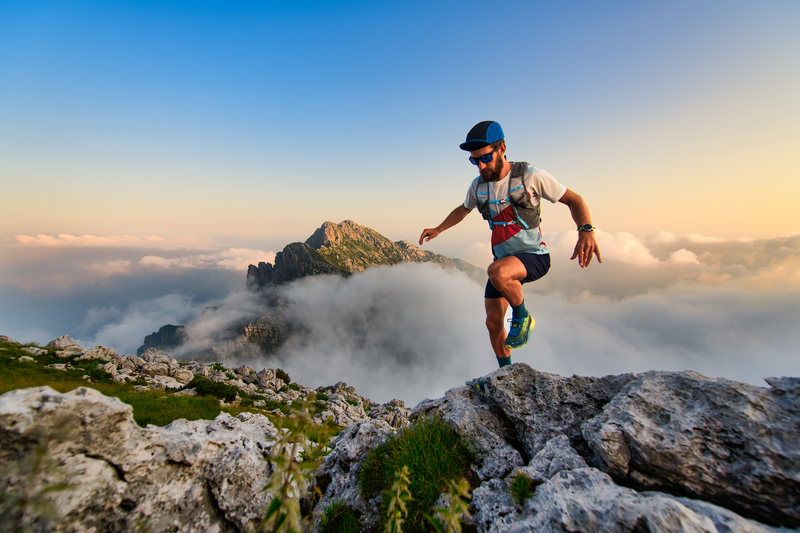
94% of researchers rate our articles as excellent or good
Learn more about the work of our research integrity team to safeguard the quality of each article we publish.
Find out more
ORIGINAL RESEARCH article
Front. Cardiovasc. Med. , 30 June 2023
Sec. Cardiac Rhythmology
Volume 10 - 2023 | https://doi.org/10.3389/fcvm.2023.1071574
This article is part of the Research Topic Atrial Fibrillation in Dementia View all 6 articles
Background: Numerous observational studies have suggested that atrial fibrillation (AF) was associated with an increased risk of vascular dementia (VaD). However, the causal genetic relationships between AF and VaD remains unclear. To evaluate the effect of AF on VaD, we performed the Mendelian randomization (MR) analysis to investigate the causal genetic relationships between AF and VaD.
Methods: The bidirectional MR analysis was conducted to explore the causal relationships between exposure and disease. We applied a series of quality assessments to select significantly and independently single nucleotide polymorphisms (SNPs) from publicly available large-scale genome-wide association studies (GWAS) databases. Three methods [Inverse variance weighted method (IVW), MR-Egger method, and weighted median (WM)method] were used to derive MR estimates. In order to ensure reliable MR results, sensitivity analyses were performed to evaluate the horizontal pleiotropy and heterogeneity.
Results: Our MR analyses revealed no significant genetic relationships between AF and the risk of VaD (IVW: OR = 1.10, 95%CI = 0.95–1.28, P = 0.20). In the reverse direction analysis, there was no evidence to support a significant genetic relationship of VaD with AF risk (IVW: OR = 1.00, 95% CI = 0.99–1.01, P = 0.52). Consistent results were obtained using different MR methods. Sensitivity analyses suggested no significant horizontal pleiotropy and heterogeneity in the study.
Conclusion: This MR analysis did not provide evidence to support the causal genetic relationships between AF on VaD risk and the causal effect of VaD on AF risk.
Vascular dementia (VaD), accounting for approximately 15% of dementia cases, is the second most common dementia after Alzheimer's disease (AD) (1). It is described as a brain injury due to impaired cerebral blood flow resulting in severe cognitive decline that interferes with family, occupational and social life (2). Similar to AD, the incidence of VaD increases rapidly with advancing age (3). As the global average life expectancy increases, the prevalence of dementia in the aging population undoubtedly imposes an enormous burden on families, healthcare institutions and the government. Currently, there are no effective and licensed treatments for VaD (1). Numerous epidemiological studies have found that the incidence of dementia has declined in recent years because of reductions in vascular risk factors and improvements in treatment modalities (4–6). Therefore, the risk factors for VaD have received much attention recently, such as advanced age, hypertension, diabetes, obesity, and cardiovascular factors (7). However, some of the risk factors remain controversial.
