- Department of Endovascular Surgery, The First Affiliated Hospital of Zhengzhou University, Zhengzhou University, Zhengzhou, China
Background: Coronary artery stenosis occurs frequently in patients with carotid artery stenosis. We developed a clinical predictive model to investigate the clinical risk of asymptomatic obstructive coronary artery stenosis in patients with carotid artery stenosis ≥ 50%.
Methods: From January 2018 to January 2022, carotid stenosis patients hospitalized at the First Affiliated Hospital of Zhengzhou University's Department of Endovascular Surgery were subjected to a retrospective analysis of their clinical information and imaging results. Excluded criteria were patients with lacking data, symptomatic coronary stenosis, prior coronary artery bypass grafting, and coronary stent implantation. Patients were separated into case and control groups according to whether or not they had obstructive coronary stenosis. Independent predictors were screened using univariate and multivariate logistic regression, and their accuracy was confirmed using least absolute shrinkage and selection operator (LASSO) regression. A Nomogram prediction model was developed using the aforementioned filtered factors. The model's discrimination and specificity were evaluated using the receiver operating characteristic curve (ROC) and Hosmer-Lemeshow goodness-of-fit test. Internal validation employed the Bootstrap procedure. The clinical decision curve analysis (DCA) of the prediction model was developed to assess the clinical applicability of the model.
Results: The investigation included a total of 227 patients, of whom 132 (58.1%) had coronary artery stenosis. Hypertension, Grade I plaque, HbA1c ≥ 7.0%, MPV ≥ 9.2fl, and Fib ≥ 3.0 g/L were independent predictors, with OR values of (2.506, 0.219, 0.457, 1.876, 2.005), according to multivariate logistic regression. Risk factor screening and validation using lasso regression. The predictors chosen based on the optimal λ value are consistent with the predictors identified by multiple regression. The area under the ROC curve (AUC) of the model based on the above predictors was 0.701 (0.633–0.770), indicating that the model discriminated well. The calibration curve of the model closely matched the actual curve, and P > 0.05 in the Hosmer-Lemeshow goodness-of-fit test indicated the model's accuracy. The results of the DCA curve demonstrate the clinical applicability of the prediction model.
Conclusion: Hypertension, grade I plaque, HbA1c ≥ 7.0%, MPV ≥ 9.2 fl, and Fib ≥ 3.0 g/L are predictors of asymptomatic coronary stenosis in patients with carotid stenosis ≥50%. The diagnostic model is clinically applicable and useful for identifying patients at high risk.
Introduction
Stroke has an elevated level of morbidity and mortality and has become a serious hazard to the health of the elderly. According to accounts, carotid stenosis causes 25% to 30% of ischemic strokes (1). Atherosclerosis is the principal cause of carotid stenosis. Stenosis occurs most frequently at the bifurcation of the common carotid, the origin of the internal carotid, and the siphon (2). Effective early treatment of carotid stenosis can reduce the incidence of ischemic stroke. Central interventions included endarterectomy of the carotid artery, stenting of the carotid artery, and drug therapy (3). Atherosclerosis has a variety of effects and can accumulate in both large and medium arteries. Patients with carotid artery stenosis and coronary artery stenosis are not uncommon. Research have demonstrated that patients with both carotid artery stenosis and coronary artery stenosis have a substantially increased risk of acute cardiovascular disease during surgery (4). Compared to symptomatic coronary stenosis, asymptomatic coronary stenosis has an elevated likelihood of misdiagnosis due to the absence of typical symptoms. A number of studies have investigated accurate predictors of asymptomatic coronary artery stenosis in patients. A retrospective study revealed that the thickness, extent, and complexity of aortic arch lesions were independent risk factors for asymptomatic coronary stenosis greater than 50% (5). In addition, patients with insulin resistance are more likely to have asymptomatic coronary artery stenosis ≥ 50% and have a positive correlation with the number and severity of coronary artery stenosis (6). The purpose of this study was to retrospectively analyze the clinical data of patients with carotid artery stenosis ≥ 50% in our center, to investigate the related predictive factors of asymptomatic obstructive coronary stenosis, and to establish a nomogram prediction model in order to provide support for early detection of occult coronary disease in patients with carotid stenosis.
Materials and methods
Patients and data collection
This survey included carotid artery stenosis patients admitted to the Department of Endovascular Surgery at the First Affiliated Hospital of Zhengzhou University between January 2018 and January 2022. By carotid ultrasound and carotid computed tomography angiography, a total of 513 patients with unilateral or bilateral carotid stenosis ≥ 50% were identified. Exclusion criteria included 45 patients with symptomatic coronary artery stenosis, 26 patients with a history of coronary stent implantation or coronary artery bypass grafting, 114 patients without carotid contrast-enhanced ultrasonography (CEUS), and 101 patients without coronary angiography. Finally, 227 patients in total met the inclusion criteria. They were split into a case group and a control group based on whether the degree of coronary artery stenosis exceeded 50%. Figure 1 shows the study's flowchart. The study was approved by the local ethics committee and was conducted in accordance with the 1964 Declaration of Helsinki.
