- 1Guangdong Provincial Key Laboratory of Traditional Chinese Medicine Information Technology, Jinan University, Guangzhou, China
- 2College of Information Science and Technology, Jinan University, Guangzhou, China
- 3Auckland Bioengnieering Institute, The University of Auckland, Auckland, New Zeanland
- 4Department of Obstetrics and Gynecology, Guangzhou Women and Children's Medical Center, Preterm Birth Prevention and Treatment Research Unit, Guangzhou Medical University, Guangzhou, China
- 5Department of Obstetrics, NanFang Hospital of Southen Medical University, Guangzhou, China
- 6Department of Obstetrics, Shenzhen People's Hospital, Shenzhen, China
Background: This study aims to compare the fetal heart rate (FHR) baseline predicted by the cardiotocograph network (CTGNet) with that estimated by clinicians.
Material and methods: A total of 1,267 FHR recordings acquired with different electrical fetal monitors (EFM) were collected from five datasets: 84 FHR recordings acquired with F15 EFM (Edan, Shenzhen, China) from the Guangzhou Women and Children's Medical Center, 331 FHR recordings acquired with SRF618B5 EFM (Sanrui, Guangzhou, China), 234 FHR recordings acquired with F3 EFM (Lian-Med, Guangzhou, China) from the NanFang Hospital of Southen Medical University, 552 cardiotocographys (CTG) recorded using STAN S21 and S31 (Neoventa Medical, Mölndal, Sweden) and Avalon FM40 and FM50 (Philips Healthcare, Amsterdam, The Netherlands) from the University Hospital in Brno, Czech Republic, and 66 FHR recordings acquired using Avalon FM50 fetal monitor (Philips Healthcare, Amsterdam, The Netherlands) at St Vincent de Paul Hospital (Lille, France). Each FHR baseline was estimated by clinicians and CTGNet, respectively. And agreement between CTGNet and clinicians was evaluated using the kappa statistics, intra-class correlation coefficient, and the limits of agreement.
Results: The number of differences <3 beats per minute (bpm), 3-5 bpm, 5–10 bpm and ≥10 bpm, is 64.88%, 15.94%, 14.44% and 4.74%, respectively. Kappa statistics and intra-class correlation coefficient are 0.873 and 0.969, respectively. Limits of agreement are −6.81 and 7.48 (mean difference: 0.36 and standard deviation: 3.64).
Conclusion: An excellent agreement was found between CTGNet and clinicians in the baseline estimation from FHR recordings with different signal loss rates.
1. Introduction
Although deaths in children have declined substantially in the past 30 years, more than 5 million still die every year (1). Electronic fetal heart rate (FHR) monitoring was introduced to detect fetuses' pathological states as early as possible in the obstetrics practice in the late 1950s. However, the misinterpretation and ambiguity of FHR patterns may increase unnecessary interventions, such as operative deliveries and cesarean sections (2–4). Different guidelines over the past decades have recommended some modifications for interpreting FHR tracings, but beliefs in the etiology of basic FHR patterns (including the baseline, the variability, accelerations, decelerations, and sinusoidal patterns) have remained essentially unchanged (5, 6). In these FHR patterns, the baseline is a pre-requisite for evaluating the other patterns (7). Gynecologists and obstetricians usually estimated the baseline by visual analysis, but the unreliability of visual interpretation with a high degree of inter-and intra-observer variability is found (7–12). Therefore, computer-assisted analysis has been sought to mitigate the variability of visual explanation (13–17).