Atrial fibrillation (AF) is a common cardiac arrhythmia with a high rate of disability and mortality (8). It is usually associated with multiple comorbidities (9). There were approximately 37.6 million cases of AF worldwide in 2017, and the number is increasing yearly (10). Patients with AF appear to be more susceptible to VaD and exhibit a worse prognosis. A meta-analysis showed that AF was significantly associated with an increased risk of VaD [odds ratio (OR) = 1.7, 95% confidence interval (CI): 1.4–3.5] (11). In the meta-analysis, we found that current cohort studies were usually corrected for risk factors such as age, hypertension, coronary artery disease, diabetes, and hyperlipidemia. However, there is always a risk of unmeasured confounders, which is relevant to address as well as the advantage of MR studies when it comes to causality.
Mendelian randomization (MR) is a robust statistical method that employs genetic variables as instruments to reveal genetic causality between exposure and disease (12). MR analysis enables to overcome reverse causality relationships as genes are essentially stable after fertilization (13). Moreover, MR analysis can eliminate the interference of confounding factors owing to the random assignment of alleles (14).
In the present study, we used the publicly available database of large-scale genome-wide relationships studies (GWAS) and applied a two-sample bidirectional MR analysis to investigate the potential causal relationships between AF and VaD.
The study was conducted in accordance with the Declaration of Helsinki. Summary-level statistics from the FinnGen GWAS database for VaD are publicly available (URL: http://r5.risteys.finngen.fi/phenocode/F5_VASCDEM, last accessed on 16 August 2022). The summary statistics for the AF are provided in the GWAS meta-analysis (URL:http://www.nature.com/articles/s41588-018-0171-3, last accessed on 16 August 2022). As the study examined anonymized and summary-level data, the informed consent was waived.
We conducted a two-sample MR study to explore the causal relationship between AF and VaD risk using the largest publicly available GWAS database. The MR study was performed based on the hypothesis that genetic variants affect the outcome only through exposure rather than other pathways. The selected IVs should be independent of confounding factors (Figure 1) (15). The bidirectional MR analysis flow is shown in Figure 2.
Figure 2. The flow chart of the bidirectional MR process. AF, atrial fibrillation; VaD, vascular dementia; SNP, single nucleotide polymorphisms; MR-PRESSO, MR-pleiotropy residual sum and outlier.
For the exposure data, we selected the largest publicly summarized GWAS database of AF. In total, more than 1,000,000 participants of European ancestry were enrolled, including 60,620 cases and 970,216 controls (16). This database compares data from six cohort studies (The Nord-Trøndelag Health Study (HUNT), the Michigan Genomics Initiative (MGI), deCODE, DiscovEHR, AFGen Consortium, and the UK Biobank). The diagnostic criteria for AF were the International Classification of Diseases (ICD) 9 and ICD 10 code. SNPs information of effect allele (EA), effect frequency (EAF), effect size (β) and P value were extracted. The baseline characteristics of the database are shown in Table 1.
As for the outcome data, we obtained pooled-level data from the Finn consortium for 881 cases and 211,508 controls in the European population. All cases of VaD were diagnosed according to the ICD 10 code.
Figure 3 shows the Flow chart of the performed analysis and construction of the genetic instruments. Firstly, each SNP should be associated closely with AF. SNPs were included if they reached genome-wide significance (P < 5 × 10−8). SNPs with F statistic great 10 to avoid bias caused by weak IVs. The formula for F statistic is detailed in Supplementary Material S1 (17). Secondly, each SNP should not be associated with confounders of the exposure-outcome relationship. SNPs were included if they satisfied the independence [linkage disequilibrium (LD), r2 ≤ 0.001]. Thirdly, each SNP should only affect the risk of outcome indicators through exposure factors and no other pathways (no pleiotropy). We remove the SNPs associated with confounding elements of VaD using PhenoScanner V2 (www.phenoscanner.medschl.cam.ac.uk) (18).
We extracted 111 significant and independent SNPs associated with AF from the largest GWAS database. 10 SNPs (rs284277, rs6689306, rs2540949, rs1458038, rs1838747, rs56201652, rs11191116, rs7915134, rs12245149, rs2759301) were removed using PhenoScanner V2 as these SNPs were associated with known confounders (hypertension, coronary artery atherosclerotic heart disease, body mass index and education), and 2 SNPs were excluded due to their mutual pairing in the double helix structure. The characteristics of the final SNPs for AF are shown in Supplementary Table S1.
