- 1National Centre for Biological Sciences (Tata Institute of Fundamental Research), Bangalore, India
- 2Molecular Biophysics Unit, Indian Institute of Science, Bangalore, India
- 3Institute of Bioinformatics and Applied Biotechnology, Bangalore, India
Introduction: Cardiomyopathies are complex heart diseases with significant prevalence around the world. Among these, primary forms are the major contributors to heart failure and sudden cardiac death. As a high-energy demanding engine, the heart utilizes fatty acids, glucose, amino acid, lactate and ketone bodies for energy to meet its requirement. However, continuous myocardial stress and cardiomyopathies drive towards metabolic impairment that advances heart failure (HF) pathogenesis. So far, metabolic profile correlation across different cardiomyopathies remains poorly understood.
Methods: In this study, we systematically explore metabolic differences amongst primary cardiomyopathies. By assessing the metabolic gene expression of all primary cardiomyopathies, we highlight the significantly shared and distinct metabolic pathways that may represent specialized adaptations to unique cellular demands. We utilized publicly available RNA-seq datasets to profile global changes in the above diseases (|log2FC| ≥ 0.28 and BH adjusted p-val 0.1) and performed gene set analysis (GSA) using the PAGE statistics on KEGG pathways.
Results: Our analysis demonstrates that genes in arachidonic acid metabolism (AA) are significantly perturbed across cardiomyopathies. In particular, the arachidonic acid metabolism gene PLA2G2A interacts with fibroblast marker genes and can potentially influence fibrosis during cardiomyopathy.
Conclusion: The profound significance of AA metabolism within the cardiovascular system renders it a key player in modulating the phenotypes of cardiomyopathies.
1. Introduction
Primary cardiomyopathies, predominantly hypertrophic cardiomyopathy (HCM), dilated cardiomyopathy (DCM), restrictive cardiomyopathy (RCM) and arrhythmogenic cardiomyopathy (ACM) are a growing global burden on public health (1–6). Primary cardiomyopathies, on the whole, are genetic but tend to be influenced by environment and lifestyle (6–12). These heterogeneous heart muscle diseases lead to heart failure and sudden cardiac death (1, 6). Notably, metabolic impairment is vital in heart failure (13, 14). Generally, the heart meets its energy demand by utilizing fatty acids, glucose, amino acid, lactate and ketone bodies, but cardiomyopathies lead to severe metabolic perturbations (15–17). In cardiovascular research, metabolic explorations have provided new insights (18, 19). The role of metabolic genes and pathways has been explored in several cardiomyopathy studies to understand the pathophysiological changes involved in the progression of cardiomyopathy (15–17, 20–22). These studies have reported a metabolic shift in energy sources during disease progression. HCM-focused transcriptome analysis showed down-regulated fatty acid metabolism (23). Similarly, in a DCM transcriptome analysis, mitochondrial dysfunction and oxidative phosphorylation pathways were significantly altered (24). Concurrently, multi-omics technologies have provided an opportunity to explore metabolic disruptions at a large scale. RNA-seq technique allows rapid measurement of global gene expression in the disease of interest. Further, it provides an indirect mechanism to assess metabolic alterations (25–27). Several computational algorithms incorporating transcriptome data and metabolic networks have been developed to assess the perturbation of biological pathways (28–30).
Over the years, oxidative phosphorylation, glucose and fatty acid metabolism have been highlighted exhibiting disturbances in individual cardiomyopathy studies. Albeit being important, individual cardiomyopathy-focused metabolic studies miss overall molecular patterns across cardiomyopathies. A comparative interpretation of the primary cardiomyopathies' metabolic alterations is crucial for a comprehensive understanding of the mechanisms of metabolic shifts. To the best of our knowledge, our study is the first to systematically explore metabolic correlations amongst primary cardiomyopathies (ACM, DCM, HCM and RCM).
This study aimed to identify shared metabolic perturbations across cardiomyopathies. We utilized gene expression profiles of primary cardiomyopathies: ACM, DCM, HCM and RCM, and donor samples. We carried out differential gene expression analysis of cardiomyopathy datasets. Further, we performed GSA on each dataset to investigate the metabolic alterations comprehensively. Apart from glycolysis and oxidative phosphorylation, the arachidonic acid (AA) metabolism pathway was significantly altered in all cardiomyopathy sets. Subsequently, we inferred the potential cell types in the candidate pathway using the snRNA-seq dataset. This analysis helped in the identification of marker genes for each cell type and the potential link between AA metabolism genes and cell type marker genes.
Earlier studies did not account for cross-comparison nor focused on arachidonic acid metabolism in depth. Arachidonic acid (AA) is a free fatty acid metabolized by cyclooxygenase (COX), lipoxygenase (LOX), and cytochrome P450 (CYP450) epoxygenase enzymes into biologically active fatty acid mediators (31). Through these mediators, AA participates in complex cardiovascular functions, including fibrosis (32, 33). COX, called Prostaglandin G/H synthases (PGHS), is attributed to synthesizing autoregulatory and homeostatic prostanoids. COX has two subtypes: COX-1 and COX-2, which convert AA to prostaglandin (PG)G2 and PGH2, respectively. These prostaglandins are further processed to various PGs such as PGD2, PGE2, PGF2α, and prostacyclin (PGI2) (34). PGD2 protects against atherosclerosis and thrombosis by increasing vascular permeability and blood flow (35). LOXs, such as 5-LOX, 12-LOX, and 15-LOX, catalyze the dioxygenation of AA to their respective hydroperoxyeicosatetraenoic acids (HPETEs), such as 5-HPETE, 12-HPETE, and 15-HPETE. These HPETEs are then transformed into hydroxyeicosatetraenoic acids (HETEs), leukotrienes (LTs), and lipoxins (LXs). The 15-LOX pathway is reported to be involved in the development of atherosclerosis (36). Epoxyeicosatrienoic acids (EETs) are metabolites produced from arachidonic acid (AA) through the action of CYP epoxygenases. These EETs are synthesized primarily by CYP2J2, CYP2C8, and CYP2C9 enzymes in the human heart, liver, and endothelial cells (37). Among these enzymes, CYP2J2 is noteworthy since it is the only human CYP2J2 epoxygenase and is highly expressed in the heart and endothelium in particular (38). CYP2J2 converts AA into four regioisomeric EETs, including 5,6-, 8,9-, 11,12-, and 14,15-EET (39). The 14,15-EET is released by the endothelium in BK-induced cardiodepression (40). Once formed, EETs are transformed into less active dihydroxyeicosatrienoic acids (DHETs) by the action of soluble epoxide hydrolase (sEH). Research has shown that EETs protect the heart against inflammation, endothelial dysfunction, cardiac remodeling, and fibrosis (41, 42). Our analysis indicates that AA enzymes are expressed in various cell types within the heart tissue, including fibroblasts, cardiomyocytes, smooth muscle cells, monocytes, macrophages, and mast cells. As a cardioprotective mechanism, the CYP2J2 gene expression was upregulated in HCM and DCM phenotypes. Further PLA2G2A gene among genes coding for enzymes of the phospholipase A2 (PLA2) superfamily was dysregulated in most cardiomyopathies under analysis. The phospholipase A2 enzymes catalyze the endogenous production of AA mainly from cell membrane phospholipids (43). These findings highlight the importance of investigating the role of AA metabolism in cardiomyopathies. Overall, our study highlights novel and clinically valuable aspects of cardiomyopathies, with implications ranging from prognosis to therapeutic intervention.
2. Materials and methods
2.1. Data acquisition and preprocessing
Transcriptomic raw data comprising RNA-seq, single-nucleus RNA-seq (snRNA-seq) and microarray for cardiomyopathy studies were acquired from the European Nucleotide Archive (ENA) database (https://www.ebi.ac.uk/ena/browser/) and Gene Expression Omnibus (GEO) database (https://www.ncbi.nlm.nih.gov/geo/). Search terms like “hypertrophic cardiomyopathy”, “dilated cardiomyopathy”, “restrictive cardiomyopathy”, and “arrhythmogenic cardiomyopathy” were searched against these databases to obtain the RNA-seq and microarray dataset results. Each result was manually reviewed and considered for inclusion if (1) the disease samples in the study indicated heart tissues from the cardiomyopathy patients, and (2) control samples came from non-failing heart patients. We focused on the studies that were supported in our R-analysis pipeline. We considered seven publicly available cardiomyopathy transcriptomic data, including five RNA-seq (SRP125284, SRP125595, SRP052978, SRP186138 and SRP061888) and two microarray datasets (GSE29819, GSE36961) using these inclusion criteria. One single-nucleus RNA sequencing (snRNA-seq) dataset (GSE183852) with dilated cardiomyopathy (DCM) was selected to explore cell type expression of candidate genes due to the cell annotation in the supplementary files of the original dataset (44). The details of the transcriptome datasets are shown in Supplementary Table S1.
