- 1Department of General Practice, Huadu District People's Hospital, Southern Medical University, Guangzhou, China
- 2Department of Emergency Medicine, The First Affiliated Hospital, Sun Yat-Sen University, Guangzhou, China
- 3Department of General Practice, The First Affiliated Hospital, Sun Yat-Sen University, Guangzhou, China
- 4Department of Guangdong Cardiovascular Institute, Guangdong Provincial People's Hospital (Guangdong Academy of Medical Sciences), Southern Medical University, Guangzhou, China
- 5Department of Cardiology, The First Affiliated Hospital, Sun Yat-Sen University, Guangzhou, China
Background: The most prevalent sustained arrhythmia in medical practice, atrial fibrillation (AF) is closely associated with a high risk of cardiovascular disease. Nevertheless, the risk of AF associated with cardiovascular risk factors has not been well elucidated. We pooled all published studies to provide a better depiction of the relationship among cardiovascular risk factors with AF.
Methods: Studies were searched in the MEDLINE, Web of Science, and EMBASE databases since initiation until January 15, 2022. Prospective cohort studies assessing the relationship a minimum of single cardiovascular risk factors to AF incidence were included if they contained adequate data for obtaining relative risks (RR) and 95% confidence intervals (CI). Random-effects models were utilized to perform independent meta-analyses on each cardiovascular risk factor. PROSPERO registry number: CRD42022310882.
Results: A total of 17,098,955 individuals and 738,843 incident cases were reported for data from 101 studies included in the analysis. In all, the risk of AF was 1.39 (95% CI, 1.30–1.49) for obesity, 1.27 (95% CI, 1.22–1.32) per 5 kg/m2 for increase in body mass index, 1.19 (95% CI, 1.10–1.28) for former smokers, 1.23 (95% CI, 1.09–1.38) for current smokers, 1.31 (95% CI, 1.23–1.39) for diabetes mellitus, 1.68 (95% CI, 1.51–1.87) for hypertension, and 1.12 (95% CI, 0.95–1.32) for dyslipidemia.
Interpretation: Adverse cardiovascular risk factors correlate with an increased risk of AF, yet dyslipidemia does not increase the risk of AF in the general population, potentially providing new insights for AF screening strategies among patients with these risk factors.
Systematic Review Registration: https://www.crd.york.ac.uk/PROSPERO/, PROSPERO identifier (CRD42022310882).
1. Introduction
As the most prevalent arrhythmia diagnosed in medical practice (1), atrial fibrillation (AF) represents an enormous public health challenge with increasing clinical and public health expenses (2). The worldwide incidence in 2010 was evaluated at 5 million cases (3), whereas the prevalence in 2015 was evaluated at 33 million (4). There will be an expected rise in the prevalence of AF in people above 60 years of age from 3.9 million to 9 million by 2050 (5). Patients with AF are at high risk for stroke, thromboembolism and heart failure, leading to severe mortality and morbidity (6, 7). Costs to the economy caused by AF in the United States have been evaluated to exceed $6 billion per year (8). Therefore, primary prevention by changing risk factors is an important way to deal with the increased burden of atrial fibrillation (9).
Risk factors not subject to change, including age and gender, are non-controllable characteristics that have been shown to affect the occurrence of AF (10). As is well known, the general risk factors of cardiovascular disease comprise, obesity, smoking, dyslipidemia, diabetes and hypertension (11). Within prior studies, obesity, increment in body mass index (BMI), diabetes, and smoking have been proved to be related to elevated risk of AF (12–18). These meta-analyses, however, were only inclusive of studies released prior to 2017. Additional, data on dyslipidemia are finite and fairly disparate. Some observational studies unexpectedly found a “cholesterol paradox” in AF (19, 20), whereas others showed nonsignificant association (21–27). Contrary to expectations, certain observational studies revealed a counterintuitive link between blood cholesterol levels and atrial fibrillation risk, while a Mendelian randomization study involving individuals of European heritage failed to substantiate this correlation (28). Although the review showed that hypertension is a significant risk factor of AF (29), there is no available meta-analysis on the incidence of AF after hypertension based on prospective cohort studies.
Within this research, a systematic review and meta-analysis of prospective cohort studies were conducted performed to assess relationships among main cardiovascular risk factors, such as hypertension, diabetes mellitus, obesity, smoking, and dyslipidemia and the incidence of AF, focusing specifically on the intensity of effects of single risk factors.
2. Materials and methods
2.1. Search strategy
We conducted and reported this systematic review based on the prespecified standards (30) outlined by the PRISMA guidelines (31). The research program was registered with PROSPERO (number CRD42022310882). We systematically scoured the Web of Science, EMBASE, and MEDLINE databases from initiation until January 15, 2022, in search of studies regarding the relationship among cardiovascular risk factors with AF. The complete search strategies are provided on the appendix. All reference lists of previous meta-analyses, related reviews, and major reports were manually scoured to find additional matching studies.
2.2. Study selection
Abstracts and titles from searched articles were screened for eligibility independently by two reviewers (Wu G and Wu J). A third reviewer (Cheng Y) adjudicated disagreement. All studies deemed eligible based on title and abstract screening were reviewed for full text by two independent reviewers (Wu G, Wu J) using the same criteria. Discussion or involvement of a third reviewer (Cheng Y) was used to resolve inconsistent eligibility ratings. Studies were eligible for inclusion if they (1) were prospective cohort studies. (2) assessed the relationship for a minimum of one cardiovascular risk factor with occurrence of AF. (3) reported outcomes for hazard ratio (HR) or relative risk (RR) with 95% confidence intervals (CI), or offered adequate data to compute these outcomes. In case of a number of publications from an identical population, we included the most informative data (having enough baseline features and the most fully adjusted risk estimates). Exclusion criteria in detail can be found in the supplement.
2.3. Date extraction and quality assessment
Data were extracted individually by two authors (Wu G and Lu Q) using a standardized data extraction table. We extracted the following features from each qualifying study: name of the first author, year of publication, population source, geo-location, gender category, mean age at baseline, mean follow-up time, number of enrollees, number of incidents, approach of evaluating exposures and results, RR with respective 95% CIs, and adjusted covariates in multivariate analysis. The quality of included studies was assessed using the Newcastle-Ottawa scale (NOS) (32) for assessable items. In the present study, we regarded studies with score of six or above to be of high quality. Any disagreements were settled via consensus.
2.4. Data synthesis and analysis
The aggregated RRs were calculated using random effects models for each study's RR or HR. Random effects were utilized since these studies were performed in a broad setting with diverse population groups. This method would require that heterogeneity be taken into account while estimating aggregate effects. When the actual RRs were unavailable, the RRs and 95% CIs were calculated based on raw data. If RRs were usable, the most complete adjusted risk estimates presented in the publication were used. Pooled relative risks were expressed with 95% confidence intervals (CIs). We expressed continuous outcome data as weighted mean differences (WMDs) or mean differences (MDs) with 95% CIs. We used the Cochran's χ2 test to evaluate heterogeneity across studies, with quantification by the Cochran's Q and I2 statistics. A value in the range of 0–25% represented minimal heterogeneity, in the range of 26–75% indicated moderate heterogeneity, whereas in the range of over 75% denoted substantial heterogeneity (33).