The electronic medical record system was queried to obtain baseline clinical data, laboratory tests, and imaging investigations. Patient characteristics including age, gender, BMI, diabetes mellitus (DM), essential hypertension, and smoking and alcohol history. Laboratory examination indexes including hemoglobin A1c (HbA1c), hematocrit (HCT), lymphocyte percentage (LY%), monocyte percentage (MONO%), platelet count (PLT), mean platelet volume (MPV), albumin (Alb), fibrinogen (Fib), β2-MG, total cholesterol (TC), triglycerides (TG), high density lipoprotein (HDL), low density lipoprotein (LDL), lipoprotein(a) [Lp(a)], glomerular filtration rate (GFR), creatinine (Cr), homocysteine (Hcy). Imaging results including sum (PS) and maximum (P-max) of the plaque thickness, the degree of carotid artery and coronary artery stenosis and the carotid plaque level.
Hypertension was defined as blood pressure > 140/90 mmHg or treatments with antihypertensive medication. The diagnosis of diabetes mellitus was based on the 2017 Standards of Medical Care in Diabetes (7). In coronary computed tomography angiography (CCTA), the degree of coronary stenosis was classified as obstructive (luminal stenosis ≥ 50% of the coronary diameter) or non-obstructive (luminal stenosis < 50%) (8). Multi-site stenosis of a single vessel is determined by the most severe stenosis, and multi-vessel stenosis is defined as stenosis of two or more vessels (9). Guidelines for the diagnosis and treatment of carotid stenosis were used to classify symptomatic carotid stenosis (SCS) and asymptomatic carotid stenosis (3). A current smoker is defined as someone who has smoked cigarettes on a regular basis within the past six months, whereas an alcoholic is defined as someone who has consumed alcohol on a regular basis for over a year. The BMI was computed by dividing the weight (kg) by the square of the height (m). P-max was measured as the vertical distance between the tip of the plaque and adventitia interface of the lumen. PS was the sum of bilateral maximal carotid plaque thickness. The PS and P-max were determined by carotid artery ultrasound (10). Using the CEUS plaque blood flow grading standard, the grade of carotid plaque was classified as grades I to IV. Grade I was defined as the absence of plaque enhancement, grade II as the presence of one to three punctate plaque enhancements, and grade III as the presence of more than three punctate plaque enhancements or one to two short-line plaque enhancements. Grade IV is characterised by the presence of two or more linear enhancements in the plaque that are penetrating or predominantly penetrating the plaque, or by a sense of flow (11).
Statistical analysis
Data analysis was performed using SPSS 23.0 and R version 4.2.1. Except for age, BMI, and HbA1c, the mean and median were chosen as the boundary values for the continuous variables of normal distribution and non-normal distribution, and the data were converted to binary variables for subsequent analysis. Multiple logistic regression included the binary logistic regression analysis factors with P < 0.05. Using backward stepwise multiple logistic analysis, independent predictors were identified. Selected predictors were cross-validated further utilising LASSO regression. The L1-penalized LASSO regression was applied to reduce the data dimensionality to avoid potential collinearity and overfitting among variables. The best lambda value was selected in LASSO regression using tenfold cross-validation. Under the lambda compression (lambda.1se), the variables with small regression coefficients were directly compressed to 0 to eliminate the corresponding variables. Using the “rms” programme, statistically significant factors were used to construct the nomogram and generate a prediction model. To evaluate the accuracy of the prediction model, we calculated the consistency index (C-index) using the “rms” programme. By constructing a calibration curve, the level of calibration was determined. The greater the consistency of the prediction model, the closer the calibration curve of the model to the standard curve. Using DCA, the clinical utility of the nomogram was determined by quantifying the net benefits of the probability of various threshold values in the array. Finally, an internal validation approach was utilised to evaluate the prediction model's stability.
Results
Patient characteristics
This study included 227 patients, involving 181 males (79.7%) and 46 females (20.3%). Subjects were divided into case and control groups according to whether they had obstructive coronary stenosis simultaneously. There were 132 cases in the case group (58.1%) and 95 cases in the control group (41.9%). There were 62 cases (47.0%) of single-vessel disease and 70 cases (53.0%) of multi-vessel disease in the case group. All patients exhibited no coronary stenosis-related symptoms.
Analysis of predictive factors
Thirty laboratory parameters and imaging variables were included as independent variables in the binary logistic regression (Table 1). Hypertension, Grade I plaque, HbA1c, MPV, and Fib were statistically significant predictors (P < 0.05) based on the results of binary logistic regression. Binary logistic regression showed that Hypertension, Grade I plaque, HbA1c, MPV, and Fib were statistically significant predictors (P < 0.05). Multivariate logistic regression included the factors with P < 0.05 in binary logistic regression. According to the results, Hypertension, Grade I plaque, HbA1c, MPV, and Fib were independent predictors (Table 2). On the included variables, LASSO regressions were conducted to evaluate the selected predictors' reliability further. Five predictors were selected based on the optimal value (λ = 0.0629). Variables included hypertension, Grade I plaque, HbA1c, MPV, and Fib. Consistent with the independent predictors identified by multiple regression, the regression coefficients were 0.203, −0.242, 0.135, −0.167, and 0.106 (Figure 2).
