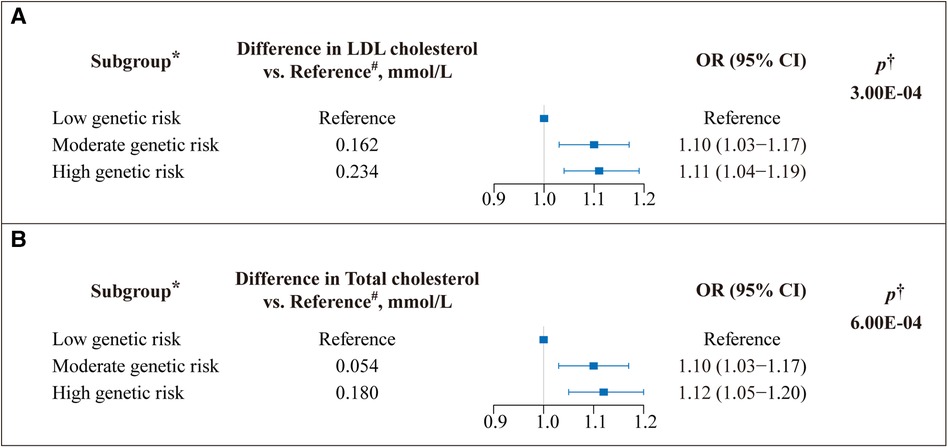
Figure 1. Effect of VEGFA genetic score on the risk of cardiovascular disease (CVD). A weighted genetic score was calculated for VEGFA for each study participant. (A) The genetic risk contributions to the CVD of SNPs on VEGFA associated with low-density lipoprotein (LDL) cholesterol and (B) total cholesterol (TC) (Unit: millimoles per liter) levels were calculated using the Ldpred2 method, respectively. OR: odds ratio; Boxes represent point estimates of effect. Lines represent 95% confidence intervals (CIs). *The genetic score was classified into three subgroups based on tertiles to determine genetic risk. A low genetic score indicates low genetic risk, and vice versa. #Represents the difference between each group's mean lipid (LDL-C/TC) level and the mean lipid level of the reference group. †Adjusted for age, sex, ethnicity, assessment center, Townsend index, alcohol frequency, smoke status, BMI, and cholesterol-lowering medicine.
3.4. One-Sample Mendelian randomization
We used GRS of VEGFA genetic variants as instrumental variables in one-sample MR analysis. In the first stage, the F statistics for instrumental variables (VEGFA variation) was 20.76 (p = 5.2×10−6), suggesting weak instrumental variable bias was avoided. In the second stage, the predicted LDL-C level produced from the first stage was significantly associated with the risk of CVD (OR = 1.06, 95% CI: 1.02–1.11, p = 8.4×10−3). These results together suggested that LDL-C level, determined by VEGFA genetic variants, was casually associated with CVD. However, since the GRS of VEGFA genetic variants was not associated (p > 0.05) with TC level, we could not infer the causal relationship between TC and CVD risk.
3.5. Sensitivity analysis
Sensitivity analyses confirmed the robustness of the observational findings. We reanalyzed the association between the five lipid levels and CVD by excluding participants who experienced a CVD event within two years of the initial follow-up. We observed no significant alterations in the results. Specifically, LDL-C, RC, and TG remained positively associated with the incidence of CVD, whereas HDL-C remained a protective factor against CVD (Supplementary Table 7).
4. Discussion
In the UK Biobank population, our investigation revealed a correlation between VEGFA variation and alternations in blood lipoprotein profiles. The increased risk of cardiovascular events could be attributed to the elevated LDL-C level reflected by the VEGFA genetic risk. These findings mimic the effects achieved by VEGFA inhibition in reducing LDL-C levels, underscoring the potential of VEGFA suppression as a viable genetic target for therapeutic interventions.
CVD is a multifaceted and heritable condition that results from a combination of genetic and environmental factors. Dyslipidemia, a common lipid abnormality, is an established risk factor for CVD. Several prospective cohort studies and meta-analyses have shown that maintaining optimal levels of LDL-C could reduce the lifetime risk of atherosclerosis, while high levels of HDL-C may protect against CVD (43–45). Our observational study unveiled associations between elevated LDL-C, RC, and TG levels with CVD. However, the relationship between TC and CVD remains uncertain. In alignment with our findings, other studies have demonstrated either a positive association (44, 46, 47) or a weak or nonexistent connection (48–50). Hence, it is crucial to perform additional research to enhance our comprehension of the factors that influence the prevention and susceptibility to CVD.