The publication bias was tested using funnel plots and Egger's and Begg's regression tests (34, 35). Further publication bias was adjusted using Duval and Tweedie nonparametric trimming and filling procedures (36). To summarize, after assessing the number of studies in the asymmetric part, the approach removed the asymmetric edges of the funnel plot. To evaluate the actual center of the funnel by applying symmetric residual studies. Next, with the real center as the axis of symmetry, the studies pruned during the first step were first appended back to the trimmed funnel plot, while an equal number of predicted studies symmetrical to the trimmed studies were also appended back to the funnel plot. The ultimate composite estimation was derived in accordance with the filled funnel plot (37). We performed a number of sensitivity analyses to examine the robustness of the primary findings and to estimate possible sources of dissimilarity. To begin, fixed effects meta-analysis was conducted to evaluate the coherence of the primary outcomes of the random effects model. Next, to investigate the influence of study quality, sensitivity analyses were performed on significant quality ingredients, comprising covariates adjusted for in multivariate analyses (<3 factors or ≥3 factors), NOS scores (<6 or ≥6), average follow-up time (<10 or ≥10 years), case identification method (self-reported or measured), and subjective representativeness (occupation-based or population/community-based). Lastly, we excluded the two largest and outlier study estimates so as to investigate the impact of these studies on the aggregate RR. In all of our analyses, we utilized STATA, version 16.0 (Stata Corp LP, College Station, TX, USA). Bilateral P values <0.05 were deemed statistically significant.
3. Results
3.1. Study selection and baseline characteristics
The systematic search of articles published before January 15, 2022, identified 7,556 results. After title and abstract screening, 471 articles were considered potentially relevant. Ultimately, 101 articles were included in the meta-analysis after a full-text review (Figure 1). The characteristics of the study are described in Table 1 while detailed information is presented in Supplementary Tables S1–S11. The typical results of this study are summarized in Figure 2. In total, 17, 098, 955 participants were included in this study to check the risk of AF in individuals with cardiovascular risk factors in comparison to those without, for a total of 738,843 AF cases. For all participants, the mean age was 56.6 years (each study ranged from 18.2 to 75.8 years). The studies selected were conducted and published among the years 1995 to 2021. 31 of these studies were carried out from North America, 49 from Europe, 17 from Asia, as well as 4 from Oceania.
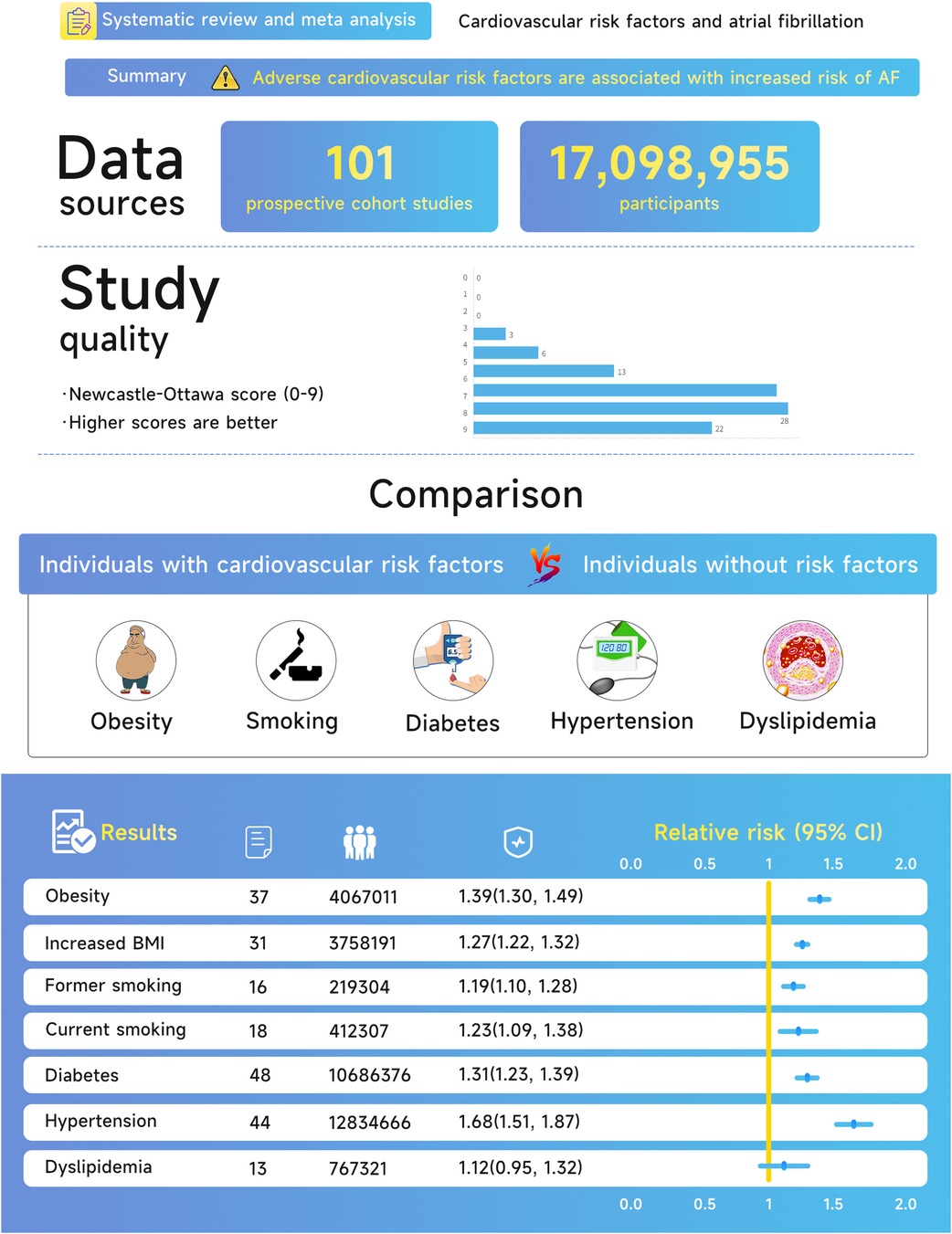
Figure 2. Central illustration of the association between cardiovascular risk factors and atrial fibrillation.
On the quality of research, 91% of enrolled studies were considered to be of excellent quality (NOS ≥ 6). There were 22 studies from occupational populations, 14 community-based researches, and 65 population-based researches among the 101 enrolled studies. Risk factor exposure was measured in 17 studies, self-reported in 2 studies, and established by additional approaches (combined physician diagnosis/medical history/present medication use/self-reported and measured data) in 82 studies. Adjusted RRs were provided for 87 studies, 77 of which adjusted for age and 76 for at least 3 significant comorbidity factors. Non-adjusted RRs were reported in 14 studies, and adjusted confounders were not available in a single study.