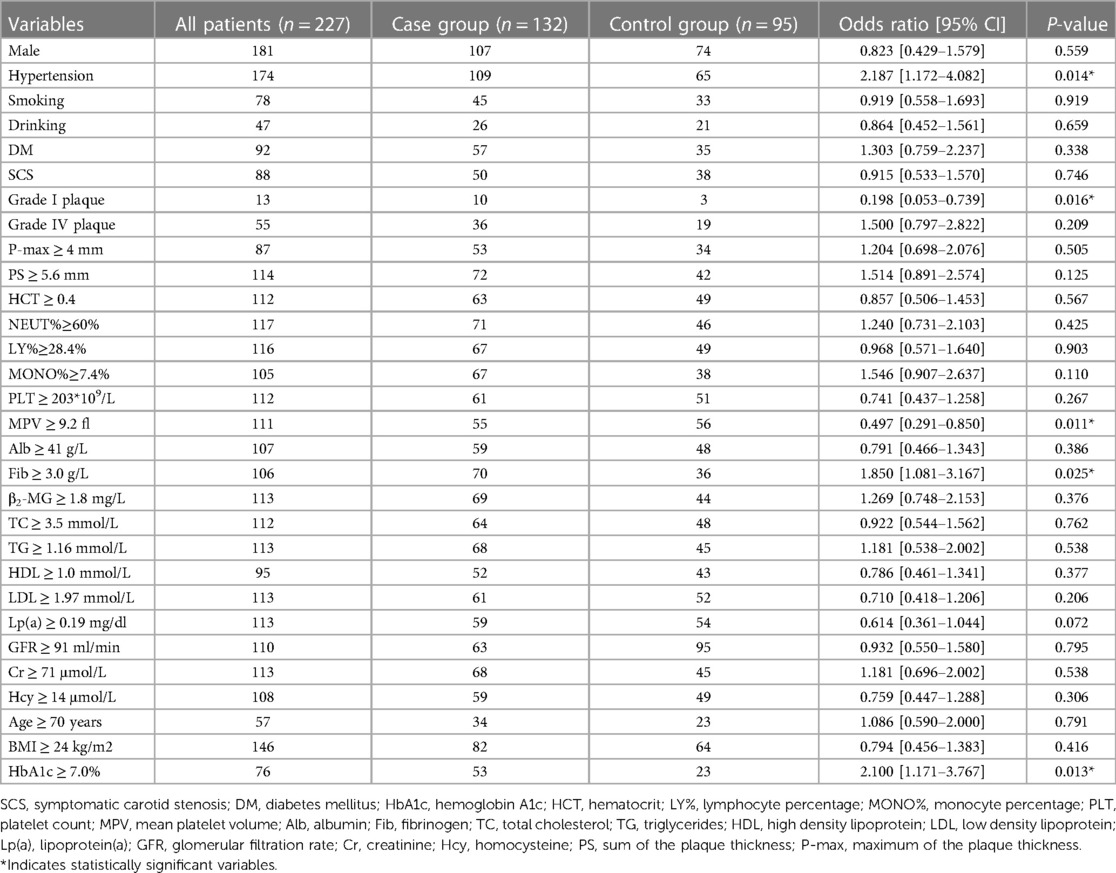
Table 1. Results of binary logistic regression analysis of the clinical characteristics of patients in the case and control groups.
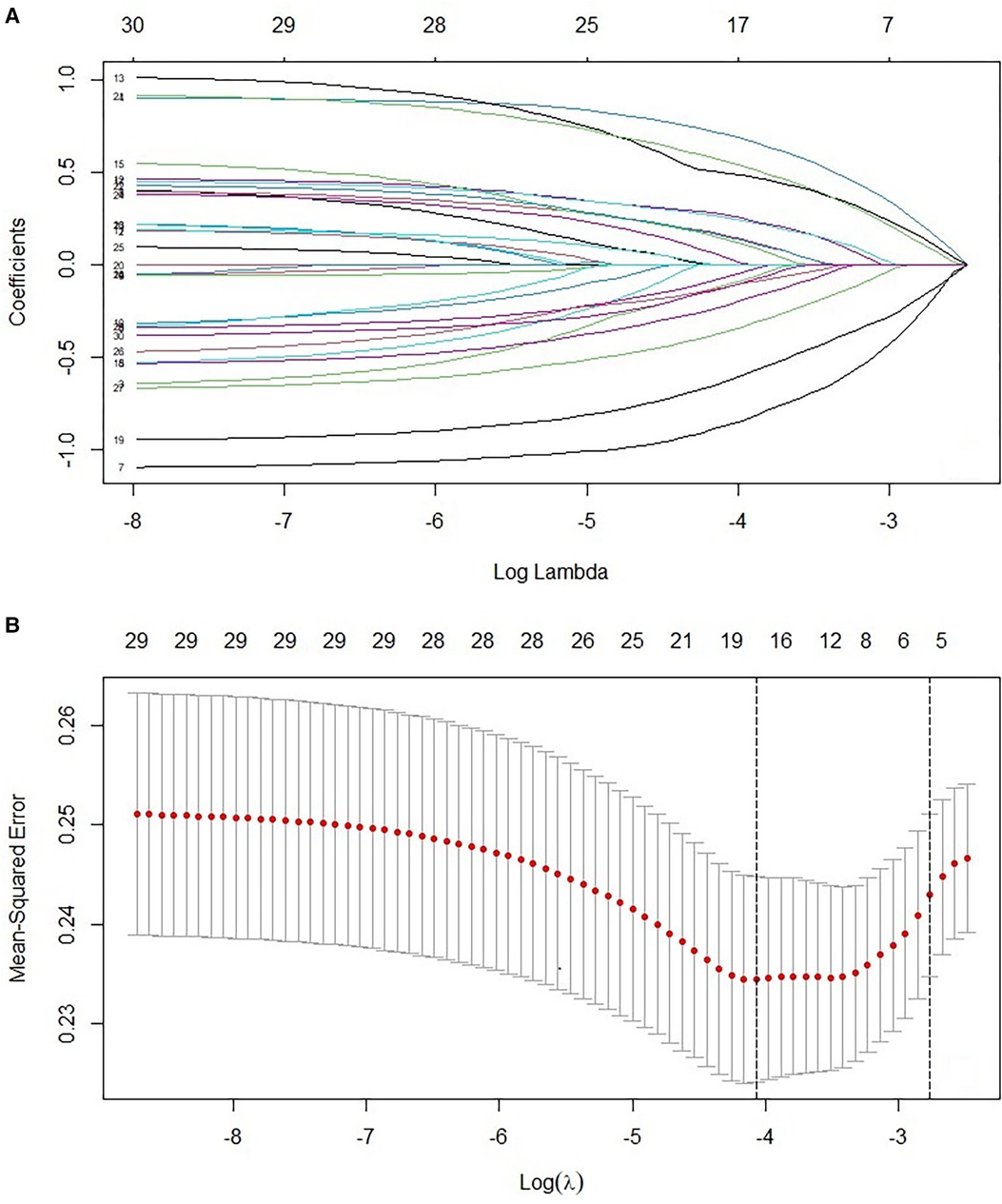
Figure 2. (A) LASSO coefficient profiles of 227 patients with obstructive coronary stenosis. A coefficient profile plot was produced against the log (λ) sequence. (B) Texture feature selection by using the least absolute shrinkage and selection operator (LASSO) binary logistic regression model. Tuning parameter (λ) selection in the LASSO model used 10-fold cross-validation via maximum AUC. A λ value of 0.0629 was chosen (1-SE criteria) according to 10-fold cross-validation.
