- 1Department of Neurology, Kyung Hee University College of Medicine, Kyung Hee University Hospital, Seoul, Republic of Korea
- 2Department of Neurology, Seoul Hospital, Ewha Womans University College of Medicine, Seoul, Republic of Korea
Background: Body mass index (BMI) is insufficient evidence as a risk factor for numerous health disorders. Body composition may be more appropriate for confirming the association with cardiovascular diseases, including atrial fibrillation (AF). This study aimed to examine the association between body composition and the occurrence of AF.
Methods: A total of 2,673,108 participants (48.6% women) without AF at baseline from the Korean national health insurance data were included. Body composition including appendicular skeletal muscle mass, body fat mass, and lean body mass were indirectly measured through validated anthropometric prediction equations. The diagnosis of AF and comorbidities were defined.
Results: With a median of 9.5 (interquartile range 9.2–10.1) years’ follow-up, 25,841 (0.96%) cases of incident AF were included. In multivariable analysis, higher appendicular skeletal muscle was related to low risk of AF [hazard ratio (HR) 0.829, 95% confidence interval (CI) 0.753–0.912 for men (fifth quintile) and HR 0.888, 95% CI 0.792–0.995 for women (fifth quintile)]. In contrast, a higher body fat mass [HR 1.345, 95% CI 1.221–1.483 for men (fifth quintile) and HR 1.420, 95% CI 1.274–1.591 for women (fifth quintile)] and lean body mass [HR 2.241, 95% CI 2.182–2.303 for men (fifth quintile) and HR 1.516, 95% CI 1.368–1.667 for women (fifth quintile)] were associated with the occurrence of AF.
Conclusions: In this study, body composition parameters were associated with the occurrence of AF. It should be noted that when appendicular skeletal muscle mass decreases and body fat mass and lean body mass increase, the risk of AF may be increased in general population except underweighted BMI group.
1. Introduction
Atrial fibrillation (AF) is the most common cardiac arrhythmia that increases the possibility of mortality, stroke, and systemic embolism (1). Globally, the present age has entered the age of aging, and the incidence of vascular risk factors has also increased. These changes have caused the incidence of AF to increase in Asian and Western populations continuously (2, 3). Therefore, it is important to control and recognize the association or risk factors for the occurrence of AF. To date, risk factors for AF, including hypertension, diabetes mellitus, coronary artery occlusive disease, aortic atheroma, poor oral hygiene, smoking, and cardiomyopathy, have been suggested. However, information regarding further modifiable associative or risk factors for AF is still lacking (4–6).
Obesity is explained as an excess of health-impairing fat mass and is commonly defined as a body mass index (BMI) ≥30 kg/m2 (7). The worldwide prevalence of obesity has increased over the past few decades, and the global burden of obesity is still increasing (8). It is well known that obesity, especially high levels of fat mass, worsens cardiovascular risk factors, including hypertension, lipoprotein metabolism, insulin resistance, and inflammation (9, 10). Considering the association of obesity with AF, obesity is associated with an increase in AF (11, 12). Therefore, it is important to develop an accurate method that measures obesity. Although BMI is frequently used to define obesity, the results of studies using BMI to determine the relationship between obesity and AF are inconsistent. Previous studies that defined obesity as an increased BMI showed a positive correlation between obesity and the risk of AF (13). On the other hand, other studies have showed the obesity paradox, where obese and overweight patients with AF, have a better prognosis than their leaner counterparts (14, 15). Therefore, body composition parameters, not simply BMI, may be more appropriate to confirm the association with disease (16).
To date, studies regarding the relationship between body composition and AF have been limited. In a previous study, increased body fat indices, independent of BMI, were related to the incidence of AF (17). Moreover, another study showed that high lean body mass was a determinant of AF incidence in postmenopausal women (18). Nevertheless, there have been few studies of longitudinal design targeting a general population of large sample sizes on the relationship of body composition with the occurrence of AF. Therefore, we aimed to investigate the association of predicted appendicular skeletal muscle mass index (pASMMI), predicted body fat mass index (pBFMI), and predicted lean body mass index (pLBMI), which were derived from an equation previously validated in the Korean population (16), with the occurrence of AF in a longitudinal setting.
2. Methods
2.1. Participants
This study was performed using the National Health Insurance Service Health Screening dataset (NHIS-HEALS) provided by the Korean government. In South Korea, adults over the age of 20 are supported to undergo free health screening every other year. The Korean government combined the results of national health screening with age, sex, and sociodemographic data as well as the national health claim data, which 97% of the South Korean population are enrolled, including household income and medical history, which includes diagnostic codes, medication prescriptions, treatment or procedure information, hospitalization, and date of mortality (19). Of these data, 2,815,596 participants who underwent a national health examination between 2010 and 2011 and aged between 20 and 79 years were used to construct a dataset (NHIS-2021-1-715) and used it through a predetermined identification and validation process (6, 20). Among 2,815,596 participants, those (n = 130,044) with at least one missing value regarding demographic data, lifestyle, and laboratory findings were excluded. Patients with a previous history of AF (n = 12,444) were excluded. Finally, 2,673,108 participants were included in this study (Figure 1). Our study was permitted by the Institutional Review Board of Ewha Womans University Seoul Hospital (Institutional Review Board approval number: SEUMC 2022-02-018).