2.2. Data processing and differential expressed genes (DEGs)
Previously described and curated raw data were downloaded and reprocessed to ensure uniform processing and normalization of each study. RNA-seq and microarray studies were processed using independent pipelines. In the RNA-seq pipeline, custom shell scripts were used to download data. Salmon (v1.5.2) (a fast and bias-aware quantification tool) was utilized to align and quantify samples using NCBI human reference transcriptome (Gencode v38) (45). The count data output of Salmon quantification was used for differential gene expression analysis between disease and donor samples. This analysis was performed using the DESeq2 (v1.26.0) package in R (v 3.6.3) (46). It uses the negative binomial distribution to model a statistical analysis for differential gene expression. It also normalizes samples automatically. Wald t-test was applied to the distribution (47). To control the false discovery rate (FDR), the resultant p-values were adjusted using Benjamini and Hochberg's test (BH) correction (48). Genes with adjusted p < 0.1 and |log2FC| ≥ 0.28 were assigned as being differentially expressed. Likewise, microarray datasets were processed with the limma (v3.42.2) package (49). A linear model was constructed between disease and control samples, and the empirical Bayes statistical method was utilized to obtain the significant genes. BH correction was used to obtain the adjusted p value. The snRNA-seq expression stored as the R object (44) was processed and analyzed using the R package “Seurat” (version 3.2.3) (50).
2.3. Sample variability and study consistency
Principal component analysis (PCA) and t-distributed stochastic neighbor embedding (t-SNE) were applied to assess the sample variability across datasets. Only RNA-seq datasets were explored for testing. These datasets were normalized using the “DESeq” method in the R package. The R function “prcomp” was used to perform PCA. On the other hand, the “Rtsne” function was utilized to perform t-SNE. Principal component and t-SNE plots, Venn diagrams, and heatmap plots were prepared using the ggplot2 R package (version 3.3.5) and the Matplotlib package in Python 3.
2.4. Gene set analysis (GSA)
The gene set analysis was carried out on the gene expression data to identify significantly perturbed pathways in each study. We utilized p-values from the differential expression analysis for all genes in individual datasets for this analysis. A ranking was generated based on these p-values, representing the input for Gene-level statistics (gene expression). The Kyoto Encyclopedia of Genes and Genomes (KEGG) pathways list file was downloaded from KEGG (https://www.kegg.jp). Parametric analysis of gene set enrichment (PAGE) was employed for GSA analysis using the Piano (version 2.2.0) package in R (51, 52). The PAGE method uses the mean of the gene-level statistics of a gene set (a particular pathway in this case) and corrects for the background, represented by all gene-level statistics. The cumulative normal distribution is used to estimate the PAGE gene-level statistics significance. Heatmap of pathways, volcano plots and bar charts were plotted using Matplotlib and Seaborn packages in Python 3.
2.5. Identification and screening of significant pathways
Pathways with PAGE statistics p-values < 0.1 were considered significant. Among many altered pathways like glycolysis and oxidative phosphorylation, arachidonic acid metabolism was chosen as the candidate for further analysis due to its significance in all primary cardiomyopathies. To show that the AA metabolism perturbation was consistent in all datasets rather than only individual studies, we used microarray data to validate its role in ACM, DCM and HCM independently. We could not find any microarray dataset for RCM, so it was not considered. Gene set analysis of these microarray studies was performed using the sorted Gene-level statistics (GSA) as previously described. Genes differentially regulated in AA metabolism were mapped to the KEGG mapper using the online server: Pathview Web (https://pathview.uncc.edu/) (53).
2.6. Finding DEGs, cell types annotation in arachidonic acid metabolism and cell-specific marker genes
Finally, to explore AA metabolism genes, AA genes were screened with a cut-off value of adjusted p < 0.1 and |log2FC| ≥ 0.28. To understand the expression of these genes in different cell types of heart tissue, gene expression and cell phenotypes were considered from an snRNA-seq study (GSE183852) (44). In this, control samples were filtered to identify the expression of screened AA metabolism genes in the donor heart cell types. Further, marker genes were identified in each cell type. The “FindMarkers” function in the Seurat package was used to accomplish this task with the cut-off values of adjusted p < 0.1, minimal percentage > 0.1, and log2FC ≥ 0.58. These marker genes were utilized to select the cell types significantly enriched within cardiomyopathies DEGs. The GSA method PAGE was employed to evaluate the significance of the DEGs against the cell-specific marker genes in the Piano (version 2.2.0) package in R (51, 52). Functional enrichment of cell-specific marker DEGs was performed using the online tool “g:Profiler” (https://biit.cs.ut.ee/gprofiler/) (54).
2.7. Identification of dysregulated marker genes interactions in the cell types
For human interactome data, PPI data (HPRD, MINT, IntAct) along with protein complex and kinase substrate data (CORUM, Phosphositeplus) were obtained from our previous study (55). Then, AA metabolism genes and cell type marker genes were mapped to the human interactome, and a subnetwork consisting of these genes was constructed. Network visualization was executed in Gephi (version 0.9.3).
3. Results
3.1. Gene expression profile in primary cardiomyopathies
We identified and selected seven studies fitting our inclusion criteria (see Supplementary Table S1 and Materials and methods), consisting of arrhythmogenic cardiomyopathy, dilated cardiomyopathy, hypertrophic cardiomyopathy and restrictive cardiomyopathy samples. Analyses were performed using 4 RNA-seq, one single-nucleus RNA-seq (snRNA-seq) and two microarray datasets. We performed sample variability analysis on normalized RNA-seq studies. The PCA and t-SNE analysis revealed variability in the samples of different datasets. Attributes like demography, genetic differences and tissue biopsies influence the above variability (Figures 1A,B). Since each dataset independently consists of control and disease samples, these variations can be ignored. For the RNA-seq differential gene expression, the negative binomial generalized linear model was used (see Materials and methods). We employed the empirical Bayes method on the generalized linear model for the microarray datasets DEGs. This analysis led to the identification of 4,158, 5,822, 3,048 and 1,655 DEGs in ACM, DCM, HCM and RCM RNA-seq studies. We screened KEGG metabolic genes amongst these DEGs and found 527, 644, 346 and 150 differentially regulated metabolic genes (see Figure 1C). Gene expression results indicate that DCM gene expression differed from other primary cardiomyopathies (see Figure 1D).
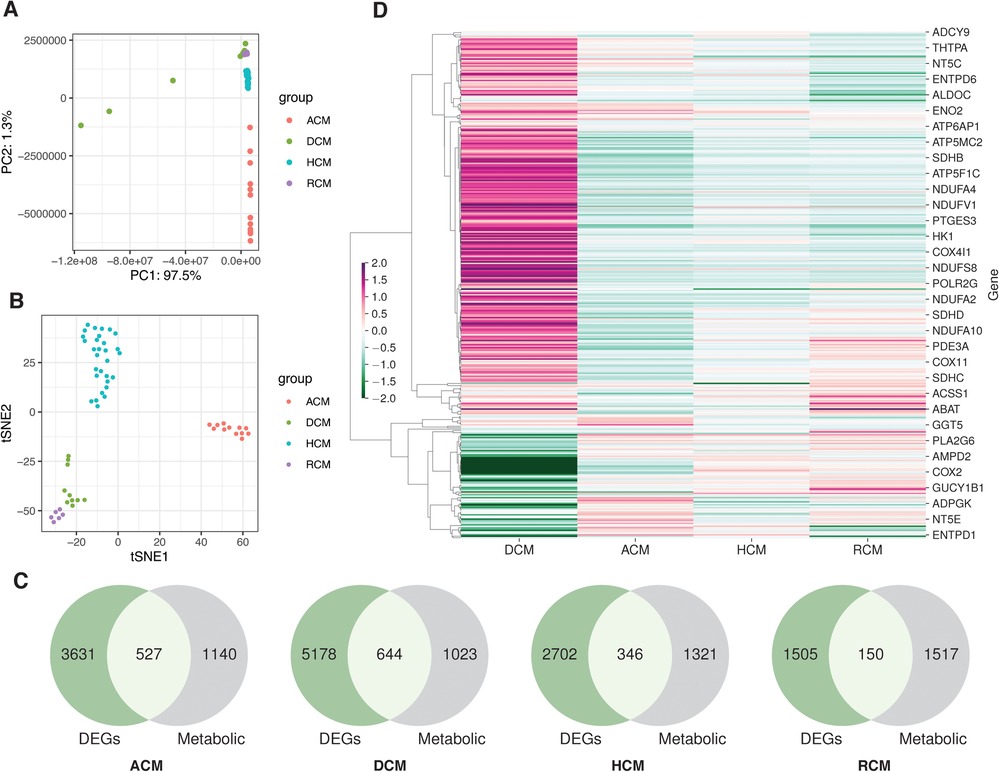
Figure 1. The transcriptome datasets in primary cardiomyopathies. (A) PCA plot of disease and donor samples in ACM, DCM, HCM and RCM. (B) The t-SNE plot of disease and donor samples in ACM, DCM, HCM and RCM. (C) Venn diagrams showing DEGs and metabolic genes in each cardiomyopathy. (D) A heatmap showing the metabolic genes’ expression in ACM, DCM, HCM and RCM phenotypes.
3.2. The metabolic pathways are significantly dysregulated in cardiomyopathies
To further understand the role of metabolic genes in cardiomyopathies, we performed gene set analysis (GSA) on RNA-seq and microarray studies. The p-values from the differential expression analysis of each dataset were ranked to estimate the gene-level statistics (see Materials and methods). The KEGG pathway metabolic gene signature was used to identify the dysregulated pathways. The GSA revealed widespread alterations of glycolysis/gluconeogenesis, TCA cycle, oxidative phosphorylation, and riboflavin, thiamine, purine and arachidonic acid metabolism (see Figure 2).