Nomogram's building and apparent performance
On the basis of the outcomes of logistic regression and LASSO regression, five predictors were eliminated and a nomogram risk prediction model was developed (Figure 3). Each variable was assigned a score, and the higher the score, the greater the risk of obstructive coronary stenosis in carotid stenosis patients.
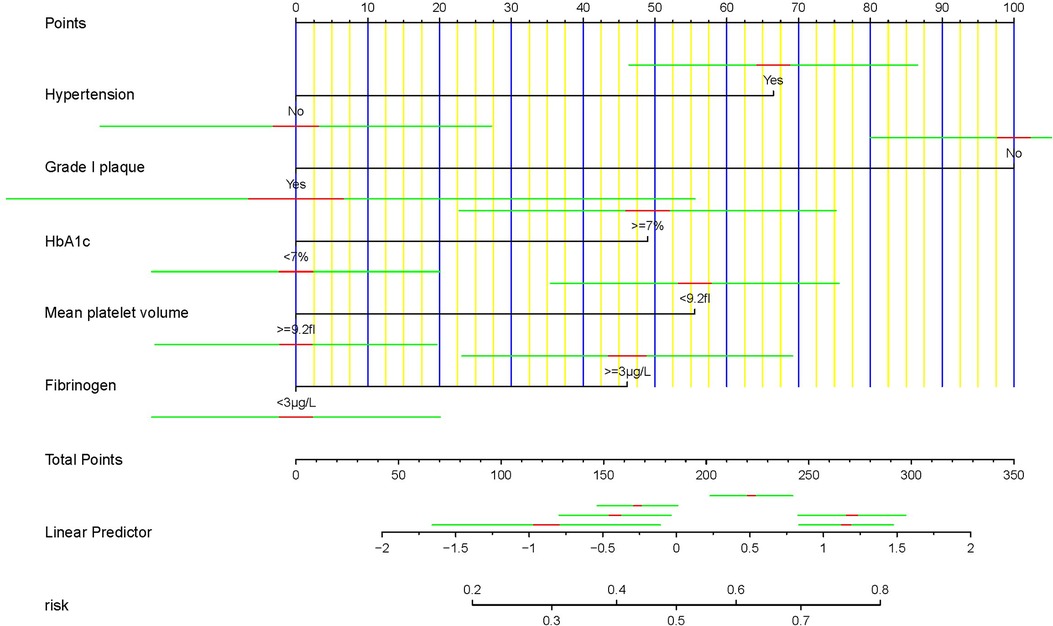
Figure 3. Nomogram for predicting the incidence of asymptomatic obstructive coronary stenosis. The construction of the nomogram included Hypertension, Grade I plaque, HbA1c, MPV, and Fib.