2.2. Predicted body composition and covariates
pASMMI, pBFMI, and pLBMI were investigated using validated anthropometric prediction equations from the Korean National Health and Nutrition Examination Survey cohort (16). In a previous study, body fat mass, lean body mass, and appendicular skeletal muscle mass were identified using dual-energy x-ray absorptiometry, and a prediction equation was constructed using different combinations of age, height, weight, level of serum creatinine, waist circumference, and lifestyle factors (physical activity, alcohol use, and smoking habit) as predictor variables (16). The combination of age, height, weight, waist circumference, serum creatinine level, and lifestyle factors were chosen in this study to create equations for the body composition. This predictive model was validated as having high predictive power, a moderate agreement rate, and low bias in the Korean general population. Appendicular skeletal muscle mass, lean body mass, and body fat mass were presented as an index (weight [kg] divided by height squared [m2]) for pASMMI, pBFMI, and pLBMI, respectively (Supplementary Methods 1). Predicted body composition including pASMMI, pBFMI, and pLBMI divided into quintiles (Supplementary Methods 2).
Detailed definitions of covariates are provided in Supplementary Methods 3 and previous studies (21). Variables including age, sex, BMI, household income (quartiles), smoking status (never, former, or current), alcohol consumption (none, moderate, or heavy), physical activity (low or moderate), comorbidities (hypertension, diabetes mellitus, dyslipidemia, cancer, renal disease, chronic obstructive pulmonary disease, obstructive sleep apnea syndrome, peripheral arterial disease, heart failure, and liver disease) were collected (6, 22, 23). Comorbidities were defined considering the International Classification of Diseases, Tenth Revision (ICD-10) codes, medication history, and laboratory findings from health examinations (6, 22).
2.3. Outcomes
The primary outcome was an occurrence. The index date was considered the date of the national health examination. If more than one check-up was performed between 2010 and 2011, the most recent health examination results were used for statistical analysis. The diagnostic accuracy of the ICD-10 code (I48) for AF in the NHIS has been validated (94%) (6). Follow-up was carried out until 31 December 2020 or until the first occurrence of death or AF.
2.4. Statistical analysis
Chi-squared tests and independent t-tests were performed to compare and categorical and continuous variables, respectively. For categorical variables, we tested the proportional hazard assumption using the Schoenfeld residuals. No departure from the proportional hazard assumption was detected (Supplementary Methods 4). The Cox proportional hazards model presented with hazard ratios (HR) with 95% confidence intervals (CI) was used to estimate the effect of body composition, pASMMI, pBFMI, and pLBMI, on the incidence of AF. In multivariable analysis, the following potential confounders were adjusted: BMI, household income, hypertension, diabetes mellitus, dyslipidemia, cancer, renal disease, chronic obstructive pulmonary disease, obstructive sleep apnea syndrome, peripheral arterial disease, heart failure, and liver disease. Generally standardized criteria of muscle and fat masses are not available currently. Sarcopenia is defined by authorized working groups such as the European Working Group on Sarcopenia in Older Persons and the Asian Working Group for Sarcopenia as the lowest quintile of study population (24, 25). According to these previous studies, we defined the lowest (first) quintile of pASMMI, pBFMI, and pLBMI from the study population as the reference group. For the sensitivity analysis, regression methods of Fine and Gray for competing risk data (death was a competing event for AF) were utilized according to sex. Subgroup analyses were performed with pASMMI, pBFMI, and pLBMI on the incidence of AF according to BMI categories; underweight (<18.5 kg/m2), normal weight (18.5–24.99 kg/m2), overweight (25–29.99 kg/m2), and obesity (≥30 kg/m2). Statistical analyses were performed using the SAS 9.4 version (SAS Inc., Cary, NC, USA) and R software, version 3.3.3 (R Foundation for Statistical Computing, Vienna, Austria). Two-sided P-values less than 0.05 were considered significant.
3. Results
Table 1 shows the results of comparing the baseline characteristics of 2,673,108 participants according to sex (n = 1,375,179 for men, n = 1,297,929 for women). The mean age of men was 47.47 ± 13.74 years and of women was 49.76 ± 14.28 years. Significant differences in BMI, smoking status, alcohol consumption, household income, physical activity, and comorbidities were observed between men and women (Table 1). According to BMI categories, the proportion of underweight, normal, overweight and obesity were as follows 3.7% (2.1% for men and 5.3% for women), 63.2% (59.3% for men and 67.3% for women), 29.3% (34.6% for men and 23.8% for women), and 3.8% (4.0% for men and 3.6% for women), respectively. The pASMMI (8.28 ± 0.78 kg/m2 for men vs. 6.26 ± 0.57 kg/m2 for women), pBFMI (5.39 ± 1.43 kg/m2 for men vs. 7.64 ± 1.94 kg/m2 for women), and pLBMI (18.64 ± 1.68 kg/m2 for men vs. 15.40 ± 1.39 kg/m2 for women) were significantly different according to sex (P < 0.001).