Several studies have evaluated the performance of different computer-assisted methods. For example, in 2016, Jezewski et al. evaluated 11 different algorithms using two inconsistency coefficients based on three properties (i.e., number, location and area) of accelerations/accelerations (18). They found that the algorithm of Arduini et al. (9, 19) outperforms other methods by achieving the lowest mean inconsistency coefficients on a private dataset with 41 FHR signals. This nonlinear filtering method is similar to the algorithm proposed by Mantel et al. (20). The difference is that Arduini's baseline is computed in 10 min windows with 5 min shift, whereas Mantel's baseline is calculated for the whole FHR tracing. Considering Mantel's method, Houzé de l'Aulnoit et al. further evaluated 11 newer algorithms by comparing the computed baselines with that estimated by clinicians on a dataset with 90 FHR signals (13). This study found that Lu and Wei's algorithm (14) achieves better results than other methods by achieving a new morphological analysis discriminant index (MADI) of 7.3%. Recently, a weighted median filter was proposed by Boudet et al. to compute the FHR baseline, and more agreement (represented by a MADI of 4.0%) with clinicians' consensus than Lu and Wei's method was shown on this dataset with 90 FHR recordings (15). Similar to Lu and Wei's method, an algorithm for the baseline estimation based on singular spectrum analysis and empirical mode decomposition was also proposed by Lu et al. (16) and evaluated on another public dataset with 552 FHR recordings. This method also was objectively evaluated on the dataset with 90 FHR recordings (13) by achieving a MADI of 15.6%. Unlike signal processing methods, the CTGNet based on deep learning was proposed in our previous study (21) and evaluated on a larger dataset with 234 FHR recordings. This method was compared with 12 signal processing methods and the lowest metrics (including the root-mean-squared difference between baselines and MADI) were obtained. These methodological studies illustrate the excellent performance of the CTGNet. However, its clinical application still requires a comparative study with large-scale multicenter data.
To evaluate the clinical usability of the CTGNet, we compare the FHR baseline predicted by the CTGNet with that estimated by clinicians using a large dataset with 1,267 FHR recordings acquired with fetal monitors of five device manufacturers.
2. Material and methods
2.1. Data source
This prospective assessment study used 1,267 FHR recordings from 5 datasets covering the years 2011 to 2021: (1) an FHR dataset with 84 recordings collected from the Guangzhou Women and Children's Medical Center of Guangzhou Medical University (GMU_DB; May 2021 to July 2021); (2) a dataset with 331 CTG records of the First Affiliated Hospital of Jinan University (JNU_DB; January 2015 to December 2020); (3) a dataset with 234 CTG records collected from the NanFang Hospital of Southern Medical University (SMU_DB; January 2012 to December 2020); (4) the open-access database with 66 FHR recordings collected at Saint Vincent de Paul Maternity Hospital of Lille Catholic University (LCU_DB; February 1st, 2011, and December 31st, 2016) (13, 22, 23) and the open-access database with 552 CTG recordings collected at the obstetrics ward of the University Hospital in Brno (UHB_DB; April 2010 and August 2012) (24–26). In these datasets, the signal loss rate of FHR recordings from GMU_DB, JNU_DB and SMU_DB is <10% per 10 min, whereas those from GMU_DB and UHB_DB are <7% and <50%, respectively (Figure 1).
The Medical Ethics Committees of the Guangzhou Women and Children's Medical Center (273A01), the Jinan University (JNUKY-2022-018) and the NanFang Hospital of Southen Medical University (NFEC-2019-024) approved this retrospective study.
2.2. Devices
Fetal monitors used for acquiring FHR recordings include: F15 EFM (Edan, Shenzhen, China), SRF618B5 EFM (Sanrui, Guangzhou, China), F3 EFM (Lian-Med, Guangzhou, China), STAN S21 and S31 (Neoventa Medical, Mölndal, Sweden), and Avalon FM40 and FM50 (Philips Healthcare, Amsterdam, The Netherlands).
2.3. Methods
According to the baseline definition of the FIGO consensus guideline (5): (1) the baseline is estimated as the mean level of the most horizontal and less oscillatory FHR segments of 10 min; (2) It is necessary to review previous and subsequent 10 min sections to estimate the baseline in recordings with unstable FHR signals. Clinicians (Z.Z. and X.P.) with more than seven years of experience in CTG analysis independently assessed the baselines of FHR tracings. In order to obtain a consistent baseline, FHR recordings were re-evaluated when the differences between clinicians exceeded three bpm, and the baseline was determined as an average of these clinicians’ estimations when their difference was less than three bpm. A difference between the baseline estimated by clinicians and that predicted with the CTGNet were was then computed to evaluate their agreement.
