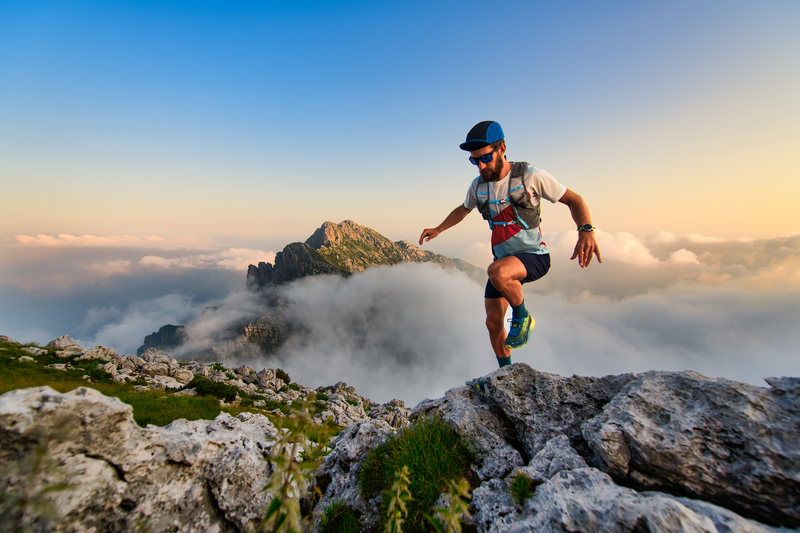
94% of researchers rate our articles as excellent or good
Learn more about the work of our research integrity team to safeguard the quality of each article we publish.
Find out more
ORIGINAL RESEARCH article
Front. Cardiovasc. Med. , 08 September 2023
Sec. Coronary Artery Disease
Volume 10 - 2023 | https://doi.org/10.3389/fcvm.2023.1178417
Background: The prognosis of ST-segment elevation myocardial infarction (STEMI) is closely linked to left ventricular ejection fraction (LVEF). In contrast to primary percutaneous coronary intervention (PPCI), thrombolysis-transfer PCI (TTPCI) is influenced by multiple factors that lead to heterogeneity in cardiac function and prognosis. The aim of this study is to develop a nomogram model for predicting early LVEF in STEMI patients with TTPCI, based on routine indicators at admission.
Method: We retrospectively reviewed data from patients diagnosed with STEMI at five network hospitals of our PCI center who performed TTPCI as door-to-balloon time (the interval between arrival at the hospital and intracoronary balloon inflation) over 120 min, from February 2018 to April 2022. Categorical variables were analyzed using Pearson χ2 tests or Fisher exact tests, while Student's t-test or Mann–Whitney U-test was used to compare continuous variables. Subsequently, independent risk factors associated with reduced LVEF one week after TTPCI were identified through comprehensive analysis by combining All-Subsets Regression with Logistic Regression. Based on these indicators, a nomogram model was developed, and validated using the area under the receiver operating characteristic (ROC) curve and the Bootstrap method.
Results: A total of 288 patients were analyzed, including 60 with LVEF < 50% and 228 with LVEF ≥ 50%. The nomogram model based on six independent risk factors including age, heart rate (HR), hypertension, smoking history, Alanine aminotransferase (ALT), and Killip class, demonstrated excellent discrimination with an AUC of 0.84 (95% CI: 0.78–0.89), predicted C-index of 0.84 and curve fit of 0.713.
Conclusions: The nomogram model incorporating age, HR, hypertension, smoking history, ALT and Killip class could accurately predict the early LVEF ≥ 50% probability of STEMI patients undergoing TTPCI, and enable clinicians' early evaluation of cardiac function in STEMI patients with TTPCI and early optimization of treatment.
STEMI represents the most serious manifestation of coronary atherosclerosis disease, with an in-hospital heart failure rate of 14.2% and a mortality rate ranging from 3% to 4% (1). Current reperfusion modalities for STEMI patients include thrombolysis, PPCI or coronary artery bypass grafting (CABG) (2). However, TTPCI is recommend by guidelines as an effective reperfusion strategy for patients with expected door-to-balloon time over 120 min at non-PCI center (3, 4). Nevertheless, multiple intermediate steps involving thrombolysis, transfer with ambulance, evaluation before PCI and the post-thrombolytic complications occurrence such as thrombolysis failure, reperfusion arrhythmias, and reinfarction post thrombolysis, as well as differences in emergency medical care contribute to the heterogeneity and uncertainty of prognosis in these populations (5). Several studies have shown a strong correlation of LVEF reduction after PCI with in-hospital mortality as well as major adverse cardiovascular events (MACEs) for STEMI patients (6–8). Additionally some biomarkers such as mid-regional precursor atrial natriuretic peptide (MR-proANP), brain natriuretic peptide (BNP) have reported the predictive value for LVEF of STEMI patients post-PCI (9–11); but few predictive model has been established for STEMI patients' LVEF after TTPCI.