In multivariable analysis, higher pASMMI was related to low risk of AF regardless of sex [HR 0.829, 95% CI 0.753–0.912, P = 0.001 for men (fifth quintile) and HR 0.888, 95% CI 0.792–0.995, P = 0.041 for women (fifth quintile)] (Table 2). In contrast, higher pBFMI [HR 1.345, 95% CI 1.221–1.483, P < 0.001 for men (fifth quintile) and HR 1.420, 95% CI 1.274–1.591, P < 0.001 for women (fifth quintile)] and pLBMI [HR 2.241, 95% CI 2.182–2.303, P < 0.001 for men (fifth quintile) and HR 1.516, 95% CI 1.368–1.667, P < 0.001 for women (fifth quintile)] were positively related to an increased possibility of AF regardless of sex (Table 2). Similar associations were also observed after considering the competing risk of mortality (Table 3).
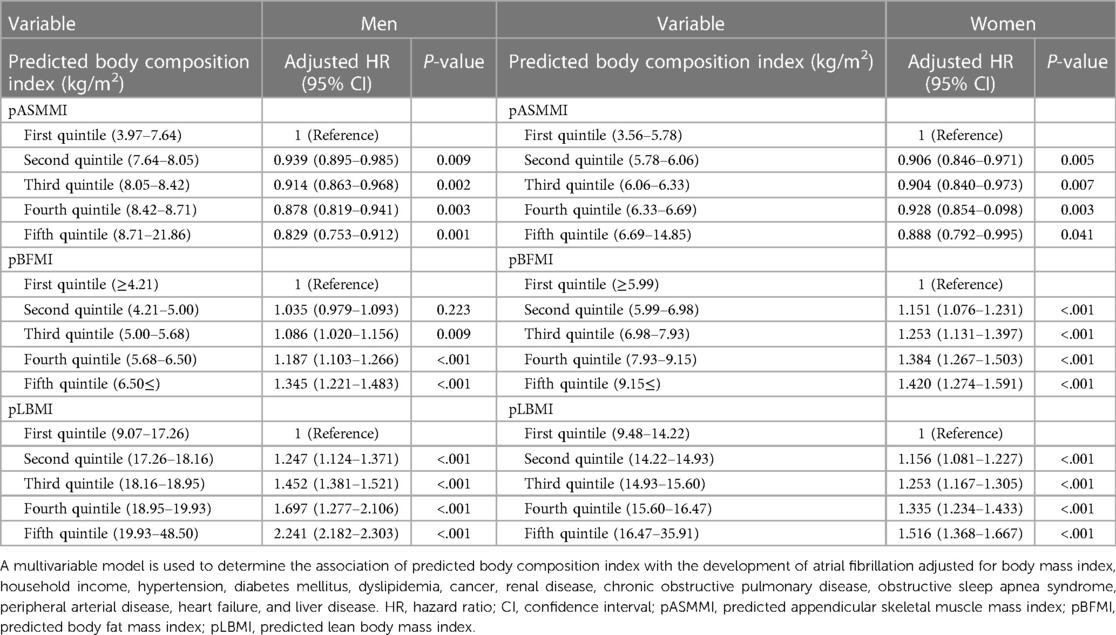
Table 2. Hazard ratios with 95% confidence interval for atrial fibrillation in multivariable Cox proportional hazards model.
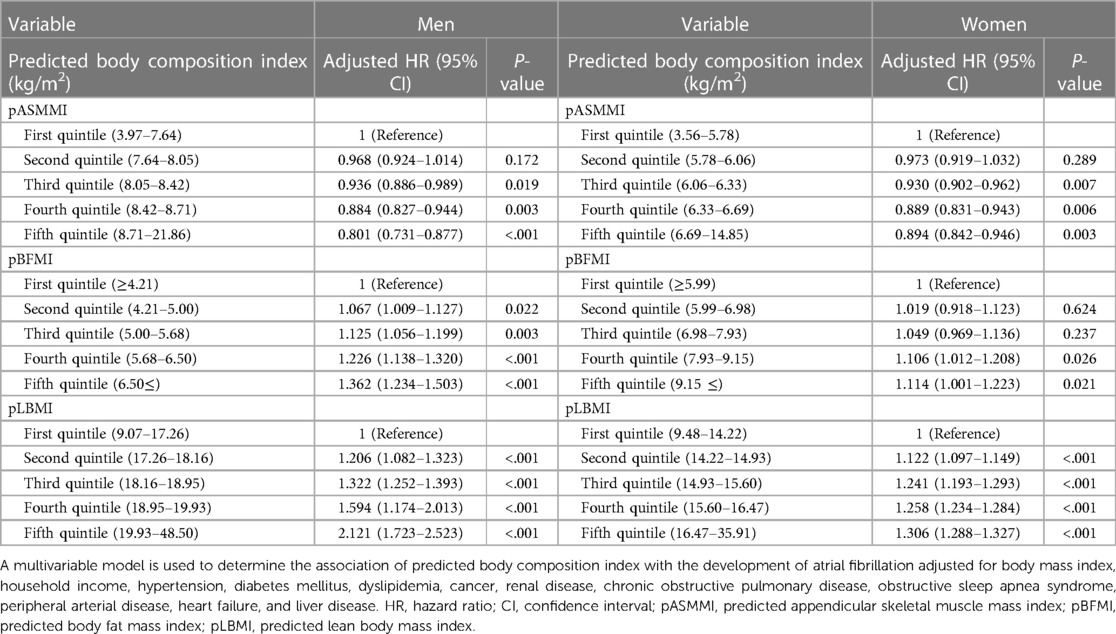
Table 3. Hazard ratios with 95% confidence interval for atrial fibrillation in competing risk analysis with fine - gray model.
