- 1State Key Laboratory of Pathogenesis, Prevention and Treatment of High Incidence Diseases in Central Asia, Department of Cardiology, First Affiliated Hospital of Xinjiang Medical University, Urumqi, China
- 2Xinjiang Key Laboratory of Cardiovascular Disease, Clinical Medical Research Institute, First Affiliated Hospital of Xinjiang Medical University, Urumqi, China
- 3The Emergency Center, First Affiliated Hospital of Xinjiang Medical University, Urumqi, China
Objective: Unplanned admission to the intensive care unit (ICU) is the major in-hospital adverse event for patients with dilated cardiomyopathy (DCM). We aimed to establish a nomogram of individualized risk prediction for unplanned ICU admission in DCM patients.
Methods: A total of 2,214 patients diagnosed with DCM from the First Affiliated Hospital of Xinjiang Medical University from January 01, 2010, to December 31, 2020, were retrospectively analyzed. Patients were randomly divided into training and validation groups at a 7:3 ratio. The least absolute shrinkage and selection operator and multivariable logistic regression analysis were used for nomogram model development. The area under the receiver operating characteristic curve, calibration curves, and decision curve analysis (DCA) were used to evaluate the model. The primary outcome was defined as unplanned ICU admission.
Results: A total of 209 (9.44%) patients experienced unplanned ICU admission. The variables in our final nomogram included emergency admission, previous stroke, New York Heart Association Class, heart rate, neutrophil count, and levels of N-terminal pro b-type natriuretic peptide. In the training group, the nomogram showed good calibration (Hosmer–Lemeshow χ2 = 14.40, P = 0.07) and good discrimination, with an optimal-corrected C-index of 0.76 (95% confidence interval: 0.72–0.80). DCA confirmed the clinical net benefit of the nomogram model, and the nomogram maintained excellent performances in the validation group.
Conclusion: This is the first risk prediction model for predicting unplanned ICU admission in patients with DCM by simply collecting clinical information. This model may assist physicians in identifying individuals at a high risk of unplanned ICU admission for DCM inpatients.
Introduction
Dilated cardiomyopathy (DCM) is characterized by dilatation and impaired function of ventricles (1). Studies have shown that 5–8.34 cases of DCM occur per 100,000 people per year, with the 5-year survival rate of only 50% (2, 3). The incidence of adverse events is an important factor that affects the prognosis of patients with DCM (4, 5). Unplanned intensive care unit (ICU) admission is the major in-hospital adverse event for DCM inpatients. Compared to direct ICU admission, unplanned ICU admission is associated with poorer in-hospital prognosis and substantially mortality rates (6, 7). Additionally, unplanned ICU admission can significantly magnify the psychological stress of patients and their families (8). Therefore, assessing risks from unplanned ICU admission is not only just for managing individualization prognosis but also for improving healthcare quality.
According to the reports, approximately 36% of unplanned ICU admission is preventable; therefore, early identification can effectively improve patient survival and rationalize the use of healthcare resources (9–11). Several scoring systems, such as Early Warning Scores (EWS) and National Early Warning Score (NEWS), have been widely developed and used for identifying patients at risk of early disease progression (12, 13); however, most risk prediction models are established based on general emergency patients, and the generic prediction model fails to fit the featured population (14). In DCM inpatients, to the best of our knowledge, no prediction models have been developed for assessing unplanned ICU admission.
In this study, we retrospectively analyzed 2,214 inpatients with DCM and without planned ICU admission at baseline and aimed to develop a nomogram for individualized prediction of unplanned ICU admission incidents in DCM patients by simply collecting clinical information.
Materials and methods
Study design and population
This study included 2,735 DCM patients from the retrospective cohort study, which was designed to evaluate the clinical outcomes and risk factors of cardiomyopathy, and the detailed protocol has been registered on www.chictr.org.cn (registration number: ChiCTR2200058051). This registration trial included 5,937 patients with primary cardiomyopathy, namely, DCM, hypertrophic cardiomyopathy (HCM), restrictive cardiomyopathy (RCM), arrhythmogenic right ventricular cardiomyopathy (ARVC), and unclassified cardiomyopathy, and the diagnostic criteria refer to the JCS/JHFS 2018 Guideline on the Diagnosis and Treatment of Cardiomyopathies (3). In addition, this registration trial excluded patients who had malignant tumors, hematological malignancy, autoimmune diseases, serious dysfunction of the kidney or liver, pregnant or lactating women, and patients younger than 18 years old. All patients were admitted to the First Affiliated Hospital of Xinjiang Medical University from January 01, 2010, to December 31, 2020, and the data were obtained from electronic medical records and follow-up.