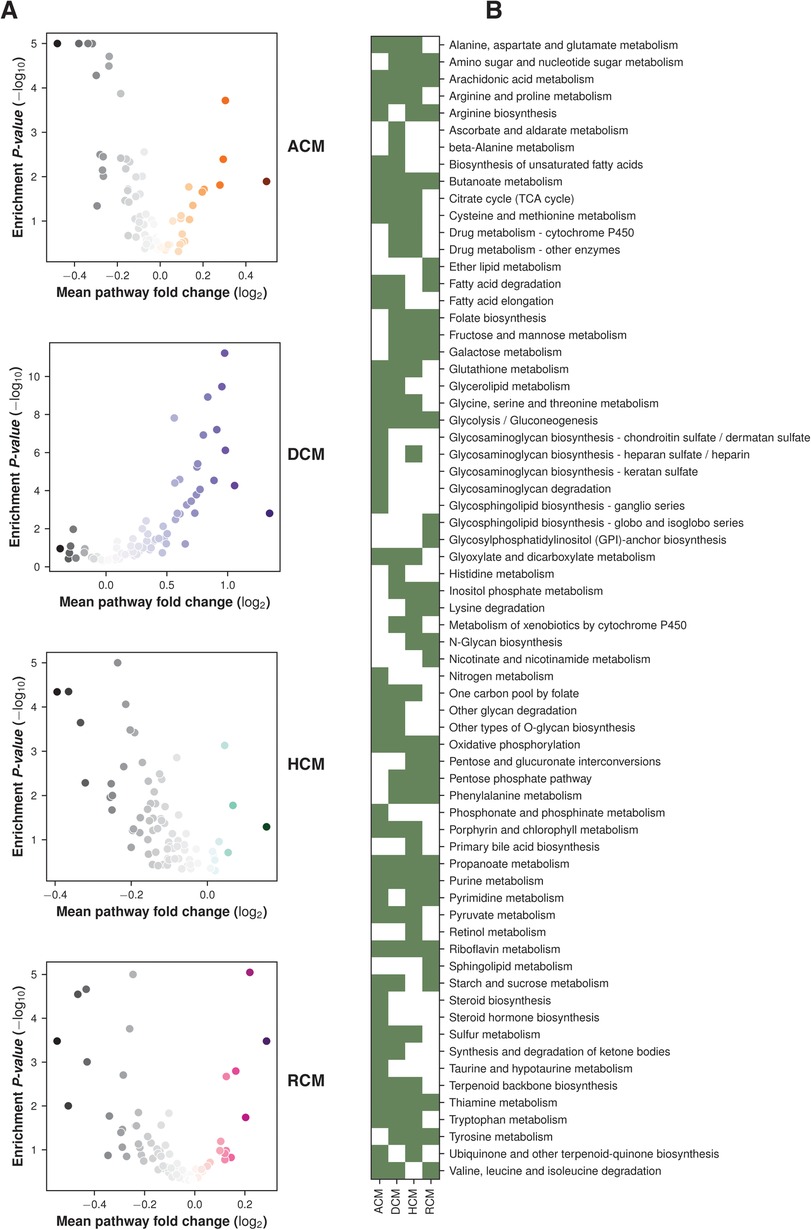
Figure 2. GSA enrichment of KEGG pathways in primary cardiomyopathies. (A) Volcano plots showing pathways enrichment and fold change in primary cardiomyopathies. (B) Heatmap showing major pathway perturbation in ACM, DCM, HCM and RCM phenotypes.
Oxidative phosphorylation metabolism, a mitochondrial-associated process, was significantly downregulated in ACM, HCM and RCM but not in DCM. It was upregulated in the DCM phenotype. Similarly, another primary energy pathway, glycolysis/gluconeogenesis metabolism, was also downregulated in ACM, HCM and RCM and upregulated in DCM. Nucleotide-specific purine metabolism was downregulated in all phenotypes except DCM. In addition, the pathway related to the amino acid arginine biosynthesis was significantly upregulated in DCM but significantly downregulated in other primary cardiomyopathies. Pathway related to co-factor metabolism, riboflavin was upregulated in DCM but downregulated in ACM, HCM and RCM. We found this surprising; it is possible that impact on metabolic pathways in DCM may vary compared to HCM or other cardiomyopathies due to its hypo-contractile nature. While some studies have indicated a downregulation of oxidative phosphorylation in DCM (56), a previous study by Verdonschot et al. reported an upregulated oxidative phosphorylation in DCM caused by truncating titin variants (TTNtv) (57). Interestingly, inositol phosphate metabolism was upregulated in HCM and RCM but downregulated in DCM. Our analysis also indicated significant downregulation of fatty acid precursor, arachidonic acid metabolism. Surprisingly, it was downregulated in the HCM and RCM phenotypes but upregulated in the ACM and DCM phenotypes (see Figure 3). A previous study highlighted that arachidonic acid (AA) induces mitochondrial depolarization in isolated myocytes by a lipoxygenase (LOX)-dependent mechanism and that such depolarization might contribute to arrhythmogenesis (58). ACM's pathological feature results from the replacing the myocardium with fibrous and fatty (fibro-fatty) tissue (59). In light of this, AA metabolism requires further investigation. As observed in the literature, major metabolic pathways are disrupted in almost all cardiomyopathies, either upregulated or downregulated, depending upon the nature of cardiomyopathy (60). Our results concluded that DCM displayed opposite trends compared to the other primary cardiomyopathies in critical metabolic pathways, such as glycolysis/gluconeogenesis, oxidative phosphorylation, riboflavin, thiamine, and purine metabolism.
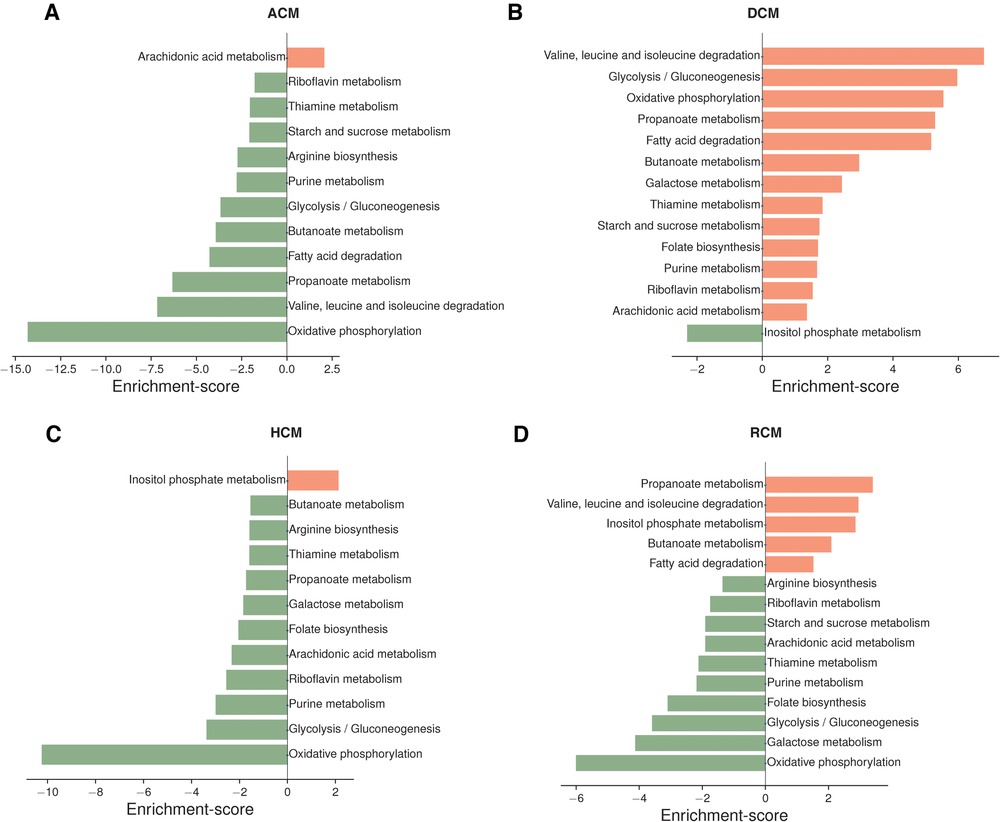
Figure 3. Top pathways enriched in ACM, DCM, HCM and RCM phenotypes (A–D). Pathways were ranked based on enrichment scores calculated by the PAGE. Pathways enriched with up-regulated genes are marked in saffron color, and those enriched with down-regulated genes are highlighted in green.
