- 1Department of Anesthesiology, The Second Affiliated Hospital of Zhejiang University School of Medicine, Hangzhou, China
- 2Department of Anesthesiology, West China Hospital of Sichuan University, Chengdu, China
- 3Department of Cardiovascular Surgery of West China Hospital, Sichuan University, Chengdu, China
Background: On-pump valve surgeries are associated with high morbidity and mortality. The present study aimed to reliably predict a composite outcome of postoperative complications using a minimum of easily accessible clinical parameters.
Methods: A total of 7,441 patients who underwent valve surgery were retrospectively analyzed. Data for 6,220 patients at West China Hospital of Sichuan University were used to develop a predictive model, which was validated using data from 1,221 patients at the Second Affiliated Hospital of Zhejiang University School of Medicine. The primary outcome was a composite of major complications: all-cause death in hospital, stroke, myocardial infarction, and severe acute kidney injury. The predictive model was constructed using the least absolute shrinkage and selection operator as well as multivariable logistic regression. The model was assessed in terms of the areas under receiver operating characteristic curves, calibration, and decision curve analysis.
Results: The primary outcome occurred in 129 patients (2.1%) in the development cohort and 71 (5.8%) in the validation cohort. Six variables were retained in the predictive model: New York Heart Association class, diabetes, glucose, blood urea nitrogen, operation time, and red blood cell transfusion during surgery. The C-statistics were 0.735 (95% CI, 0.686–0.784) in the development cohort and 0.761 (95% CI, 0.694–0.828) in the validation cohort. For both cohorts, calibration plots showed good agreement between predicted and actual observations, and ecision curve analysis showed clinical usefulness. In contrast, the well-established SinoSCORE did not accurately predict the primary outcome in either cohort.
Conclusions: This predictive nomogram based on six easily accessible variables may serve as an “early warning” system to identify patients at high risk of major complications after valve surgery.
Clinical Trial Registration: [www.ClinicalTrials.gov], identifier [NCT04476134].
Introduction
Annually, approximately 275 000 heart valve surgeries involving cardiopulmonary bypass (CPB) are carried out worldwide to treat symptomatic valve disease (1), and more than 20 000 of those surgeries end with patient morbidity or mortality (2, 3). The rate of mortality after cardiac surgery varies from 1.0% to 8.6% (2), and rates are also high for stroke (2.2%), myocardial infarction (1.5%) and severe acute kidney injury (1.3%) (4–6). Early identification of patients who are at risk of major complications may improve their management and prognosis after valve surgery.
Several models have been constructed to predict the risk of complications after cardiac surgery (7–13). Most of them (9–11), such as the Sino System for Coronary Operative Risk Evaluation (SinoSCORE), were developed to predict a specific adverse event after surgery based on coronary artery bypass grafting (CABG). Classical models, such as the scoring systems of the Society of Thoracic Surgeons (STS) and the European System for Cardiac Operative Risk Evaluation II (EuroSCORE II), were developed from data from patients in the West and involve many predictors, making them difficult to apply in the clinic (7, 8, 13).
The present study aimed to develop a predictive model that was based on Asian patients and that involved a relatively small number of easily accessible clinical parameters. The model exploits the fact that the various adverse events after valve surgery share similar risk factors, such as diabetes, New York Heart Association (NYHA) class, use of CPB, operation time and need for transfusion (14–16). This overlap in risk factors probably reflects that many of these events share the same causes of poor organ perfusion and systemic inflammation induced by CPB (17–19). Therefore, when developing the model, we designed it to predict not one specific adverse event but a composite outcome of major complications.
Materials and methods
This study was approved by the Ethics Committees of the Second Affiliated Hospital of Zhejiang University School of Medicine in Hangzhou (096/2017) and West China Hospital of Sichuan University in Chengdu (256/2017). The requirement for informed consent was waived due to the retrospective nature of this study. The present study took its data from a previously described dataset that we have previously analyzed for other purposes (16, 20, 21).
Participants
To be enrolled in the present study, patients in our database had been at least 18 years old, scheduled for valve surgery under CPB with or without CABG from January 1, 2011 to June 30, 2017 at West China Hospital, or from September 1, 2013 to June 30, 2017 at the Second Affiliated Hospital of Zhejiang University School of Medicine. Patients were excluded if they (1) underwent emergency surgery, (2) died in the operation room, (3) could not be weaned off CPB, or (4) underwent combined surgery involving aortic replacement or tumor resection. Two investigators who were blind to the study hypothesis used a predefined form to collect relevant data from the database.