The results for the association of body composition with incident AF according to BMI subgroups are shown in Table 4 and Figures 2A,B. A higher pASMMI was related to a decreased possibility of AF in both sexes. In contrast, a higher pLBMI was also related to an increased risk of AF, except the underweight group in both sexes. Moreover, increased pBFMI was related to the incidence of AF, except the underweight group in men.
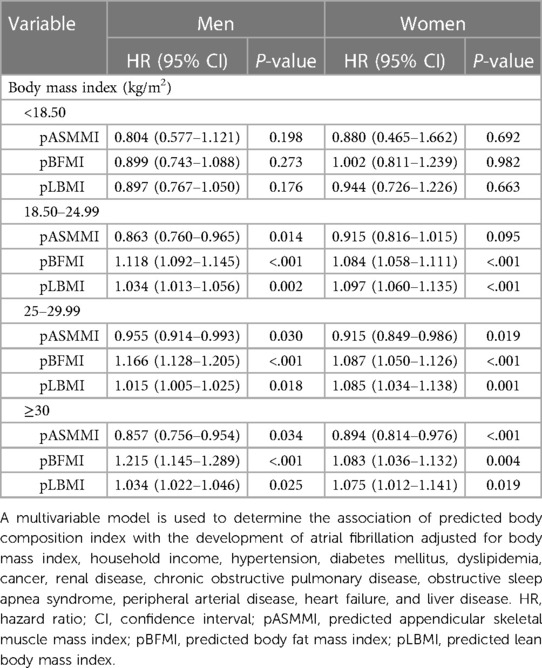
Table 4. Subgroup analysis according to body mass index categories regarding predicted body composition indices and atrial fibrillation.
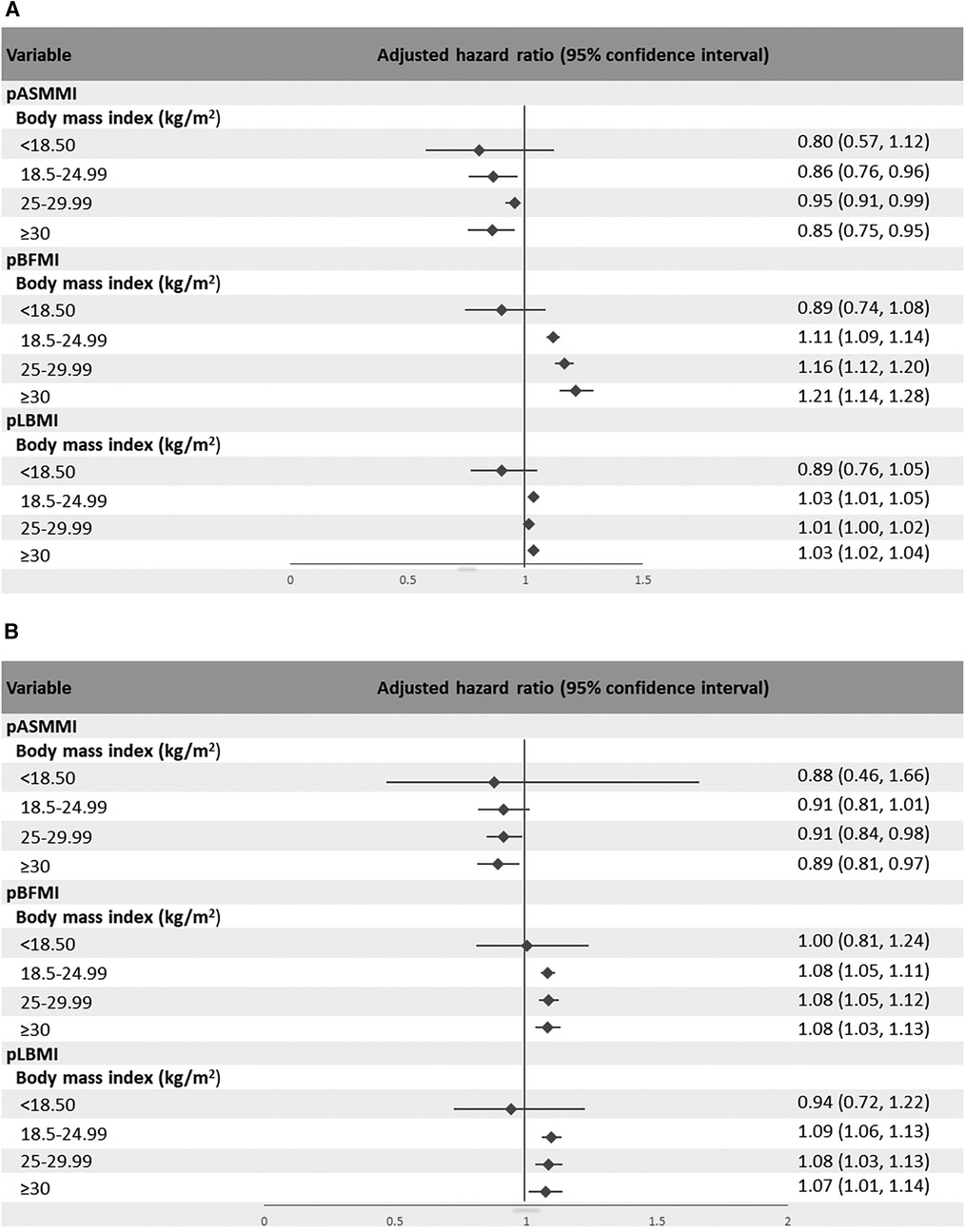
Figure 2. Forest plot showing the hazard ratio and 95% confidence intervals of the association between body composition indices according to subcategories of body mass index and atrial fibrillation for (A) men and (B) women.