3.3. Arachidonic acid metabolism is perturbed in primary cardiomyopathies
We focused on arachidonic acid metabolism from previous results due to its involvement in all cardiomyopathies and its less studied nature in cardiomyopathies. We chose independent studies from microarray datasets to validate AA metabolism disruption in the ACM, DCM, and HCM human heart tissues. After GSA on validation datasets, we observed that ∼76% of KEGG pathways enriched in the original RNA-seq datasets were also significantly enriched in microarray sets. In the validation set, too, AA was dysregulated across ACM, DCM and HCM phenotypes (see Supplementary Figure S1). As referred in the previous result that arachidonic acid (AA) induces mitochondrial depolarization via lipoxygenase (LOX), which may be attributed to arrhythmogenesis (58). Therefore, we focused on DEGs to further understand the molecular players in AA metabolism. We identified 25 AA metabolism DEGs in primary cardiomyopathies (see Table 1). Genes like AKR1C3, CYP2J2, EPHX2, LTC4S, PLA2G2A, PLA2G5, PTGDS and PTGIS were particularly interesting (see Figure 4 and Supplementary Figure S2). The aldo/keto reductase superfamily protein-coding gene AKR1C3 was downregulated in DCM and HCM but upregulated in ACM. A recent work suggests that AKR1C3 might be involved in the process of ferroptosis in cardiac myocytes and may act as a bio-marker for Acute Myocardial Infarction (AMI) (61). The cytochrome P450 superfamily protein-coding gene CYP2J2 was upregulated in ACM, DCM and HCM phenotypes. CYP2J2 coding for cytochrome P-450 2J2 is reported to be implicated in hypertension and coronary artery disease (CAD) (62). The epoxide hydrolase family protein-coding gene EPHX2 encoding sEH was upregulated in DCM and RCM but downregulated in ACM. This gene was shown to be associated with heart failure in a rat model of heart failure (63). The MAPEG family protein-coding gene LTC4S was downregulated in HCM while being upregulated in ACM and DCM. Nobili et al. highlighted that LTC4S antagonists could protect against a hypoxic heart (64). The phospholipase A2 family (PLA2) protein-coding gene PLA2G2A was upregulated in DCM and downregulated in HCM and RCM. At the same time, the PLA2 family protein-coding gene PLA2G5 and glutathione-independent prostaglandin D synthase enzyme coding-protein PTGDS were downregulated in ACM, HCM and RCM. Phospholipase A2 (PLA2) enzyme is attributed to as a risk factor for coronary heart disease (65). The enzyme coded by PTGDS catalyzes the conversion of prostaglandin H2 to prostaglandin D2. It is recognized as a circulating marker for cardiovascular injuries and the severity of CAD (66). Lastly, the cytochrome P450 superfamily protein-coding gene PTGIS was upregulated in ACM, while being downregulated in DCM and HCM (see Figure 5A). The endogenous expression of the PGIS gene product is reported to have a potentially protective effect against hereditary pulmonary arterial hypertension (HPAH) (67). In summary, AA genes are significantly dysregulated in primary cardiomyopathies.
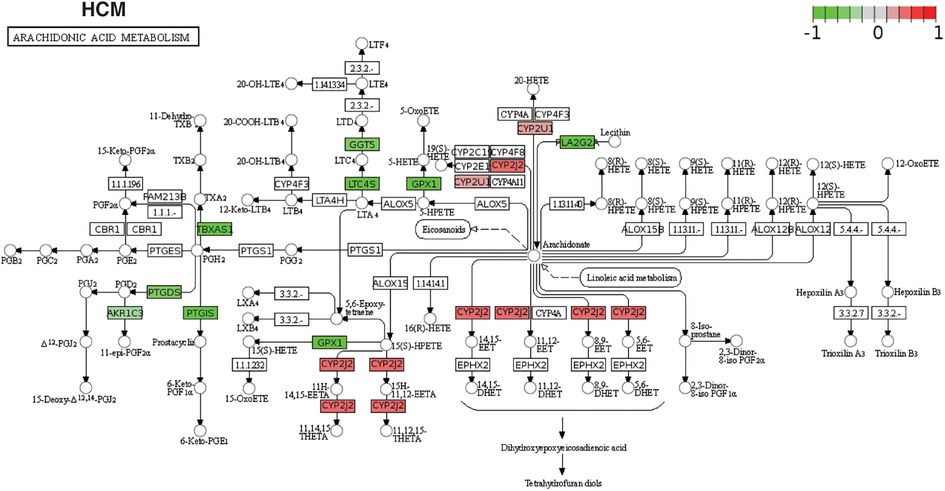
Figure 4. The KEGG pathway map of AA metabolism DEGs. Differentially expressed AA metabolism genes are mapped to the KEGG pathway map in individual studies (in this case, HCM). The red color on the map represents the up-regulated genes, and green marks the down-regulated genes in the study.
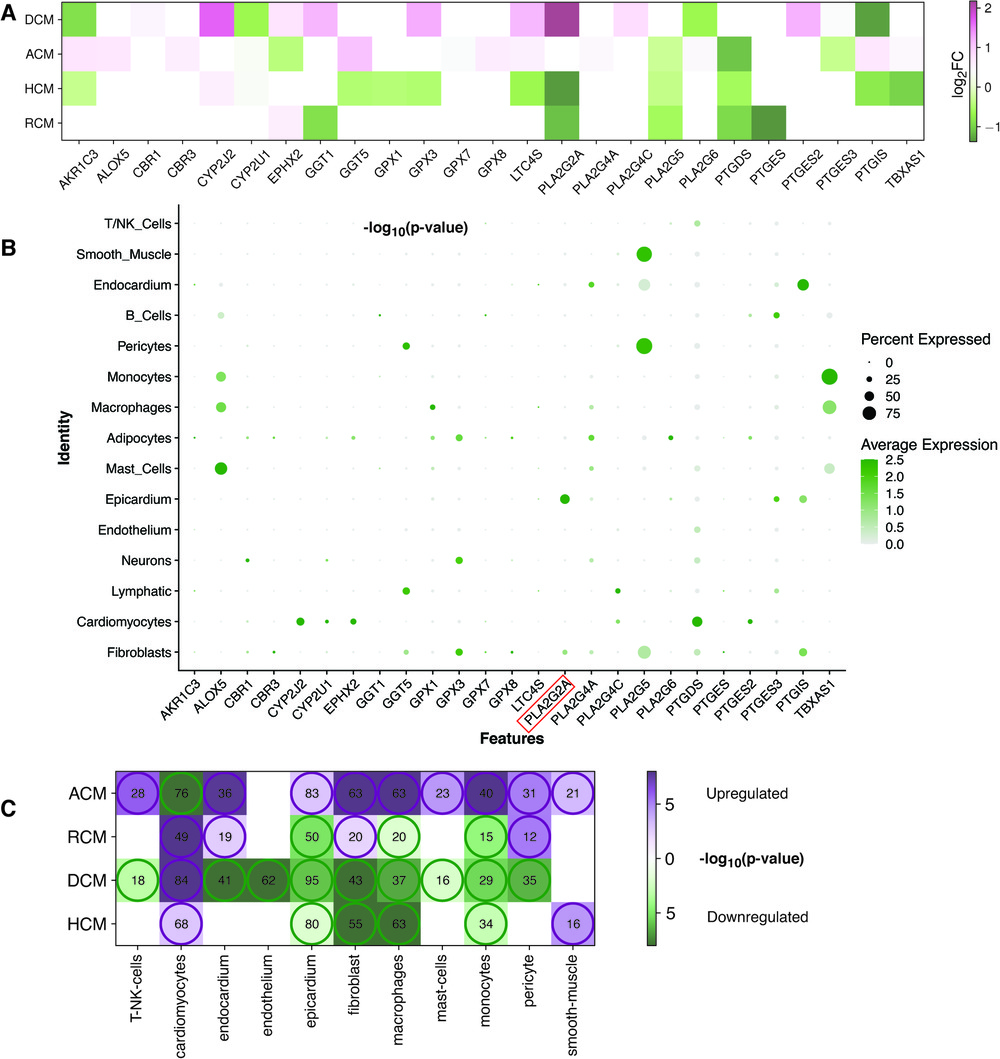
Figure 5. AA DEGs genes and their expression in heart cell types. (A) A heatmap showing the AA metabolism DEGs in the individual cardiomyopathy gene expression analyses (ACM, DCM, HCM and RCM). (B) The bubble plot shows the expression of these AA metabolism DEGs in different cell types of the heart tissue from the analysis of the snRNA-Seq dataset (GSE183852). (C) This heatmap represents the up-regulated or down-regulated enrichment of cell types (violet representing up-regulated and green showing the down-regulated) and the size of their marker genes found in DEGs of ACM, DCM, HCM and RCM phenotypes.
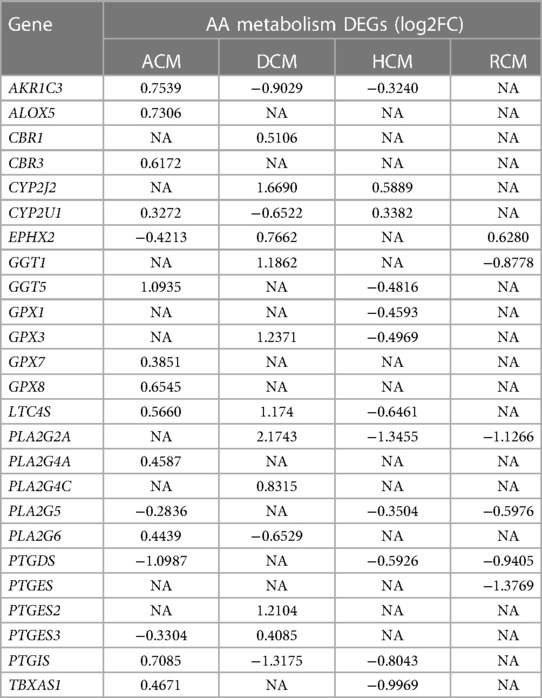
Table 1. A list of differentially expressed genes in arachidonic acid (AA) metabolism in arrhythmogenic, dilated, hypertrophic and restrictive cardiomyopathies.