2.4. Statistical analysis
For each FHR recording, baseline values estimated by CTGNet and the consensus of clinicians were attributed to 5 bpm classes (such as class 0: ≤100 bpm, class 1: 100 <baseline ≤105, and class 2: 105 <baseline ≤110) in the following manner (7): (1) when the baseline difference does not exceed five bpm, CTGNet's and clinicians' baselines are assigned to the same class according to their mean (e.g., if CTGNet's baseline value is 109 and clinicians' estimation is 113, both values are assigned to the class 110–115); (2) when the baseline difference exceeds five bpm, baseline values are assigned to their respective classes. Kappa and intra-class correlation (ICC) coefficient values (i.e., excellent agreement: >0.75, good agreement: 0.4–0.75 and poor agreement: <0.4) were calculated to evaluate agreement in the baseline estimation and 95% confidence intervals (95% CI) were computed for all results.
3. Results
Table 1 summarizes the comparisons of the baselines predicted by the CTGNet and those estimated by clinicians. In 99% of FHR recordings from GMU_DB, JNU_DB and SMU_DB, differences do not exceed 5 bpm, whereas the percentages of differences less than 5 bpm dropped to 58%–85% in FHR recordings from LCU_DB and UHB_DB. For the baseline difference ≥5 bpm, proportions are <0.5% for datasets from China (i.e., GMU_DB, JNU_DB and SMU_DB), 13.6% for LCU_DB, and 42.2% for UHB_DB, respectively. In general, the ratio of differences <5 bpm and ≥5 bpm is 4:1 among the 1,267 FHR recordings.
According to Kappa values (i.e., excellent agreement: Kappa >0.75, good agreement: 0.4 ≤Kappa ≤0.75 and poor agreement: Kappa <0.4), agreement in the baseline estimation is excellent for different datasets. However, Kappa values for datasets from China (i.e., GMU_DB, JNU_DB and SMU_DB) are >0.98, whereas those for LCU_DB and UHB_DB are 0.885 and 0.771, respectively. For 1,267 FHR recordings, the Kappa value and the correlation coefficient between manual measurement and CTGNet calculation are 0.873 and 0.969, respectively.
Figures 2A–E are Bland-Altman plots demonstrating the interchangeability of the clinical measurement and the CTGNet for the baseline estimation. The mean differences are <1. Limits of agreement for GMU_DB, JNU_DB, SMU_DB, LUC_DB and UHB_DB, respectively, are −0.82/0.93, −1.34/0.93, −1.63/3.39, −13.10/14.52 and −9.05/9.90. Maximum differences for GMU_DB, JNU_DB, SMU_DB, LUC_DB and UHB_DB, are 2.81 bpm, 3.43 bpm, 16.52 bpm, 48.88 bpm and 18.86 bpm, respectively.
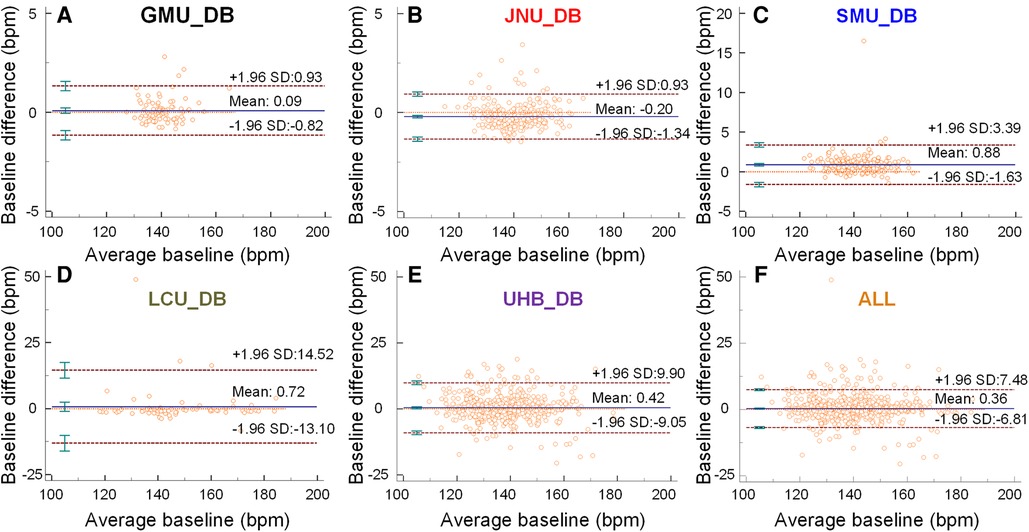
Figure 2. The baselines predicted by the CTGNet vs. those estimated by clinicians. (A–F) Bland-Altman plots for a comparison between automatic measurement and manual-measurement results about baselines on GMU_DB, JNU_DB, SMU_DB, LUC_DB, UHB_DB and ALL, respectively. Mean ±1.96 standard deviation (SD) is given.