4. Discussion
The main finding of our study was that pASMMI was negatively associated with the risk of AF, and pBFMI and pLBMI were positively associated with the incidence of AF.
Generally, skeletal muscle mass is considered beneficial to health. However, it is rarely known how skeletal muscle mass affects for risk of AF. A previous study showed that patients with AF have a lower percentage of skeletal muscle mass (26). In addition, both sarcopenia and the ratio of muscle components are associated with electrocardiogram abnormalities, including AF (27). In line with previous report that sarcopenic overweight/obese was associated with risk of AF (28) and sarcopenia was associated with cardiovascular disease including AF in older patients (27), our report that pASMMI showed a negative association with risk of AF regardless BMI. Because skeletal muscle generates considerable metabolic and oxygen demand in the body, it affects cardiac muscle, cardiac output, and heart rate (29).
Many previous epidemiologic studies have shown that obesity increases the risk of AF; however, confusion, including unexpected J- or U-shaped associations, has often been observed (30). Some studies in relation to obesity showed that greater lean body mass was a strong independent risk factor for AF, and fat mass was also related to a higher risk of AF (17, 18, 31, 32). Although previous studies showed that body fat composition/distribution, which may be linked to non-alcoholic fatty liver disease (NAFLD), has been associated with a higher occurrence of AF (33, 34), our study showed that body fat composition was associated with occurrence of AF after adjusting liver disease including NAFLD. Furthermore, though several controversies exist, regardless of BMI, our study showed a positive relationship between higher pBFMI and the incidence of AF, in line with the findings of previous studies (27, 32). Body fat mass is related to an increased risk of hypertension, insulin resistance, diabetes, coronary heart disease, and heart failure, which contribute to AF (34). Furthermore, increased left atrial size, volume overload, left ventricular diastolic dysfunction, and left atrial dysfunction due to body fat mass further contribute to electrophysiological remodeling and conduction abnormalities resulting in AF (35, 36).
Furthermore, our study showed a positive relationship between pLBMI and the risk of AF, in line with the findings of previous studies (31, 32, 37). But, because participants with low lean body mass were a relatively unhealthy group (38), effect lowering the risk of AF might be offset in participants with underweighted BMI. Also, in present study, the absolute number of underweighted BMI groups is small. For those factors, the significant association between high lean body mass and AF risk might not be observed in underweighted BMI group. In addition, because most overweight and obese people have high predicted lean body mass and fat mass, a higher pLBMI was related to an increased risk of AF in overweight and obese participants (38).
In participants with underweight BMI, we observed a negative relationship between pLBMI and pASMMI and the risk of AF. A previous study suggested that being underweight was significantly related to an increased risk of AF (39). There are several potential mechanisms underlying this obesity paradox. An animal study suggested that the loss of myostatin, a well-known negative regulator of skeletal muscle growth that causes sarcopenia, can lead to AF (40). Studies have linked AF to a deficiency of trace elements (41). However, all of the predicted body composition indices were not significantly related to the occurrence of AF in underweight participants due to the small number of underweight participants.
The strength of our study is that it showed an association between body composition indices and AF in a large sample of the general Korean population in a longitudinal setting. Furthermore, our study could potentially serve as evidence for future randomized clinical trials investigating whether the risk of AF changes with the regulation of body composition and also facilitate future comparative research with direct measurements of body composition using dual energy x-ray absorptiometry or computed tomography scan. In addition, our findings could be practically utilized as evidence for the importance of maintaining a healthy body composition. In other words, our results could be used as educational materials for the general population, highlighting that the potential benefits of maintaining or increasing skeletal muscle mass and decreasing body fat mass cause reducing the risk of AF. However, our study has some limitations. First, although it was conducted with a longitudinal design, this is a retrospective study; therefore, we could not confirm the causal relationship or exclude confounders. Second, we used the equation for predicted body composition validated in the Korean population for large-scale analysis rather than directly conducting dual-energy x-ray absorptiometry. In addition, because the equation was estimated for the Korean population, these findings may not be generalizable to other ethnicities. Third, the predicted body composition indices were measured once or twice during the study period; thus, possible serial changes in body composition were not considered. Finally, this is an epidemiological study that cannot explain the basic mechanism of the association between body composition and AF.