Endpoint
The primary outcome was a composite of the following major complications: (1) all-cause mortality (22), (2) myocardial infarction (22), (3) stroke (23), and (4) severe acute kidney injury (24). All major complications were defined as those occurring new after surgery and during hospitalization. Detailed definitions are provided in Supplementary Table S1.
Data collection
Data were extracted from the database relevant to variables that we considered potential predictors of the composite primary outcome, based on general clinical practice, transfusion-related adverse outcomes after cardiac surgery (21, 25), risk factors of acute kidney injury (16) and other research (8, 9, 26). Variables included age, sex, and ethnicity; body max index (BMI), current smoking, current alcohol consumption, comorbidities and medical history; American Society of Anesthesiologists (ASA) physical status; NYHA classification; pre-operative medication and laboratory findings; type of surgery and intra-operative data. More details are provided in Supplementary Table S1.
Potential predictors were excluded if data for them were missing for >10% of patients. When data were missing for no more than 10% of patients, multiple imputation based on five replications was performed.
Statistical analysis
Data were analyzed using R 4.1.0 (https://www.r-project.org). Continuous data were tested for normality and presented as mean (SD) if normally distributed, or as median (IQR) if skewed. Differences between the development and validation groups were assessed for significance using the Mann–Whitney U test, Student's t test, or Welch's t test as appropriate. Categorical data were reported as number (proportion), and intergroup differences were assessed using Fisher's exact test or the chi-squared test.
To reduce the risk of over-fitting in the predictive model, potential predictors were selected using least absolute shrinkage and selection operator (LASSO) regression. Ten-fold cross-validation was used to determine the penalty parameter (λ), which was optimized based on the criterion of one standard error away from the minimum binomial deviance. Variables with non-zero coefficients were entered into multivariate logistic regression, the results of which were expressed as odds ratios (ORs) and 95% CIs.
Based on the weight of each predictor in the model, a nomogram was drawn. The performance of the model was assessed as recommended (27) in terms of the the areas under receiver operating characteristic curves (AUC) to discriminate patients who did or did not experience the composite primary outcome, the calibration curve and accompanying Brier score, and the net benefit as determined using decision curve analysis. AUC was corrected using bootstrapping (1,000 replications). These performance assessments were carried out for the development cohort from West China Hospital and for the validation cohort from the Second Affiliated Hospital of Zhejiang University School of Medicine. To benchmark our model's performance, we compared it to the performance of SinoSCORE against both cohorts.
Results
A total of 7,696 patients who underwent valve surgery at the two study sites were screened for enrollment, of whom 255 patients were excluded because they underwent emergency surgery (10), died during the operation (19), could not be weaned off CPB (4), or underwent a combination of surgical procedures also involving ascending aortic replacement or tumor resection (222). More details are shown in Supplementary Figure S1.
In the end, 7,441 patients were included in the final analysis, comprising 6,220 in the development cohort and 1,221 in the validation cohort. The two groups were comparable in most baseline and intraoperative parameters (Table 1, Supplementary Table S2). Compared to the validation group, patients in the development group were significantly older (50 ± 11 vs. 56 ± 11 years), more likely to be women (55.5% vs. 50.5%), more likely to undergo multi-valve surgery (55.2% vs. 41.8%), and less likely to undergo a combination of valve surgery and CABG (1.1% vs. 6.6%).
Across all patients at both study sites, the composite of major complications occurred in 200 (2.7%), comprising 86 deaths (1.2%), 9 cases of myocardial infarction (0.1%), 27 cases of stroke (0.4%), and 125 cases of severe acute kidney injury (1.7%; Supplementary Table S3). The development cohort showed significantly lower rates of the composite outcome (129/6,220, 2.1% vs. 71/1,221, 5.8%; P < 0.001) as well as significantly lower rates of stroke (0.3% vs. 1.1%, P < 0.01) and severe acute kidney injury (0.7% vs. 4.5%, P < 0.01). In contrast, rates of mortality and myocardial infarction did not differ significantly between the two cohorts.