Since setting the threshold of P value at <5 × 10−8 obtains few SNPs for VaD, we relaxed the threshold of P value to <5 × 10−6 (19, 20). 12 SNPs were obtained from the GWAS database (Supplementary Table S2). No SNPs were included because of palindromic sequence and PhenoScanner V2.
All included SNPs F statistics were greater than 10, indicating no weak instrument bias in our study.
We used three approaches to evaluate MR estimates of AF with VaD, including inverse-variance weighted (IVW), weight median (WM) and MR-Egger. The IVW method was the primary method as it was the Wald ratio of individual SNPs for meta-analysis, which assumes that all IVs were valid (21). The intercept restriction of the IVW method is zero, which indicates that it can provide unbiased estimates if there is no pleiotropy (22). In the MR study, the presence of pleiotropy may lead to bias and unstable MR estimates (23). Therefore, we applied MR-Egger and WM as additional MR estimates. When up to 50% of the information comes from invalid instrumental variables, the WM method can be used as more reliable causal MR estimates (24). Although the MR-Egger approach is known to be somewhat resistant to the existence of pleiotropy, it nevertheless suffers from its effects and has reduced statistical power (25). However, it can detect whether the pleiotropy was present (22). Although these methods were less accurate, they might provide wider confidence intervals (CIs) (26). If these methods yield inconsistent MR estimates, we will tighten the IVs and reperform the analysis.
Sensitivity analyses were applied to ensure the reliability of the MR results. The heterogeneity of each SNP was evaluated by Cochran's Q statistic. In the present study, we used Cochran's Q statistic (P < 0.05) to represent the significant heterogeneity between the estimates of each included SNP. The intercept of the MR-Egger regression provided an indication of horizontal pleiotropy (P < 0.05 indicates the existence of horizontal pleiotropy). In addition, MR-Pleiotropy Residual Sum and Outlier methods (MR-PRESSO) were used to detect the outliers and potential horizontal pleiotropy (global P < 0.05 suggests the presence of horizontal pleiotropy). If outliers are found, they will be removed to obtain a more accurate corrected estimate (27). Finally, leave-one-out analysis was employed to evaluate the stability of MR estimates after excluding the individual SNP (28). Additionally, we switched the outcome and exposure and reperformed the MR and sensitivity analyses to avoid the possibility of reverse causation. The two-sample MR study was analyzed by Two-Sample MR (version 0.5.5) in R (version 4.2.0).
The results of MR estimation and sensitivity analyses are presented in Table 2. According to the IVW result, no significant causal relationship between AF and the risk of VaD was found (OR = 1.10, 95% CI = 0.95–1.28, P = 0.20). The MR-Egger (OR = 1.21, 95% CI = 0.90–1.62, P = 0.21) and WM (OR = 1.18, 95% CI = 0.90–1.55, P = 0.24) methods showed similar results (Figure 4A). Cochran's Q statistic showed no significant heterogeneity in the estimates of included SNPs (P = 0.19). In addition, the leave-one-out analysis also suggested that the result of MR estimates was stable (Figure 4B). The MR Egger's intercept (intercept = −0.01 and P = 0.48) and MR-PRESSO (global P = 0.18) revealed no significant horizontal pleiotropy in our study (Figure 4C). Further, the funnel plot was symmetrical, indicating that the results were reliable (Figure 4D).
Figure 4. Forest plot (A), leave-one-out analysis (B), scatter plot (C) and funnel plot (D) of the effect of atrial fibrillation on vascular dementia (VaD). Figure A shows the MR estimate of each SNP effect on VaD. Figure B depicts the changes of MR estimates after excluding individual SNP. The three lines in Figure C illustrate the estimated effect sizes by three MR methods (IVW, MR-Egger and WM). No significant horizontal pleiotropy was found for the MR-Egger's intercept. Figure D demonstrates that the funnel plot is symmetric, which indicates that the MR estimates are reliable.
We additionally performed a reverse MR study using VaD as exposure and AF as outcome to assess whether reverse causality exists. The flow of the reverse MR is shown in Figure 1. The results showed no significant causal relationship between VaD and AF risk using the IVW (OR = 1.00, 95%CI = 0.99–1.01, P = 0.52), MR-Egger (OR = 1.01, 95%CI = 0.99–1.02, P = 0.38), and WM (OR = 1.00, 95%CI = 0.99–1.01, P = 0.93) methods (Figure 5A and Table 2). In addition, cochran's Q statistic did not suggest significant heterogeneity (P = 0.45) (Table 2). The leave-one-out analysis did not find that our MR estimates were strongly affected by individual SNP (Figure 5B). The intercept of MR-Egger was 0.0019 (P = 0.61), which showed no significant horizontal pleiotropy (Figure 5C and Table 2). The funnel plot also showed that our results were reliable (Figure 5D).