These most divergent examples in each database are shown in Figures 3A–F. It can be observed that the difference is large when the signal loss rate increases. Anyway, on the whole dataset including 1,267 FHR recordings, the mean difference, deviation and limits of agreement are 0.36, 3.64 and −6.81/7.48, respectively.
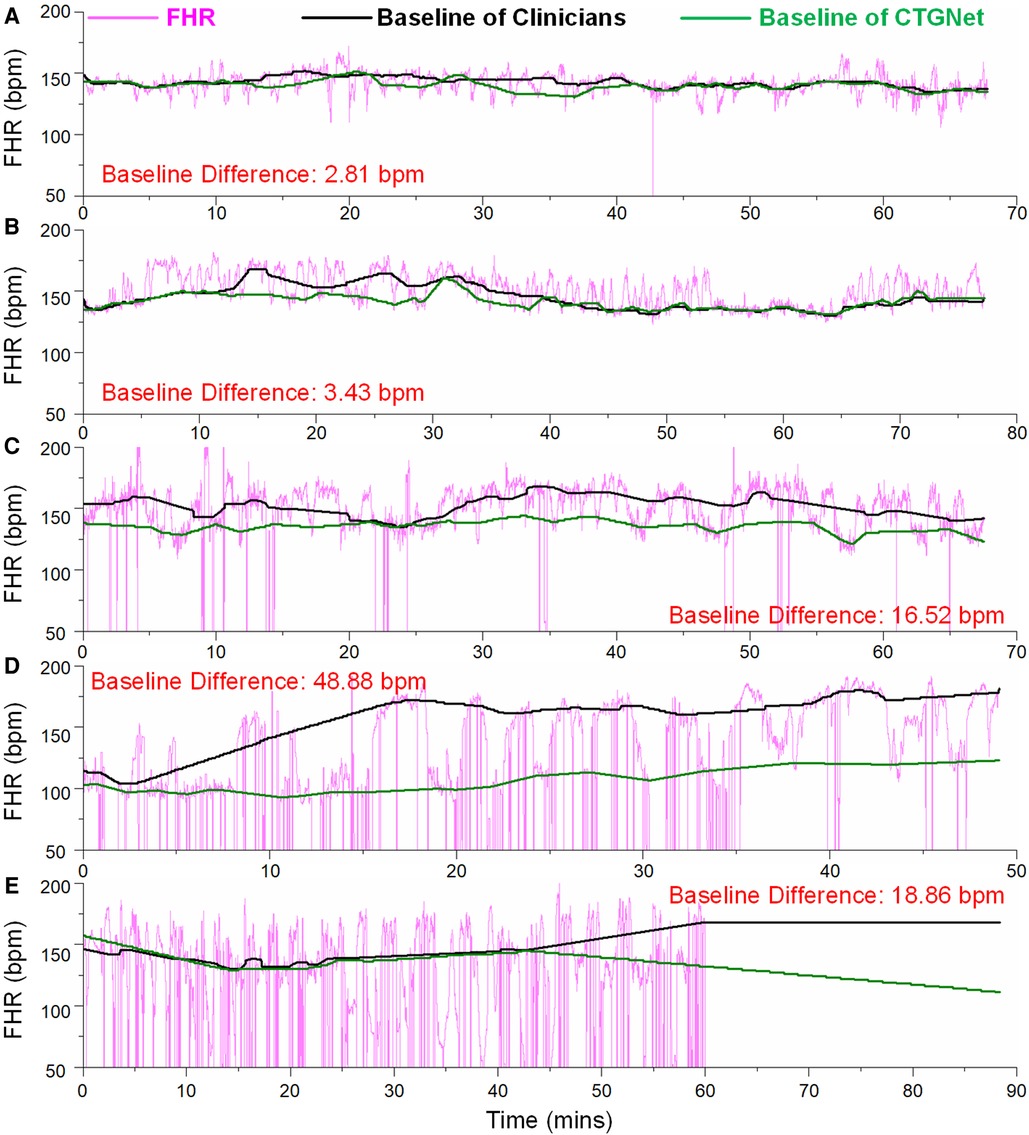
Figure 3. The most divergent examples in each database. (A) The maximum difference of 2.81 bpm on the FHR tracing from GMU_DB; (B) the maximum difference of 3.43 bpm on the FHR tracing from JNU_DB; (C) the maximum difference of 16.52 bpm on the FHR tracing from SMU_DB; (D) the maximum difference of 48.88 bpm on the FHR tracing from LUC_DB; (E) the maximum difference of 18.86 bpm on the FHR tracing from UHB_DB.