Model development and assessment
LASSO analysis of 60 variables generated two λ parameters, one corresponding to the minimum binomial deviance and the other corresponding to one standard error away from that deviance. We chose the latter λ value because it imposed a stricter penalty and could therefore reduce the number of covariates more than the former value. Indeed, increasing λ to 0.008, corresponding to one standard error away from the minimum λ, led to only six candidate predictors in the logistic model (Figures 1A,B): NYHA class (I, II, III or IV), diabetes (yes or no), blood glucose, blood urea nitrogen (BUN), red blood cell (RBC) transfusion and operation time (Table 2).
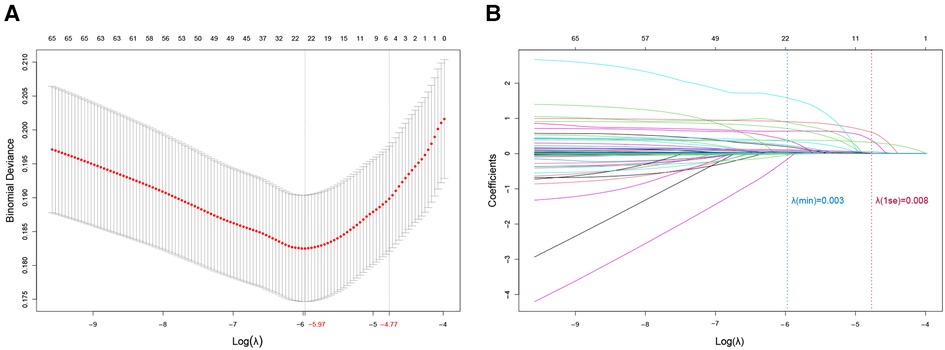
Figure 1. Predictor selection using least absolute shrinkage and selection operator (LASSO) regression. (A) Optimization of the penalty tuning parameter λ using 10-fold cross-validation with the minimum criterion. The number of predictors is indicated at the top of the plot. Dotted vertical lines indicate the minimum criterion and one standard error away from it. An optimal value of log (λ) = −4.82 was selected. (B) Coefficient profile plot of the predictors. The number of predictors is indicated at the top of the plot. The ordinate is the coefficient value. Each curve in the figure represents the trajectory of the change of the coefficient of each independent variable, and the coefficient of variable is eventually compressed to 0 as the λ parameter increases. Dotted vertical lines were plotted at ideal values, based on the same criteria as in (A). Six predictors with non-zero coefficients were selected.
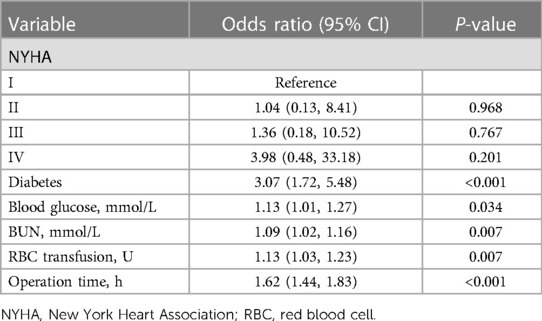
Table 2. Assessment of potential predictors of the composite outcome after valve surgery based on LASSO regression.
A nomogram was generated based on the model, in which each predictor was scored and the individual scores were summed to obtain the overall probability of the primary outcome (Figure 2A). For example, one patient was diabetic (15 points) and belonged to NYHA class III (4 points). He or she had preoperative levels of blood glucose of 8 mmol/L (10 points) and BUN of 5 mmol/L (6 points). Surgery lasted 4 h (25 points), during which he or she received 5 units of RBC (8 points). The total score for this patient was 68 points, corresponding to 7% composite risk of major complications.