3.4. Arachidonic acid metabolism regulators cell types and marker genes in these cells
Next, we performed meta-analysis of the expression of the previous 25 AA metabolism genes in heart cell types. We utilized the cell types annotated in the human heart from an earlier single-nucleus RNA sequencing (snRNA-seq) study (GSE183852) (44). These cells were grouped into 15 clusters. Earlier mentioned genes AKR1C3, CYP2J2, EPHX2, LTC4S, PLA2G2A, PLA2G5, PTGDS and PTGIS were predominantly expressed in cardiomyocytes, epicardium, smooth muscle, endocardium, pericytes and fibroblasts (see Figure 5B). Inflammation remains a key player in heart failure (HF) pathogenesis, in both acute and chronic HF. Our analysis revealed multiple immune and inflammation inducing cell types were associated with AA metabolism. Studies have highlighted the role of AA metabolism in inflammation (39, 68). Interestingly, genes common in up to two phenotypes were expressed in more immune cells like mast cells, macrophages and monocytes. The lipoxygenase gene family member ALOX5, the glutathione peroxidase family member GPX1 and the cytochrome P450 superfamily member TBXAS1 belong to this category. The glutathione peroxidase family members GPX3, PLA2G5 and PTGIS were expressed in a significant number of fibroblasts (greater than 50%). The presence of AKR1C3 and LTC4S was negligible in all the major cell types of the heart.
T/NK cells, cardiomyocytes, endocardium, endothelium, epicardium, fibroblast, macrophages, mast cells, myocytes, pericytes and smooth muscles were further explored for marker gene identification. A few AA metabolic genes were present in multiple cell types but were left out as marker genes in all cell types as per the marker definition strategy. PLA2G2A gene is such an example that was expressed in fibroblast and epicardium. However, due to its higher expression in the epicardium, it was missed as a marker in fibroblast. Once the marker genes in the above cell types were identified, GSA was carried out on these genes set to inquire enrichment of these markers within upregulated or downregulated genes of ACM, DCM, HCM and RCM (see Figure 5C, Supplementary Tables S2–S5). The cardiomyocytes marker genes were enriched within upregulated genes of DCM, HCM and RCM (84, 68, 49) but within downregulated genes of ACM (76) (enrichment p-values < 0.1 and gene adjusted p < 0.1 & |log2FC| ≥ 0.28). In comparison, marker genes of fibroblasts were enriched within upregulated genes of ACM and RCM (63, 20) and within downregulated genes of DCM and HCM phenotypes (enrichment p-values < 0.1 and gene adjusted p < 0.1 & |log2FC| ≥ 0.28). Moreover, the marker genes of pericyte and endocardium were enriched within upregulated genes of ACM and RCM but within downregulated genes of DCM. Likewise, the smooth muscle marker genes were enriched within upregulated genes of ACM and RCM only. In this, a total of 21 and 16 marker genes were found to be upregulated (enrichment p-values < 0.1 and gene adjusted p < 0.1 & |log2FC| ≥ 0.28). The marker genes of the epicardium, macrophages and monocytes were enriched within downregulated genes of DCM, HCM and RCM and opposite in ACM phenotype. The T/NK cells and mast cell marker genes were enriched in the upregulated genes of ACM but enriched within downregulated genes of DCM. Lastly, endothelium marker genes were enriched within upregulated genes of DCM (62) only (enrichment p-values < 0.1 and gene adjusted p < 0.1 & |log2FC| ≥ 0.28). Overall, expression of AA genes in heart tissue cell types and enrichment analysis on marker genes of these cell types demonstrates that AA metabolic genes are expressed in cardiomyocytes, fibroblast and immune cells, and a large proportion of cell type marker genes are dysregulated in all primary cardiomyopathies.
3.5. Network analysis reveals association of key arachidonic acid metabolism regulator PLA2G2A and fibroblast marker genes
We next performed in-depth analysis of the significantly enriched marker genes within DEGs of primary cardiomyopathies. Gene ontology analysis of cardiomyocytes and fibroblast cell type enriched marker genes revealed biological processes associated with muscle contraction and extracellular matrix organization, confirming the relevance of these markers (see Supplementary Figures S3–S5). The AA metabolism DEGs and cell type enriched marker genes were mapped to human interactome to understand their underlying association (see Materials and methods). Cardiac fibrosis is a significant player in cardiomyopathies. Therefore, we examined fibroblast cell types in this analysis. The fibroblast cell type dysregulated marker genes were associated with the PLA2G2A gene of AA metabolism in ACM, DCM and HCM (see Figure 6 and Supplementary Figures S6–S8). In the RCM phenotype, the interaction was missing, possibly due to much fewer overall DEGs in RCM. Network analysis of dysregulated HCM marker genes revealed that AA gene PLA2G2A interacts with DCN, which interacts with FN1, COL4A4, SLIT2, EGFR, GSN, COL1A2, ROBO1, COL4A1 and ELN that are primarily involved in extracellular matrix (ECM) organization and heart development. Similarly, DCM-specific dysregulated marker genes network uncovered PLA2G2A interaction with DCN, which was directly linked to FBN1 and COL4A1. These genes are primarily involved in ECM organization and anatomical structure morphogenesis. Collectively, network analysis demonstrates that the phospholipase A2 family gene PLA2G2A influences fibroblasts and may be involved in fibrosis during cardiomyopathy.
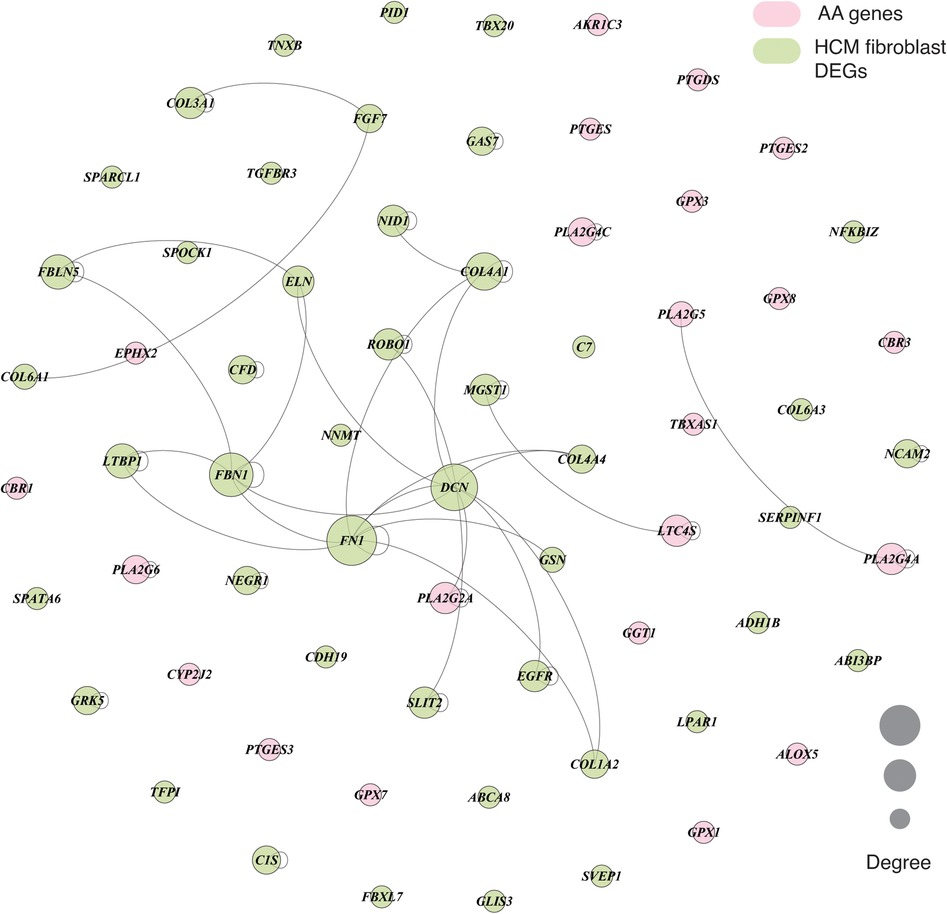
Figure 6. A graph showing the interaction between AA metabolism DEGs and cell type marker DEGs in fibroblast cell type in HCM phenotype. The interaction network consists of protein-protein, protein complex and substrate-kinase interactions. In the graph, node size represents the degree of the individual nodes, and pink and light green colors highlight the AA DEGs and fibroblast marker genes, respectively.
4. Discussion
Primary cardiomyopathies like ACM, DCM, HCM and RCM are heterogenous heart muscle diseases with poor prognosis, and are a leading cause of sudden cardiac death (6–12). The current study accomplishes GSA on the transcriptome profile to understand metabolic pathways perturbation across primary cardiomyopathies. Among the GSA-enriched KEGG metabolic pathways between cardiomyopathy and normal samples, glycolysis/gluconeogenesis, oxidative phosphorylation, nucleotides metabolism, amino acid biosynthesis, cofactor metabolism, and fatty acid precursor arachidonic acid metabolism were statistically significant. Previous studies have reported oxidative phosphorylation, glycolysis and fatty acid metabolism shift in individual cardiomyopathies (23, 24). In this work, we carried out an integrated analysis of AA metabolic alteration across cardiomyopathies (see Figure 7).