To investigate the individualized risk of unplanned ICU admission in DCM inpatients, a total of 2,735 patients were initially evaluated and 521 were excluded, leading to ultimately 2,214 patients in this study, of which 209 experienced unplanned ICU admission (ICU+) and 2005 were not admitted to the ICU (ICU−). Patient selection and study flow are shown in Figure 1. The inclusion criteria for the present study were as follows (15): (1) left ventricular end-diastolic dimension (LVEDD) >5.0 cm in females and >5.5 cm in males; and (2) left ventricular ejection fraction (LVEF) <45%. We excluded patients who (1) had ischemic heart disease, hypertensive heart disease, valvular heart disease, or congenital heart disease; (2) had direct admission to ICU; (3) had severe hepatic and renal failure; (4) were admitted for surgical procedures; (5) were younger than 18 years; and (6) had incomplete clinical information. Finally, 2,214 eligible patients were included in this study. Electronic medical records were fully reviewed by two independent reviewers according to the inclusion and exclusion criteria. All study personnel had formal training prior to participation in the study.
Data collection
All data were obtained from the first measurement at admission. Demographic data, comorbidities, blood tests, and echocardiographic results were included for all patients. Comorbidities included hypertension, diabetes mellitus, stroke, atrial fibrillation (AF), and chronic obstructive pulmonary disease (COPD).
Patients who reported smoking in the previous 6 months were considered current smokers. Similarly, patients who consumed alcohol in the last half a year were considered current drinkers. Hypertension was defined as patients with at least three resting measurements above 140/90 mmHg taken from at least two separate healthcare visits or history of hypertension with active treatment, as suggested by the American Heart Association (16). Diabetes was defined as having a history of diabetes with using hypoglycemic drugs or random intravenous plasma glucose of 200 mg/dL (11.1 mmol/L), or 2-h plasma glucose of 200 mg/dL (11.1 mmol/L) after an oral glucose tolerance test (OGTT), fasting blood glucose (FPG) of 126 mg/dL (7.0 mmol/L), or hemoglobin A1c (HbA1c) of 6.5% (17). Stoke was diagnosed by magnetic resonance imaging (MRI) combined with clinical neurological dysfunction (18) and a patient known to had stroke prior to this visit was categorized as prior stroke. AF included all types of previously diagnosed AF, including paroxysmal AF, persistent AF, and permanent AF (19). COPD was defined as a disease characterized by persistent respiratory symptoms and airflow limitation and diagnosed by the Global initiative for chronic Obstructive Lung Disease (GOLD) (20). Severe renal insufficiency was defined as estimated glomerular filtration rate (eGFR) <30 mL/min (21). Severe hepatic insufficiency is defined as alanine transaminase (ALT) or aspartate aminotransferase (AST) exceeding the upper limit of normal by a factor of 5, specifically AST >180 U/L and ALT >260 U/L (22).
Outcome assessment
The primary outcome was unplanned ICU admission, which was defined as transfer to the ICU due to deterioration or developed complications (23).
Statistical analysis
All statistical analysis were performed using Social Package for the Social Sciences (SPSS) version 26.0 (SPSS Inc., Chicago, IL, United States), and R software version 4.0.3 (https://cran.r-project.org). The R software mainly include package of “glmnet,” “caret,”“rms,” “pROC,” “rmda,” and so on.
Division of datasets
A total of 2,214 DCM patients were randomly divided into two groups, the training group (n = 1,551) and the validation group (n = 663), at a theoretical ratio of 7 : 3.
Variable selection
The least absolute shrinkage and selection operator (LASSO) regression is an efficient statistical method to filter out the most important features from high-dimensional data. We performed LASSO regression in the training group to screen out the most useful predictor variables for unplanned ICU admissions. The nonzero coefficient characteristic variables corresponding to the maximum λ within 1 standard deviation (SD) of the mean error was the final model predictor variables.
Model development and validation
The risk prediction model was developed by multivariate logistic regression, where the dependent variable in the model was unplanned ICU admission, while the independent variables included predictors selected from the LASSO regression. To provide clinicians with a quantitative tool to predict the risk of unplanned ICU admissions, we constructed a nomogram based on multivariate logistic regression analysis. Nomogram performance was evaluated by both discriminations, presented as C-index and the area under the receiver operating characteristic curve (AUC), and calibration, expressed as the Hosmer–Lemeshow test and calibration plot. Discrimination and calibration were also accounted for in estimating the validity of the model in the validation group. To assess the clinical validity of the model, the decision curve analysis (DCA) and clinical impact curve (CIC) were constructed, which were mainly quantitative analyses of the net returns under different threshold probabilities.
Categorical variables were expressed as frequencies (%) and continuous variables were expressed as mean ± SD or median (interquartile range). The differences in baseline characteristics between the two groups were examined by independent-samples t-test or Mann–Whiney U-test for continuous variables and the Pearson chi-square test (Pearson χ2 test) or Fisher exact test for categorical variables, as appropriate. All tests were two-sided, and a P value <0.05 was considered statistically significant.