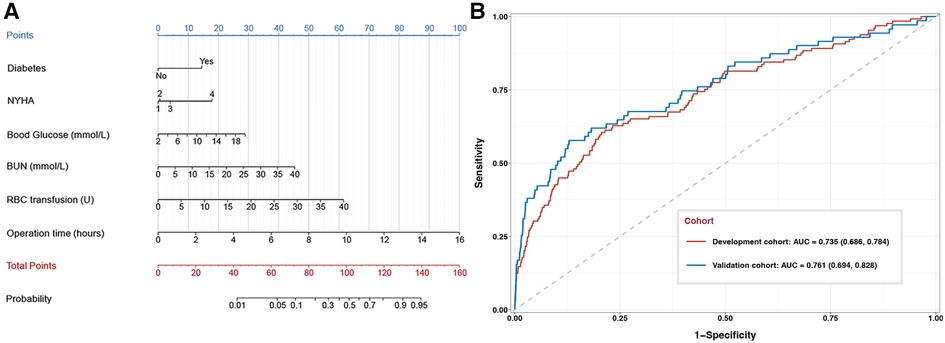
Figure 2. Development and performance assessment of a nomogram to predict composite major complications after valve surgery. (A) The nomogram. NYHA, New York Heart Association; BUN, blood urea nitrogen; RBC, red blood cell. (B) Receiver operating characteristic curves for the nomogram against the development cohort (red) or validation cohort (blue). The area under the curve (AUC) together with the 95% CI: 0.735 (95% CI, 0.686–0.784) in the development cohort and 0.761 (95% CI, 0.694–0.828) in the validation cohort.
The nomogram discriminated between patients who experienced or not the primary outcome with an AUC of 0.735 (95% CI, 0.686–0.784) in the development cohort and 0.761 (95% CI, 0.694–0.828) in the validation cohort (Figure 2B). When patients were stratified as low- or high-risk based on the optimal cut-off nomogram score of 52.46 points, incidence of the primary outcome was confirmed to be significantly different between low- and high-risk patients, both in the development cohort (1.0% vs. 5.8%) and validation cohort (2.9% vs. 15.8%).
Calibration curves showed good agreement between observed results and predicted results for the development cohort (Figure 3A) and validation cohort (Figure 3B). The corresponding Brier scores were 0.02 and 0.05, where the ideal score is 0. Decision curve analysis suggested acceptable performance for both the development cohort (Figure 4A) as well as the validation cohort (Figure 4B), although the model performed better for the development cohort.
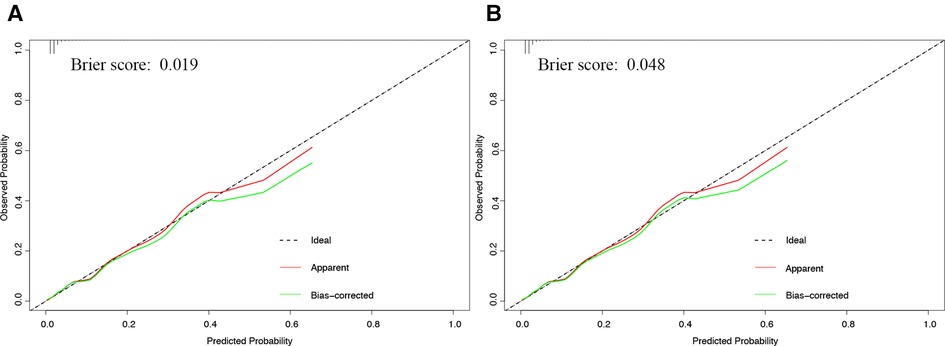
Figure 3. Calibration curve of development and validation cohorts. Calibration curves showing agreement between the nomogram's predicted probability of a composite of major complications and actual probability of the composite in the (A) development or (B) validation cohort. The dashed diagonal line in the figure indicates when predicted and observed probabilities match perfectly. The red and green fitted curves were apparent and bias-corrected calibration curves respectively. The corresponding Brier scores are shown at the upper left of each plot.
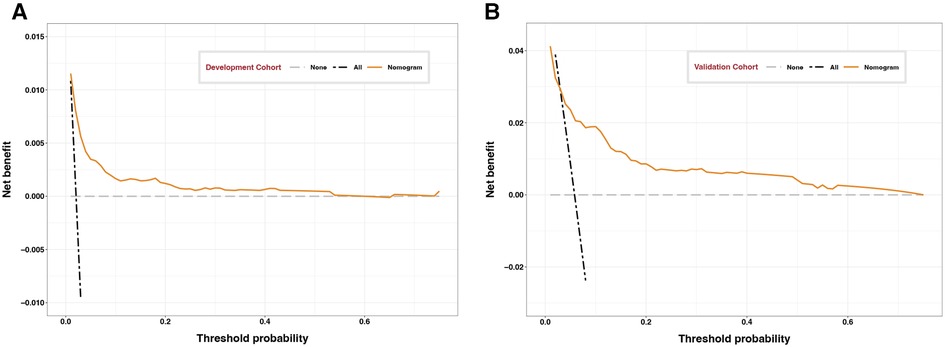
Figure 4. Decision curves of development and validation cohorts. Decision curve analysis assessing the clinical utility of the nomogram for the (A) development or (B) validation cohort as a function of probability thresholds. Dotted lines represent the states when no patient (black) or all patients (gray) experience the composite outcome of major complications. Clinical benefit is indicated when the orange line lies to the right of the black dotted line and above the gray dotted line.