We developed the GRS based on genetic variants in VEGFA by utilizing GWAS summary data from the GLGC. Our GRS is designed to mimic the lifetime exposure of lipids. SNP association tests in GWAS are typically conducted one SNP at a time, leading to strong linkage disequilibrium (LD) across the genome and biased estimates of independent effect (51). We used the LDpred2 method to construct the VEGFA GRS to overcome this issue. LDpred2 is an updated and powerful tool that derives multi-gene scores based solely on summary statistics and LD matrices. Unlike other gene scoring methods that rely on marker pruning with LD and applying p-value thresholds to association statistics, LDpred2 retains more information to improve prediction accuracy (38) and solves the problem of model specification errors while improving computational efficiency. It has been used in assessing the risk of numerous diseases and has significantly enhanced the performance and reliability of risk prediction in recent years (52–55).
Stratifying populations by different VEGFA genetic risks, we have found that individuals with higher VEGFA genetic risk have an increased risk of CVD, which aligns with previous studies. Li et al. identified VEGF gene polymorphisms rs699947 and haplotypes as potential genetic markers for coronary heart disease pathogenesis (56). Another meta-analysis demonstrated that VEGFA rs699947 C>A, rs3025039 C>T, and rs2010963 G>C polymorphisms are risk factors for coronary heart disease (57). Animal studies have also shown that overexpression of VEGFA increases the likelihood of atherosclerosis in ApoE-deficient mice (26). Clinical research also supports controlling angiogenesis and VEGFA to improve the quality of life and life expectancy among cardiac patients (58). In addition, a marginal elevation in LDL-C levels was observed across low-, moderate- to high-genetic-risk subgroups; however, the clinical implications of this result remain uncertain. Firstly, owing to the multifactorial nature of CVD and the complexities of lipid profiles, neither LDL-C levels nor VEGFA variations act in isolation. This, in part, elucidates the rationale behind the marginal LDL-C increases observed in our study. Secondly, the clinical paradigm of LDL-C reduction has evolved as a cornerstone in CVD prevention and management. Recent guidelines for blood lipid management emphasize the regular monitoring of treatment efficacy and the surveillance of potential adverse reactions. These guidelines advocate flexible treatment plans to ensure sustained adherence to lipid standards. Furthermore, studies have established a correlation between cumulative LDL-C exposure, lipid-lowering treatment, and the risk of atherosclerotic cardiovascular disease (ASCVD) (59, 60). Therefore, from a collective perspective, meticulous attention should be directed toward achieving early, sustained, and stable attainment of LDL-C targets. Lastly, precision medicine seeks to tailor medical decisions and interventions to individual characteristics. Our findings, although showing marginal changes, may contribute to more precise risk prediction. In the context of precision medicine, identifying subgroups with even minor increases in LDL-C levels can help guide targeted interventions and preventive strategies.
Given VEGFA's pivotal role in vascular angiogenesis across diverse physiological and pathological contexts, as well as its significant contribution to vascular homeostasis (61, 62), a previous study has revealed a robust association between common genetic variations linked to VEGFA and both HDL-C and LDL-C (30). Furthermore, Dabravolski et al. have proposed the potential of the VEGF family as a therapeutic target for atherosclerosis (63). Thus, by utilizing a lipid genetic score constructed from genetic variations associated with VEGFA, our study extended its inquiry, shedding light on a causal link between lipid metabolism and CVD risk. Previous research has firmly established the additional CVD risk associated with pathogenic FH variations (64–66). Currently, statins, ezetimibe, bile acid sequestrants, and PCSK9 inhibitors are used as standard agents for lipid-lowering interventions. However, whether administered individually or in combination, these treatments often engender intolerable side effects, necessitating the exploration of novel drug targets and clinical trials (67). Mechanistic investigations into the interplay between VEGFA and lipid metabolism suggest that VEGFA may downregulate lipoprotein lipase activity, leading to the accumulation of TC within large lipoprotein particles, including chylomicrons and VLDL, thereby fostering atherogenic changes (26). Clinical studies further report that lipid levels in the bloodstream can modulate VEGFA expression and influence biological activity (68). In addition, molecular mechanistic studies have shown that elevated LDL level impairs angiogenesis via disrupting an endothelial cell-autonomous signaling network (TNFα/NF-κB/HIF/VEGF) that governs angiogenesis in hypoxic responses (69). This may represent a mechanistic link through which lipid levels impact the onset and progression of CVD. Our genetic findings underscore that VEGFA variations accounting for elevated LDL-C levels correlate with an increased risk of cardiovascular events, thus establishing a causal connection between LDL-C and CVD. This genetic evidence lends support to the proposition of VEGFA as a promising therapeutic target.