In conclusion, our results demonstrated that a lower muscle mass, higher lean body mass, and higher fat mass were related to an increased risk of AF in general population except underweighted BMI group. These associations between AF and body composition differed according to the BMI categories. For studies with longitudinal settings and large sample sizes, body composition, including body fat mass, lean body mass, and skeletal muscle mass, may be more accurate in confirming the association with AF than BMI.
Data availability statement
The raw data supporting the conclusions of this article will be made available by the authors, without undue reservation.
Ethics statement
The studies involving humans were approved by the Institutional Review Board of Ewha Womans University Seoul Hospital (Institutional Review Board approval number: SEUMC 2022-02-018) The studies were conducted in accordance with the local legislation and institutional requirements. Informed consent was waived because retrospective anonymized data were used.
Author contributions
HGW, MKK, and T-JS contributed to the conception or design of the work; HGW and T-JS contributed to the acquisition, analysis, or interpretation of data for the work; HGW, MKK, and T-JS drafted the manuscript; HGW, MKK, and T-JS critically revised the manuscript. All gave final approval and agreed to be accountable for all aspects of work ensuring integrity and accuracy. All authors contributed to the article and approved the submitted version.
Funding
This work was supported by the National Research Foundation of Korea (NRF) grant funded by the Korea government (MSIT) (No. RS-2023-00239251 to HGW). This project was supported by a grant from the Basic Science Research Program through the National Research Foundation of Korea funded by the Ministry of Education (2021R1F1A1048113 to T-JS,). This work was supported by the Institute of Information & Communications Technology Planning & Evaluation (IITP) grant funded by the Korean government (MSIT) (2022-0-00621 to T-JS, Development of artificial intelligence technology that provides dialog-based multi-modal explainability). This research was supported by a grant from the Korea Health Technology R&D Project through the Korea Health Industry Development Institute (KHIDI), funded by the Ministry of Health & Welfare, Republic of Korea (grant number: HI22C073600, RS-2023-00262087 to T-JS). The funding source had no role in the design, conduct, or reporting of this study.
Conflict of interest
The authors declare that the research was conducted in the absence of any commercial or financial relationships that could be construed as a potential conflict of interest.
Publisher's note
All claims expressed in this article are solely those of the authors and do not necessarily represent those of their affiliated organizations, or those of the publisher, the editors and the reviewers. Any product that may be evaluated in this article, or claim that may be made by its manufacturer, is not guaranteed or endorsed by the publisher.
Supplementary material
The Supplementary Material for this article can be found online at: https://www.frontiersin.org/articles/10.3389/fcvm.2023.1159087/full#supplementary-material
References
1. Chugh SS, Havmoeller R, Narayanan K, Singh D, Rienstra M, Benjamin EJ, et al. Worldwide epidemiology of atrial fibrillation: a global burden of disease 2010 study. Circulation. (2014) 129(8):837–47. doi: 10.1161/CIRCULATIONAHA.113.005119
2. Go AS, Hylek EM, Phillips KA, Chang Y, Henault LE, Selby JV, et al. Prevalence of diagnosed atrial fibrillation in adults: national implications for rhythm management and stroke prevention: the AnTicoagulation and risk factors in atrial fibrillation (ATRIA) study. JAMA. (2001) 285(18):2370–5. doi: 10.1001/jama.285.18.2370
3. Guo Y, Tian Y, Wang H, Si Q, Wang Y, Lip GYH. Prevalence, incidence, and lifetime risk of atrial fibrillation in China: new insights into the global burden of atrial fibrillation. Chest. (2015) 147(1):109–19. doi: 10.1378/chest.14-0321
4. Lau DH, Nattel S, Kalman JM, Sanders P. Modifiable risk factors and atrial fibrillation. Circulation. (2017) 136(6):583–96. doi: 10.1161/CIRCULATIONAHA.116.023163
5. Song TJ, Kim YD, Yoo J, Kim J, Chang HJ, Hong GR, et al. Association between aortic atheroma and cerebral small vessel disease in patients with ischemic stroke. J Stroke. (2016) 18(3):312–20. doi: 10.5853/jos.2016.00171
6. Chang Y, Woo HG, Park J, Lee JS, Song TJ. Improved oral hygiene care is associated with decreased risk of occurrence for atrial fibrillation and heart failure: a nationwide population-based cohort study. Eur J Prev Cardiol. (2020) 27(17):1835–45. doi: 10.1177/2047487319886018
7. WHO. Obesity: preventing and managing the global epidemic. In: Report of a WHO consultation. World health organ tech rep ser. Geneva: World Health Organization (2000). p. 1–253.