Figure 5. Forest plot (A), leave-one-out analysis (B), scatter plot (C) and funnel plot (D) of the effect of vascular dementia on atrial fibrillation (AF). Figure A presents the MR estimated effect sizes for AF. Figure B shows the changes in MR estimates after excluding each individual SNP. The three lines in Figure C illustrate the estimated effect sizes by three MR methods (IVW, MR-Egger and WM). The intercept of MR-Egger suggests no significant heterogeneity. Figure D shows that the funnel plot is symmetric and the MR result is robust.
We applied two-sample MR methods based on large-scale GWAS databases to investigate the causal genetic relationship between AF and VaD. It was demonstrated that there was no causal relationship between AF and the risk of developing VaD. Besides, no indication supports that VaD was causally related to AF risk.
Up to now, several observational studies have shown a significant relationship between AF and dementia (29–33). The prestigious Rotterdam Prospective Study, which recruited 81,483 participants for 20 years of follow-up, found that AF was significantly associated with all-cause dementia (OR = 1.33,95% CI = 1.02–1.73), and that this relationship was independent of stroke (32). In addition, a large meta-analysis by Papanastasiou et al. that included 43 observational studies yielded a similar conclusion (OR = 1.6,95% CI = 1.3–2.1). The subgroup analysis also confirmed that AF was related to a higher risk of both AD and VaD (11). However, there were some limitations in the previous meta-analysis, including confounding factors, reverse causality, inconsistent diagnostic criteria, and significant heterogeneity. Notably, The MR research by Pan et al. showed no causal relationship between genetically predicted AF and the risk of AD development (34). VaD is the second leading cause of dementia after AD (1). Currently, its causality with AF remains unknown in the field of genetic prediction.
It should be interpreted with caution that AF did not show a significant genetic causal relationship to VaD. One possible explanation is that VaD and AF share many common risk factors, such as hypertension, diabetes, hypercholesterolemia, arterial blockage and heart failure, and other risk factors may interfere with the relationship between AF and VaD risk in the real world (35, 36). A study involving 135 patients reported that AF, hypertension, and angina were associated with higher rates of cognitive dysfunction (37). Yaffe et al. discovered that lower cognitive function was associated with higher cumulative exposure to hyperglycemia, hypertension, and hypercholesterolemia in 3,381 patients (38). Regrettably, these studies did not adequately exclude the common risk factors, and the subgroup analysis of AF and VaD were not conducted in some studies. Importantly, our MR study only explored the genetic causality of AF and VaD. (i.e., the presence or absence of AF and VaD). Certain studies suggest that the occurrence of VaD may be related to the type and pattern of AF. Chen et al. found that cognitive function scores were generally lower in persistent AF (monitored by a 14-day ECG patch assessment) compared to paroxysmal AF based on the large prospective US cohort study based on the Atherosclerosis Risk in Communities (ARIC) (39). A sizable cohort study reported that patients with persistent AF showed lower cerebral blood flow and more severe poor brain perfusion than patients with paroxysmal AF (40). In terms of inflammation, a growing body of studies found higher inflammatory markers in patients with persistent AF compared to the paroxysmal AF population (41–43). A retrospective examination of 619 patients with cardiogenic stroke by Deguchi et al. revealed that individuals with persistent AF had worse prognoses, more severe neurological impairments, and a higher frequency of significant artery occlusions (44).
Although our MR study did not find significant genetic causal relationships between AF and VaD. However, we still have reason to believe that there are some other potential factors that we have not yet focused on or observed that contribute to this relationship in the real world. These potential factors might be common risk factors interference, the cumulative effect of persistent episodes of AF, and inherent limitations in observational studies. Future studies could be considered for in-depth optimization in this area.
Genetic and environmental factors often combine to cause disease. Future studies could focus on the interaction between environmental factors (e.g., lifestyle, dietary habits.) and genetic factors to better understand the relationship between AF and VaD. Recent observational studies suggest that oral anticoagulants and statins may protect cognitive function in AF patients (45–47). And future studies could further investigate the mechanisms and therapeutic effects of these drugs in preventing the development of VaD in patients with AF, which would provide new treatment options for clinical practice. In addition, cardioversion or radiofrequency ablation therapy may improve cognitive function in patients with AF, which may help in the clinical management of these patients (48).