It is important to note that our study has several limitations. Firstly, despite extensive evidence of VEGFA's role in lipid regulation and CVD treatment (60, 63, 70), there is currently a lack of effective agents capable of targeting VEGFA to modulate lipid levels in either experimental or clinical settings. Predicting or investigating the effects of genetic variants that mimic therapeutic actions and assessing their population-specific specificity can be inherently challenging. Secondly, in alignment with all MR studies, we must acknowledge our inability to validate our instrumental variable hypothesis empirically. Our estimates are susceptible to biases stemming from pleiotropy or confounding factors. Therefore, where conditions allow, it becomes imperative to bolster causal inferences through more robust randomized controlled trials. Thirdly, given the polygenic underpinnings of lipid expression profiles and CVD risk, our investigation concentrated solely on evaluating the impact of a single genetic variation within the VEGFA gene on CVD susceptibility. It is worth noting that while numerous studies in the field of lipid metabolism and its association with CVD have historically focused on variations in single genes, such as PCSK9, HMGCR, and ACLY (5, 71), VEGFA may only represent a single node in this complex network. Thus, further research endeavors aimed at elucidating interactions between VEGFA and other genes and exploring VEGFA's integration into the broader polygenic context are warranted to bridge the gap between monogenic insights and polygenic complexities. Lastly, it is essential to acknowledge that, due to database limitations, the population included in the MR study only consisted of individuals from the UK Biobank. Subsequent research should expand data collection to encompass diverse populations, ensuring the generalizability of research findings.
In conclusion, within the UK Biobank population, we have observed a statistical association between LDL-C levels influenced by VEGFA variations and susceptibility to cardiovascular events. Our findings suggest the potential importance of VEGFA inhibition in addressing CVD caused by lipid dysregulation. While our discovery highlights VEGFA as a promising therapeutic target in cardiovascular research, it's essential to recognize that additional experimental and clinical validation is warranted to firmly establish its application and significance in CVD prevention and precision treatment.
Data availability statement
Publicly available datasets were analyzed in this study. This data can be found here: UK Biobank (https://www.ukbiobank.ac.uk); Global Lipids Genetics Consortium (http://csg.sph.umich.edu/willer/public/Lipids2013/).
Ethics statement
Ethical approval was not required for the study involving humans in accordance with the local legislation and institutional requirements. Written informed consent to participate in this study was not required from the participants or the participants’ legal guardians/next of kin in accordance with the national legislation and the institutional requirements.
Author contributions
Conceptualization: YZ and TZ. Data acquisition, analysis, and interpretation: HC, JY, ZC, and XL. Statistical analysis: XL and ZC. Writing – original draft: HC and YZ. Writing – review and editing: YZ, TZ, JY, WQ, and HC. All authors contributed to the article and approved the submitted version.
Funding
This work was supported by the National Natural Science Foundation of China (82000462 and 82170883) (YZ).
Acknowledgments
The authors thank all the participants and investigators of the UK Biobank study for their contributions. The current investigation was conducted in compliance with the UK Biobank Project Number 78559.
Conflict of interest
The authors declare that the research was conducted in the absence of any commercial or financial relationships that could be construed as a potential conflict of interest.
Publisher's note
All claims expressed in this article are solely those of the authors and do not necessarily represent those of their affiliated organizations, or those of the publisher, the editors and the reviewers. Any product that may be evaluated in this article, or claim that may be made by its manufacturer, is not guaranteed or endorsed by the publisher.