3.2. Obesity/BMI
An aggregate of 5,824,726 participants with 120,266 events enrolled in the investigation of the relationship of obesity/BMI with AF risk. On the whole, patients with AF had a higher mean BMI than controls (MD, 0.74 kg/m2; 95% CI, 0.52 -0.95), and there was statistically significant heterogeneity between studies (I2 = 90.2%; P < 0.01) (Supplementary figure S1). With a pooled RR on AF of 1.39 (95% CI, 1.30–1.49) for obesity and 1.27 (95% CI, 1.22–1.32) for each 5-unit increase in BMI, there was proof for a high degree of heterogeneity between the studies (I2 = 85.9% for obesity, P < 0.01; I2 = 90.5% for increased BMI, P < 0.01) (Figures 3A,B). After analyses of studies of excellent quality, studies enrolled in communities/populations, studies of average follow-up over 10 years, studies measuring height and weight, and fixed-effects models, there was no substantial variation in AF risk estimates associated with obesity or BMI, yet a high degree of heterogeneity remained (Supplementary Table S12). There was no measurable change in the pooled RR after excluding the two largest and outlier studies, and the estimated values for each case lay inside the confidence range of the aggregate estimate (Supplementary Table S12). Nevertheless, when the analysis of obesity and AF was limited to population/community-based studies, the summary RRs revealed no material variation, but significantly less heterogeneity (I2 = 4.6%, P = 0.40). Neither funnel plot, Egger's test, nor Begg's test published evidence of bias (Supplementary Figures S3A,B).
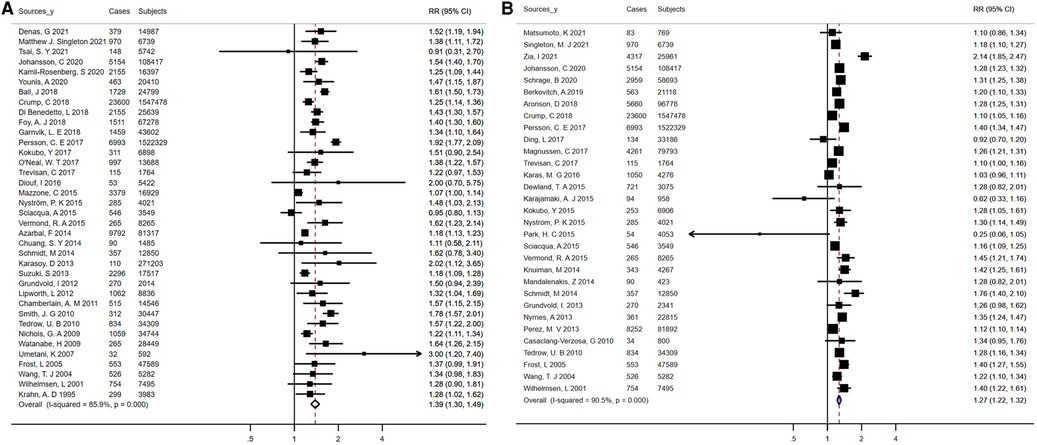
Figure 3. Forest plots for atrial fibrillation incidence (A) summary relative risks for obesity; (B) summary relative risks for Per 5 kg/m2 increase in body mass Index.
3.3. Smoking
This analysis included 18 studies reporting risk estimates for AF among former and current smokers as compared to never smokers, involving 412,307 participants and 19,907 events. Totally, for current smokers vs. never smokers and former smokers vs. never smokers, the pooled RRs were 1.23 (95% CI, 1.09–1.38) and 1.19 (95% CI, 1.10–1.28), separately (Figures 4A,B). Consistent increases in AF risk were observed among current and former smokers when analyses were repeated using fixed-effects models, comprising studies based on population/community, studies with an average follow-up exceeding 10 years, studies of high quality, and studies adjusted for over 3 confounders (Supplementary Table S14). Heterogeneity between studies was statistically significant in the analysis of current smokers, but partially not in the analysis of former smokers (I2 ranged from 59.5% to 89.8% for current smokers and 1.9% to 74.0% for former smokers, Supplementary Table S14). The sensitivity analysis showed no meaningful difference in RR after omitting an outlier study from the main analysis (Supplementary Table S14). Meanwhile, for current smokers (Egger's, t = − 0.79, P = 0.44; Begg's, z = 0.68, P = 0.50) and former smokers (Egger's, t = 0.49, P = 0.63; Begg's, z = 0.77, P = 0.44), no statistical proof of publication bias was available (data not shown).
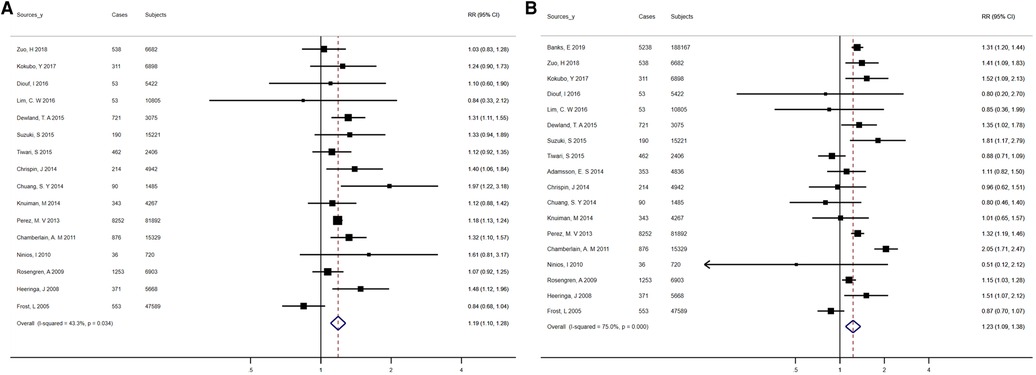
Figure 4. Forest plots for atrial fibrillation incidence (A) summary relative risks for former smokers vs. nonsmokers; (B) summary relative risks for current smokers versus nonsmokers.
3.4. Diabetes mellitus
With regard to diabetes mellitus, there were forty-eight studies included in the analysis, which reported 543,572 events in 106,863,776 participants. The overall pooled RR for the risk of AF in association with diabetes was 1.31 (95% CI, 1.23–1.39), with a high degree of heterogeneity between studies (I2 = 87.8%, P < 0.01) (Figure 5A). The pooled RRs did not vary significantly following analysis using fixed-effects models, inclusion of studies with an average duration of follow-up over 10 years, population/community-based studies, or high-quality studies, with a high degree of heterogeneity among studies (Supplementary Table S15). Heterogeneity remained after exclusion of the two largest studies (I2 = 81.1%, P < 0.01), and sensitivity analysis omitting one outlier study from the main analysis showed no material variation in the results (Supplementary Table S15). Potential publication bias was noted based on asymmetric funnel plots, Egger's test (t = 4.12, P < 0.01) and Begg's test (z = 2.43, P = 0.02) (data not shown). For assessing the effect of underlying publication bias, the trim and fill approach along with 15 extra estimation studies were applied to balance the funnel plot and an adjusted summary random-effects RR was calculated, which showed a statistically significant relationship of diabetes and AF [RR = 1.12 (95% CI, 1.05–1.19)], indicating that when we considered the effect of publication bias, there was still a positive association (Supplementary Figure S4).