This study aims to develop a nomogram model predicting early LVEF for patients with TTPCI, according to routine clinical and laboratory indicators at the time of entering our PCI center, which can assist clinical physician in early evaluation of cardiac function, guide the timing of PCI treatment, optimize protection strategies and improve the prognosis.
We conducted a retrospective analysis of data from patients with STEMI who underwent thrombolysis followed by at the regional coordination chest pain center in the General Hospital of Southern Theater Command of PLA between February 2018 and April 2022. All included patients were diagnosed STEMI at five network hospitals of our center with door-to-balloon time of over 120 min. Patients with incomplete clinical data, or first medical contact time (FMC) >12 h, or pre-existing cardiac insufficiency were excluded from the analysis. All patients were given 300 mg aspirin and 300–600 mg clopidogrel immediately after diagnosis, followed by thrombolysis with teneprase (Guangzhou Mingkang Biological Co., Ltd., State Drug Administration S20150001, 16 mg, 10 s intravenous), and anticoagulation with normal heparin sodium or enoxaparin. Vital signs of the patients were observed for 30–120 min after thrombolysis, and then transferred to the General Hospital of Southern Theater Command of PLA. If thrombolysis failed, PCI was performed immediately. Otherwise, coronary angiography or PCI was carried out within 24 h. M-mode echocardiographic measurement was conducted one week after TTPCI and patients were categorized into two groups according to LVEF: reduced group (LVEF < 50%) and preserved group (LVEF ≥ 50%).The formula used for calculation was as follows: LVEF = (left ventricular end-diastolic volume - left ventricular end-systolic volume)/L left ventricular end-diastolic volume × 100%.This study has been approved by the Medical Ethics Committee of the General Hospital of Southern Theater Command of PLA (No. NZLLKZ2022035).
A total of 57 variables were included: (1) demographic data [age, gender, weight, heart rate (HR), etc.]; (2) epidemiological data [hypertension, diabetes, coronary artery disease (CAD), smoking history, alcohol intake, etc.]; (3) time efficiency metrics (first medical contact (FMC), door-to-needle (D-to-N), door-to-balloon (D-to-B), etc.); (4) angiographic features (number and distribution of offender vessels, TIMI blood flow grading, etc.); and (5) clinical and laboratory data. The information was obtained from the database of our chest pain center as well as the electronic medical record system and interventional procedure management system.
Categorical variables were presented as frequency percentages while continuous variables were reported either as median with min-max or mean ± standard deviation. Prior to statistical analysis for normality assessment of continuous variables we used Shapiro–Wilk test. For categorical variables, Pearson's χ2 test or Fisher's exact test was employed while continuous variables were employed either by Student's t-test or Mann–Whitney U-test as appropriate.
All variables were screened by Backward Stepwise Regression according to the Akaike's Information Criterion (AIC). When the AIC value stopped declining, the full subset regression was used to screen for the variable with the largest adjusted R-squared. The odds ratio (OR) and its corresponding 95% confidence interval (CI) were calculated by univariate logistic regression and multivariate logistic regression respectively. The nomogram model was developed with those independent factors. The prediction accuracy was internally verified using the bootstrap self-sampling method, and the results were visually presented in a calibration curve. The discrimination ability of the nomogram was assessed by the receiver operating characteristic (ROC) curve, and a calibration plot was used to determine the degree of agreement between predicted and observed outcomes. The goodness of fit was evaluated using the Hosmer-Lemeshow test.Finally, the decision curve analysis (DCA) was used to assess the clinical utility of the nomogram. All tests were two-sided with an alpha level of 0.05. All data management and statistical analyses were performed using IBM SPSS V24: IBM Corp. Released 2016. IBM SPSS Statistics for Windows, Version 24.0. Armonk, NY: IBM Corp. (You can check the details via https://www.ibm.com/support/pages/howcite-ibm-spss-statistics-or-earlier-versions-spss) and the R software, version 4.1.2.
From 1 February 2018 to 30 April 2022, our center received a total of 305 STEMI patients who had undergone thrombolysis in network hospitals prior to transfer for PCI. Seventeen cases were excluded due to age (<18 years or >80 years), death during transfer, patient refusal of PCI procedure, and incomplete data, and the study ultimately enrolled a total of 288 patients (Figure 1). Among the selected subjects, the LVEF reduction group included 54 (90%) male and 6 (10%) female, while the LVEF preservation group consisted of 212 (93%) males and 16 (7%) females.
Figure 1. Flow chart of the study population selection. A total of 305 patients diagnosed with STEMI in primary hospitals and treated with pharmacological thrombolysis and reperfusion strategy were included in this study. However, 17 patients were excluded because of age >80 years, death in transportion, rejected PCI, or missing data, leaving 288 patients who were divided into two groups according to LVEF measured by echocardiography within 1 week after TTPCI.