Supplementary material
The Supplementary Material for this article can be found online at: https://www.frontiersin.org/articles/10.3389/fcvm.2023.1240288/full#supplementary-material
References
1. Vaduganathan M, Mensah GA, Turco JV, Fuster V, Roth GA. The global burden of cardiovascular diseases and risk: a compass for future health. J Am Coll Cardiol. (2022) 80(25):2361–71. doi: 10.1016/j.jacc.2022.11.005
2. Abdullah SM, Defina LF, Leonard D, Barlow CE, Radford NB, Willis BL, et al. Long-term association of low-density lipoprotein cholesterol with cardiovascular mortality in individuals at low 10-year risk of atherosclerotic cardiovascular disease. Circulation. (2018) 138(21):2315–25. doi: 10.1161/CIRCULATIONAHA.118.034273
3. Ahmed HM, Miller M, Nasir K, McEvoy JW, Herrington D, Blumenthal RS, et al. Primary low level of high-density lipoprotein cholesterol and risks of coronary heart disease, cardiovascular disease, and death: results from the multi-ethnic study of atherosclerosis. Am J Epidemiol. (2016) 183(10):875–83. doi: 10.1093/aje/kwv305
4. Saeed A, Feofanova EV, Yu B, Sun W, Virani SS, Nambi V, et al. Remnant-like particle cholesterol, low-density lipoprotein triglycerides, and incident cardiovascular disease. J Am Coll Cardiol. (2018) 72(2):156–69. doi: 10.1016/j.jacc.2018.04.050
5. Ference BA, Ray KK, Catapano AL, Ference TB, Burgess S, Neff DR, et al. Mendelian Randomization study of ACLY and cardiovascular disease. N Engl J Med. (2019) 380(11):1033–42. doi: 10.1056/NEJMoa1806747
6. Holmes MV, Asselbergs FW, Palmer TM, Drenos F, Lanktree MB, Nelson CP, et al. Mendelian randomization of blood lipids for coronary heart disease. Eur Heart J. (2015) 36(9):539–50. doi: 10.1093/eurheartj/eht571
7. Sun L, Clarke R, Bennett D, Guo Y, Walters RG, Hill M, et al. Causal associations of blood lipids with risk of ischemic stroke and intracerebral hemorrhage in Chinese adults. Nat Med. (2019) 25(4):569–74. doi: 10.1038/s41591-019-0366-x
8. Gu X, Li Y, Chen S, Yang X, Liu F, Li Y, et al. Association of lipids with ischemic and hemorrhagic stroke: a prospective cohort study among 267 500 Chinese. Stroke. (2019) 50(12):3376–84. doi: 10.1161/STROKEAHA.119.026402
9. Zhong GC, Huang SQ, Peng Y, Wan L, Wu YQ, Hu TY, et al. HDL-C is associated with mortality from all causes, cardiovascular disease and cancer in a J-shaped dose-response fashion: a pooled analysis of 37 prospective cohort studies. Eur J Prev Cardiol. (2020) 27(11):1187–203. doi: 10.1177/2047487320914756
10. Curb JD, Abbott RD, Rodriguez BL, Masaki K, Popper J, Chen R, et al. Prospective association between low and high total and low-density lipoprotein cholesterol and coronary heart disease in elderly men. J Am Geriatr Soc. (2004) 52(12):1975–80. doi: 10.1111/j.1532-5415.2004.52551.x
11. Ding M, Wennberg A, Gigante B, Walldius G, Hammar N, Modig K. Lipid levels in midlife and risk of atrial fibrillation over 3 decades-experience from the Swedish AMORIS cohort: a cohort study. PLoS Med. (2022) 19(8):e1004044. doi: 10.1371/journal.pmed.1004044
12. Sachdeva A, Cannon CP, Deedwania PC, Labresh KA, Smith SC Jr., Dai D, et al. Lipid levels in patients hospitalized with coronary artery disease: an analysis of 136,905 hospitalizations in get with the guidelines. Am Heart J. (2009) 157(1):111–7.e2. doi: 10.1016/j.ahj.2008.08.010
13. Al-Mallah MH, Hatahet H, Cavalcante JL, Khanal S. Low admission LDL-cholesterol is associated with increased 3-year all-cause mortality in patients with non ST segment elevation myocardial infarction. Cardiol J. (2009) 16(3):227–33. doi: 10.1590/1806-9282.67.02.20200548