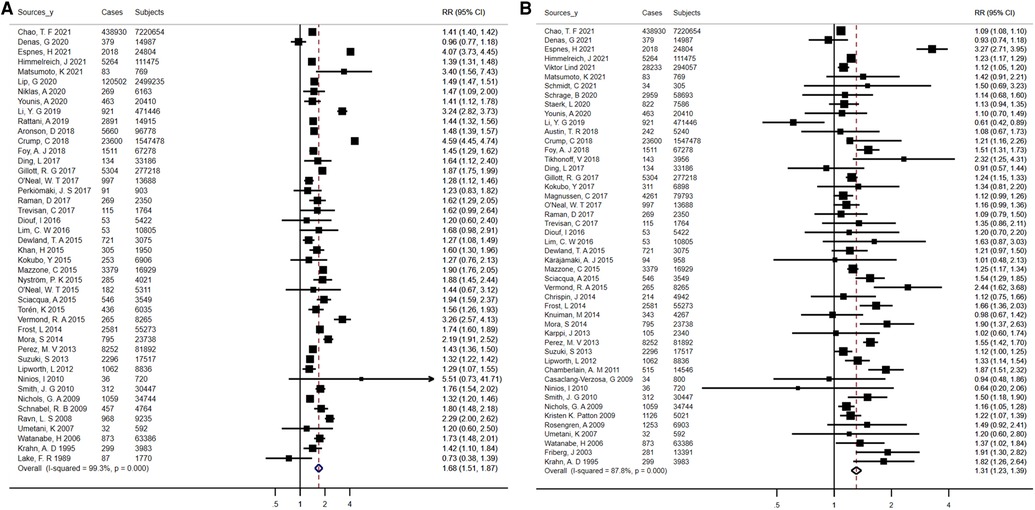
Figure 5. Forest plots for atrial fibrillation incidence (A) summary relative risks for diabetes; (B) summary relative risks for hypertension.
3.5. Hypertension
Reported 12,834,666 individuals and 634,988 events in 44 studies were included to assess the risk of AF among participants with hypertension. Altogether, compared with normotensive participants, hypertensive participants had an increased risk of AF [RR = 1.68 (95% CI, 1.51–1.87) for the random-effects model; RR = 1.51 (95% CI, 1.50–1.52) for the fixed-effects model] (Figure 5B). There was high heterogeneity among studies (I2 = 99.3%, P < 0.01) (Supplementary Table S15). There was little variation in risk estimates based on the different exclusion and inclusion criteria, but heterogeneity remained, as indicated by the results of the sensitivity analysis (Supplementary Table S15). According to the Egger's test (t = 1.45, P = 0.15) or the Begg's test (z = 0.23, P = 0.82), no significant publication bias was observed (data not shown).
3.6. Dyslipidemia
Involving 928,247 participants and 29,834 events, the analyses were performed to explore the relationship between AF prevalence and dyslipidemia. The overall pooled RR for the risk of AF associated with dyslipidemia was 1.12 (95% CI, 0.95–1.32), with a high degree of heterogeneity between studies (I2 = 92.9%, P < 0.01) (Figure 6). The mean levels of total cholesterol and triglycerides were not significantly related to AF (Supplementary Figures S2A,B). In addition, Low-density lipoprotein cholesterol (LDL-C) levels had no effect on the occurrence of AF (WMD, 0.02 mmol/L; 95% CI, −0.05 to 0.09), with statistically significant heterogeneity (I2 = 61.8%; P = 0.02). High-density lipoprotein cholesterol (HDL-C) levels were on average 0.05 mmol/L (95% CI, −0.07 to −0.02) lower in patients with atrial fibrillation than in controls, again with significant heterogeneity (I2 = 81.6%; P < 0.01). The results of sensitivity analyses showed no substantial change in the risk estimates for AF associated with dyslipidemia according to various exclusion and inclusion criteria, whereas heterogeneity persisted (Supplementary Table S16). When we repeated the analysis of total cholesterol and AF in studies with long-term follow-up (≥10 years), studies based on population/community, and studies of high quality, the pooled WMD remained statistically insignificant (Supplementary Table S17). Regarding the association between triglycerides and AF, the positive correlation was noted when we limited the meta-analysis to studies with a longer follow-up (≥10 years) (Supplementary Table S17). The negative association between HDL-C and AF persisted in analyses of studies based on population/community, high quality studies, and studies with an average follow-up over 10 years (I2 statistic values ranged from 72.7% to 87.7%), but disappeared in analyses of studies adjusted for at least 3 confounders and studies with large cohorts (Supplementary Table S18). No evidence of bias was published by funnel plot, Begg's test, or Egger's test (Supplementary Figures S5A,S6).
4. Discussion
The major risk factors for cardiovascular disease were discovered in association with enhanced risk of AF in this meta-analysis of a large sample size (over 17 million participants). The findings of our study broaden previous reports by showing not only that individual with well-established risk factors always underwent a heightened risk of AF, as well as demonstrating the relationship between AF and less well-defined risk factors. As far as we know, this is the first meta-analysis based entirely on prospective cohort studies summarizing literature on the association between hypertension with new-onset AF. Our pooled data reinforce findings from single studies. It is worth noting that there is no significant association between dyslipidemia and AF risk. Consistent to prior studies, the positive relationships among diabetes, smoking, increased BMI, obesity and AF were also demonstrated in our study. Despite the fact that obesity has been identified as a risk factor for AF, it is commonly found in conjunction with additional metabolic abnormalities, like dyslipidemia, hypertension and hyperglycemia, potentially mediating the relationship of obesity with the risk of AF (16, 38, 39). Recent studies found that both metabolically healthy and unhealthy obesity increase the risk of atrial fibrillation (40). Furthermore, there is a positive correlation between the cumulative burden of metabolic syndrome diagnostic criteria and its components over time and the risk of developing AF (41). In line to these discoveries, our study included obese individuals with a minimum of one metabolic abnormality and did observe a 39% increased risk of AF. In addition, the joint presence of risk factors probably related to additive impacts. Consequently, a thorough assessment of relevant risk factors is necessary when assessing the risk of AF.
Regarding hypertension, studies are numerous, but inconclusive. Several prospective cohort studies have shown that a strong risk factor for cardiovascular disease (CVD), including atrial fibrillation, is hypertension (29). Furthermore, in the study reported by Coccina F et al, both ambulatory and clinical systolic blood pressure prospectively predicted the onset of AF and daytime, nighttime, and 24-hour systolic blood pressure had similar correlations with future AF (42). Nevertheless, these studies covered a limited subgroup of population in general and were based on systolic blood pressure, potentially biasing the outcomes. Conversely, in a more general population, we indeed observed an increased risk of AF in those with pre-existing hypertension by 68%.