There were significant differences in HR, smoking history, thrombolytic recanalization, chest pain relief, reperfusion arrhythmia, congestive heart failure, Killip class, CCU time, length of stay, thirty-day cerebral infarction, thirty-day mortality at admission between the LVEF-reduced (LVEF < 50%) group and LVEF-preserved (LVEF ≥ 50%) group (p < 0.05) (Table 1).
Table 1. Clinical characteristics of patients in the LVEF-reduced and LVEF-preserved groups at admission.
Additionally, the two groups showed great distinctions in white blood cell count (WBC), neutrophil count, neutrophil-to-lymphocyte ratio (NLR), alanine aminotransferase (ALT), blood urea nitrogen (BUN), triglyceride (TG), and D-dimer within 24 h after admission (Table 2).
Table 2. Laboratory parameters of patients in the LVEF-reduced and LVEF-preserved groups at admission.
By the full subset regression analyses, we got a total of 26 potential risk factors (Figure 2A). Six variables were identified as independent risk factors, including age (OR: 0.97; 95% CI: 0.94–1.00; p = 0.037); HR (OR: 0.96; 95% CI: 0.94–0.98; p = 0.001); hypertension (OR: 2.37; 95% CI: 1.14–4.91; p = 0.021); smoking history (OR: 2.82; 95% CI: 1.32–5.98; p = 0.007); ALT (OR: 0.98; 95% CI: 0.97–0.99; p < 0.001); and Killip class [Killip class 2 (OR: 0.33; 95% CI: 0.14–0.79; p = 0.013), Killip class 3 (OR: 0; 95% CI: 0.00-Inf; p = 0.985), Killip class 4 (OR: 0.14; 95% CI:0.02–0.77; p = 0.024)]. They were utilized to build the nomogram for prediction of LVEF ≥ 50% probability in STEMI patients with TTPCI (Figure 2B). The nomogram based on the six independent risk factors is shown in Figure 3. The “Total points” in the model represents the probability of predicting LVEF ≥ 50% for the STEMI patients in the first week after TTPCI.
Figure 2. (A) Screening plot of 26 variables by all-subsets regression, HR, heart rate; SBP, systolic blood pressure; TR, thrombolytic recanalization; WBC, white blood cell; PLT, platelet; dBil, direct bilirubin; Alb, albumin; ALT, alanine aminotransferase; LDL.C, low-density lipoprotein cholesterin; APTT, activated partial thromboplastin time; TT, thrombin time; BNP, brain natriuretic peptide; MYO, myohemoglobin; LAD, left anterior descending; LCA, left circumflex artery; LMC, left main coronary. (B) Regression coefficient of independent risk factors and forest plots. A total of six independent variables were obtained through both univariate and multivariate logistic regression. OR, odds ratios; 95% CI, 95% confidence interval.
Figure 3. A nomogram for predicting the LVEF ≥ 50% probability of STEMI patients with PCI. Age, years; HR, heart rate, rate/min; Hypertension; Smoking history; ALT, alanine aminotransferase, U/L; Killip class. Instructions for using the nomogram: (1) Draw a vertical line based on the value of each variable to obtain the corresponding point; (2) Add all six points to obtain the total point; (3) Draw a vertical line based on the total point to determine the estimated the LVEF ≥ 50% probability one week after TTPCI.
The AUC of ROC curve for the nomogram was 0.84 (95% CI: 0.78–0.89) (Figure 4A). According to the Hosmer-Lemeshow goodness-of-fit test, the nomogram was demonstrated a satisfactory fit (p = 0.713), with a Mean Absolute Error (MAE) of 0.023 for the calibration curve (Figure 4B), which indicated that the prediction model exhibits strong predictive capabilities. Additionaly, the decision curve analysis (DCA) demonstrated that the net benefit of the prediction model was significantly higher compared with the two extreme conditions (Figure 5), suggesting its superior overall net benefit in predicting LVEF among patients with STEMI.
Figure 4. (A) The receiver operating characteristic (ROC) curve (a) and calibration curve (b) for the established nomogram. OR, odds ratios; AUC, area under the curve; 95% CI, 95% confidence interval. Calibration curve reflects the extent to which the model correctly estimates the absolute probability or agreement between the predicted probability and observed outcomes. (B) The y-axis represents the actual LVEF ≥ 50% probability. The x-axis represents the predicted LVEF ≥ 50% probability. The black dot at the top represents the prediction probability corresponding to the actual observation, the black dotted line represents the ideal predicted value, and the solid line represents the actual predicted value.
Figure 5. Determination of decision point via decision curve analysis (DCA) and clinical impact curve. (1) Decision curve for the prediction model. The decision curve analysis graphically shows the clinical usefulness of the nomogram based on a continuum of potential thresholds for LVEF ≥ 50% probability falling (x-axis) and the net benefit of using the nomogram to stratify patients (y-axis). Net benefit curves are plotted across probability thresholds for 6 options: “all” assume all patients have LVEF < 50%, “none” assume no patients have LVEF < 50%. Net benefit = (true positives/N)-(false positives/N) × (weighting factor). Weighting factor = threshold probability/(1-threshold probability). (2) Clinical impact curve for nomogram. The red line shows the total number who would be deemed as high risk of LVEF < 50% for each risk thresh.