14. Hamazaki T, Okuyama H, Ogushi Y, Hama R. Towards a paradigm shift in cholesterol treatment. A re-examination of the cholesterol issue in Japan. Ann Nutr Metab. (2015) 66(Suppl 4):1–116. doi: 10.1159/000381654
15. Apte RS, Chen DS, Ferrara N. VEGF in signaling and disease: beyond discovery and development. Cell. (2019) 176(6):1248–64. doi: 10.1016/j.cell.2019.01.021
16. Ferrara N, Adamis AP. Ten years of anti-vascular endothelial growth factor therapy. Nat Rev Drug Discovery. (2016) 15(6):385–403. doi: 10.1038/nrd.2015.17
17. Abhinand CS, Raju R, Soumya SJ, Arya PS, Sudhakaran PR. VEGF-A/VEGFR2 signaling network in endothelial cells relevant to angiogenesis. J Cell Commun Signal. (2016) 10(4):347–54. doi: 10.1007/s12079-016-0352-8
18. Herbert SP, Stainier DY. Molecular control of endothelial cell behaviour during blood vessel morphogenesis. Nat Rev Mol Cell Biol. (2011) 12(9):551–64. doi: 10.1038/nrm3176
19. Xin H, Zhong C, Nudleman E, Ferrara N. Evidence for pro-angiogenic functions of VEGF-ax. Cell. (2016) 167(1):275–84.e6. doi: 10.1016/j.cell.2016.08.054
20. Shaik F, Cuthbert GA, Homer-Vanniasinkam S, Muench SP, Ponnambalam S, Harrison MA. Structural basis for vascular endothelial growth factor receptor activation and implications for disease therapy. Biomolecules. (2020) 10(12):1673. doi: 10.3390/biom10121673
21. Hagberg CE, Falkevall A, Wang X, Larsson E, Huusko J, Nilsson I, et al. Vascular endothelial growth factor B controls endothelial fatty acid uptake. Nature. (2010) 464(7290):917–21. doi: 10.1038/nature08945
22. Ning FC, Jensen N, Mi J, Lindström W, Balan M, Muhl L, et al. VEGF-B ablation in pancreatic β-cells upregulates insulin expression without affecting glucose homeostasis or islet lipid uptake. Sci Rep. (2020) 10(1):923. doi: 10.1038/s41598-020-57599-2
23. Nurmi H, Saharinen P, Zarkada G, Zheng W, Robciuc MR, Alitalo K. VEGF-C is required for intestinal lymphatic vessel maintenance and lipid absorption. EMBO Mol Med. (2015) 7(11):1418–25. doi: 10.15252/emmm.201505731
24. Shew T, Wolins NE, Cifarelli V. VEGFR-3 signaling regulates triglyceride retention and absorption in the intestine. Front Physiol. (2018) 9:1783. doi: 10.3389/fphys.2018.01783
25. Seki T, Hosaka K, Fischer C, Lim S, Andersson P, Abe M, et al. Ablation of endothelial VEGFR1 improves metabolic dysfunction by inducing adipose tissue browning. J Exp Med. (2018) 215(2):611–26. doi: 10.1084/jem.20171012
26. Heinonen SE, Kivelä AM, Huusko J, Dijkstra MH, Gurzeler E, Mäkinen PI, et al. The effects of VEGF-A on atherosclerosis, lipoprotein profile, and lipoprotein lipase in hyperlipidaemic mouse models. Cardiovasc Res. (2013) 99(4):716–23. doi: 10.1093/cvr/cvt148
27. Zhang F, Zarkada G, Han J, Li J, Dubrac A, Ola R, et al. Lacteal junction zippering protects against diet-induced obesity. Science. (2018) 361(6402):599–603. doi: 10.1126/science.aap9331
28. Collén A, Bergenhem N, Carlsson L, Chien KR, Hoge S, Gan LM, et al. VEGFA mRNA for regenerative treatment of heart failure. Nat Rev Drug Discovery. (2022) 21(1):79–80. doi: 10.1038/s41573-021-00355-6
29. Żak MM, Gkontra P, Clemente C, Squadrito ML, Ferrarini A, Mota RA, et al. Sequential bone-marrow cell delivery of VEGFA/S1P improves vascularization and limits adverse cardiac remodeling after myocardial infarction in mice. Hum Gene Ther. (2019) 30(7):893–905. doi: 10.1089/hum.2018.194