4. Discussion
Reliable FHR interpretation is the base of the fetal state assessment. The poor recognition performance of FHR patterns can propagate the error to subsequent steps, thereby decreasing classification accuracy. In all these FHR patterns, the baseline is a precondition for evaluating of the other patterns. Visual estimation of the FHR baseline is subject to inter-and intra-observer variability. Computer-assisted baseline estimation has been proposed as a promising way to reduce this variability. In order to evaluate the performance of our computer-assisted method (i.e., CTGNet), a comparison of FHR baseline estimation by the CTGNet and a consensus of clinicians presents in this study.
Baselines assigned by computer-assisted methods had been compared with those estimated by clinicians in several studies (7–12). In the studies of Arduini et al. and Ayres-de-Campo et al., limits of agreement (LoA) were no more than −6.45 and 7.07 in ≤150 FHR tracings. In addition, Kappa value and ICC coefficient also were used to evaluate agreement in baseline determination between a computer-assisted method and several experts in previous studies. Obtained Kappa values varied from 0.18 to 0.97, while the ICC coefficient was within the range of 0.83–0.98 (Table 2). All these results were obtained on several small datasets with ≤150 FHR tracings acquired with EFM from ≤3 different manufacturers. In the present study, a larger dataset with 1,267 FHR tracings acquired with EFM from 5 different manufacturers was used to evaluate agreement in baseline determination between the CTGNet and clinicians. This dataset included ∼50% high-quality tracings with a signal loss rate of <10% per 10 min and ∼50% low-quality recordings with a signal loss rate of <50% per 30 min. Kappa values were >0.98 for these high-quality tracings from GMU_DB, JNU_DB and SMU_DB, while the Kappa value was 0.771 for the lowest-quality FHR recordings (n = 552) from UHB_DB. Regardless, an excellent agreement was obtained on the whole dataset (n = 1,276). These results indicate possibilities for the clinical application of CTGNet in FHR baseline estimation.
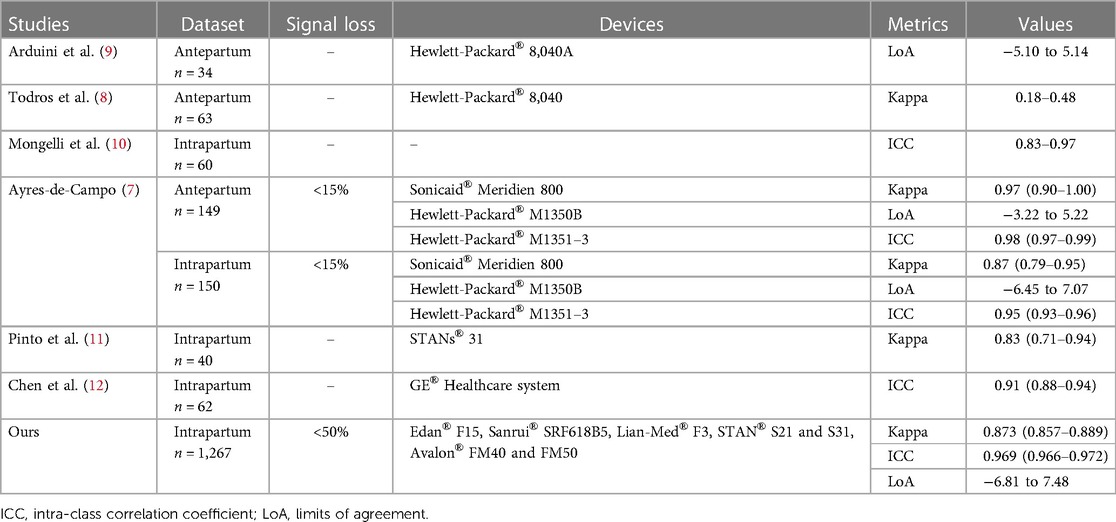
Table 2. Studies of comparison between baselines assigned by computer-assisted methods and those estimated by clinicians.