Benchmarking of the model against SinoSCORE
SinoSCORE performed worse than our nomogram at predicting the primary outcome, giving AUC 0.597 (95% CI, 0.563–0.631) across all patients, 0.545 (95% CI, 0.508–0.582) in the development cohort and 0.654 (95% CI, 0.591–0.716) in the validation cohort (Supplementary Figure S3). SinoSCORE overestimated the composite risk of major complications in our sample: it predicted rates of 15.8% across all patients, 13.3% in the development cohort and 28.7% in the validation cohort, compared to the corresponding actual rates of 2.7%, 2.1% and 5.8%.
Discussion
Severe complications, including myocardial infarction, stroke, and severe acute kidney injury strongly compromise prognosis after cardiac surgery (5, 28–30). Using data from more than 7,000 patients at two large tertiary hospitals in China, the present study established a simple risk model based on six easily accessible variables in order to predict composite risk of major complications after valve surgery. To our knowledge, this is the first model that can predict composite risk of major complications after valve surgery. The model may help improve risk screening and management, ultimately leading to better prognosis.
Our model is much simpler than EuroSCORE II (8) or SinoSCORE (9): it includes only four preoperative variables (diabetes, NYHA class, blood glucose and BUN) and two intraoperative variables (intraoperative RBC transfusion and operation time). With only six variables, our nomogram is easier to use. In addition, the predictive variables were all routine test indicators in clinical practice, so information collection would not bring additional burden as time and resources can be saved. It can quickly help to optimize resources and implement prophylactic strategies. The nomogram model is able to identify patients at risk for postoperative major complications immediately after surgery. It may provide “early warning” to medical staff. Patients who are deemed at high risk based on our model may require more careful attention to preoperative blood glucose level and renal function, and they may benefit from individualized perioperative blood management (31) as well as efforts to shorten surgery time as much as possible.
All the variables in our model, except NYHA class, differ from those in the SinoSCORE (9), but are consistent with other reported risk factors of cardiac surgery (16, 32, 33). As reported previously (34), we found that SinoSCORE overestimated the risk of major complications even in patients underwent CABG. This relatively poor performance may reflect that SinoSCORE was designed a decade ago based on data from Chinese patients who underwent CABG. We suggest that SinoSCORE may no longer be suitable for predicting risk of major complications as a result of advances in the surgical treatment and management of cardiac patients.
Surgery type did not substantially influence risk of primary outcome in our sample: it did not survive variable selection in LASSO regression, nor did it substantially alter risk after we manually added it into the regression model along with the six final predictors (Supplementary Table S3). This negative result may reflect that only 70 patients (1.1%) in the development cohort underwent valve replacement with CABG. It may also reflect that patients after most types of complex surgery are generally monitored closely throughout the perioperative period, which may help prevent postoperative complications. Further research with larger samples undergoing different types of cardiac surgery should explore the potential influence of type of surgery on risk of major complications.
Our findings should be interpreted with caution in light of several limitations. The retrospective design meant that we lacked sufficient data to consider some potentially relevant variables, such as preoperative angina or affected mobility (8). Indeed, we benchmarked our model only against SinoSCORE because our database lacked information needed to determine several parameters that appear in EuroSCORE II and other previously published models. Second, there are different incidences of composite outcome in derivation and validation cohorts (2.1% vs. 5.8%), which maybe partly due to different clinical practices and baseline characteristics especially preoperative status. Fortunately, our model shows good discrimination and calibration in both two cohorts. Third, we validated our model with only one external cohort. Given the complexity of cardiac surgery and the therefore large number of perioperative parameters that may differ across medical centers, our results need to be verified and extended through research at additional sites.