Despite our demonstration of an increased risk of AF in patients with hypertension and diabetes, it remains uncertain whether changing risk factors reduces the risk of AF. A meta-analysis conducted by Healey, J.S. et al. in 2005 included 11 randomized controlled human trials and demonstrated a 28% reduction in the risk of AF in angiotensin-converting enzyme inhibitor/angiotensin receptor blocker (ACEI/ARB) users compared to non-users (43). In addition, a 2015 meta-analysis showed that ACEI/ARBS could reduce the incidence of atrial fibrillation recurrence within 3 months and in long-term follow-up (44). For the treatment of diabetes, a meta-analysis based on 35 randomized controlled trials showed that sodium-glucose co-transport 2 (SGLT2) inhibitors significantly reduced the incidence of AF compared to placebo (45). Most recent meta-analyses also revealed that SGLT2 inhibitors were associated with a lower risk of AF (46, 47). These further suggest that hypertension and diabetes may be a cause of AF. However, recent evidence suggested a lack of significant protective effect of statins in the primary prevention of AF in the general population (48, 49), which also indicated that there was no apparent association between dyslipidemia and the risk of AF. With the exception of this, there was no causal relationship between total cholesterol, triglycerides, LDL cholesterol, HDL cholesterol and AF in the latest multivariate mendelian randomization study (50).
The specific bio-mechanisms behind these correlations are not completely clear, but can be attributed to some extent to the effects of structural (e.g., left atrial size), hemodynamic (e.g., left atrial stretch), electrical (e.g., altered conduction patterns due to atrial myocardial fibrosis), neurological (e.g., autonomic dysregulation), and inflammatory changes.
4.1. Inflammatory factors
It has been shown that accumulation of pericardial fat (51) and a systemic pro-inflammatory state produced by cytokines released from adipose tissue (52), as well as progressive atrial structural and electrical remodeling associated with obesity (53), may mediate the risk of atrial fibrillation.
4.2. Role of nicotine
Levels of nicotine in cigarettes produce an increase in plasma catecholamine levels (54), blood pressure and heart rate (55), and act as a potent inhibitor of cardiac A-type K+ channels. In addition, there is microRNA downregulation and transforming growth factor upregulation by nicotine, which leads to proarrhythmic atrial fibrosis (56).
4.3. Blood pressure and arterial stiffness
The relationship between increased blood pressure and atrial fibrillation exists for several explanations. It is directly related to the increase in left atrial diameter and blood pressure (57). Furthermore, measured by pulse pressure, increased arterial stiffness is associated with atrial fibrillation (58). Inflammation of the left atrium and arterial stiffness, which is associated with pulmonary venous fibrosis, which is the origin of atrial fibrillation, are potentially seen in people with elevated blood pressure, including pre-hypertension (59).
4.4. Insulin resistance and left ventricular hypertrophy
The cardiac autonomic neuropathy, similar to the peripheral autonomic neuropathy observed in diabetes, is a serious but neglected complication of diabetes (60, 61). Whereas, it is a hypothesis that cardiac autonomic dysfunction may be an important mechanism for the increased tendency of AF in diabetic patients. Added to this, insulin resistance is associated with an increased risk of left ventricular hypertrophy, which is itself a major risk factor for AF (62).
4.5. Dyslipidemia, cardiac load, and hyperthyroid status
Despite limited research on the mechanisms of dyslipidemia and AF, corresponding alterations in cardiac load (23), alterations in cardiac ion channels (63–65), and hyperthyroid status (66) may play a role. But whether this mechanism explains the observed association is hypothetical and requires further study.
Our findings may be useful in informing public health policy and allocating scarce resources for prevention. The RRs of each included study were pooled in our study to assess the intensity of the correlation, perform sensitivity analyses to examine the coherence of the relationship, and discuss potential biological mechanisms. In addition, the temporal nature of the prior exposure of the results was supported by the fact that all studies included in our analysis were prospective cohort designs. Moreover, the proof that treating several risk factors can decrease AF risk has been added. With this indicating that risk factors for CVD might as well be causative contributors to AF, more in-depth screening for AF in individuals having such risk factors is warranted.
To be acknowledged, the present meta-analysis was limited in the following aspects. First, we limited our analysis to individual risk elements, and there was a clear potential for the intensity of correlation to be weaker when multi-factor analysis was used. Second, there was substantial heterogeneity noted among the analyses of cardiovascular risk factors in spite of attempts at managing cross-study heterogeneity through proper meta-analysis techniques. However, in several sensitivity analyses, the risk estimates did not differ substantially, suggesting that heterogeneity may not affect the main results. Third, the potential for remnant or non-measured confounders could not be excluded, although the studies we included made attempts at controlling for a variety of identified risk factors. Fourth, some evidence suggested that publication bias was present solely within the analysis of diabetes. As shown in the funnel plot, there were some studies that lacked neutral or negative findings, indicating a potential overvaluation in the relationship. Fifth, the causal relationship cannot be determined in light of our present data based on observational studies, even though our results were robust and coherent across various sensitivity analyses. Sixth, cohorts of patients in some of the studies included in our meta-analysis were comparatively young and followed up for a short period of time, possibly contributing to the low prevalence of AF. The pooled RRs, nevertheless, did not materially vary when we performed repeated analyses of studies followed for over 10 years. Seventh, it was important to note that to extend such discoveries into African populations should be approached cautiously and would warrant additional inquiry, as our meta-analysis was primarily grounded in non-African research. Eighth, The studies we assessed might not have consistently differentiated between the various types of AF and diabetes. This disparity could lead to result discrepancies owing to variations in pathophysiological mechanisms and clinical implications within these subtypes. Future studies could enhance their insights by employing stratified analyses based on AF and diabetes subtypes.
Collectively, adverse cardiovascular risk factors were related to elevated risk of AF. Nevertheless, dyslipidemia does not increase the risk of AF. People with hypertension have a 68% increased relative risk of developing AF compared to those without hypertension, while obese patients had a 39% increased risk. In addition, current and former smoking were also associated with an increased risk of AF. Meanwhile, there is a need for caution in explaining the relationship of diabetes with AF, as publication bias is required to be considered.
Data availability statement
The original contributions presented in the study are included in the article/Supplementary Material, further inquiries can be directed to the corresponding authors.
Author contributions
Concept and design: WM, YC, GW. Acquisition, analysis, or interpretation of data: WM, YC, GW, JW, QL. Drafting of the manuscript: WM, YC, GW, JW, QL. Critical revision of the manuscript for important intellectual content: WM, YC, GW, JW. Statistical analysis: WM, YC, GW. All author contributed to the article and approved the submitted version.