Arachidonic acid (AA) metabolism is an important mediator of cardiovascular processes such as fibrosis and inflammation. However, its role in cardiomyopathies is less explored (31, 32). Arachidonic acid is a free fatty acid that gets transformed into biologically active mediators by COX, LOX, and CYP450 epoxygenase enzymes (31). These mediators allow AA to participate in complex cardiovascular functions (32). Our study highlighted the statistically significant dysregulation of AA metabolism in all primary cardiomyopathies. This was also found to be consistent in the validation set. Further, AA metabolic DEGs in our study were expressed in heart tissue cell types like cardiomyocytes, fibroblasts, epicardium, endocardium, smooth muscle, and pericytes and immune cells such as macrophages, monocytes and mast cells at the single-cell level. Our analysis showed that the AA metabolic genes ALOX5 and TBXAS1 were present in macrophages, monocytes and mast cells. However, these were not significant in a majority of cardiomyopathies. These results point to a potential role of AA metabolism in modulating fibrosis and inflammation in cardiomyopathies.
Cardiomyopathies are generally characterized by cardiac fibrosis (69). Cardiac fibrosis results from the dysregulation of the balance between the synthesis and degradation of extracellular matrix (ECM) proteins (69, 70). It has been suggested that fibrosis primarily manifests in the interstitial space in pressure-induced cardiac remodeling without cardiomyocyte deletion. Whereas volume overload-induced cardiac remodeling leads to a significant reduction in cardiomyocytes, and fibrosis is triggered, especially in myocardial infarction (MI) (39). Fibroblast cells synthesize the ECM proteins and get involved in tissue repair and remodeling after injury in heart tissues during cardiomyopathies (69–71). We investigated whether AA metabolism genes were associated with dysregulated fibroblast marker genes. Our analysis showed the interaction between the AA metabolism gene PLA2G2A and the fibroblast marker gene DCN, which was linked to many other fibroblast marker genes. Fibroblast marker genes' gene ontology enrichment analysis confirmed their role in ECM organization. Decorin (DCN) belongs to chondroitin sulfate proteoglycan and has been shown to exhibit antifibrotic effects in the mouse model (72). In short, our analysis demonstrated that AA metabolism genes interact with the ECM proteins, such as decorin and others, directly or indirectly and might influence cardiac fibrosis in primary cardiomyopathies.
Epoxyeicosatrienoic acids (EETs) are metabolites that arise from arachidonic acid (AA) through the activity of CYP epoxygenases. Successive studies have revealed that EETs protect the heart against inflammation, cardiac remodeling, endothelial dysfunction, and fibrosis (41, 73). EETs have been reported to be nitric oxide (NO) independent vasodilators in vivo (74). Other analyses suggest that EETs act as an endothelium-derived hyperpolarizing factor (EDHF), inducing vasodilation of vascular smooth muscle by activating Ca2+-activated K+ channels (75). CYP2J2, CYP2C8, and CYP2C9 enzymes are major sources of synthesis of EETs (37). Our analysis also showed upregulation of CYP2J2 gene expression in DCM as well as HCM. Notably, CYP2J2 is the only human CYP2J2 epoxygenase and is highly expressed in the heart and endothelium (38). CYP2J2 catalyzes AA into four regioisomeric EETs, including 5,6-, 8,9-, 11,12-, and 14,15-EET (39). It will be worthwhile to explore CYPJ2J2 further in cardiomyopathies.
This study has limitations due to heterogeneity in samples aggregated from different transcriptome studies. Sequencing methodology, environment and sequencing depth are the main challenges in an integrative analysis. Quantification of RCM RNA-seq reads was missing for many genes due to less coverage of these genes. Next, this study only demonstrates statistically perturbed pathways, important DEGs and heart cell types and their interactions in each cardiomyopathy through computational analysis. Experimental validation in an in vitro or in vivo system was not performed due to the limitation of resources. Lastly, ACM, DCM, HCM and RCM are highly heterogeneous diseases. Thus, their classification may vary substantially in the population.
Regardless of the limitations, the current study provides new insights into cardiomyopathy research. This work recognizes the arachidonic acid metabolism as a potential regulator of all major primary cardiomyopathies. Apart from this, the study also demonstrates the expression of AA metabolism genes in major heart-specific cell types and immune cells, facilitating a better interpretation of its potential roles in the disease. The interaction between the products of AA gene PLA2G2A and fibroblast marker gene DCN could present a promising therapeutic target to regulate cardiac fibrosis.
5. Conclusion
Arachidonic acid metabolism is perturbed in primary cardiomyopathies. AA metabolism genes are expressed in most heart cell types and immune cells, influencing the immune activation and cardiac fibrosis. The association of the dysregulated AA gene PLA2G2A with fibroblast marker gene DCN may be an important factor related to fibrosis.
Data availability statement
Existing datasets are available in a publicly accessible repository: Publicly available datasets were analyzed in this study. This data can be found here: http://caps.ncbs.res.in/download/cardiomyo_transcriptome.
Author contributions
RS and PC conceptualized the study. PC integrated data, performed data analysis and visualization. PC wrote the manuscript and RS wrote the final draft of manuscript. All authors contributed to the article and approved the submitted version.
Funding
RS acknowledges funding and support provided by JC Bose Fellowship (JBR/2021/000006) from Science and Engineering Research Board, India and Bioinformatics Centre Grant funded by Department of Biotechnology, India (BT/PR40187/BTIS/137/9/2021). RS would also like to thank Institute of Bioinformatics and Applied Biotechnology for the funding through her Mazumdar-Shaw Chair in Computational Biology (IBAB/MSCB/182/2022).
Acknowledgments
We acknowledge the contributions from the publicly available databases as the source of original data in our study. We thank CAPS lab members for their technical help and discussions. This work was inspired by the heart ailments of our collaborator, the late Prof N. Srinivasan of Indian Institute of Science. The authors thank NCBS (TIFR) for infrastructural facilities. PC would like to thank NCBS-TIFR (Department of Atomic Energy, India) for the fellowship.
In memoriam
This paper is dedicated to and in the memory of our late collaborator, Prof. N Srinivasan.
Conflict of interest
The authors declare that the research was conducted in the absence of any commercial or financial relationships that could be construed as a potential conflict of interest.
Publisher's note
All claims expressed in this article are solely those of the authors and do not necessarily represent those of their affiliated organizations, or those of the publisher, the editors and the reviewers. Any product that may be evaluated in this article, or claim that may be made by its manufacturer, is not guaranteed or endorsed by the publisher.
Supplementary material
The Supplementary Material for this article can be found online at: https://www.frontiersin.org/articles/10.3389/fcvm.2023.1110119/full#supplementary-material.