Conclusions
We present a nomogram that may reliably identify patients at high composite risk of major complications after valve surgery. This tool may improve the monitoring and management of this vulnerable patient population.
Data availability statement
The original contributions presented in the study are included in the article/Supplementary Material, further inquiries can be directed to the corresponding author.
Ethics statement
This study was approved by the Ethics Committees of the Second Affiliated Hospital of Zhejiang University School of Medicine in Hangzhou (096/2017) and West China Hospital of Sichuan University in Chengdu (256/2017).
Author contributions
Conception and design: ZZC, YSW, LD, MY. Data collection: YM, JL, ZW, YQG. Data analysis and interpretation: ZZC, YSW, YYY. Manuscript writing: all authors. All authors contributed to the article and approved the submitted version.
Funding
This study was supported by the 1.3.5 Project for Disciplines of Excellence at West China Hospital of Sichuan University (2017-120) and by the Science Foundation of Sichuan Province (2022NSFSC1411); the National Clinical Key Specialty Construction Project of China 2021 (2021-LCZDZK-01); No competing interests declared.
Conflict of interest
The authors declare that the research was conducted in the absence of any commercial or financial relationships that could be construed as a potential conflict of interest.
Publisher's note
All claims expressed in this article are solely those of the authors and do not necessarily represent those of their affiliated organizations, or those of the publisher, the editors and the reviewers. Any product that may be evaluated in this article, or claim that may be made by its manufacturer, is not guaranteed or endorsed by the publisher.
Supplementary material
The Supplementary Material for this article can be found online at: https://www.frontiersin.org/articles/10.3389/fcvm.2023.1132428/full#supplementary-material.
References
1. Rabkin E, Schoen FJ. Cardiovascular tissue engineering. Cardiovasc Pathol. (2002) 11(6):305–17. doi: 10.1016/s1054-8807(02)00130-8
2. Bowdish ME, D'Agostino RS, Thourani VH, Desai N, Shahian DM, Fernandez FG, et al. The society of thoracic surgeons adult cardiac surgery database: 2020 update on outcomes and research. Ann Thorac Surg. (2020) 109(6):1646–55. doi: 10.1016/j.athoracsur.2020.03.003
3. Movahed MR, Etemad S, Hashemzadeh M, Hashemzadeh M. Persistent reduction in the age adjusted mortality rate from aortic valve surgery in the United State with elimination of gender gap in recent years. Am J Cardiovasc Dis. (2020) 10(4):522–7. PMID: 33224604.33224604
4. Sultan I, Bianco V, Kilic A, Jovin T, Jadhav A, Jankowitz B, et al. Predictors and outcomes of ischemic stroke after cardiac surgery. Ann Thorac Surg. (2020) 110(2):448–56. doi: 10.1016/j.athoracsur.2020.02.025
5. Sprung J, Abdelmalak B, Gottlieb A, Mayhew C, Hammel J, Levy PJ, et al. Analysis of risk factors for myocardial infarction and cardiac mortality after major vascular surgery. Anesthesiology. (2000) 93(1):129–40. doi: 10.1097/00000542-200007000-00023
6. Wang C, Gao Y, Tian Y, Wang Y, Zhao W, Sessler DI, et al. Prediction of acute kidney injury after cardiac surgery from preoperative N-terminal pro-B-type natriuretic peptide. Br J Anaesth. (2021) 127(6):862–70. doi: 10.1016/j.bja.2021.08.015
7. O'Brien SM, Shahian DM, Filardo G, Ferraris VA, Haan CK, Rich JB, et al. The society of thoracic surgeons 2008 cardiac surgery risk models: part 2–isolated valve surgery. Ann Thorac Surg. (2009) 88(1 Suppl):S23–42. doi: 10.1016/j.athoracsur.2009.05.056