Studies have indicated that LVEF of STEMI patient is closely associated with in-hospital and long-term mortality as well as the occurrence of MACE (6–8). Multiple factors can influence early LVEF in patients with STEMI. Arso et al. demonstrated that TTPCI had a significantly higher incidence of in-hospital cardiovascular events compared with PPCI due to variability in patient baseline status, thrombolysis and execution of the transfer process, ultimately leading to greater variability in cardiac function (12). Currently, a multitude of studies (13–15) have utilized single laboratory indicators or demographic data to prognosticate cardiac function in STEMI patients following PCI. However, despite its simplicity and convenience, the overall predictive performance remains suboptimal due to various influencing factors. Our study reported a nomogram for early prediction of LVEF ≥ 50% probability following TTPCI in STEMI patients, incorporating three baseline parameters (age, smoking history, and hypertension) as well as three clinically available measures (HR, ALT, and Killip class).These multidimensional measures collectively provided a comprehensive estimation of the probability of LVEF ≥ 50%. The research error had been eliminated, leading to significant enhancement in the predictive performance of the model.
Age was an independent risk factor for reduced LVEF in STEMI patients after TTPCI in our study. Advanced age leads to impaired endothelial cell function and diffuse coronary artery calcification, increasing the risk of coronary microvascular obstruction (13), which is considered an independent risk factor for unfavorable consequences including frequent coronary events, left ventricular hypertrophy and heart failure in acute myocardial infarction (AMI) (16, 17).Qin et al. found that age ≥75 years was an independent risk factor for heart failure readmission within 30 days in patients treated with PCI, which is similar to our results (18). Therefore, a more aggressive strategy of hemodynamic reconstruction and cardioprotection in elderly STEMI patients is crucial for protection of cardiac function and long-term prognosis.
Smoking history is the well-established independent risk factors which accounts for almost 50% of STEMI patients (19), but its impact on STEMI prognosis remains uncertain. Some studies illustrate that smokers are more sensitive to thrombolytic therapy and experience lower mortality rates in comparison to non-smokers. This is probably related to the fact that the component of coronary obstruction in smokers is mainly acute thrombus rather than chronic atherosclerotic plaque, known as “the smoker's paradox” (20). However, other studies suggest that smoking causes a 2-fold increase in both AMI morbidity and mortality (21, 22). Elderly AMI patients who smoke are particularly vulnerable to long-term mortality (23). In our study, we did not found that smoking had an effect on the reduction of LVEF after TTPCI, which does not negate the damaging outcome that smoking on the cardiovascular system. Prospective studies with larger sample sizes are needed to further observe the relationship between smoking and outcome after TTPCI.
Hypertension is another important factor in AMI complicated by left ventricular dysfunction or congestive heart failure (24, 25). The related mechanisms include: (1) Elevated shear stress in blood leads to impaired vascular endothelial function, thereby compromising the ability of endothelium-mediated hyperpolarization to regulate contraction of smooth muscle cells (26); (2) increased nitric oxide production and inflammatory factors released from vascular endothelial cells in hypertensive patients lead to coronary microcirculatory dysfunction (27); and (3) oxidative stress caused by excessive bioavailability of reactive oxygen species (ROS) in hypertensive patients aggravates vascular damage (28). Thus, hypertension may result in more severe myocardial ischemia and increased cardiac load, thereby affecting cardiac function in STEMI patients.However, our results did not show that hypertension caused a reduction in LVEF for the pupulation with TTPCI. This maybe explained by the cause of a compensatory increase in myocardial systolic function (29). Moreover, standard manage of TTPCI significantly alleviate the effect of ischemia on cardiac function.
Interestingly, our study has shown that elevated ALT is an independent risk factor for predicting a decline in LVEF among STEMI patients. This is because liver receives approximately 25% of the cardiac output (CO) and is more sensitive to hypoperfusion resulting from reduced CO or hepatic artery blood flow (30). Furthermore, decreased CO often leads to hepatic venous stasis, which is the pathological basis of acute cardiogenic liver injury (31). Therefore, ALT reflects the state of organ perfusion and congestion based on cardiac pump function. A clinical trial (32) involving 105 patients with reduced ejection fraction found that AST/ALT ratio independently predicted the severity of cardiac dysfunction while a large study (33) also found that elevated ALT not only strongly correlated with MACEs, but also served as an important predictor of long-term mortality in AMI patients. Therefore, the inclusion of ALT as a predictor in the model reflects the organ-organ interactions in STEMI patients with early cardiac decompensation.