To our knowledge, this is the first study to evaluate the causal relationships between AF and VaD using MR methods. Our study has many strengths. The first is the MR design, Genetic variation in genomic regions has larger sample sizes and is less prone to potentially unmeasurable confounders than traditional observational studies. Moreover, reverse causality can be prevented because genetic variants are assigned at the time of conception.
Our study has some limitations. Firstly, epigenetic issues (such as DNA methylation, non-coding RNA regulation and chromatin remodeling) in MR studies are challenging to avoid. Secondly, the winner's curse may overestimate the genetic relationships between AF and VaD. Thus, we used three MR methods to evaluate MR estimates to avoid this bias. These methods yielded consistent results, which suggests that the winner's curse in our MR analysis is relatively small. Fourthly, we were unable to further calculate the population overlap rate as the FinnGen did not provide details of VaD cohorts. Thirdly, most of the people in these datasets were of European ancestry, which restricts the application of our findings to populations with more varied ethnic backgrounds. Finally, we could not obtain complete demographic and clinical information, limiting us from conducting additional subgroup analysis.
In conclusion, our MR study does not support a genetic causality between AF and VaD risk. Also, there was no causal relationships between VaD and AF risk. Updated MR studies will be needed when more valid statistical approaches are accessible to generate less biased results, or more extensive GWAS databases are available to obtain more accurate MR estimates. Secondly, further mechanistic studies and large-sample, multicenter, and follow-up studies are also warranted to validate our results in the real world. MR will need further to analyze other types of dementia and all-cause dementia when the GAWS database becomes more extensive. Thirdly, it is also important to emphasize that our MR analysis only investigates the genetic relationships, but other types of causal relationships cannot be revealed.
The original contributions presented in the study are included in the article/Supplementary Material, further inquiries can be directed to the corresponding author/s.
Ethical review and approval was not required for the study on human participants in accordance with the local legislation and institutional requirements. Written informed consent for participation was not required for this study in accordance with the national legislation and the institutional requirements.
YD: conceived and designed the study. YG and TJ: data collection, data analysis, and drafting of the paper. YC: consulted literature and helped with the language editing. The article's submission was reviewed and approved by all authors. All authors contributed to the article and approved the submitted version.
The Zhejiang Provincial Natural Science Foundation of China funded this research with Grant No. LGF18H020007.
We are thankful to the institutions and groups that provided the GWAS database.
The authors declare that the research was conducted in the absence of any commercial or financial relationships that could be construed as a potential conflict of interest.
All claims expressed in this article are solely those of the authors and do not necessarily represent those of their affiliated organizations, or those of the publisher, the editors and the reviewers. Any product that may be evaluated in this article, or claim that may be made by its manufacturer, is not guaranteed or endorsed by the publisher.
The Supplementary Material for this article can be found online at: https://www.frontiersin.org/articles/10.3389/fcvm.2023.1071574/full#supplementary-material.
1. O'Brien JT, Thomas A. Vascular dementia. Lancet. (2015) 386(10004):1698–706. doi: 10.1016/S0140-6736(15)00463-8