Funding
The study was also financially supported by the grants from National Natural Science Foundation of China (81600260, 82270333), Guangdong Basic and Applied Basic Research Foundation (2021A1515010405, 2022A1515012358), and High-level Talents Introduction Plan of Guangdong Provincial People's Hospital (KY012023007). The funders of this research did not take part in research conception, literature screening, data extraction, statistical analysis, results discussion, or report preparation.
Conflict of interest
The authors declare that the research was conducted in the absence of any commercial or financial relationships that could be construed as a potential conflict of interest.
Publisher's note
All claims expressed in this article are solely those of the authors and do not necessarily represent those of their affiliated organizations, or those of the publisher, the editors and the reviewers. Any product that may be evaluated in this article, or claim that may be made by its manufacturer, is not guaranteed or endorsed by the publisher.
Supplementary material
The Supplementary Material for this article can be found online at: https://www.frontiersin.org/articles/10.3389/fcvm.2023.1110424/full#supplementary-material
References
1. Go AS, Hylek EM, Phillips KA, Chang Y, Henault LE, Selby JV, et al. Prevalence of diagnosed atrial fibrillation in adults: national implications for rhythm management and stroke prevention: the anticoagulation and risk factors in atrial fibrillation (atria) study. JAMA. (2001) 285:2370–5. doi: 10.1001/jama.285.18.2370
2. Ball J, Carrington MJ, McMurray JJ, Stewart S. Atrial fibrillation: profile and burden of an evolving epidemic in the 21st century. Int J Cardiol. (2013) 167:1807–24. doi: 10.1016/j.ijcard.2012.12.093
3. Chugh SS, Havmoeller R, Narayanan K, Singh D, Rienstra M, Benjamin EJ, et al. Worldwide epidemiology of atrial fibrillation: a global burden of disease 2010 study. Circulation. (2014) 129:837–47. doi: 10.1161/CIRCULATIONAHA.113.005119
4. GBD 2015 Disease and Injury Incidence and Prevalence Collaborators: Vos T, Allen C, Arora M, Barber RM, Bhutta ZA, Brown A, et al. Global, regional, and national incidence, prevalence, and years lived with disability for 310 diseases and injuries, 1990–2015: a systematic analysis for the global burden of disease study 2015. Lancet (London, England). (2016) 388:1545–602. doi: 10.1016/S0140-6736(16)31678-6
5. Rahman F, Kwan GF, Benjamin EJ. Global epidemiology of atrial fibrillation. Nat Rev Cardiol. (2014) 11:639–54. doi: 10.1038/nrcardio.2014.118
6. Lip GY, Tse HF, Lane DA. Atrial fibrillation. Lancet (London, England). (2012) 379:648–61. doi: 10.1016/S0140-6736(11)61514-6
7. Odutayo A, Wong CX, Hsiao AJ, Hopewell S, Altman DG, Emdin CA. Atrial fibrillation and risks of cardiovascular disease, renal disease, and death: systematic review and meta-analysis. BMJ (Clinical Research ed.). (2016) 354:i4482. doi: 10.1136/bmj.i4482
8. Coyne KS, Paramore C, Grandy S, Mercader M, Reynolds M, Zimetbaum P. Assessing the direct costs of treating nonvalvular atrial fibrillation in the United States. Value Health. (2006) 9:348–56. doi: 10.1111/j.1524-4733.2006.00124.x
9. Lau DH, Nattel S, Kalman JM, Sanders P. Modifiable risk factors and atrial fibrillation. Circulation. (2017) 136:583–96. doi: 10.1161/CIRCULATIONAHA.116.023163
10. Andrade J, Khairy P, Dobrev D, Nattel S. The clinical profile and pathophysiology of atrial fibrillation: relationships among clinical features, epidemiology, and mechanisms. Circ Res. (2014) 114:1453–68. doi: 10.1161/CIRCRESAHA.114.303211
11. Ageno W, Becattini C, Brighton T, Selby R, Kamphuisen PW. Cardiovascular risk factors and venous thromboembolism: a meta-analysis. Circulation. (2008) 117:93–102. doi: 10.1161/CIRCULATIONAHA.107.709204
12. Aune D, Feng T, Schlesinger S, Janszky I, Norat T, Riboli E. Diabetes mellitus, blood glucose and the risk of atrial fibrillation: a systematic review and meta-analysis of cohort studies. J Diabetes Complicat. (2018) 32:501–11. doi: 10.1016/j.jdiacomp.2018.02.004
13. Aune D, Schlesinger S, Norat T, Riboli E. Tobacco smoking and the risk of atrial fibrillation: a systematic review and meta-analysis of prospective studies. Eur J Prev Cardiol. (2018) 25:1437–51. doi: 10.1177/2047487318780435
14. Wang Q, Guo Y, Wu C, Yin L, Li W, Shen H, et al. Smoking as a risk factor for the occurrence of atrial fibrillation in men versus women: a meta-analysis of prospective cohort studies. Heart Lung Circ. (2018) 27:58–65. doi: 10.1016/j.hlc.2017.01.021
15. Zhu W, Yuan P, Shen Y, Wan R, Hong K. Association of smoking with the risk of incident atrial fibrillation: a meta-analysis of prospective studies. Int J Cardiol. (2016) 218:259–66. doi: 10.1016/j.ijcard.2016.05.013
16. Asad Z, Abbas M, Javed I, Korantzopoulos P, Stavrakis S. Obesity is associated with incident atrial fibrillation independent of gender: a meta-analysis. J Cardiovasc Electrophysiol. (2018) 29:725–32. doi: 10.1111/jce.13458
17. Aune D, Sen A, Schlesinger S, Norat T, Janszky I, Romundstad P, et al. Body mass index, abdominal fatness, fat mass and the risk of atrial fibrillation: a systematic review and dose-response meta-analysis of prospective studies. Eur J Epidemiol. (2017) 32:181–92. doi: 10.1007/s10654-017-0232-4
18. Wong CX, Sun MT, Odutayo A, Emdin CA, Mahajan R, Lau DH, et al. Associations of epicardial, abdominal, and overall adiposity with atrial fibrillation. Circ Arrhythm Electrophysiol. (2016) 9(12):e0043. doi: 10.1161/CIRCEP.116.004378
19. Lee HJ, Lee SR, Choi EK, Han KD, Oh S. Low lipid levels and high variability are associated with the risk of new-onset atrial fibrillation. J Am Heart Assoc. (2019) 8:e012771. doi: 10.1161/JAHA.119.012771
20. Mora S, Akinkuolie AO, Sandhu RK, Conen D, Albert CM. Paradoxical association of lipoprotein measures with incident atrial fibrillation. Circ Arrhythm Electrophysiol. (2014) 7:612–9. doi: 10.1161/CIRCEP.113.001378