8. Dai H, Alsalhe TA, Chalghaf N, Riccò M, Bragazzi NL, Wu J. The global burden of disease attributable to high body mass index in 195 countries and territories, 1990-2017: an analysis of the global burden of disease study. PLoS Med. 2020;17(7):e1003198. doi: 10.1371/journal.pmed.1003198
9. Ortega FB, Lavie CJ, Blair SN. Obesity and cardiovascular disease. Circ Res. (2016) 118(11):1752–70. doi: 10.1161/CIRCRESAHA.115.306883
10. Krauss RM, Winston M, Fletcher RN, Grundy SM. Obesity: impact of cardiovascular disease. Circulation. (1998) 98(14):1472–6. doi: 10.1161/01.CIR.98.14.1472
11. Ueno K, Kaneko H, Kamiya K, Itoh H, Okada A, Suzuki Y, et al. Relationship of normal-weight central obesity with the risk for heart failure and atrial fibrillation: analysis of a nationwide health check-up and claims database. Eur Heart J Open. (2022) 2(3):oeac026. doi: 10.1093/ehjopen/oeac026
12. Pouwels S, Topal B, Knook MT, Celik A, Sundbom M, Ribeiro R, et al. Interaction of obesity and atrial fibrillation: an overview of pathophysiology and clinical management. Expert Rev Cardiovasc Ther. (2019) 17(3):209–23. doi: 10.1080/14779072.2019.1581064
13. Lee H, Choi EK, Lee SH, Han KD, Rhee TM, Park CS, et al. Atrial fibrillation risk in metabolically healthy obesity: a nationwide population-based study. Int J Cardiol. (2017) 240:221–7. doi: 10.1016/j.ijcard.2017.03.103
14. Sandhu RK, Ezekowitz J, Andersson U, Alexander JH, Granger CB, Halvorsen S, et al. The ‘obesity paradox’ in atrial fibrillation: observations from the ARISTOTLE (apixaban for reduction in stroke and other thromboembolic events in atrial fibrillation) trial. Eur Heart J. (2016) 37(38):2869–78. doi: 10.1093/eurheartj/ehw124
15. Wang J, Yang YM, Zhu J, Zhang H, Shao XH, Tian L, et al. Overweight is associated with improved survival and outcomes in patients with atrial fibrillation. Clin Res Cardiol. (2014) 103(7):533–42. doi: 10.1007/s00392-014-0681-7
16. Lee G, Chang J, Hwang S-S, Son JS, Park SM. Development and validation of prediction equations for the assessment of muscle or fat mass using anthropometric measurements, serum creatinine level, and lifestyle factors among Korean adults. NRP. (2020) 15(1):95–105. doi: 10.4162/nrp.2021.15.1.95
17. Tikkanen E, Gustafsson S, Knowles JW, Perez M, Burgess S, Ingelsson E. Body composition and atrial fibrillation: a Mendelian randomization study. Eur Heart J. (2019) 40(16):1277–82. doi: 10.1093/eurheartj/ehz003
18. Worm MS, Bager CL, Blair JPM, Secher NH, Riis BJ, Christiansen C, et al. Atrial fibrillation is associated with lean body mass in postmenopausal women. Sci Rep. (2020) 10(1):573. doi: 10.1038/s41598-019-57167-3
19. Chang Y, Lee JS, Woo HG, Ryu DR, Kim JW, Song TJ. Improved oral hygiene care and chronic kidney disease occurrence: a nationwide population-based retrospective cohort study. Medicine (Baltimore). (2021) 100(47):e27845. doi: 10.1097/MD.0000000000027845
20. Kim J, Kim HJ, Jeon J, Song TJ. Association between oral health and cardiovascular outcomes in patients with hypertension: a nationwide cohort study. J Hypertens. (2022) 40(2):374–81. doi: 10.1097/HJH.0000000000003022
21. Lee K, Lee JS, Kim J, Lee H, Chang Y, Woo HG, et al. Oral health and gastrointestinal cancer: a nationwide cohort study. J Clin Periodontol. (2020) 47(7):796–808. doi: 10.1111/jcpe.13304
22. Kim HJ, Park MS, Shin JI, Park J, Kim DH, Jeon J, et al. Associations of heart failure with susceptibility and severe complications of COVID-19: a nationwide cohort study. J Med Virol. (2022) 94(3):1138–45. doi: 10.4162/nrp.2021.15.1.95
23. Sundararajan V, Henderson T, Perry C, Muggivan A, Quan H, Ghali WA. New ICD-10 version of the charlson comorbidity index predicted in-hospital mortality. J Clin Epidemiol. (2004) 57(12):1288–94. doi: 10.1016/j.jclinepi.2004.03.012
24. Chen LK, Woo J, Assantachai P, Auyeung TW, Chou MY, Iijima K, et al. Asian working group for sarcopenia: 2019 consensus update on sarcopenia diagnosis and treatment. J Am Med Dir Assoc. (2020) 21(3):300–7.e2. doi: 10.1016/j.jamda.2019.12.012
25. Cruz-Jentoft AJ, Baeyens JP, Bauer JM, Boirie Y, Cederholm T, Landi F, et al. Sarcopenia: european consensus on definition and diagnosis: report of the European working group on sarcopenia in older people. Age Ageing. (2010) 39(4):412–23. doi: 10.1093/ageing/afq034
26. Anaszewicz M, Banas W, Wawrzenczyk A, Budzynski J. Body composition in patients with atrial fibrillation. Acta Cardiol Sin. (2019) 35(5):484–92. doi: 10.6515/ACS.201909_35(5).20190323A