Our study demonstrates that an increased HR is hugely associated with the occurrence of reduced LVEF after TTPCI. HR is a predictor of heart failure and MACEs for AMI patients (34–36).The main pathophysiological mechanisms of HR abnormalities in STEMI patients are related to sympathetic excitation and vagal inhibition (37, 38). The equilibrium of HR is pivotal in upholding the electrical stability of ventricular myocardium and averting the emergence of lethal ventricular arrhythmias (39). A previous study confirmed that heart rate variability (HRV) (40) and heart rate kinetics after AMI are enormously correlated with survival in patients with lower LVEF (41). The Killip class is a widely used to evaluate severity of heart failure after AMI (42). Numerous studies have confirmed the close relationship between higher Killip class and reduced LVEF in STEMI patients (43–45), suggesting higher Killip class increases risk of death (46). In addition, Killip class predicts impaired left ventricular systolic function in AMI patients (43). Our study found that the higher the Killip class was associated with lower the LVEF in STEMI patients, consistent with the previous findings (47), showing the Killip class (OR: 1.449, 95% CI: 1.090–1.928, p = 0.011) as an independent predictor of reduced LVEF (≤45%) at hospital discharge.
In this study, we collected real clinical and laboratory data from STEMI patients who underwent regional cooperative TTPC. We meticulously analyzed the data to eliminate confounding factors, including competing risks. Furthermore, we observed that a combination of old age, elevated heart rate, increased ALT levels, and high Killip class can be suggested a lower probability of LVEF ≥ 50% one week after TTPCI. However, the study had several limitations as well. Firstly, it should be noted that it was a retrospective study and limited by a relatively small sample size. Secondly, the nomogram has not undergone external validation in another cohort of STEMI patients, and its predictive performance requires further confirmation. Thirdly, the LVEF is a relatively single endpoint indicator; thus expanding the sample size is necessary to explore predictive model for long-term prognosis of STEMI patients.
In the study, we developed a nomogram that could accurately predicts the risk of early LVEF in STEMI patients with TTPCI, using easily accessible clinical indicators such as age, HR, hypertension, smoking history, ALT and Killip class. Clinicians can focus on early risk stratification and cardiac function protection with the nomogram so as to select appropriate reperfusion strategies in a timely manner. More prospective studies are expected to further confirm the early benefits of the model for a larger population.
The original contributions presented in the study are included in the article/Supplementary Material, further inquiries can be directed to the corresponding authors.
The studies involving humans were approved by the Medical Ethics Committee of the General Hospital of Southern Theater Command of PLA. The studies were conducted in accordance with the local legislation and institutional requirements. Written informed consent for participation was not required from the participants or the participants’ legal guardians/next of kin because it is a retrospective study and the data was from the electronic database.
LS, HY, Z-YY, and L-YP contributed to the conception and design, acquisition, drafting of the manuscript, and critical revision for important intellectual content. P-SW, J-ZH, and H-YK contributed to the interpretation of the data and analysis. PN and T-YQ contributed to the conception and design, reviewing of the manuscript, and critical revision for important intellectual content. All authors contributed to the article and approved the submitted version.
This present study was supported by grants from the Science and Technology Program of Guangzhou, China (No.201704020157).
The authors would like to express sincere gratitude to all the individuals who have provided with help and guidance at Guangzhou University of Traditional Chinese Medicine and General Hospital of the Southern Theatre Command.
The authors declare that the research was conducted in the absence of any commercial or financial relationships that could be construed as a potential conflict of interest.
All claims expressed in this article are solely those of the authors and do not necessarily represent those of their affiliated organizations, or those of the publisher, the editors and the reviewers. Any product that may be evaluated in this article, or claim that may be made by its manufacturer, is not guaranteed or endorsed by the publisher.