2. Iadecola C. The pathobiology of vascular dementia. Neuron. (2013) 80(4):844–66. doi: 10.1016/j.neuron.2013.10.008
3. Wolters FJ, Ikram MA. Epidemiology of dementia: the burden on society, the challenges for research. Methods Mol Biol. (2018) 1750:3–14. doi: 10.1007/978-1-4939-7704-8_1
4. Satizabal CL, Beiser AS, Chouraki V, Chêne G, Dufouil C, Seshadri S. Incidence of dementia over three decades in the framingham heart study. N Engl J Med. (2016) 374(6):523–32. doi: 10.1056/NEJMoa1504327
5. Wu YT, Beiser AS, Breteler MMB, Fratiglioni L, Helmer C, Hendrie HC, et al. The changing prevalence and incidence of dementia over time—current evidence. Nat Rev Neurol. (2017) 13(6):327–39. doi: 10.1038/nrneurol.2017.63.28497805
6. Grande G, Qiu C, Fratiglioni L. Prevention of dementia in an ageing world: evidence and biological rationale. Ageing Res Rev. (2020) 64:101045. doi: 10.1016/j.arr.2020.101045
7. Gorelick PB, Counts SE, Nyenhuis D. Vascular cognitive impairment and dementia. Biochim Biophys Acta. (2016) 1862(5):860–8. doi: 10.1016/j.bbadis.2015.12.015
8. Zimetbaum P. Atrial fibrillation. Ann Intern Med. (2017) 166(5):Itc33–itc48. doi: 10.7326/AITC201703070
9. Heijman J, Linz D, Schotten U. Dynamics of atrial fibrillation mechanisms and comorbidities. Annu Rev Physiol. (2021) 83:83–106. doi: 10.1146/annurev-physiol-031720-085307
10. Lippi G, Sanchis-Gomar F, Cervellin G. Global epidemiology of atrial fibrillation: an increasing epidemic and public health challenge. Int J Stroke. (2021) 16(2):217–21. doi: 10.1177/1747493019897870
11. Papanastasiou CA, Theochari CA, Zareifopoulos N, Arfaras-Melainis A, Giannakoulas G, Karamitsos TD, et al. Atrial fibrillation is associated with cognitive impairment, all-cause dementia, vascular dementia, and Alzheimer's Disease: a systematic review and meta-analysis. J Gen Intern Med. (2021) 36(10):3122–35. doi: 10.1007/s11606-021-06954-8
12. Emdin CA, Khera AV, Kathiresan S. Mendelian randomization. JAMA. (2017) 318(19):1925–6. doi: 10.1001/jama.2017.17219
13. Davey Smith G, Hemani G. Mendelian randomization: genetic anchors for causal inference in epidemiological studies. Hum Mol Genet. (2014) 23(R1):R89–98. doi: 10.1093/hmg/ddu328
14. Smith GD, Ebrahim S. Mendelian randomization: prospects, potentials, and limitations. Int. J. Epidemiol. (2004) 33:30–42. doi: 10.1093/ije/dyh132
15. Boef AG, Dekkers OM, le Cessie S. Mendelian randomization studies: a review of the approaches used and the quality of reporting. Int J Epidemiol. (2015) 44(2):496–511. doi: 10.1093/ije/dyv071
16. Nielsen JB, Thorolfsdottir RB, Fritsche LG, Zhou W, Skov MW, Graham SE, et al. Biobank-Driven genomic discovery yields new insight into atrial fibrillation biology. Nat Genet. (2018) 50(9):1234–9. doi: 10.1038/s41588-018-0171-3
17. Bowden J, Del Greco MF, Minelli C, Davey Smith G, Sheehan NA, Thompson JR. Assessing the suitability of summary data for two-sample Mendelian randomization analyses using mr-egger regression: the role of the I2 statistic. Int J Epidemiol. (2016) 45(6):1961–74. doi: 10.1093/ije/dyw220
18. Kamat MA, Blackshaw JA, Young R, Surendran P, Burgess S, Danesh J, et al. Phenoscanner V2: an expanded tool for searching human genotype-phenotype associations. Bioinformatics. (2019) 35(22):4851–3. doi: 10.1093/bioinformatics/btz469
19. Zuber V, Cameron A, Myserlis EP, Bottolo L, Fernandez-Cadenas I, Burgess S, et al. Leveraging genetic data to elucidate the relationship between COVID-19 and ischemic stroke. J Am Heart Assoc. (2021) 10(22):e022433. doi: 10.1161/JAHA.121.022433
20. Hu X, Zhao J, Lin Z, Wang Y, Peng H, Zhao H, et al. Mendelian randomization for causal inference accounting for pleiotropy and sample structure using genome-wide summary statistics. Proc Natl Acad Sci U S A. (2022) 119(28):e2106858119. doi: 10.1073/pnas.2106858119
21. Pierce BL, Burgess S. Efficient design for Mendelian randomization studies: subsample and 2-sample instrumental variable estimators. Am J Epidemiol. (2013) 178(7):1177–84. doi: 10.1093/aje/kwt084