21. Kokubo Y, Watanabe M, Higashiyama A, Nakao YM, Kusano K, Miyamoto Y. Development of a basic risk score for incident atrial fibrillation in a Japanese general population- the suita study. Circ J. (2017) 81:1580–8. doi: 10.1253/circj.CJ-17-0277
22. Knuiman M, Briffa T, Divitini M, Chew D, Eikelboom J, McQuillan B, et al. A cohort study examination of established and emerging risk factors for atrial fibrillation: the busselton health study. Eur J Epidemiol. (2014) 29:181–90. doi: 10.1007/s10654-013-9875-y
23. Alonso A, Yin X, Roetker NS, Magnani JW, Kronmal RA, Ellinor PT, et al. Blood lipids and the incidence of atrial fibrillation: the multi-ethnic study of atherosclerosis and the framingham heart study. J Am Heart Assoc. (2014) 3:e001211. doi: 10.1161/JAHA.114.001211
24. Nyrnes A, Mathiesen EB, Njølstad I, Wilsgaard T, Løchen ML. Palpitations are predictive of future atrial fibrillation. An 11-year follow-up of 22,815 men and women: the tromsø study. Eur J Prev Cardiol. (2013) 20:729–36. doi: 10.1177/2047487312446562
25. Alonso A, Krijthe BP, Aspelund T, Stepas KA, Pencina MJ, Moser CB, et al. Simple risk model predicts incidence of atrial fibrillation in a racially and geographically diverse population: the charge-af consortium. J Am Heart Assoc. (2013) 2:e000102. doi: 10.1161/JAHA.112.000102
26. Lopez FL, Agarwal SK, Maclehose RF, Soliman EZ, Sharrett AR, Huxley RR, et al. Blood lipid levels, lipid-lowering medications, and the incidence of atrial fibrillation: the atherosclerosis risk in communities study. Circ Arrhythm Electrophysiol. (2012) 5:155–62. doi: 10.1161/CIRCEP.111.966804
27. Rosengren A, Hauptman PJ, Lappas G, Olsson L, Wilhelmsen L, Swedberg K. Big men and atrial fibrillation: effects of body size and weight gain on risk of atrial fibrillation in men. Eur Heart J. (2009) 30:1113–20. doi: 10.1093/eurheartj/ehp076
28. Norby FL, Eryd SA, Niemeijer MN, Rose LM, Smith AV, Yin X, et al. Association of lipid-related genetic variants with the incidence of atrial fibrillation: the afgen consortium. PloS One. (2016) 11:e0151932. doi: 10.1371/journal.pone.0151932
29. Kokubo Y, Matsumoto C. Hypertension is a risk factor for several types of heart disease: review of prospective studies. Adv Exp Med Biol. (2017) 956:419–26. doi: 10.1007/5584_2016_99
30. Booth A. Prospero’s progress and activities 2012/13. Syst Rev. (2013) 2:111. doi: 10.1186/2046-4053-2-111
31. Moher D, Liberati A, Tetzlaff J, Altman DG. Preferred reporting items for systematic reviews and meta-analyses: the prisma statement. PLoS Med. (2009) 6:e1000097. doi: 10.1371/journal.pmed.1000097
32. Wells G, Shea B, O'Connell J. The Newcastle-Ottawa scale (nos) for assessing the quality of nonrandomised studies in meta-analyses. Ottawa Health Res Inst Web Site. (2014) 7.
33. Higgins JP, Thompson SG, Deeks JJ, Altman DG. Measuring inconsistency in meta-analyses. BMJ (Clinical Research ed.). (2003) 327:557–60. doi: 10.1136/bmj.327.7414.557
34. Egger M, Davey Smith G, Schneider M, Minder C. Bias in meta-analysis detected by a simple, graphical test. BMJ (Clinical Research ed.). (1997) 315:629–34. doi: 10.1136/bmj.315.7109.629
35. Begg CB, Mazumdar M. Operating characteristics of a rank correlation test for publication bias. Biometrics. (1994) 50:1088–101. doi: 10.2307/2533446
36. Higgins JP, Thompson SG. Quantifying heterogeneity in a meta-analysis. Stat Med. (2002) 21:1539–58. doi: 10.1002/sim.1186
37. Duval S, Tweedie R. Trim and fill: a simple funnel-plot-based method of testing and adjusting for publication bias in meta-analysis. Biometrics. (2000) 56:455–63. doi: 10.1111/j.0006-341X.2000.00455.x
38. Nyström PK, Carlsson AC, Leander K, de Faire U, Hellenius ML, Gigante B. Obesity, metabolic syndrome and risk of atrial fibrillation: a Swedish, prospective cohort study. PloS One. (2015) 10:e0127111. doi: 10.1371/journal.pone.0127111
39. Watanabe H, Tanabe N, Watanabe T, Darbar D, Roden DM, Sasaki S, et al. Metabolic syndrome and risk of development of atrial fibrillation: the niigata preventive medicine study. Circulation. (2008) 117:1255–60. doi: 10.1161/CIRCULATIONAHA.107.744466
40. Feng T, Vegard M, Strand LB, Laugsand LE, Mørkedal B, Aune D, et al. Metabolically healthy obesity and risk for atrial fibrillation: the hunt study. Obesity (Silver Spring, Md.). (2019) 27:332–8. doi: 10.1002/oby.22377
41. Ahn HJ, Han KD, Choi EK, Jung JH, Kwon S, Lee SR, et al. Cumulative burden of metabolic syndrome and its components on the risk of atrial fibrillation: a nationwide population-based study. Cardiovasc Diabetol. (2021) 20:20. doi: 10.1186/s12933-021-01215-8
42. Coccina F, Pierdomenico AM, De Rosa M, Cuccurullo C, Pierdomenico SD. Association of clinic and ambulatory blood pressure with new-onset atrial fibrillation: a meta-analysis of observational studies. J Clin Hypertens. (2021) 23:1104–11. doi: 10.1111/jch.14256
43. Healey JS, Baranchuk A, Crystal E, Morillo CA, Garfinkle M, Yusuf S, et al. Prevention of atrial fibrillation with angiotensin-converting enzyme inhibitors and angiotensin receptor blockers: a meta-analysis. J Am Coll Cardiol. (2005) 45:1832–9. doi: 10.1016/j.jacc.2004.11.070
44. Zhao D, Wang ZM, Wang LS. Prevention of atrial fibrillation with renin-angiotensin system inhibitors on essential hypertensive patients: a meta-analysis of randomized controlled trials. J Biomed Res. (2015) 29:475–85. doi: 10.7555/JBR.29.20140149
45. Usman MS, Siddiqi TJ, Memon MM, Khan MS, Rawasia WF, Talha Ayub M, et al. Sodium-glucose co-transporter 2 inhibitors and cardiovascular outcomes: a systematic review and meta-analysis. Eur J Prev Cardiol. (2018) 25:495–502. doi: 10.1177/2047487318755531