The high loss rate of FHR tracings severely affected the performance of CTGNet. In the present study, the Kappa value was reduced from 1.0 for the FHR recordings from JNU_DB with a low signal loss rate to 0.771 for the low-quality recordings from UHB_DB with a high signal loss rate. Although high-quality recordings with a signal loss of ≤20% are recommended by the FIGO guidelines to assess the FHR patterns (5), low-quality tracings with a mean signal loss of 28%–55% are shown in clinical practice (27, 28). For example, the mean signal loss of 13% and 30% were found during the first and the second stage of labor, respectively (29). Therefore, the CTGNet can be further trained on datasets with low-quality tracings to improve its robustness.
On these five datasets, we further compared the performance of our method (30) with existing signal processing methods. The method proposed by Mantal et al. achieved the best performance on the GMU_DB and JNU_DB datasets. Our method achieved the best results on the JNU_DB and LCU_DB datasets. The method proposed by Boudet et al. achieved the best results on the UHB_DB dataset. In the comprehensive evaluation of the five datasets, Boudet's method and our method ranked first and second respectively, and their baseline differences were both less than 3 bpm (Table 3).
5. Conclusions
The CTGNet for the FHR baseline estimation provided an excellent agreement with clinicians. However, this occurs in FHR recordings with low and medium signal loss rates. In the future, the CTGNet can be further improved by training it with more low-quality tracings.
Data availability statement
The original contributions presented in the study are included in the article, further inquiries can be directed to the corresponding authors.
Ethics statement
The Medical Ethics Committees of the Guangzhou Women and Children's Medical Center (273A01), the Jinan University (JNUKY-2022-018) and the NanFang Hospital of Southen Medical University (NFEC-2019-024) approved this study. Written informed consent was not required to participate in this study in accordance with the national legislation and the institutional requirements.
Author contributions
Conceptualization, JB, XP, YL, ZZ, and HW; writing-original draft preparation, JB and XP; writing-review and editing, JB, ZZ, and XP; visualization, JB; funding acquisition, HW, JB, and YL; data collection, ZZ, YL, XG, and JB; data annotation, XP and ZZ. All authors contributed to the article and approved the submitted version.
Funding
This research was funded by the Guangdong Basic and Applied Basic Research Foundation (2023A1515012833) (JB), the Science and Technology Program of Guangzhou (202201010544) (JB), Guangdong Provincial Key Laboratory of Traditional Chinese Medicine Informatization (2021B1212040007) (YL), and the China Scholarship Council (JB).
Conflict of interest
The authors declare that the research was conducted in the absence of any commercial or financial relationships that could be construed as a potential conflict of interest.
Publisher's note
All claims expressed in this article are solely those of the authors and do not necessarily represent those of their affiliated organizations, or those of the publisher, the editors and the reviewers. Any product that may be evaluated in this article, or claim that may be made by its manufacturer, is not guaranteed or endorsed by the publisher.