1. Xiang D, Xiang X, Zhang W, Yi S, Zhang J, Gu X, et al. Management and outcomes of patients with STEMI during the COVID-19 pandemic in China. J Am Coll Cardiol. (2020) 76(11):1318–24. doi: 10.1016/j.jacc.2020.06.039
2. Reed GW, Rossi JE, Cannon CP. Acute myocardial infarction. Lancet. (2017) 389(10065):197–210. doi: 10.1016/s0140-6736(16)30677-8
3. Heidenreich PA, Bozkurt B, Aguilar D, Allen LA, Byun JJ, Colvin MM, et al. 2022 AHA/ACC/HFSA guideline for the management of heart failure: a report of the American college of cardiology/American heart association joint committee on clinical practice guidelines. J Am Coll Cardiol. (2022) 79(17):e263–421. doi: 10.1016/j.jacc.2021.12.012
4. Ibanez B, James S, Agewall S, Antunes MJ, Bucciarelli-Ducci C, Bueno H, et al. 2017 ESC guidelines for the management of acute myocardial infarction in patients presenting with ST-segment elevation: the task force for the management of acute myocardial infarction in patients presenting with ST-segment elevation of the European society of cardiology (ESC). Eur Heart J. (2018) 39(2):119–77. doi: 10.1093/eurheartj/ehx393
5. Ting HH, Rihal CS, Gersh BJ, Haro LH, Bjerke CM, Lennon RJ, et al. Regional systems of care to optimize timeliness of reperfusion therapy for ST-elevation myocardial infarction: the mayo clinic STEMI protocol. Circulation. (2007) 116(7):729–36. doi: 10.1161/CIRCULATIONAHA.107.699934
6. Sun LY, Gaudino M, Chen RJ, Bader Eddeen A, Ruel M. Long-term outcomes in patients with severely reduced left ventricular ejection fraction undergoing percutaneous coronary intervention vs coronary artery bypass grafting. JAMA Cardiol. (2020) 5(6):631–41. doi: 10.1001/jamacardio.2020.0239
7. Gallone G, Kang J, Bruno F, Han JK, De Filippo O, Yang HM, et al. Impact of left ventricular ejection fraction on procedural and long-term outcomes of bifurcation percutaneous coronary intervention. Am J Cardiol. (2022) 172:18–25. doi: 10.1016/j.amjcard.2022.02.015
8. Chen YW, Lee WC, Fang HY, Sun CK, Sheu JJ. Coronary artery bypass graft surgery brings better benefits to heart failure hospitalization for patients with severe coronary artery disease and reduced ejection fraction. Diagnostics. (2022) 12(9):2233. doi: 10.3390/diagnostics12092233
9. Gavara J, Marcos-Garces V, Lopez-Lereu MP, Monmeneu JV, Rios-Navarro C, de Dios E, et al. Magnetic resonance assessment of left ventricular ejection fraction at any time post-infarction for prediction of subsequent events in a large multicenter STEMI registry. J Magn Reson Imaging. (2022) 56(2):476–87. doi: 10.1002/jmri.27789
10. Parenica J, Kala P, Pavkova MG, Tomandl J, Spinar J, Littnerova S, et al. Natriuretic peptides, nitrite/nitrate and superoxide dismutase have additional value on top of the GRACE score in prediction of one-year mortality and rehospitalisation for heart failure in STEMI patients - multiple biomarkers prospective cohort study. Int J Cardiol. (2016) 211:96–104. doi: 10.1016/j.ijcard.2016.02.135
11. Lindberg S, Jensen JS, Pedersen SH, Galatius S, Goetze JP, Mogelvang R. MR-proANP improves prediction of mortality and cardiovascular events in patients with STEMI. Eur J Prev Cardiol. (2015) 22(6):693–700. doi: 10.1177/2047487314538856
12. Arso IA, Setianto BY, Taufiq N, Hartopo AB. In-hospital major cardiovascular events between STEMI receiving thrombolysis therapy and primary PCI. Acta Med Indones. (2014) 46(2):124–30.25053685
13. Herrmann J, Lerman A. The endothelium: dysfunction and beyond. J Nucl Cardiol. (2001) 8(2):197–206. doi: 10.1067/mnc.2001.114148
14. Vatan MB, Çakmak AC, Ağaç S, Eynel E, Erkan H. The systemic immune-inflammation index predicts impaired myocardial perfusion and short-term mortality in ST-segment elevation myocardial infarction patients. Angiology. (2023) 74(4):365–73. doi: 10.1177/00033197221106886
15. Li J, Zhao Z, Jiang H, Jiang M, Yu G, Li X. Predictive value of elevated alanine aminotransferase for in-hospital mortality in patients with acute myocardial infarction. BMC Cardiovasc Disord. (2021) 21(1):82. doi: 10.1186/s12872-021-01903-z
16. De Luca L, Cicala SD, D'Errigo P, Cerza F, Mureddu GF, Rosato S, et al. Impact of age, gender and heart failure on mortality trends after acute myocardial infarction in Italy. Int J Cardiol. (2022) 348:147–51. doi: 10.1016/j.ijcard.2021.12.023
17. Fam JM, Khoo CY, Lau YH, Lye WK, Cai XJ, Choong LHL, et al. Age and diabetes mellitus associated with worse outcomes after percutaneous coronary intervention in a multi-ethnic Asian dialysis patient population. Singapore Med J. (2021) 62(6):300–4. doi: 10.11622/smedj.2020025