30. Stathopoulou MG, Bonnefond A, Ndiaye NC, Azimi-Nezhad M, El Shamieh S, Saleh A, et al. A common variant highly associated with plasma VEGFA levels also contributes to the variation of both LDL-C and HDL-C. J Lipid Res. (2013) 54(2):535–41. doi: 10.1194/jlr.P030551
31. Tang F, Zhang S, Wang H, Xu S, Yang S, Zhu X, et al. lncRNA H19 promotes ox-LDL-induced dysfunction of human aortic endothelial cells through the miR-152/VEGFA axis. J Healthc Eng. (2022) 2022:3795060. doi: 10.1155/2022/3795060
32. Yeung SL A, Lam H, Schooling CM. Vascular endothelial growth factor and ischemic heart disease risk: a Mendelian randomization study. J Am Heart Assoc. (2017) 6(8):e005619. doi: 10.1161/JAHA.117.005619
33. Camaré C, Pucelle M, Nègre-Salvayre A, Salvayre R. Angiogenesis in the atherosclerotic plaque. Redox Biol. (2017) 12:18–34. doi: 10.1016/j.redox.2017.01.007
34. Meng R, Pei Z, Chen B, Ma R, Zhang C, Chen B, et al. Age-related change of serum angiogenic factor levels in patients with coronary artery disease. Acta Cardiol. (2009) 64(6):735–40. doi: 10.2143/AC.64.6.2044736
35. Wain LV, Shrine N, Miller S, Jackson VE, Ntalla I, Soler Artigas M, et al. Novel insights into the genetics of smoking behaviour, lung function, and chronic obstructive pulmonary disease (UK BiLEVE): a genetic association study in UK biobank. Lancet Respir Med. (2015) 3(10):769–81. doi: 10.1016/S2213-2600(15)00283-0
36. Welsh S, Peakman T, Sheard S, Almond R. Comparison of DNA quantification methodology used in the DNA extraction protocol for the UK biobank cohort. BMC Genomics. (2017) 18(1):26. doi: 10.1186/s12864-016-3391-x
37. Privé F, Arbel J, Vilhjálmsson BJ. LDpred2: better, faster, stronger. Bioinformatics (Oxford, England). (2020) 36(22–23):5424–31. doi: 10.1093/bioinformatics/btaa1029
38. Vilhjálmsson BJ, Yang J, Finucane HK, Gusev A, Lindström S, Ripke S, et al. Modeling linkage disequilibrium increases accuracy of polygenic risk scores. Am J Hum Genet. (2015) 97(4):576–92. doi: 10.1016/j.ajhg.2015.09.001
39. Willer CJ, Schmidt EM, Sengupta S, Peloso GM, Gustafsson S, Kanoni S, et al. Discovery and refinement of loci associated with lipid levels. Nat Genet. (2013) 45(11):1274–83. doi: 10.1038/ng.2797
40. Sekula P, Del Greco MF, Pattaro C, Köttgen A. Mendelian randomization as an approach to assess causality using observational data. J Am Soc Nephrol. (2016) 27(11):3253–65. doi: 10.1681/ASN.2016010098
41. Benjamini Y, Hochberg Y. Controlling the false discovery rate: a practical and powerful approach to multiple testing. J R Stat Soc Ser B. (1995) 57(1):289–300. doi: 10.1111/j.2517-6161.1995.tb02031.x
42. Lye SH, Chahil JK, Bagali P, Alex L, Vadivelu J, Ahmad WA, et al. Genetic polymorphisms in LDLR, APOB, PCSK9 and other lipid related genes associated with familial hypercholesterolemia in Malaysia. PLoS One. (2013) 8(4):e60729. doi: 10.1371/journal.pone.0060729
43. Imaizumi VM, Laurindo LF, Manzan B, Guiguer EL, Oshiiwa M, Otoboni A, et al. Garlic: a systematic review of the effects on cardiovascular diseases. Crit Rev Food Sci Nutr. (2023) 63(24):6797–819. doi: 10.1080/10408398.2022.2043821
44. Jung E, Kong SY, Ro YS, Ryu HH, Shin SD. Serum cholesterol levels and risk of cardiovascular death: a systematic review and a dose-response meta-analysis of prospective cohort studies. Int J Environ Res Public Health. (2022) 19(14):8272. doi: 10.3390/ijerph19148272
45. Zhang Y, Pletcher MJ, Vittinghoff E, Clemons AM, Jacobs DR Jr., Allen NB, et al. Association between cumulative low-density lipoprotein cholesterol exposure during young adulthood and middle age and risk of cardiovascular events. JAMA Cardiol. (2021) 6(12):1406–13. doi: 10.1001/jamacardio.2021.3508