References
1. Maron BJ, Towbin JA, Thiene G, Antzelevitch C, Corrado D, Arnett D, et al. Contemporary definitions and classification of the cardiomyopathies. Circulation. (2006) 113:1807–16. doi: 10.1161/CIRCULATIONAHA.106.174287
2. Chien KR. Genotype, phenotype: upstairs, downstairs in the family of cardiomyopathies. J Clin Invest. (2003) 111:175–8. doi: 10.1172/JCI17612
3. Maron BJ, Gardin JM, Flack JM, Gidding SS, Kurosaki TT, Bild DE. Prevalence of hypertrophic cardiomyopathy in a general population of young adults. Circulation. (1995) 92:785–9. doi: 10.1161/01.CIR.92.4.785
4. Hershberger RE, Hedges DJ, Morales A. Dilated cardiomyopathy: the complexity of a diverse genetic architecture. Nat Rev Cardiol. (2013) 10:531–47. doi: 10.1038/NRCARDIO.2013.105
5. Basso C, Corrado D, Marcus FI, Nava A, Thiene G. Arrhythmogenic right ventricular cardiomyopathy. Lancet. (2009) 373:1289–300. doi: 10.1016/S0140-6736(09)60256-7
6. McKenna WJ, Judge DP. Epidemiology of the inherited cardiomyopathies. Nat Rev Cardiol. (2020) 18(1):22–36. doi: 10.1038/s41569-020-0428-2
7. Olivotto I, D’Amati G, Basso C, van Rossum A, Patten M, Emdin M, et al. Defining phenotypes and disease progression in sarcomeric cardiomyopathies: contemporary role of clinical investigations. Cardiovasc Res. (2015) 105:409–23. doi: 10.1093/CVR/CVV024
8. Lehman SJ, Crocini C, Leinwand LA. Targeting the sarcomere in inherited cardiomyopathies. Nat Rev Cardiol. (2022) 19(6):353–63. doi: 10.1038/s41569-022-00682-0
9. Bhatnagar A. Environmental determinants of cardiovascular disease. Circ Res. (2017) 121:162. doi: 10.1161/CIRCRESAHA.117.306458
10. Ismahil MA, Hamid T, Haberzettl P, Gu Y, Chandrasekar B, Srivastava S, et al. Chronic oral exposure to the aldehyde pollutant acrolein induces dilated cardiomyopathy. Am J Physiol Heart Circ Physiol. (2011) 301:H2050–60. doi: 10.1152/AJPHEART.00120.2011
11. Djoussé L, Driver JA, Gaziano JM. Relation between modifiable lifestyle factors and lifetime risk of heart failure: the Physicians’ health study I. J Am Med Assoc. (2009) 302:394. doi: 10.1001/JAMA.2009.1062
12. Piano MR. Alcoholic cardiomyopathy: incidence, clinical characteristics, and pathophysiology. Chest. (2002) 121:1638–50. doi: 10.1378/CHEST.121.5.1638
13. Neubauer S. The failing heart: an engine out of fuel. N Engl J Med. (2007) 356:1140–51. doi: 10.1056/NEJMRA063052
14. Taegtmeyer H. Cardiac metabolism as a target for the treatment of heart failure. Circulation. (2004) 110:894–6. doi: 10.1161/01.CIR.0000139340.88769.D5
15. Haas J, Frese KS, Sedaghat-Hamedani F, Kayvanpour E, Tappu R, Nietsch R, et al. Energy metabolites as biomarkers in ischemic and dilated cardiomyopathy. Int J Mol Sci. (2021) 22:1999. doi: 10.3390/IJMS22041999
16. Volani C, Rainer J, Hernandes VV, Meraviglia V, Pramstaller PP, Smárason SV, et al. Metabolic signature of arrhythmogenic cardiomyopathy. Metabolites. (2021) 11:195. doi: 10.3390/METABO11040195/S1
17. Sacchetto C, Sequeira V, Bertero E, Dudek J, Maack C, Calore M. Metabolic alterations in inherited cardiomyopathies. J Clin Med. (2019) 8(12):2195. doi: 10.3390/JCM8122195
18. Marcinkiewicz-Siemion M, Ciborowski M, Kretowski A, Musial WJ, Kaminski KA. Metabolomics—a wide-open door to personalized treatment in chronic heart failure? Int J Cardiol. (2016) 219:156–63. doi: 10.1016/J.IJCARD.2016.06.022
19. Rankin NJ, Preiss D, Welsh P, Sattar N. Applying metabolomics to cardiometabolic intervention studies and trials: past experiences and a roadmap for the future. Int J Epidemiol. (2016) 45:1351–71. doi: 10.1093/IJE/DYW271
20. Jørgenrud B, Jalanko M, Heliö T, Jääskeläinen P, Laine M, Hilvo M, et al. The metabolome in Finnish carriers of the MYBPC3-Q1061X mutation for hypertrophic cardiomyopathy. PLoS One. (2015) 10:e0134184. doi: 10.1371/JOURNAL.PONE.0134184
21. Alexander D, Lombardi R, Rodriguez G, Mitchell MM, Marian AJ. Metabolomic distinction and insights into the pathogenesis of human primary dilated cardiomyopathy. Eur J Clin Invest. (2011) 41:527–38. doi: 10.1111/J.1365-2362.2010.02441.X
22. van der Velden J, Tocchetti CG, Varricchi G, Bianco A, Sequeira V, Hilfiker-Kleiner D, et al. Metabolic changes in hypertrophic cardiomyopathies: scientific update from the working group of myocardial function of the European society of cardiology. Cardiovasc Res. (2018) 114:1273–80. doi: 10.1093/CVR/CVY147
23. Pei J, Schuldt M, Nagyova E, Gu Z, el Bouhaddani S, Yiangou L, et al. Multi-omics integration identifies key upstream regulators of pathomechanisms in hypertrophic cardiomyopathy due to truncating MYBPC3 mutations. Clin Epigenetics. (2021) 13:1–20. doi: 10.1186/S13148-021-01043-3/FIGURES/6
24. Sweet ME, Cocciolo A, Slavov D, Jones KL, Sweet JR, Graw SL, et al. Transcriptome analysis of human heart failure reveals dysregulated cell adhesion in dilated cardiomyopathy and activated immune pathways in ischemic heart failure. BMC Genomics. (2018) 19:1–14. doi: 10.1186/S12864-018-5213-9/FIGURES/7
25. Xiao Z, Dai Z, Locasale JW. Metabolic landscape of the tumor microenvironment at single cell resolution. Nat Commun. (2019) 10(1):1–12. doi: 10.1038/s41467-019-11738-0
26. Gaude E, Frezza C. Tissue-specific and convergent metabolic transformation of cancer correlates with metastatic potential and patient survival. Nat Commun. (2016) 7(1):1–9. doi: 10.1038/ncomms13041
27. Li X, Tang L, Deng J, Qi X, Zhang J, Qi H, et al. Identifying metabolic reprogramming phenotypes with glycolysis-lipid metabolism discoordination and intercellular communication for lung adenocarcinoma metastasis. Commun Biol. (2022) 5(1):1–13. doi: 10.1038/s42003-022-03135-z
28. Tarca AL, Bhatti G, Romero R. A comparison of gene set analysis methods in terms of sensitivity, prioritization and specificity. PLoS One. (2013) 8:e79217. doi: 10.1371/JOURNAL.PONE.0079217
29. Curtis RK, Orešič M, Vidal-Puig A. Pathways to the analysis of microarray data. Trends Biotechnol. (2005) 23:429–35. doi: 10.1016/J.TIBTECH.2005.05.011
30. Çakir T. Reporter pathway analysis from transcriptome data: metabolite-centric versus reaction-centric approach. Sci Rep. (2015) 5(1):1–10. doi: 10.1038/srep14563
31. Wang B, Wu L, Chen J, Dong L, Chen C, Wen Z, et al. Metabolism pathways of arachidonic acids: mechanisms and potential therapeutic targets. Signal Transduct Target Ther. (2021) 6(1):1–30. doi: 10.1038/s41392-020-00443-w
32. Levick SP, Loch DC, Taylor SM, Janicki JS. Arachidonic acid metabolism as a potential mediator of cardiac fibrosis associated with inflammation. J Immun. (2007) 178:641–6. doi: 10.4049/JIMMUNOL.178.2.641
33. Zhou Y, Khan H, Xiao J, Cheang WS. Effects of arachidonic acid metabolites on cardiovascular health and disease. Int J Mol Sci. (2021) 22:12029. doi: 10.3390/IJMS222112029
34. Simmons DL, Botting RM, Hla T. Cyclooxygenase isozymes: the biology of prostaglandin synthesis and inhibition. Pharmacol Rev. (2004) 56:387–437. doi: 10.1124/PR.56.3.3
35. Zhang J, Yang C, Qiu H, Yang J, Wu K, Ding S, et al. 14,15-EET involved in the development of diabetic cardiac hypertrophy mediated by PPARs. Prostaglandins Other Lipid Mediat. (2022) 159:106620. doi: 10.1016/J.PROSTAGLANDINS.2022.106620
36. Bender G, Schexnaydre EE, Murphy RC, Uhlson C, Newcomer ME. Membrane-dependent activities of human 15-LOX-2 and its murine counterpart: implications for murine models of atherosclerosis. J Biol Chem. (2016) 291:19413–24. doi: 10.1074/JBC.M116.741454
37. Zhang M, Shu H, Chen C, He Z, Zhou Z, Wang DW. Epoxyeicosatrienoic acid: a potential therapeutic target of heart failure with preserved ejection fraction. Biomed Pharmacother. (2022) 153:113326. doi: 10.1016/J.BIOPHA.2022.113326
38. Wu S, Moomaw CR, Tomer KB, Falck JR, Zeldin DC. Molecular cloning and expression of CYP2J2, a human cytochrome P450 arachidonic acid epoxygenase highly expressed in heart. J Biol Chem. (1996) 271:3460–8. doi: 10.1074/JBC.271.7.3460
39. Lai J, Chen C. The role of epoxyeicosatrienoic acids in cardiac remodeling. Front Physiol. (2021) 12:117. doi: 10.3389/FPHYS.2021.642470/BIBTEX
40. Rastaldo R, Paolocci N, Chiribiri A, Penna C, Gattullo D, Pagliaro P. Cytochrome P-450 metabolite of arachidonic acid mediates bradykinin-induced negative inotropic effect. Am J Physiol Heart Circ Physiol. (2001) 280:2823–32. doi: 10.1152/AJPHEART.2001.280.6.H2823/ASSET/IMAGES/LARGE/H40710863005.JPEG
41. Imig JD, Hammock BD. Soluble epoxide hydrolase as a therapeutic target for cardiovascular diseases. Nat Rev Drug Discovery. (2009) 8:794–805. doi: 10.1038/nrd2875