8. Nashef SA, Roques F, Sharples LD, Nilsson J, Smith C, Goldstone AR, et al. EuroSCORE II. Eur J Cardiothorac Surg. (2012) 41(4):734–44; discussion 744–35. doi: 10.1093/ejcts/ezs043
9. Zheng Z, Zhang L, Li X, Hu S, Chinese CRS. SinoSCORE: a logistically derived additive prediction model for post-coronary artery bypass grafting in-hospital mortality in a Chinese population. Front Med. (2013) 7(4):477–85. doi: 10.1007/s11684-013-0284-0
10. Shahian DM, O'Brien SM, Filardo G, Ferraris VA, Haan CK, Rich JB, et al. The society of thoracic surgeons 2008 cardiac surgery risk models: part 1–coronary artery bypass grafting surgery. Ann Thorac Surg. (2009) 88(1 Suppl):S2–22. doi: 10.1016/j.athoracsur.2009.05.053
11. Hu Z, Chen S, Du J, Gu D, Wang Y, Hu S, et al. An in-hospital mortality risk model for patients undergoing coronary artery bypass grafting in China. Ann Thorac Surg. (2020) 109(4):1234–42. doi: 10.1016/j.athoracsur.2019.08.020
12. Wessler BS, Lundquist CM, Koethe B, Park JG, Brown K, Williamson T, et al. Clinical prediction models for valvular heart disease. J Am Heart Assoc. (2019) 8(20):e011972. doi: 10.1161/JAHA.119.011972
13. Shahian DM, O'Brien SM, Filardo G, Ferraris VA, Haan CK, Rich JB, et al. The society of thoracic surgeons 2008 cardiac surgery risk models: part 3–valve plus coronary artery bypass grafting surgery. Ann Thorac Surg. (2009) 88(1 Suppl):S43–62. doi: 10.1016/j.athoracsur.2009.05.055
14. Bucerius J, Gummert JF, Borger MA, Walther T, Doll N, Onnasch JF, et al. Stroke after cardiac surgery: a risk factor analysis of 16,184 consecutive adult patients. Ann Thorac Surg. (2003) 75(2):472–8. doi: 10.1016/s0003-4975(02)04370-9
15. Farzaneh-Far A, Phillips HR, Shaw LK, Starr AZ, Fiuzat M, O'Connor CM, et al. Ischemia change in stable coronary artery disease is an independent predictor of death and myocardial infarction. JACC Cardiovasc Imaging. (2012) 5(7):715–24. doi: 10.1016/j.jcmg.2012.01.019
16. Wang YS, Chen DX, Yan M, Wu Z, Guo YQ, Li Q, et al. Prediction of the severity of acute kidney injury after on-pump cardiac surgery. J Clin Anesth. (2022) 78:110664. doi: 10.1016/j.jclinane.2022.110664
17. Kraft F, Schmidt C, Van Aken H, Zarbock A. Inflammatory response and extracorporeal circulation. Best Pract Res Clin Anaesthesiol. (2015) 29(2):113–23. doi: 10.1016/j.bpa.2015.03.001
18. McKhann GM, Grega MA, Borowicz LM Jr, Baumgartner WA, Selnes OA. Stroke and encephalopathy after cardiac surgery: an update. Stroke. (2006) 37(2):562–71. doi: 10.1161/01.STR.0000199032.78782.6c
19. Hausenloy DJ, Yellon DM. Ischaemic conditioning and reperfusion injury. Nat Rev Cardiol. (2016) 13(4):193–209. doi: 10.1038/nrcardio.2016.5
20. Heafner T, Bews K, Kalra M, Oderich G, Colglazier J, Shuja F, et al. Transfusion timing and postoperative myocardial infarction and death in patients undergoing common vascular procedures. Ann Vasc Surg. (2020) 63:53–62. doi: 10.1016/j.avsg.2019.08.090
21. Zhou L, Liu X, Yan M, Zhao W, Luo D, Liu J, et al. Postoperative nadir hemoglobin and adverse outcomes in patients undergoing on-pump cardiac operation. Ann Thorac Surg. (2021) 112(3):708–16. doi: 10.1016/j.athoracsur.2021.01.016
22. Hogervorst E, Rosseel P, van der Bom J, Bentala M, Brand A, van der Meer N, et al. Tolerance of intraoperative hemoglobin decrease during cardiac surgery. Transfusion. (2014) 54(10 Pt 2):2696–704. doi: 10.1111/trf.12654
23. Murphy GJ, Pike K, Rogers CA, Wordsworth S, Stokes EA, Angelini GD, et al. Liberal or restrictive transfusion after cardiac surgery. N Engl J Med. (2015) 372(11):997–1008. doi: 10.1056/NEJMoa1403612