References
1. Ashorn P, Black RE, Lawn JE, Ashorn U, Klein N, Hofmeyr J, et al. The lancet small vulnerable newborn series: science for a healthy start. Lancet. (2020) 396(10253):743–5. doi: 10.1016/S0140-6736(20)31906-1
2. Ekengård F, Cardell M, Herbst A. Low sensitivity of the new FIGO classification system for electronic fetal monitoring to identify fetal acidosis in the second stage of labor. Eur J Obstet Gynecol Reprod Biol: X. (2021) 9:100120. doi: 10.1016/j.eurox.2020.100120
3. Jonsson M, Söderling J, Ladfors L, Nordström L, Nilsson M, Algovik M, et al. Implementation of a revised classification for intrapartum fetal heart rate monitoring and association to birth outcome: a national cohort study. Acta Obstet Gynecol Scand. (2022) 101(2):183–92. doi: 10.1111/aogs.14296
4. Vejux N, Ledu R, D’ercole C, Piechon L, Loundou A, Bretelle F. Guideline choice for CTG analysis influences first caesarean decision. J Matern-Fetal Neonatal Med. (2017) 30(15):1816–9. doi: 10.1080/14767058.2016.1228050
5. Ayres-De-Campos D, Spong CY, Chandraharan E, Panel FIFMEC. FIGO Consensus guidelines on intrapartum fetal monitoring: cardiotocography. Int J Gynaecol Obstet. (2015) 131(1):13–24. doi: 10.1016/j.ijgo.2015.06.020
6. Mohan M, Ramawat J, La Monica G, Jayaram P, Fattah SA, Learmont J, et al. Electronic intrapartum fetal monitoring: a systematic review of international clinical practice guidelines. AJOG Global Reports. (2021) 1(2):100008. doi: 10.1016/j.xagr.2021.100008
7. Ayres-de-Campos D, Bernardes J. Comparison of fetal heart rate baseline estimation by SisPorto 2.01 and a consensus of clinicians. Eur J Obstet Gynecol Reprod Biol. (2004) 117(2):174–8. doi: 10.1016/j.ejogrb.2004.03.013
8. Todros T, Preve CU, Plazzotta C, Biolcati M, Lombardo P. Fetal heart rate tracings: observers versus computer assessment. Eur J Obstet Gynecol Reprod Biol. (1996) 68:83–6. doi: 10.1016/0301-2115(96)02487-6
9. Arduini D, Rizzo G, Giannini F, Garzetti G, Romanini C. Computerized analysis of fetal heart-rate. 2. Comparison with the interpretation of experts. J Matern-Fetal Investig. (1993) 3(3):165–8.
10. Mongelli M, Dawkins R, Chung T, Sahota D, Spencer JA, Chang AM. Computerised estimation of the baseline fetal heart rate in labour: the low frequency line. Br J Obstet Gynaecol. (1997) 104(10):1128–33. doi: 10.1111/j.1471-0528.1997.tb10935.x
11. Pinto P, Bernardes J, Costa-Santos C, Amorim-Costa C, Silva M, Ayres-de-Campos D. Development and evaluation of an algorithm for computer analysis of maternal heart rate during labor. Comput Biol Med. (2014) 49:30–5. doi: 10.1016/j.compbiomed.2014.03.007
12. Chen C-Y, Yu C, Chang C-C, Lin C-W. Comparison of a novel computerized analysis program and visual interpretation of cardiotocography. Plos One. (2014) 9(12):e112296. doi: 10.1371/journal.pone.0112296
13. De L’aulnoit AH, Boudet S, Demailly R, Delgranche A, Génin M, Peyrodie L, et al. Automated fetal heart rate analysis for baseline determination and acceleration/deceleration detection: a comparison of 11 methods versus expert consensus. Biomed Signal Processing Control. (2019) 49:113–23. doi: 10.1016/j.bspc.2018.10.002
14. Lu Y, Wei S. 2012 IEEE 11th international conference on signal processing. Nonlinear Baseline Estimation Of Fhr Signal Using Empirical Mode Decomposition, IEEE (2012).
15. Boudet S, De L’aulnoit AH, Demailly R, Peyrodie L, Beuscart R, De L’aulnoit DH. Fetal heart rate baseline computation with a weighted median filter. Comput Biol Med. (2019) 114:103468. doi: 10.1016/j.compbiomed.2019.103468
16. Lu Y, Zhang X, Jing L, Li X, Fu X. Estimation of the foetal heart rate baseline based on singular spectrum analysis and empirical mode decomposition. Future Gener Comput Syst. (2020) 112:126–35. doi: 10.1016/j.future.2020.05.008
17. Georgieva A, Abry P, Chudácek V, Djuric PM, Frasch MG, Kok R, et al. Computer-based intrapartum fetal monitoring and beyond: a review of the 2nd workshop on signal processing and monitoring in labor (October 2017, Oxford, UK). Acta Obstet Gynecol Scand. (2019) 98(9):1207–17. doi: 10.1111/aogs.13639
18. Jezewski J, Horoba K, Roj D, Wrobel J, Kupka T, Matonia A. Evaluating the fetal heart rate baseline estimation algorithms by their influence on detection of clinically important patterns. Biocybern Biomed Eng. (2016) 36(4):562–73. doi: 10.1016/j.bbe.2016.06.003
19. Arduini D, Rizzo G, Piana G, Bonalumi A, Brambilla P, Romanini CJJOM-FI. Computerized analysis of fetal heart-rate. 1. Description of the system (2ctg). J Matern-Fetal Investig. (1993) 3(3):159–63.