Assessment of the degree of calibration for the risk prediction model
To facilitate the evaluation of the risk prediction model's degree of rectification in this investigation, we developed a correction curve. As shown in Figure 4, the x-axis represents the predicted risk for asymptomatic obstructive coronary stenosis, and the y -axis represents the realization of asymptomatic obstructive coronary stenosis. The solid diagonal line represents the prediction of the ideal model, and the dashed line represents the actual predictive ability. The closer the dashed line is to the diagonal, the greater the predictive ability. Hosmer-Lemeshow test showed that the model had good calibration (χ2 = 2.24, P = 0.987).
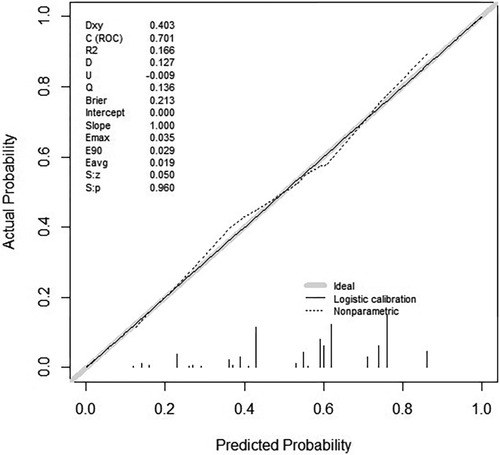
Figure 4. Curve of calibration for the risk prediction model of asymptomatic obstructive coronary stenosis.
Assessment of the accuracy
To evaluate the model's accuracy, we first calculated the C-index. When plotting a ROC curve, we can use AUC to quantify the predictor's efficacy. We then determined the model's AUC and constructed a ROC curve. The calculated AUC values of 0.701 (95% CI: 0.633–0.770) indicate that the model is accurate (Figure 5). The ROC curve was internally validated using the Bootstrap 1,000 times self-sampling method, and the AUC was found to be 0.668.
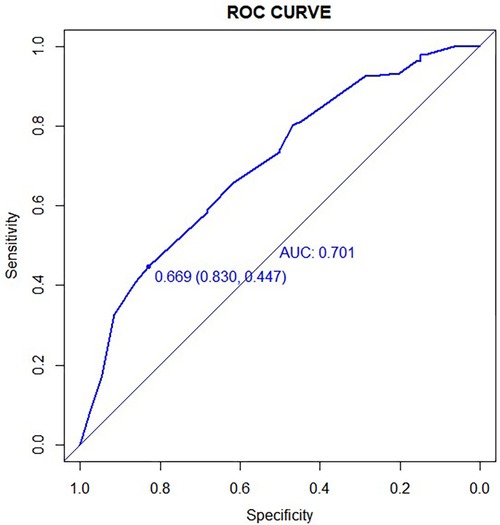
Figure 5. The ROC curve corresponding to the asymptomatic obstructive stenosis risk prediction model. The AUC value was 0.701%.
Clinical net benefit
DCA was utilized to determine if the prediction model could enhance clinical decision-making (Figure 6). The y-axis indicates the net benefit, and the x-axis represents the probability of the threshold value. The black solid line represents no intervention, at which the net benefit is zero. The Grey solid line represents the intervention, and the net benefit is an oblique line with a negative slope. The green solid line represents the realized profits of the asymptomatic obstructive coronary stenosis risk prediction model. The DCA curve shows that the threshold of 0.08–0.70 has the largest clinical net income.
Discussion
This study examined the statistical characteristics, imaging examination, and laboratory examination results of asymptomatic coronary artery stenosis in patients with carotid artery stenosis ≥ 50%. Univariate and multivariate logistic regression were utilized to identify independent risk factors, including hypertension, grade I plaque, HbA1c, MPV, and Fib. Using LASSO regression, the accuracy of the selected predictive factors was further confirmed, and a clinical predictive model was developed. According to the ROC curve, the AUC of the prediction model is 0.701, indicating that the model has a high degree of discrimination. Fit tests and clinical decision curves demonstrated that the predictive model was well calibrated and applicable in the clinical setting. Due to the limited sample size of this study, all data were only modeled as a training set, and the model was Bootstrapped 1,000 times for internal validation. The results demonstrate that the model of prediction is stable.
The most common cause of carotid stenosis is atherosclerosis, a multifactorial, progressive systemic disease (12). Plaque rupture, arterial embolism caused by thrombosis, and hemodynamic disturbance caused by stenosis comprise the majority of the carotid stenosis-induced ischemic stroke mechanism (13). Coronary artery disease is more common in carotid stenosis patients. According to studies, the prevalence of coronary artery disease in patients with carotid stenosis ranges from 13% to 86%, with the prevalence increasing with the severity of carotid stenosis (4). Asymptomatic coronary stenosis is easier to neglect than symptomatic coronary stenosis due to the absence of typical symptoms. Consistent with previous research, 58.1% of the 132 patients in this study experienced moderate or severe coronary stenosis complications. Due to the substantial increase in cardiovascular risk among patients with carotid artery stenosis and coronary artery stenosis coexisting, it is crucial to investigate the coexistence of carotid artery and coronary artery stenosis.