18. Qin Y, Wei X, Han H, Wen Y, Gu K, Ruan Y, et al. Association between age and readmission after percutaneous coronary intervention for acute myocardial infarction. Heart. (2020) 106(20):1595–603. doi: 10.1136/heartjnl-2019-316103
19. Steele L, Lloyd A, Fotheringham J, Sultan A, Iqbal J, Grech ED. A retrospective cross-sectional study on the association between tobacco smoking and incidence of ST-segment elevation myocardial infarction and cardiovascular risk factors. Postgrad Med J. (2015) 91(1079):492–6. doi: 10.1136/postgradmedj-2015-133269
20. Gupta T, Kolte D, Khera S, Harikrishnan P, Mujib M, Aronow WS, et al. Smoker’s paradox in patients with ST-segment elevation myocardial infarction undergoing primary percutaneous coronary intervention. J Am Heart Assoc. (2016) 5(4):3370. doi: 10.1161/JAHA.116.003370
21. Sia CH, Ko J, Zheng H, Ho AF, Foo D, Foo LL, et al. Association between smoking status and outcomes in myocardial infarction patients undergoing percutaneous coronary intervention. Sci Rep. (2021) 11(1):6466. doi: 10.1038/s41598-021-86003-w
22. Banks E, Joshy G, Korda RJ, Stavreski B, Soga K, Egger S, et al. Tobacco smoking and risk of 36 cardiovascular disease subtypes: fatal and non-fatal outcomes in a large prospective Australian study. BMC Med. (2019) 17(1):128. doi: 10.1186/s12916-019-1351-4
23. Bucholz EM, Beckman AL, Kiefe CI, Krumholz HM. Smoking status and life expectancy after acute myocardial infarction in the elderly. Heart. (2016) 102(2):133–9. doi: 10.1136/heartjnl-2015-308263
24. Reinstadler SJ, Stiermaier T, Eitel C, Saad M, Metzler B, de Waha S, et al. Antecedent hypertension and myocardial injury in patients with reperfused ST-elevation myocardial infarction. J Cardiovasc Magn Reson. (2016) 18(1):80. doi: 10.1186/s12968-016-0299-1
25. De Luca G, Dirksen MT, Spaulding C, Kelbæk H, Schalij M, Thuesen L, et al. Impact of hypertension on clinical outcome in STEMI patients undergoing primary angioplasty with BMS or DES: insights from the DESERT cooperation. Int J Cardiol. (2014) 175(1):50–4. doi: 10.1016/j.ijcard.2014.04.180
26. Goto K, Ohtsubo T, Kitazono T. Endothelium-dependent hyperpolarization (EDH) in hypertension: the role of endothelial Ion channels. Int J Mol Sci. (2018) 19(1):315. doi: 10.3390/ijms19010315
27. Xu BL, Zhou WL, Zhu TP, Cheng KY, Li YJ, Zhan HJ, et al. A full-width half-maximum method to assess retinal vascular structural changes in patients with ischemic heart disease and microvascular anginga. Sci Rep. (2019) 9(1):11019. doi: 10.1038/s41598-019-47194-5
28. Montezano AC, Dulak-Lis M, Tsiropoulou S, Harvey A, Briones AM, Touyz RM. Oxidative stress and human hypertension: vascular mechanisms, biomarkers, and novel therapies. Can J Cardiol. (2015) 31(5):631–41. doi: 10.1016/j.cjca.2015.02.008
29. Fung MJ, Thomas L, Leung DY. Left ventricular function and contractile reserve in patients with hypertension. Eur Heart J Cardiovasc Imaging. (2018) 19(11):1253–9. doi: 10.1093/ehjci/jex338
30. Bannon L, Merdler I, Bar N, Lupu L, Banai S, Jacob G, et al. The cardio-hepatic relation in STEMI. J Pers Med. (2021) 11(12):1241. doi: 10.3390/jpm11121241
31. Wells ML, Venkatesh SK. Congestive hepatopathy. Abdom Radiol. (2018) 43(8):2037–51. doi: 10.1007/s00261-017-1387-x
32. Ewid M, Sherif H, Allihimy AS, Alharbi SA, Aldrewesh DA, Alkuraydis SA, et al. AST/ALT ratio predicts the functional severity of chronic heart failure with reduced left ventricular ejection fraction. BMC Res Notes. (2020) 13(1):178. doi: 10.1186/s13104-020-05031-3
33. Baars T, Sowa JP, Neumann U, Hendricks S, Jinawy M, Kälsch J, et al. Liver parameters as part of a non-invasive model for prediction of all-cause mortality after myocardial infarction. Arch Med Sci. (2020) 16(1):71–80. doi: 10.5114/aoms.2018.75678
34. Perne A, Schmidt FP, Hochadel M, Giannitsis E, Darius H, Maier LS, et al. Admission heart rate in relation to presentation and prognosis in patients with acute myocardial infarction. Treatment regimens in German chest pain units. Herz. (2016) 41(3):233–40. doi: 10.1007/s00059-015-4355-7
35. Cygankiewicz I. Heart rate turbulence. Prog Cardiovasc Dis. (2013) 56(2):160–71. doi: 10.1016/j.pcad.2013.08.002
36. Parodi G, Bellandi B, Valenti R, Memisha G, Giuliani G, Velluzzi S, et al. Heart rate as an independent prognostic risk factor in patients with acute myocardial infarction undergoing primary percutaneous coronary intervention. Atherosclerosis. (2010) 211(1):255–9. doi: 10.1016/j.atherosclerosis.2010.02.017