20. Mantel R, Van Geijn H, Caron F, Swartjes J, Van Woerden E, Jongsma H. Computer analysis of antepartum fetal heart rate: 1. Baseline determination. Int J Biomed Comput. (1990) 25(4):261–72. doi: 10.1016/0020-7101(90)90030-X
21. Zhong M, Yi H, Lai F, Liu M, Zeng R, Kang X, et al. CTGNet: automatic analysis of fetal heart rate from cardiotocograph using artificial intelligence. Maternal-Fetal Med. (2022) 4(02):103–12. doi: 10.1097/FM9.0000000000000147
22. De L’aulnoit AH, Boudet S, Demailly R, Peyrodie L, Beuscart R, De L’aulnoit DH. 2016 38th annual international conference of the IEEE engineering in medicine and biology society (EMBC). Baseline Fetal Heart Rate Analysis: Eleven Automatic Methods Versus Expert Consensus, IEEE (2016).
23. Boudet S, Houzé de l’Aulnoit A, Demailly R, Delgranche A, Peyrodie L, Beuscart R, et al. Fetal heart rate signal dataset for training morphological analysis methods and evaluating them against an expert consensus. (2019):2019070039. doi: 10.20944/preprints201907.0039.v1
24. Chudácek V, Spilka J, Burša M, Janku P, Hruban L, Huptych M, et al. Open access intrapartum CTG database. BMC pregnancy Childbirth. (2014) 14(1):1–12. doi: 10.1186/1471-2393-14-16
25. Romagnoli S, Sbrollini A, Burattini L, Marcantoni I, Morettini M, Burattini L. Annotation dataset of the cardiotocographic recordings constituting the “CTU-CHB intra-partum CTG database”. Data Brief. (2020) 31:105690. doi: 10.1016/j.dib.2020.105690
26. Goldberger AL, Amaral LA, Glass L, Hausdorff JM, Ivanov PC, Mark RG, et al. Physiobank, PhysioToolkit, and PhysioNet: components of a new research resource for complex physiologic signals. Circulation. (2000) 101(23):e215–20. doi: 10.1161/01.CIR.101.23.e215
27. Li Y, Gonik B. Continuous fetal heart rate monitoring in patients with preterm premature rupture of membranes undergoing expectant management. J Matern-Fetal Neonatal Med. (2009) 22(7):589–92. doi: 10.1080/14767050902906378
28. Sänger N, Hayes-Gill B, Schiermeier S, Hatzmann W, Yuan J, Herrmann E, et al. Prenatal foetal non-invasive ECG instead of Doppler CTG–a better alternative? Geburtshilfe Frauenheilkd. (2012) 72(07):630–3. doi: 10.1055/s-0032-1315012
29. Faiz Z, Van’t Hof EM, Colenbrander GJ, Lippes R, Bakker PC. The quality of intrapartum cardiotocography in preterm labour. J Perinat Med. (2022) 50(1):74–81. doi: 10.1515/jpm-2021-0214
Keywords: artificial intelligence, deep learning, fetal heart rate, baseline, computer analysis
Citation: Bai J, Pan X, Lu Y, Zhong M, Wang H, Zheng Z and Guo X (2023) Comparison of fetal heart rate baseline estimation by the cardiotocograph network and clinicians: a multidatabase retrospective assessment study. Front. Cardiovasc. Med. 10:1059211. doi: 10.3389/fcvm.2023.1059211
Received: 8 October 2022; Accepted: 21 July 2023;
Published: 9 August 2023.
Edited by:
Ahsan H. Khandoker, Khalifa University, United Arab EmiratesReviewed by:
Faezeh Marzbanrad, Monash University, AustraliaIgor Victorovich Lakhno, Kharkiv National Medical University, Ukraine
© 2023 Bai, Pan, Lu, Zhong, Wang, Zheng and Guo. This is an open-access article distributed under the terms of the Creative Commons Attribution License (CC BY). The use, distribution or reproduction in other forums is permitted, provided the original author(s) and the copyright owner(s) are credited and that the original publication in this journal is cited, in accordance with accepted academic practice. No use, distribution or reproduction is permitted which does not comply with these terms.
*Correspondence: Zheng Zheng libra2046@126.com Xiaohui Guo 2285902525@qq.com
†These authors have contributed equally to this work