24. Schneider A, Ostermann M. The AKI glossary. Intensive Care Med. (2017) 43(6):893–7. doi: 10.1007/s00134-017-4751-y
25. Ming Y, Liu J, Zhang F, Chen C, Zhou L, Du L, et al. Transfusion of red blood cells, fresh frozen plasma, or platelets is associated with mortality and infection after cardiac surgery in a dose-dependent manner. Anesth Analg. (2020) 130(2):488–97. doi: 10.1213/ANE.0000000000004528
26. Shahian DM, Jacobs JP, Badhwar V, Kurlansky PA, Furnary AP, Cleveland JC Jr, et al. The society of thoracic surgeons 2018 adult cardiac surgery risk models: part 1-background, design considerations, and model development. Ann Thorac Surg. (2018) 105(5):1411–8. doi: 10.1016/j.athoracsur.2018.03.002
27. Alba AC, Agoritsas T, Walsh M, Hanna S, Iorio A, Devereaux PJ, et al. Discrimination and calibration of clinical prediction models: users’ guides to the medical literature. J Am Med Assoc. (2017) 318(14):1377–84. doi: 10.1001/jama.2017.12126
28. Hobson CE, Yavas S, Segal MS, Schold JD, Tribble CG, Layon AJ, et al. Acute kidney injury is associated with increased long-term mortality after cardiothoracic surgery. Circulation. (2009) 119(18):2444–53. doi: 10.1161/CIRCULATIONAHA.108.800011
29. Goldfarb M, Drudi L, Almohammadi M, Langlois Y, Noiseux N, Perrault L, et al. Outcome reporting in cardiac surgery trials: systematic review and critical appraisal. J Am Heart Assoc. (2015) 4(8):e002204. doi: 10.1161/JAHA.115.002204
30. Gaudino M, Rahouma M, Di Mauro M, Yanagawa B, Abouarab A, Demetres M, et al. Early versus delayed stroke after cardiac surgery: a systematic review and meta-analysis. J Am Heart Assoc. (2019) 8(13):e012447. doi: 10.1161/JAHA.119.012447
31. Desai N, Schofield N, Richards T. Perioperative patient blood management to improve outcomes. Anesth Analg. (2018) 127(5):1211–20. doi: 10.1213/ANE.0000000000002549
32. Li S, Tang BY, Zhang B, Wang CP, Zhang WB, Yang S, et al. Analysis of risk factors and establishment of a risk prediction model for cardiothoracic surgical intensive care unit readmission after heart valve surgery in China: a single-center study. Heart Lung. (2019) 48(1):61–8. doi: 10.1016/j.hrtlng.2018.07.013
33. Head SJ, Osnabrugge RL, Howell NJ, Freemantle N, Bridgewater B, Pagano D, et al. A systematic review of risk prediction in adult cardiac surgery: considerations for future model development. Eur J Cardiothorac Surg. (2013) 43(5):e121–129. doi: 10.1093/ejcts/ezt044
Keywords: prediction model, composite major complications, all-cause mortality, stroke, myocardial infarction, severe acute kidney injury
Citation: Cheng Z, Wang Y, Liu J, Ming Y, Yao Y, Wu Z, Guo Y, Du L and Yan M (2023) A novel model for predicting a composite outcome of major complications after valve surgery. Front. Cardiovasc. Med. 10:1132428. doi: 10.3389/fcvm.2023.1132428
Received: 27 December 2022; Accepted: 28 April 2023;
Published: 17 May 2023.
Edited by:
Giuseppe Gatti, Azienda Sanitaria Universitaria Giuliano Isontina, ItalyReviewed by:
Arrigo Francesco Giuseppe Cicero, University of Bologna, ItalyFabrizio D'Ascenzo, University of Turin, Italy
© 2023 Cheng, Wang, Liu, Ming, Yao, Guo, Wu, Du and Yan. This is an open-access article distributed under the terms of the Creative Commons Attribution License (CC BY). The use, distribution or reproduction in other forums is permitted, provided the original author(s) and the copyright owner(s) are credited and that the original publication in this journal is cited, in accordance with accepted academic practice. No use, distribution or reproduction is permitted which does not comply with these terms.
*Correspondence: Min Yan enJ5YW5taW5Aemp1LmVkdS5jbg==