37. Zuern CS, Eick C, Rizas KD, Stoleriu C, Barthel P, Scherer C, et al. Severe autonomic failure in moderate to severe aortic stenosis: prevalence and association with hemodynamics and biomarkers. Clin Res Cardiol. (2012) 101(7):565–72. doi: 10.1007/s00392-012-0427-3
38. Sestito A, Valsecchi S, Infusino F, Sgueglia GA, Bellocci F, Zecchi P, et al. Differences in heart rate turbulence between patients with coronary artery disease and patients with ventricular arrhythmias but structurally normal hearts. Am J Cardiol. (2004) 93(9):1114–8. doi: 10.1016/j.amjcard.2004.01.037
39. Laborde S, Mosley E, Thayer JF. Heart rate variability and cardiac vagal tone in psychophysiological research - recommendations for experiment planning, data analysis, and data reporting. Front Psychol. (2017) 8:213. doi: 10.3389/fpsyg.2017.00213
40. Yıldız BS, Özkan E, Esin F, Özkan H, Alihanoğlu Y, Kılıç İD, et al. Evaluation of heart rate variability in patients with coronary artery ectasia and coronary artery disease. Turk Kardiyol Dern Ars. (2016) 44(4):306–14. doi: 10.5543/tkda.2015.84899
41. Hayano J, Ueda N, Kisohara M, Yuda E, Carney RM, Blumenthal JA. Survival predictors of heart rate variability after myocardial infarction with and without low left ventricular ejection fraction. Front Neurosci. (2021) 15:610955. doi: 10.3389/fnins.2021.610955
42. Taguchi E, Konami Y, Inoue M, Suzuyama H, Kodama K, Yoshida M, et al. Impact of killip classification on acute myocardial infarction: data from the SAIKUMA registry. Heart Vessels. (2017) 32(12):1439–47. doi: 10.1007/s00380-017-1017-0
43. Bordejevic DA, Pârvănescu T, Petrescu L, Mornoș C, Olariu I, Crișan S, et al. Left ventricular remodeling risk predicted by two-dimensional speckle tracking echocardiography in acute myocardial infarction patients with midrange or preserved ejection fraction in western Romania. Ther Clin Risk Manag. (2021) 17:249–58. doi: 10.2147/TCRM.S295251
44. Alzuhairi KS, Lønborg J, Ahtarovski KA, Nepper-Christensen L, Kyhl K, Lassen JF, et al. Sub-acute cardiac magnetic resonance to predict irreversible reduction in left ventricular ejection fraction after ST-segment elevation myocardial infarction: a DANAMI-3 sub-study. Int J Cardiol. (2020) 301:215–9. doi: 10.1016/j.ijcard.2019.10.034
45. Yao J, Xie Y, Liu Y, Tang Y, Xu J. Prediction factors of 6-month poor prognosis in acute myocardial infarction patients. Front Cardiovasc Med. (2020) 7:130. doi: 10.3389/fcvm.2020.00130
46. de Carvalho LP, Gao F, Chen Q, Sim LL, Koh TH, Foo D, et al. Long-term prognosis and risk heterogeneity of heart failure complicating acute myocardial infarction. Am J Cardiol. (2015) 115(7):872–8. doi: 10.1016/j.amjcard.2015.01.010
Keywords: STEMI, TTPCI, LVEF, prediction model, nomogram
Citation: Liu S, Jiang Z, Zhang Y, Pang S, Hou Y, Liu Y, huang Y, Peng N and Tang Y (2023) A nomogramic model for predicting the left ventricular ejection fraction of STEMI patients after thrombolysis-transfer PCI. Front. Cardiovasc. Med. 10:1178417. doi: 10.3389/fcvm.2023.1178417
Received: 21 March 2023; Accepted: 23 August 2023;
Published: 8 September 2023.
Edited by:
Konstantinos Koskinas, Inselspital University Hospital Bern, SwitzerlandReviewed by:
Su Ozgur, EgeSAM-Ege University Translational Pulmonary Research Center, Türkiye© 2023 Liu, Jiang, Zhang, Pang, Hou, Liu, huang, Peng and Tang. This is an open-access article distributed under the terms of the Creative Commons Attribution License (CC BY). The use, distribution or reproduction in other forums is permitted, provided the original author(s) and the copyright owner(s) are credited and that the original publication in this journal is cited, in accordance with accepted academic practice. No use, distribution or reproduction is permitted which does not comply with these terms.
*Correspondence: Na Peng cG5hdHpAMTYzLmNvbQ== Youqing Tang MjY4OTc2MDAyOUBxcS5jb20=
†These authors have contributed equally to this work and share first authorship
Disclaimer: All claims expressed in this article are solely those of the authors and do not necessarily represent those of their affiliated organizations, or those of the publisher, the editors and the reviewers. Any product that may be evaluated in this article or claim that may be made by its manufacturer is not guaranteed or endorsed by the publisher.
Research integrity at Frontiers
Learn more about the work of our research integrity team to safeguard the quality of each article we publish.