22. Hemani G, Zheng J, Elsworth B, Wade KH, Haberland V, Baird D, et al. The mr-base platform supports systematic causal inference across the human phenome. Elife. (2018) 7:1–29. doi: 10.7554/eLife.34408
23. Hartwig FP, Davies NM, Hemani G, Davey Smith G. Two-sample Mendelian randomization: avoiding the downsides of a powerful, widely applicable but potentially fallible technique. Int J Epidemiol. (2016) 45(6):1717–26. doi: 10.1093/ije/dyx028
24. Bowden J, Davey Smith G, Haycock PC, Burgess S. Consistent estimation in Mendelian randomization with some invalid instruments using a weighted median estimator. Genet Epidemiol. (2016) 40(4):304–14. doi: 10.1002/gepi.21965
25. Burgess S, Thompson SG. Erratum to: interpreting findings from Mendelian randomization using the mr-egger method. Eur J Epidemiol. (2017) 32(5):391–2. doi: 10.1007/s10654-017-0276-5
26. Burgess S, Thompson SG. Interpreting findings from Mendelian randomization using the mr-egger method. Eur J Epidemiol. (2017) 32(5):377–89. doi: 10.1007/s10654-017-0255-x
27. Verbanck M, Chen CY, Neale B, Do R. Detection of widespread horizontal pleiotropy in causal relationships inferred from Mendelian randomization between complex traits and diseases. Nat Genet. (2018) 50(5):693–8. doi: 10.1038/s41588-018-0099-7
28. Kennedy OJ, Pirastu N, Poole R, Fallowfield JA, Hayes PC, Grzeszkowiak EJ, et al. Coffee consumption and kidney function: a Mendelian randomization study. Am J Kidney Dis. (2020) 75(5):753–61. doi: 10.1053/j.ajkd.2019.08.025
29. Liu DS, Chen J, Jian WM, Zhang GR, Liu ZR. The association of atrial fibrillation and dementia incidence: a meta-analysis of prospective cohort studies. J Geriatr Cardiol. (2019) 16(3):298–306. doi: 10.11909/j.issn.1671-5411.2019.03.006
30. Islam MM, Poly TN, Walther BA, Yang HC, Wu CC, Lin MC, et al. Association between atrial fibrillation and dementia: a meta-analysis. Front Aging Neurosci. (2019) 11:305. doi: 10.3389/fnagi.2019.00305
31. Kwok CS, Loke YK, Hale R, Potter JF, Myint PK. Atrial fibrillation and incidence of dementia: a systematic review and meta-analysis. Neurology. (2011) 76(10):914–22. doi: 10.1212/WNL.0b013e31820f2e38
32. de Bruijn RF, Heeringa J, Wolters FJ, Franco OH, Stricker BH, Hofman A, et al. Association between atrial fibrillation and dementia in the general population. JAMA Neurol. (2015) 72(11):1288–94. doi: 10.1001/jamaneurol.2015.2161
33. Marzona I, O'Donnell M, Teo K, Gao P, Anderson C, Bosch J, et al. Increased risk of cognitive and functional decline in patients with atrial fibrillation: results of the ontarget and transcend studies. CMAJ. (2012) 184(6):E329–36. doi: 10.1503/cmaj.111173
34. Pan Y, Wang Y, Wang Y. Investigation of causal effect of atrial fibrillation on alzheimer disease: a Mendelian randomization study. J Am Heart Assoc. (2020) 9(2):e014889. doi: 10.1161/jaha.119.014889
35. Iadecola C, Duering M, Hachinski V, Joutel A, Pendlebury ST, Schneider JA, et al. Vascular cognitive impairment and dementia: jacc scientific expert panel. J Am Coll Cardiol. (2019) 73(25):3326–44. doi: 10.1016/j.jacc.2019.04.034
36. Cortes-Canteli M, Gispert JD, Salvadó G, Toribio-Fernandez R, Tristão-Pereira C, Falcon C, et al. Subclinical atherosclerosis and brain metabolism in middle-aged individuals: the pesa study. J Am Coll Cardiol. (2021) 77(7):888–98. doi: 10.1016/j.jacc.2020.12.027
37. Mielke MM, Rosenberg PB, Tschanz J, Cook L, Corcoran C, Hayden KM, et al. Vascular factors predict rate of progression in Alzheimer disease. Neurology. (2007) 69(19):1850–8. doi: 10.1212/01.wnl.0000279520.59792.fe
38. Eastman JA, Kaup AR, Bahorik AL, Butcher X, Attarha M, Marcus GM, et al. Remote assessment of cardiovascular risk factors and cognition in middle-aged and older adults: proof-of-concept study. JMIR Form Res. (2022) 6(2):e30410. doi: 10.2196/30410
39. Chen LY, Agarwal SK, Norby FL, Gottesman RF, Loehr LR, Soliman EZ, et al. Persistent but not paroxysmal atrial fibrillation is independently associated with lower cognitive function: aric study. J Am Coll Cardiol. (2016) 67(11):1379–80. doi: 10.1016/j.jacc.2015.11.064