42. Ma K, Yang J, Shao Y, Li P, Guo H, Wu J, et al. Therapeutic and prognostic significance of arachidonic acid in heart failure. Circ Res. (2022) 130:1056–71. doi: 10.1161/CIRCRESAHA.121.320548
43. Sonnweber T, Pizzini A, Nairz M, Weiss G, Tancevski I. Arachidonic acid metabolites in cardiovascular and metabolic diseases. Int J Mol Sci. (2018) 19:3285. doi: 10.3390/IJMS19113285
44. Koenig AL, Shchukina I, Amrute J, Andhey PS, Zaitsev K, Lai L, et al. Single-cell transcriptomics reveals cell-type-specific diversification in human heart failure. Nat Cardiol Rev. (2022) 1(3):263–80. doi: 10.1038/s44161-022-00028-6
45. Patro R, Duggal G, Love MI, Irizarry RA, Kingsford C. Salmon provides fast and bias-aware quantification of transcript expression. Nat Methods. (2017) 14(4):417–9. doi: 10.1038/nmeth.4197
46. Love MI, Huber W, Anders S. Moderated estimation of fold change and dispersion for RNA-Seq data with DESeq2. Genome Biol. (2014) 15:1–21. doi: 10.1186/S13059-014-0550-8/FIGURES/9
47. Wald A. Tests of statistical hypotheses concerning several parameters when the number of observations is large. Trans Am Math Soc. (1943) 54:426–82. doi: 10.1090/S0002-9947-1943-0012401-3
48. Benjamini Y, Hochberg Y. Controlling the false discovery rate: a practical and powerful approach to multiple testing. J R Stat Soc Series B. (1995) 57:289–300. doi: 10.1111/J.2517-6161.1995.TB02031.X
49. Ritchie ME, Phipson B, Wu D, Hu Y, Law CW, Shi W. et al.Limma powers differential expression analyses for RNA-sequencing and microarray studies. Nucleic Acids Res. (2015) 43:e47–e47. doi: 10.1093/NAR/GKV007
50. Hao Y, Hao S, Andersen-Nissen E, Mauck WM, Zheng S, Butler A, et al. Integrated analysis of multimodal single-cell data. Cell. (2021) 184:3573–87.e29. doi: 10.1016/J.CELL.2021.04.048
51. Kim SY, Volsky DJ. PAGE: parametric analysis of gene set enrichment. BMC Bioinform. (2005) 6:144. doi: 10.1186/1471-2105-6-144
52. Väremo L, Nielsen J, Nookaew I. Enriching the gene set analysis of genome-wide data by incorporating directionality of gene expression and combining statistical hypotheses and methods. Nucleic Acids Res. (2013) 41:4378. doi: 10.1093/NAR/GKT111
53. Luo W, Pant G, Bhavnasi YK, Blanchard SG, Brouwer C. Pathview web: user friendly pathway visualization and data integration. Nucleic Acids Res. (2017) 45:W501–8. doi: 10.1093/NAR/GKX372
54. Raudvere U, Kolberg L, Kuzmin I, Arak T, Adler P, Peterson H, et al. g:Profiler: a web server for functional enrichment analysis and conversions of gene lists (2019 update). Nucleic Acids Res. (2019) 47:W191–8. doi: 10.1093/NAR/GKZ369
55. Chauhan PK, Sowdhamini R. Integrative network analysis interweaves the missing links in cardiomyopathy diseasome. Sci Rep. (2022) 12(1):1–11. doi: 10.1038/s41598-022-24246-x
56. Shao Z, Koh W, Ni Y, Li W, Agatisa-Boyle B, Merkurjev D, et al. RNA sequence analyses throughout the course of mouse cardiac laminopathy identify differentially expressed genes for cell cycle control and mitochondrial function. Sci Rep. (2020) 10(1):1–14. doi: 10.1038/s41598-020-63563-x
57. Verdonschot JAJ, Hazebroek MR, Derks KWJ, Barandiarán Aizpurua A, Merken JJ, Wang P, et al. Titin cardiomyopathy leads to altered mitochondrial energetics, increased fibrosis and long-term life-threatening arrhythmias. Eur Heart J. (2018) 39:864–73. doi: 10.1093/EURHEARTJ/EHX808
58. Haworth RA, Potter KT, Russell DC. Role of arachidonic acid, lipoxygenase, and mitochondrial depolarization in reperfusion arrhythmias. Am J Physiol Heart Circ Physiol. (2010) 299:165–74. doi: 10.1152/AJPHEART.00906.2009/ASSET/IMAGES/LARGE/ZH40071094200008.JPEG
59. Chelko SP, Keceli G, Carpi A, Doti N, Agrimi J, Asimaki A, et al. Exercise triggers CAPN1-mediated AIF truncation, inducing myocyte cell death in arrhythmogenic cardiomyopathy. Sci Transl Med. (2021) 13(581):eabf0891. doi: 10.1126/SCITRANSLMED.ABF0891/SUPPL_FILE/ABF0891_SM.PDF
60. Gaar-Humphreys KR, van den Brink A, Wekking M, Asselbergs FW, van Steenbeek FG, Harakalova M, et al. Targeting lipid metabolism as a new therapeutic strategy for inherited cardiomyopathies. Front Cardiovasc Med. (2023) 10:19. doi: 10.3389/FCVM.2023.1114459/BIBTEX
61. Liang J, Cao Y, He M, Li W, Huang G, Ma T, et al. AKR1C3 and its transcription factor HOXB4 are promising diagnostic biomarkers for acute myocardial infarction. Front Cardiovasc Med. (2021) 8:1049. doi: 10.3389/FCVM.2021.694238
62. Tantray JA, Reddy KP, Jamil K, Yerra SK. Role of cytochrome epoxygenase (CYP2J2) in the pathophysiology of coronary artery disease in south Indian population. Indian Heart J. (2019) 71:60–4. doi: 10.1016/J.IHJ.2018.11.011
63. Monti J, Fischer J, Paskas S, Heinig M, Schulz H, Gösele C, et al. Soluble epoxide hydrolase is a susceptibility factor for heart failure in a rat model of human disease. Nat Genet. (2008) 40(5):529–37. doi: 10.1038/ng.129
64. Nobili E, Salvado MD, Folkersen L, Castiglioni L, Kastrup J, Wetterholm A, et al. Cysteinyl leukotriene signaling aggravates myocardial hypoxia in experimental atherosclerotic heart disease. PLoS One. (2012) 7:e41786. doi: 10.1371/JOURNAL.PONE.0041786
65. Hurt-Camejo E, Camejo G, Peilot H, Öörni K, Kovanen P. Phospholipase A2 in vascular disease. Circ Res. (2001) 89:298–304. doi: 10.1161/HH1601.095598
66. Liao Y, Dong Z, Liao H, Chen Y, Hu L, Yu Z, et al. Lipid metabolism patterns and relevant clinical and molecular features of coronary artery disease patients: an integrated bioinformatic analysis. Lipids Health Dis. (2022) 21:1–12. doi: 10.1186/S12944-022-01696-W/FIGURES/6
67. Stearman RS, Cornelius AR, Lu X, Conklin DS, Del Rosario MJ, Lowe AM, et al. Functional prostacyclin synthase promoter polymorphisms impact in pulmonary arterial hypertension. Am J Respir Crit Care Med. (2014) 189:1110–20. doi: 10.1164/RCCM.201309-1697OC/SUPPL_FILE/DISCLOSURES.PDF
68. Schmelzer KR, Kubala L, Newman JW, Kim IH, Eiserich JP, Hammock BD. Soluble epoxide hydrolase is a therapeutic target for acute inflammation. Proc Natl Acad Sci U S A. (2005) 102:9772–7. doi: 10.1073/PNAS.0503279102/SUPPL_FILE/03279FIG6.PDF
69. Eijgenraam TR, Silljé HHW, de Boer RA. Current understanding of fibrosis in genetic cardiomyopathies. Trends Cardiovasc Med. (2020) 30:353–61. doi: 10.1016/J.TCM.2019.09.003
70. Davis J, Molkentin JD. Myofibroblasts: trust your heart and let fate decide. J Mol Cell Cardiol. (2014) 70:9–18. doi: 10.1016/J.YJMCC.2013.10.019
71. Hinderer S, Schenke-Layland K. Cardiac fibrosis: a short review of causes and therapeutic strategies. Adv Drug Deliv Rev. (2019) 146:77–82. doi: 10.1016/J.ADDR.2019.05.011
72. Yan W, Wang P, Zhao CX, Tang J, Xiao X, Wang DW. Decorin gene delivery inhibits cardiac fibrosis in spontaneously hypertensive rats by modulation of transforming growth factor-beta/smad and p38 mitogen-activated protein kinase signaling pathways. Hum Gene Ther. (2009) 20:1190–200. doi: 10.1089/HUM.2008.204
73. Qiu H, Li N, Liu JY, Harris TR, Hammock BD, Chiamvimonvat N. Soluble epoxide hydrolase inhibitors and heart failure. Cardiovasc Ther. (2011) 29:99–111. doi: 10.1111/J.1755-5922.2010.00150.X
74. Imig JD, Falck JR, Wei S, Capdevila JH. Epoxygenase metabolites contribute to nitric oxide-independent afferent arteriolar vasodilation in response to bradykinin. J Vasc Res. (2001) 38:247–55. doi: 10.1159/000051053
Keywords: cardiomyopathies, GSA, metabolism, transcriptome, heart failure, arachidonic acid
Citation: Chauhan PK and Sowdhamini R (2023) Transcriptome data analysis of primary cardiomyopathies reveals perturbations in arachidonic acid metabolism. Front. Cardiovasc. Med. 10:1110119. doi: 10.3389/fcvm.2023.1110119
Received: 28 November 2022; Accepted: 9 May 2023;
Published: 23 May 2023.
Edited by:
Chen Yao, National Institutes of Health (NIH), United StatesReviewed by:
Ralph Knöll, AstraZeneca, SwedenNazareno Paolocci, Johns Hopkins University, United States
© 2023 Chauhan and Sowdhamini. This is an open-access article distributed under the terms of the Creative Commons Attribution License (CC BY). The use, distribution or reproduction in other forums is permitted, provided the original author(s) and the copyright owner(s) are credited and that the original publication in this journal is cited, in accordance with accepted academic practice. No use, distribution or reproduction is permitted which does not comply with these terms.
*Correspondence: Ramanathan Sowdhamini bWluaUBuY2JzLnJlcy5pbg==