Hypertension was an independent risk factor for carotid stenosis with asymptomatic obstructive coronary stenosis in this study. High blood pressure damages the arterial wall and increases collagen deposition in the stroma, which causes the tunica media to thicken and the artery to become constricted. Hypertension is frequently viewed as a disease that damages large and small arteries, and lesions of corresponding arteries contribute to the occurrence and progression of hypertension. Prior research has demonstrated that hypertension is a risk factor for stroke. Patients with hypertension are substantially more susceptible to suffering a stroke than those with normal blood pressure (14). In a study of the lifetime risk of coronary heart disease, the lifetime risk of coronary heart disease in male hypertensive patients was 26.95% and the lifetime risk of coronary heart disease in female hypertensive patients was 14.85%, both of which were higher than the average level (15). High blood pressure is more likely to cause injury to the carotid and coronary arteries, resulting in their constriction.
HbA1c is produced by a sluggish, continuous, and irreversible glycation reaction and may reflect changes in patients’ blood glucose levels over the previous 8 to 12 weeks. In clinical practice, it is considered an essential indicator of blood sugar control in diabetic patients. Studies have shown that HbA1c levels are strongly associated with the risk of diabetes-related complications and cardiovascular disease (16). In general, a glycated hemoglobin level below 7.0% is advised for glycemic control (17). In a study of healthy controls and subjects with T2DM, glycemic fluctuations rather than persistent glycemic elevation caused endothelial cell injury, and intensive glycemic control reduced the incidence of microvascular and macrovascular complications by 25% (18, 19). In this study, patients with HbA1c levels greater than 7.0% were more likely to have asymptomatic coronary stenosis (OR = 2.005). It is advantageous to reduce this risk by enhancing blood glucose regulation and decreasing blood glucose fluctuations.
The investigation included imaging measures to assess the morphology of the plaque. CEUS is a common noninvasive technique for detecting plaque neovascularization. New blood vessels in the plaque are immature and readily rupture, resulting in inflammation of the vessel wall and vessel wall injury (20). High-grade carotid plaques as measured by CEUS are associated with more severe and unstable coronary artery disease, and the carotid plaque enhancement intensity is an independent predictor of secondary cardiovascular events in patients with coronary heart disease (21). This study evaluated the coronary status of patients with grade I or IV plaque and discovered that patients with grade I plaque were substantially less likely to develop asymptomatic obstructive coronary stenosis than controls (OR = 0.219). Using carotid plaque grade as a predictive parameter may increase the probability of identifying coronary stenosis.
Fib is a coagulation factor that promotes platelet aggregation, the growth, proliferation, and contraction of smooth muscle and endothelial cells, as well as increases blood viscosity and damages endothelial cells. Consequently, it is crucial to the pathogenesis of cardiovascular disease. Studies have shown that high levels of Fib are associated with an increased risk of stroke and poor patient outcomes (22). In terms of coronary stenosis, elevated Fib levels are generally regarded as an independent predictor of the presence and severity of coronary artery disease. This may be because fibrinogen stimulates the migration and proliferation of smooth muscle cells, and its degradation products are chemotactic for leukocytes and macrophages (23, 24).
MPV ≥ 9.2fl was a predictor of coronary stenosis events (OR = 0.457) and was protective in this study. MPV reflects the average platelet size, and aberrant MPV is associated with a variety of diseases, including diabetes, cardiovascular disease, and chronic obstructive pulmonary disease (25). High MPV levels have been associated with ST-segment elevation myocardial infarction in patients with coronary heart disease, and it is also regarded as an independent risk factor for inadequate coronary collateral compensation and a poor prognosis (26, 27). The above conclusion differs somewhat from the findings of this study, likely because the majority of the previous studies focused on the effects of acute coronary artery disease. In a study examining the effect of MPV on long-term outcomes following coronary intervention in patients with stable coronary artery disease, decreased MPV levels were associated with inferior outcomes (28). In addition, studies have demonstrated a correlation between systemic inflammation and platelet size. The MPV decreases as the degree of inflammation increases, whereas the MPV increases as the degree of inflammation decreases (29). The aforementioned findings suggest that elevated MPV does not increase the risk of stable coronary artery disease in patients with carotid artery stenosis; however, additional research is required to determine its cardiovascular effects.
This study analyzed the risk factors for asymptomatic obstructive coronary artery stenosis in patients with carotid artery stenosis ≥ 50% and constructed a clinical model with strong predictive ability. Nevertheless, given that this is a retrospective investigation, there are some limitations. First, it is necessary to exclude cases with insufficient data, which could introduce selection bias, and additional prospective studies are required for validation. Secondly, the sample size of this study is insufficient, and the proportion of male patients is high; therefore, it is necessary to increase the sample size in order to further assess the results' reliability.
Conclusion
This study concluded that there is a significant correlation between carotid artery stenosis and coronary artery stenosis, and that asymptomatic and occult coronary artery stenosis warrants special attention. In patients with carotid stenosis, hypertension, grade I plaque, mean platelet volume ≥ 9.2 fl, fibrinogen ≥ 3.0 g/L, and glycosylated hemoglobin ≥ 7.0% were independent predictors of asymptomatic obstructive coronary stenosis. The clinical diagnostic model based on the aforementioned variables is capable of predicting the risk of coronary artery stenosis to a certain degree.
Data availability statement
The raw data supporting the conclusions of this article will be made available by the authors, without undue reservation.