27. Heshmat R, Shafiee G, Ostovar A, Fahimfar N, Maleki Birjandi S, Jabbari M, et al. Relationship between sarcopenia and electrocardiographic abnormalities in older people: the bushehr elderly health program. Front Med (Lausanne. (2021) 8:656181. doi: 10.3389/fmed.2021.656181
28. Xia MF, Chen LY, Wu L, Ma H, Li XM, Li Q, et al. Sarcopenia, sarcopenic overweight/obesity and risk of cardiovascular disease and cardiac arrhythmia: a cross-sectional study. Clin Nutr. (2021) 40(2):571–80. doi: 10.1016/j.clnu.2020.06.003
29. Bella JN, Devereux RB, Roman MJ, O'Grady MJ, Welty TK, Lee ET, et al. Relations of left ventricular mass to fat-free and adipose body mass: the strong heart study. The strong heart study investigators. Circulation. (1998) 98(23):2538–44. doi: 10.1161/01.CIR.98.23.2538
30. Wang TJ, Parise H, Levy D, D’Agostino RB, Wolf PA, Vasan RS, et al. Obesity and the risk of new-onset atrial fibrillation. JAMA. (2004) 292(20):2471–7. doi: 10.1001/jama.292.20.2471
31. Fenger-Grøn M, Overvad K, Tjønneland A, Frost L. Lean body mass is the predominant anthropometric risk factor for atrial fibrillation. J Am Coll Cardiol. (2017) 69(20):2488–97. doi: 10.1016/j.jacc.2017.03.558
32. Frost L, Benjamin EJ, Fenger-Gron M, Pedersen A, Tjonneland A, Overvad K. Body fat, body fat distribution, lean body mass and atrial fibrillation and flutter. A danish cohort study. Obesity (Silver Spring, Md). (2014) 22(6):1546–52. doi: 10.1002/oby.20706
33. Lee S-R, Han K-D, Choi E-K, Oh S, Lip GYH. Nonalcoholic fatty liver disease and the risk of atrial fibrillation stratified by body mass index: a nationwide population-based study. Sci Rep. (2021) 11(1):3737. doi: 10.1038/s41598-021-83367-x
34. Alon L, Corica B, Raparelli V, Cangemi R, Basili S, Proietti M, et al. Risk of cardiovascular events in patients with non-alcoholic fatty liver disease: a systematic review and meta-analysis. Eur J Prev Cardiol. (2022) 29(6):938–46. doi: 10.1093/eurjpc/zwab212
35. Goudis CA, Korantzopoulos P, Ntalas IV, Kallergis EM, Ketikoglou DG. Obesity and atrial fibrillation: a comprehensive review of the pathophysiological mechanisms and links. J Cardiol. (2015) 66(5):361–9. doi: 10.1016/j.jjcc.2015.04.002
36. Aune D, Sen A, Norat T, Janszky I, Romundstad P, Tonstad S, et al. Body mass index, abdominal fatness, and heart failure incidence and mortality: a systematic review and dose-response meta-analysis of prospective studies. Circulation. (2016) 133(7):639–49. doi: 10.1161/CIRCULATIONAHA.115.016801
37. Fenger-Grøn M, Vinter N, Frost L. Body mass and atrial fibrillation risk: status of the epidemiology concerning the influence of fat versus lean body mass. Trends Cardiovasc Med. (2020) 30(4):205–11. doi: 10.1016/j.tcm.2019.05.009
38. Lee DH, Keum N, Hu FB, Orav EJ, Rimm EB, Willett WC, et al. Predicted lean body mass, fat mass, and all cause and cause specific mortality in men: prospective US cohort study. BMJ (Clinical Research ed). (2018) 362:k2575. doi: 10.1136/bmj.k2575
39. Kang SH, Choi EK, Han KD, Lee SR, Lim WH, Cha MJ, et al. Underweight is a risk factor for atrial fibrillation: a nationwide population-based study. Int J Cardiol. (2016) 215:449–56. doi: 10.1016/j.ijcard.2016.04.036
40. Rosenberg MA, Das S, Quintero Pinzon P, Knight AC, Sosnovik DE, Ellinor PT, et al. A novel transgenic mouse model of cardiac hypertrophy and atrial fibrillation. J Atr Fibrillation. (2012) 4(5):415. doi: 10.4022/jafib.415
Keywords: body composition, body mass index, atrial fibrillation, big data, analysis
Citation: Woo HG, Kang MK and Song T-J (2023) Association of predicted body composition with occurrence of atrial fibrillation. Front. Cardiovasc. Med. 10:1159087. doi: 10.3389/fcvm.2023.1159087
Received: 5 February 2023; Accepted: 26 September 2023;
Published: 10 October 2023.
Edited by:
Basil Nwaneri Okeahialam, University of Jos, NigeriaReviewed by:
Bartosz Krzowski, Medical University of Warsaw, PolandKwonduk Seo, National Health Insurance Service Ilsan Hospital, Republic of Korea
© 2023 Woo, Kang and Song. This is an open-access article distributed under the terms of the Creative Commons Attribution License (CC BY). The use, distribution or reproduction in other forums is permitted, provided the original author(s) and the copyright owner(s) are credited and that the original publication in this journal is cited, in accordance with accepted academic practice. No use, distribution or reproduction is permitted which does not comply with these terms.
*Correspondence: Tae-Jin Song knstar@ewha.ac.kr
†These authors have contributed equally to this work
‡ORCID Tae-Jin Song orcid.org/0000-0002-9937-762X