46. Peters SA, Singhateh Y, Mackay D, Huxley RR, Woodward M. Total cholesterol as a risk factor for coronary heart disease and stroke in women compared with men: a systematic review and meta-analysis. Atherosclerosis. (2016) 248:123–31. doi: 10.1016/j.atherosclerosis.2016.03.016
47. Dong J, Yang S, Zhuang Q, Sun J, Wei P, Zhao X, et al. The associations of lipid profiles with cardiovascular diseases and death in a 10-year prospective cohort study. Front Cardiovasc Med. (2021) 8:745539. doi: 10.3389/fcvm.2021.745539
48. Ravnskov U. High cholesterol may protect against infections and atherosclerosis. QJM. (2003) 96(12):927–34. doi: 10.1093/qjmed/hcg150
49. Ulmer H, Kelleher C, Diem G, Concin H. Why eve is not adam: prospective follow-up in 149650 women and men of cholesterol and other risk factors related to cardiovascular and all-cause mortality. J Womens Health (Larchmt). (2004) 13(1):41–53. doi: 10.1089/154099904322836447
50. Ravnskov U, de Lorgeril M, Diamond DM, Hama R, Hamazaki T, Hammarskjöld B, et al. LDL-C does not cause cardiovascular disease: a comprehensive review of the current literature. Expert Rev Clin Pharmacol. (2018) 11(10):959–70. doi: 10.1080/17512433.2018.1519391
51. Choi SW, Mak TS, O'Reilly PF. Tutorial: a guide to performing polygenic risk score analyses. Nat Protoc. (2020) 15(9):2759–72. doi: 10.1038/s41596-020-0353-1
52. Briggs SEW, Law P, East JE, Wordsworth S, Dunlop M, Houlston R, et al. Integrating genome-wide polygenic risk scores and non-genetic risk to predict colorectal cancer diagnosis using UK biobank data: population based cohort study. Br Med J. (2022) 379:e071707. doi: 10.1136/bmj-2022-071707
53. Ni G, Zeng J, Revez JA, Wang Y, Zheng Z, Ge T, et al. A comparison of ten polygenic score methods for psychiatric disorders applied across multiple cohorts. Biol Psychiatry. (2021) 90(9):611–20. doi: 10.1016/j.biopsych.2021.04.018
54. Ge T, Irvin MR, Patki A, Srinivasasainagendra V, Lin YF, Tiwari HK, et al. Development and validation of a trans-ancestry polygenic risk score for type 2 diabetes in diverse populations. Genome Med. (2022) 14(1):70. doi: 10.1186/s13073-022-01074-2
55. Miyazawa K, Ito K, Ito M, Zou Z, Kubota M, Nomura S, et al. Cross-ancestry genome-wide analysis of atrial fibrillation unveils disease biology and enables cardioembolic risk prediction. Nat Genet. (2023) 55(2):187–97. doi: 10.1038/s41588-022-01284-9
56. Li L, Pan Y, Dai L, Liu B, Zhang D. Association of genetic polymorphisms on vascular endothelial growth factor and its receptor genes with susceptibility to coronary heart disease. Med Sci Monit. (2016) 22:31–40. doi: 10.12659/MSM.895163
57. Wang Y, Huang Q, Liu J, Wang Y, Zheng G, Lin L, et al. Vascular endothelial growth factor A polymorphisms are associated with increased risk of coronary heart disease: a meta-analysis. Oncotarget. (2017) 8(18):30539–51. doi: 10.18632/oncotarget.15546
58. Braile M, Marcella S, Cristinziano L, Galdiero MR, Modestino L, Ferrara AL, et al. VEGF-A in cardiomyocytes and heart diseases. Int J Mol Sci. (2020) 21(15):5294. doi: 10.3390/ijms21155294
59. Ference BA, Graham I, Tokgozoglu L, Catapano AL. Impact of lipids on cardiovascular health: JACC health promotion series. J Am Coll Cardiol. (2018) 72(10):1141–56. doi: 10.1016/j.jacc.2018.06.046