Author contributions
ZL and HC: designed the study. CQ, CL: collected the data. YL: helped with the statistical analysis. CQ: wrote the manuscript. CL, YL, ZL and HC: revised the manuscript. All authors contributed to the article and approved the submitted version.
Conflict of interest
The authors declare that the research was conducted in the absence of any commercial or financial relationships that could be construed as a potential conflict of interest.
Publisher's note
All claims expressed in this article are solely those of the authors and do not necessarily represent those of their affiliated organizations, or those of the publisher, the editors and the reviewers. Any product that may be evaluated in this article, or claim that may be made by its manufacturer, is not guaranteed or endorsed by the publisher.
References
1. Kleindorfer DO, Khoury J, Moomaw CJ, Alwell K, Woo D, Flaherty ML, et al. Stroke incidence is decreasing in whites but not in blacks: a population-based estimate of temporal trends in stroke incidence from the greater Cincinnati/northern Kentucky stroke study. Stroke. (2010) 41(7):1326–31. doi: 10.1161/strokeaha.109.575043
2. Song P, Fang Z, Wang H, Cai Y, Rahimi K, Zhu Y, et al. Global and regional prevalence, burden, and risk factors for carotid atherosclerosis: a systematic review, meta-analysis, and modelling study. Lancet Glob Health. (2020) 8(5):e721–9. doi: 10.1016/s2214-109x(20)30117-0
3. AbuRahma AF, Avgerinos ED, Chang RW, Darling RC, Duncan AA, Forbes TL, et al. Society for vascular surgery practice guidelines for management of extracranial clinical cerebrovascular disease. J Vasc Surg. (2022) 75(1s):4s–22s. doi: 10.1016/j.jvs.2021.04.073
4. Liu ZJ, Fu WG, Guo ZY, Shen LG, Shi ZY, Li JH. Updated systematic review and meta-analysis of randomized clinical trials comparing carotid artery stenting and carotid endarterectomy in the treatment of carotid stenosis. Ann Vasc Surg. (2012) 26(4):576–90. doi: 10.1016/j.avsg.2011.09.009
5. Ma X, Kong Q, Wang C, Rajah G, Ding YC, Zhang YR, et al. Predicting asymptomatic coronary artery stenosis by aortic arch plaque in acute ischemic cerebrovascular disease: beyond the cervicocephalic atherosclerosis? Chin Med J. (2019) 132(8):905–13. doi: 10.1097/CM9.0000000000000174
6. Thai PV, Tien HA, Van Minh H, Valensi P. Triglyceride glucose index for the detection of asymptomatic coronary artery stenosis in patients with type 2 diabetes. Cardiovasc Diabetol. (2020) 19(1):137. doi: 10.1186/s12933-020-01108-2
7. Shen JI, Nicholas SB, Williams S, Norris KC. Evidence for and against ACC/AHA 2017 guideline for target systolic blood pressure of <130 mmHg in persons with type 2 diabetes. Curr Cardiol Rep. (2019) 21(11):149. doi: 10.1007/s11886-019-1251-4
8. Park HB, Heo R, Hartaigh BÓ, Cho I, Gransar H, Nakazato R, et al. Atherosclerotic plaque characteristics by CT angiography to identify coronary lesions that cause ischemia: a direct comparison to fractional flow reserve. JACC Cardiovasc Imaging. (2015) 8(1):1–10. doi: 10.1016/j.jcmg.2014.11.002
9. Raff GL, Abidov A, Achenbach S, Berman DS, Boxt LM, Budoff MJ, et al. SCCT Guidelines for the interpretation and reporting of coronary computed tomographic angiography. J Cardiovasc Comput Tomogr. (2009) 3(2):122–36. doi: 10.1016/j.jcct.2009.01.001
10. Xia S, Qiu W, Cai A, Kong B, Xu L, Wu Z, et al. The association of lipoprotein (a) and intraplaque neovascularization in patients with carotid stenosis: a retrospective study. BMC Cardiovasc Disord. (2021) 21(1):285. doi: 10.1186/s12872-021-02038-x
11. Huang Y, Liu Q, Xu J, Zhu W, Jiang J, Tang L, et al. Contrast-enhanced ultrasound perfusion patterns and serum lipid signatures of vulnerable carotid artery plaque in predicting stroke: a cohort study of carotid stenosis in Chinese patients. Clin Hemorheol Microcirc. (2020) 75(3):349–59. doi: 10.3233/ch-190804
12. Kwak BR, Bäck M, Bochaton-Piallat ML, Caligiuri G, Daemen MJ, Davies PF. Biomechanical factors in atherosclerosis: mechanisms and clinical implications. Eur Heart J. (2014) 35(43):3013–20, 3020a–3020d. doi: 10.1093/eurheartj/ehu353
13. Heck D, Jost A. Carotid stenosis, stroke, and carotid artery revascularization. Prog Cardiovasc Dis. (2021) 65:49–54. doi: 10.1016/j.pcad.2021.03.005
14. Li AL, Ji Y, Zhu S, Hu ZH, Xu XJ, Wang YW, et al. Risk probability and influencing factors of stroke in followed-up hypertension patients. BMC Cardiovasc Disord. (2022) 22(1):328. doi: 10.1186/s12872-022-02780-w