40. Gardarsdottir M, Sigurdsson S, Aspelund T, Rokita H, Launer LJ, Gudnason V, et al. Atrial fibrillation is associated with decreased total cerebral blood flow and brain perfusion. Europace. (2018) 20(8):1252–8. doi: 10.1093/europace/eux220
41. Tang RB, Dong JZ, Liu XP, Ma CS. Inflammation and atrial fibrillation: is chlamydia pneumoniae a candidate pathogen of atrial fibrillation? Med Hypotheses. (2006) 67(3):462–6. doi: 10.1016/j.mehy.2006.03.017
42. Zhang J, Zhang Y, Liu Y, Niu X. Naringenin attenuates cognitive impairment in a rat model of vascular dementia by inhibiting hippocampal oxidative stress and inflammatory response and promoting N-methyl-D-aspartate receptor signaling pathway. Neurochem Res. (2022) 47(11):3402–13. doi: 10.1007/s11064-022-03696-9
43. Trares K, Bhardwaj M, Perna L, Stocker H, Petrera A, Hauck SM, et al. Association of the inflammation-related proteome with dementia development at older age: results from a large, prospective, population-based cohort study. Alzheimers Res Ther. (2022) 14(1):128. doi: 10.1186/s13195-022-01063-y
44. Deguchi I, Fukuoka T, Hayashi T, Maruyama H, Sehara Y, Kato Y, et al. Clinical outcomes of persistent and paroxysmal atrial fibrillation in patients with stroke. J Stroke Cerebrovasc Dis. (2014) 23(10):2840–4. doi: 10.1016/j.jstrokecerebrovasdis.2014.07.010
45. Barber M, Tait RC, Scott J, Rumley A, Lowe GD, Stott DJ. Dementia in subjects with atrial fibrillation: hemostatic function and the role of anticoagulation. J Thromb Haemost. (2004) 2(11):1873–8. doi: 10.1111/j.1538-7836.2004.00993.x
46. Lee SR, Choi EK, Park SH, Jung JH, Han KD, Oh S, et al. Comparing warfarin and 4 direct oral anticoagulants for the risk of dementia in patients with atrial fibrillation. Stroke. (2021) 52(11):3459–68. doi: 10.1161/STROKEAHA.120.033338
47. Chao TF, Liu CJ, Chen SJ, Wang KL, Lin YJ, Chang SL, et al. Statins and the risk of dementia in patients with atrial fibrillation: a nationwide population-based cohort study. Int J Cardiol. (2015) 196:91–7. doi: 10.1016/j.ijcard.2015.05.159
Keywords: atrial fibrillation, vascular dementia, causality, Mendelian randomization, risk
Citation: Gao Y-f, Jin T-y, Chen Y and Ding Y-h (2023) No causal genetic relationships between atrial fibrillation and vascular dementia: A bidirectional Mendelian randomization study. Front. Cardiovasc. Med. 10:1071574. doi: 10.3389/fcvm.2023.1071574
Received: 16 October 2022; Accepted: 20 March 2023;
Published: 30 June 2023.
Edited by:
Li Ni, Huazhong University of Science and Technology, ChinaReviewed by:
Jingxin Li, Jiangsu Provincial Center for Disease Control and Prevention, China© 2023 Gao, Jin, Chen and Ding. This is an open-access article distributed under the terms of the Creative Commons Attribution License (CC BY). The use, distribution or reproduction in other forums is permitted, provided the original author(s) and the copyright owner(s) are credited and that the original publication in this journal is cited, in accordance with accepted academic practice. No use, distribution or reproduction is permitted which does not comply with these terms.
*Correspondence: Ya-hui Ding ZGluZ3loQHpqaGVhcnQuY29t
†These authors have contributed equally to this work
Specialty Section: This article was submitted to Cardiac Rhythmology, a section of the journal Frontiers in Cardiovascular Medicine
Disclaimer: All claims expressed in this article are solely those of the authors and do not necessarily represent those of their affiliated organizations, or those of the publisher, the editors and the reviewers. Any product that may be evaluated in this article or claim that may be made by its manufacturer is not guaranteed or endorsed by the publisher.
Research integrity at Frontiers
Learn more about the work of our research integrity team to safeguard the quality of each article we publish.