60. Wang L, Zheng W, Yang J, Ali A, Qin H. Mechanism of Astragalus membranaceus alleviating acquired hyperlipidemia induced by high-fat diet through regulating lipid metabolism. Nutrients. (2022) 14(5):954. doi: 10.3390/nu14050954
61. Ylä-Herttuala S, Rissanen TT, Vajanto I, Hartikainen J. Vascular endothelial growth factors: biology and current status of clinical applications in cardiovascular medicine. J Am Coll Cardiol. (2007) 49(10):1015–26. doi: 10.1016/j.jacc.2006.09.053
62. Carmeliet P, Jain RK. Molecular mechanisms and clinical applications of angiogenesis. Nature. (2011) 473(7347):298–307. doi: 10.1038/nature10144
63. Dabravolski SA, Khotina VA, Omelchenko AV, Kalmykov VA, Orekhov AN. The role of the VEGF family in atherosclerosis development and its potential as treatment targets. Int J Mol Sci. (2022) 23(2):931. doi: 10.3390/ijms23020931
64. Trinder M, Francis GA, Brunham LR. Association of monogenic vs polygenic hypercholesterolemia with risk of atherosclerotic cardiovascular disease. JAMA Cardiol. (2020) 5(4):390–9. doi: 10.1001/jamacardio.2019.5954
65. Guijarro C, Civeira F, Masana L. Genetic confirmation of monogenic familial hypercholesterolemia advises a more intensive lipid-lowering approach. JAMA Cardiol. (2020) 5(12):1452–3. doi: 10.1001/jamacardio.2020.4114
66. Kafyra M, Kalafati IP, Gavra I, Siest S, Dedoussis GV. Associations of VEGF-A-related variants with adolescent cardiometabolic and dietary parameters. Nutrients. (2023) 15(8):1884. doi: 10.3390/nu15081884
67. di Mauro G, Zinzi A, Scavone C, Mascolo A, Gaio M, Sportiello L, et al. PCSK9 inhibitors and neurocognitive adverse drug reactions: analysis of individual case safety reports from the eudravigilance database. Drug Saf. (2021) 44(3):337–49. doi: 10.1007/s40264-020-01021-3
68. Schüler R, Seebeck N, Osterhoff MA, Witte V, Flöel A, Busjahn A, et al. VEGF and GLUT1 are highly heritable, inversely correlated and affected by dietary fat intake: consequences for cognitive function in humans. Mol Metab. (2018) 11:129–36. doi: 10.1016/j.molmet.2018.02.004
69. Jin F, Zheng X, Yang Y, Yao G, Ye L, Doeppner TR, et al. Impairment of hypoxia-induced angiogenesis by LDL involves a HIF-centered signaling network linking inflammatory TNFα and angiogenic VEGF. Aging. (2019) 11(2):328–49. doi: 10.18632/aging.101726
70. Zhou Y, Zhu X, Cui H, Shi J, Yuan G, Shi S, et al. The role of the VEGF family in coronary heart disease. Front Cardiovasc Med. (2021) 8:738325. doi: 10.3389/fcvm.2021.738325
Keywords: VEGFA variation, cardiovascular disease, lipid metabolism, genetic risk score, Mendelian randomization
Citation: Chen H, Lv X, Yang J, Chen Z, Qiao W, Zhou T and Zhang Y (2023) Variation in VEGFA and risk of cardiovascular disease in the UK Biobank. Front. Cardiovasc. Med. 10:1240288. doi: 10.3389/fcvm.2023.1240288
Received: 14 June 2023; Accepted: 14 November 2023;
Published: 28 November 2023.
Edited by:
Wai San Cheang, University of Macau, China© 2023 Chen, Lv, Yang, Chen, Qiao, Zhou and Zhang. This is an open-access article distributed under the terms of the Creative Commons Attribution License (CC BY). The use, distribution or reproduction in other forums is permitted, provided the original author(s) and the copyright owner(s) are credited and that the original publication in this journal is cited, in accordance with accepted academic practice. No use, distribution or reproduction is permitted which does not comply with these terms.
*Correspondence: Tao Zhou zhout93@mail.sysu.edu.cn Yang Zhang zhangy2293@mail.sysu.edu.cn
†These authors have contributed equally to this work and share first authorship