15. Turin TC, Okamura T, Afzal AR, Rumana N, Watanabe M, Higashiyama A, et al. Impact of hypertension on the lifetime risk of coronary heart disease. Hypertens Res. (2016) 39(7):548–51. doi: 10.1038/hr.2016.23
16. Tong H, Wang D, Fang M. Correlation between the variability of glycosylated hemoglobin and cardiovascular risk in new-onset T2DM patients. Contrast Media Mol Imaging. (2022) 2022:5370301. doi: 10.1155/2022/5370301
17. Kim KJ, Choi J, Bae JH, Kim KJ, Yoo HJ, Seo JA, et al. Time to reach target glycosylated hemoglobin is associated with long-term durable glycemic control and risk of diabetic complications in patients with newly diagnosed type 2 diabetes Mellitus: a 6-year observational study. Diabetes Metab J. (2021) 45(3):368–78. doi: 10.4093/dmj.2020.0046
18. Ceriello A, Esposito K, Piconi L, Ihnat MA, Thorpe JE, Testa R, et al. Oscillating glucose is more deleterious to endothelial function and oxidative stress than mean glucose in normal and type 2 diabetic patients. Diabetes. (2008) 57(5):1349–54. doi: 10.2337/db08-0063
19. Stratton IM, Adler AI, Neil HA, Matthews DR, Manley SE, Cull CA, et al. Association of glycaemia with macrovascular and microvascular complications of type 2 diabetes (UKPDS 35): prospective observational study. Br Med J. (2000) 321(7258):405–12. doi: 10.1136/bmj.321.7258.405
20. Shah F, Balan P, Weinberg M, Reddy V, Neems R, Feinstein M, et al. Contrast-enhanced ultrasound imaging of atherosclerotic carotid plaque neovascularization: a new surrogate marker of atherosclerosis? Vasc Med. (2007) 12(4):291–7. doi: 10.1177/1358863(07083363
21. Deyama J, Nakamura T, Takishima I, Fujioka D, Kawabata K, Obata JE, et al. Contrast-enhanced ultrasound imaging of carotid plaque neovascularization is useful for identifying high-risk patients with coronary artery disease. Circ J. (2013) 77(6):1499–507. doi: 10.1253/circj.cj-12-1529
22. Pikija S, Trkulja V, Mutzenbach JS, McCoy MR, Ganger P, Sellner J. Fibrinogen consumption is related to intracranial clot burden in acute ischemic stroke: a retrospective hyperdense artery study. J Transl Med. (2016) 14(1):250. doi: 10.1186/s12967-016-1006-6
23. Deveci B, Gazi E. Relation between globulin, fibrinogen, and albumin with the presence and severity of coronary artery disease. Angiology. (2021) 72(2):174–80. doi: 10.1177/0003319720959985
24. Smith EB. Fibrinogen, fibrin and fibrin degradation products in relation to atherosclerosis. Clin Haematol. (1986) 15(2):355–70. doi: 10.1016/S0308-2261(18)30021-3
25. Pafili K, Penlioglou T, Mikhailidis DP, Papanas N. Mean platelet volume and coronary artery disease. Curr Opin Cardiol. (2019) 34(4):390–8. doi: 10.1097/hco.0000000000000624
26. Sincer I, Gunes Y, Mansiroglu AK, Cosgun M, Aktas G. Association of mean platelet volume and red blood cell distribution width with coronary collateral development in stable coronary artery disease. Postepy Kardiol Interwencyjnej. (2018) 14(3):263–9. doi: 10.5114/aic.2018.78329
27. Choi DH, Kang SH, Song H. Mean platelet volume: a potential biomarker of the risk and prognosis of heart disease. Korean J Intern Med. (2016) 31(6):1009–17. doi: 10.3904/kjim.2016.078
28. Wada H, Dohi T, Miyauchi K, Shitara J, Endo H, Doi S, et al. Mean platelet volume and long-term cardiovascular outcomes in patients with stable coronary artery disease. Atherosclerosis. (2018) 277:108–12. doi: 10.1016/j.atherosclerosis.2018.08.08.048
Keywords: carotid stenosis, coronary stenosis, prediction model, nomogram, LASSO regression analysis
Citation: Qin C, Li C, Luo Y, Li Z and Cao H (2023) Construction and validation of a clinical prediction model for asymptomatic obstructive coronary stenosis in patients with carotid stenosis. Front. Cardiovasc. Med. 10:1096020. doi: 10.3389/fcvm.2023.1096020
Received: 11 November 2022; Accepted: 17 August 2023;
Published: 7 September 2023.
Edited by:
Marcone Lima Sobreira, Sao Paulo State University, BrazilReviewed by:
Bu-Lang Gao, Hebei Medical University, ChinaRafael De Athayde Soares, Hospital do Servidor Público Estadual, Brazil
© 2023 Qin, Li, Luo, Li and Cao. This is an open-access article distributed under the terms of the Creative Commons Attribution License (CC BY). The use, distribution or reproduction in other forums is permitted, provided the original author(s) and the copyright owner(s) are credited and that the original publication in this journal is cited, in accordance with accepted academic practice. No use, distribution or reproduction is permitted which does not comply with these terms.
*Correspondence: Hui Cao ZmNjY2FvaDJAenp1LmVkdS5jbg==