46. Zheng RJ, Wang Y, Tang JN, Duan JY, Yuan MY, Zhang JY. Association of sglt2 inhibitors with risk of atrial fibrillation and stroke in patients with and without type 2 diabetes: a systemic review and meta-analysis of randomized controlled trials. J Cardiovasc Pharmacol. (2021) 79:e145–152. doi: 10.1097/FJC.0000000000001183
47. Ong HT, Teo YH, Teo YN, Syn NL, Wee CF, Leong S, et al. Effects of sodium/glucose cotransporter inhibitors on atrial fibrillation and stroke: a meta-analysis. J Stroke Cerebrovasc Dis. (2022) 31:106159. doi: 10.1016/j.jstrokecerebrovasdis.2021.106159
48. Fauchier L, Pierre B, de Labriolle A, Grimard C, Zannad N, Babuty D. Antiarrhythmic effect of statin therapy and atrial fibrillation a meta-analysis of randomized controlled trials. J Am Coll Cardiol. (2008) 51:828–35. doi: 10.1016/j.jacc.2007.09.063
49. Alves-Cabratosa L, García-Gil M, Comas-Cufí M, Ponjoan A, Martí-Lluch R, Parramon D, et al. Statins and new-onset atrial fibrillation in a cohort of patients with hypertension. Analysis of electronic health records, 2006–2015. PloS One. (2017) 12:e0186972. doi: 10.1371/journal.pone.0186972
50. Jiang Q, Qin D, Yang L, Lin Y, Zhai L, Zhang Y, et al. Causal effects of plasma lipids on the risk of atrial fibrillation: a multivariable Mendelian randomization study. Nutr Metab Cardiovasc Dis. (2021) 31:1569–78. doi: 10.1016/j.numecd.2021.02.011
51. Nalliah CJ, Sanders P, Kottkamp H, Kalman JM. The role of obesity in atrial fibrillation. Eur Heart J. (2016) 37:1565–72. doi: 10.1093/eurheartj/ehv486
52. Scridon A, Dobreanu D, Chevalier P, Şerban RC. Inflammation, a link between obesity and atrial fibrillation. Inflamm Res. (2015) 64:383–93. doi: 10.1007/s00011-015-0827-8
53. Abed HS, Samuel CS, Lau DH, Kelly DJ, Royce SG, Alasady M, et al. Obesity results in progressive atrial structural and electrical remodeling: implications for atrial fibrillation. Heart Rhythm. (2013) 10:90–100. doi: 10.1016/j.hrthm.2012.08.043
54. Haass M, Kübler W. Nicotine and sympathetic neurotransmission. Cardiovasc Drugs Ther. (1997) 10:657–65. doi: 10.1007/BF00053022
55. Benowitz NL, Jacob P, Jones RT, Rosenberg J. Interindividual variability in the metabolism and cardiovascular effects of nicotine in man. J Pharmacol Exp Ther. (1982) 221:368–72. 7077531
56. Shan H, Zhang Y, Lu Y, Zhang Y, Pan Z, Cai B, et al. Downregulation of mir-133 and mir-590 contributes to nicotine-induced atrial remodelling in canines. Cardiovasc Res. (2009) 83:465–72. doi: 10.1093/cvr/cvp130
57. Vaziri SM, Larson MG, Lauer MS, Benjamin EJ, Levy D. Influence of blood pressure on left atrial size. The framingham heart study. Hypertension. (1995) 25:1155–60. doi: 10.1161/01.HYP.25.6.1155
58. Mitchell GF, Vasan RS, Keyes MJ, Parise H, Wang TJ, Larson MG, et al. Pulse pressure and risk of new-onset atrial fibrillation. JAMA. (2007) 297:709–15. doi: 10.1001/jama.297.7.709
59. Pan NH, Tsao HM, Chang NC, Lee CM, Chen YJ, Chen SA. Dilated left atrium and pulmonary veins in patients with calcified coronary artery: a potential contributor to the genesis of atrial fibrillation. J Cardiovasc Electrophysiol. (2009) 20:153–8. doi: 10.1111/j.1540-8167.2008.01290.x
60. Vinik AI, Maser RE, Mitchell BD, Freeman R. Diabetic autonomic neuropathy. Diabetes Care. (2003) 26:1553–79. doi: 10.2337/diacare.26.5.1553
61. Valensi P, Sachs RN, Harfouche B, Lormeau B, Paries J, Cosson E, et al. Predictive value of cardiac autonomic neuropathy in diabetic patients with or without silent myocardial ischemia. Diabetes Care. (2001) 24:339–43. doi: 10.2337/diacare.24.2.339
62. Watanabe K, Sekiya M, Tsuruoka T, Funada J, Kameoka H. Effect of insulin resistance on left ventricular hypertrophy and dysfunction in essential hypertension. J Hypertens. (1999) 17:1153–60. doi: 10.1097/00004872-199917080-00015
63. Baartscheer A, Schumacher CA, Wekker V, Verkerk AO, Veldkamp MW, van Oort RJ, et al. Dyscholesterolemia protects against ischemia-induced ventricular arrhythmias. Circ Arrhythm Electrophysiol. (2015) 8:1481–90. doi: 10.1161/CIRCEP.115.002751
64. Epshtein Y, Chopra AP, Rosenhouse-Dantsker A, Kowalsky GB, Logothetis DE, Levitan I. Identification of a c-terminus domain critical for the sensitivity of kir2.1 to cholesterol. Proc Natl Acad Sci U S A. (2009) 106:8055–60. doi: 10.1073/pnas.0809847106
65. Balse E, El-Haou S, Dillanian G, Dauphin A, Eldstrom J, Fedida D, et al. Cholesterol modulates the recruitment of kv1.5 channels from rab11-associated recycling endosome in native atrial myocytes. Proc Natl Acad Sci U S A. (2009) 106:14681–6. doi: 10.1073/pnas.0902809106
Keywords: cardiovascular risk factors, atrial fibrillation, association, meta-analysis and systematic review, prospective cohort studies
Citation: Wu G, Wu J, Lu Q, Cheng Y and Mei W (2023) Association between cardiovascular risk factors and atrial fibrillation. Front. Cardiovasc. Med. 10:1110424. doi: 10.3389/fcvm.2023.1110424
Received: 8 December 2022; Accepted: 24 August 2023;
Published: 11 September 2023.
Edited by:
Rui Providência, University College London, United KingdomReviewed by:
Marc Badoz, Centre Hospitalier Universitaire de Besançon, FranceTeresa Strisciuglio, University of Naples Federico II, Italy
Catrin Sohrabi, Royal Free Hospital, United Kingdom
Jonathan Bray, Oxford University Hospitals NHS Trust, United Kingdom
© 2023 Wu, Wu, Lu, Cheng and Mei. This is an open-access article distributed under the terms of the Creative Commons Attribution License (CC BY). The use, distribution or reproduction in other forums is permitted, provided the original author(s) and the copyright owner(s) are credited and that the original publication in this journal is cited, in accordance with accepted academic practice. No use, distribution or reproduction is permitted which does not comply with these terms.
*Correspondence: Yunjiu Cheng cheng831011@sina.com Weiyi Mei meiweiyi@mail.sysu.edu.cn
†These authors have contributed equally to this work