Result
Patient characteristics in the training group
A total of 1,551 (male, 73.1%) and 663 patients (male, 72.39%) with DCM comprised the training and validation groups, respectively. There were 147 (9.47%) and 62 (9.35%) patients who had unplanned ICU admission in the training and validation groups, respectively. As shown in Table 1, compared with the ICU− patients, ICU+ patients, have a higher ratio of emergency admission (EA), β blocker usage, New York Heart Association (NYHA) class, heart rate, white blood cell count, neutrophil count, aspartate aminotransferase, higher N-terminal pro b-type natriuretic peptide, larger right ventricular diameter (RV), and larger right atrial diameter (RA) (all P < 0.05, respectively); the lymphocyte count, monocyte count, fasting blood glucose, serum albumin, serum sodium, and serum potassium were significantly lower than those in the ICU− group (all P < 0.05, respectively).
Patients in the ICU+ group were more likely to have larger RA and RV (all P < 0.05), and the left atrial diameter (LA), LVEDD, left ventricular end-systolic diameter (LVESD), and LVEF had no significant difference between the two groups.
Variable selection
In order to select variables that could predict the primary outcome, we included prespecified variables and variables that were statistically different between two groups into the LASSO regression analysis. The prespecified variables were selected based on clinical experience and current literature reports, as well as consensus on DCM prognostic stratification (24, 25). They were age, LVEDD, LVESD, and LVEF. Finally, we selected six statistically significant variables including emergency admission, previous stroke, NYHA class, heart rate, neutrophil count, and N-terminal pro b-type natriuretic peptide (NT-proBNP)/100 (Figure 2).
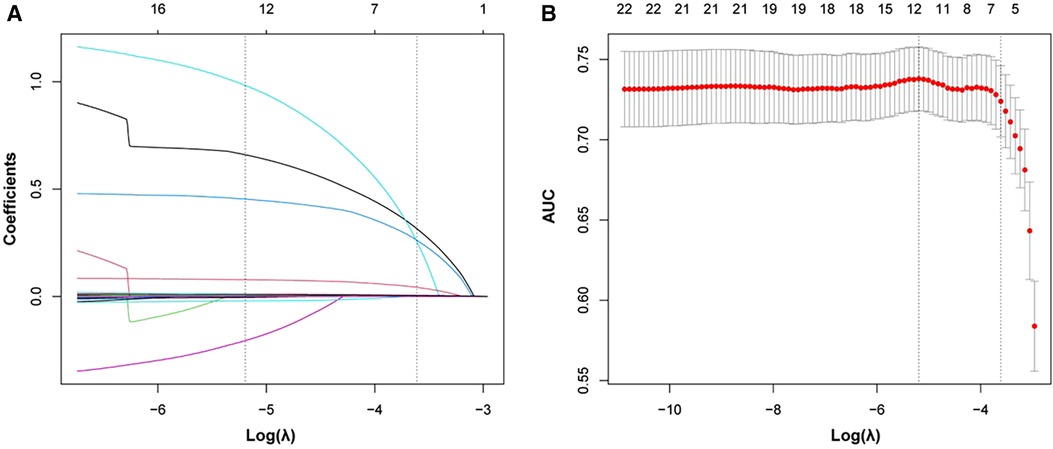
Figure 2. Significant variables selection using the LASSO. (A) Plot of each variable’s coefficient profile against log(lambda). (B) Ten-fold cross-validation used to validate the optimal lambda in the LASSO model. LASSO, least absolute shrinkage and selection operator.
Model development
In order to simplify the model and make it easier to use, based on optimal cutoff values, we converted heart rate (100 beats/min) and neutrophil count (4.385 × 109/L) into classified variables. Then, we used logistic regression analysis to analyze the incidence of unplanned ICU admission of DCM patients in the training group, finding that ED [odds ratio (OR): 2.13; 95% confidence interval (CI): 1.48–3.06, P < 0.001], previous stroke (OR: 3.12, 95% CI: 1.76–5.55, P < 0.001), NYHA class IV (OR: 1.81, 95% CI: 1.23–2.65, P = 0.002), heart rate (OR: 2.63, 95% CI: 1.54–3.33, P < 0.001), neutrophil count (OR: 1.90, 95% CI: 1.31–2.76, P = 0.001), and NT-proBNP/100 (OR: 1.01, 95% CI: 1.01–1.01, P < 0.001) were independent risk factors for unplanned ICU admission in DCM patients (P < 0.001) (Table 2).
Nomogram model display
Six independent risk variables were used to build a nomogram for predicting the risk of unplanned ICU admission in patients with DCM. The scores corresponding to each predictor variable in the nomogram were summed, and the resulting probability value corresponding to the total score is the probability of risk of unplanned ICU admission (Figure 3).
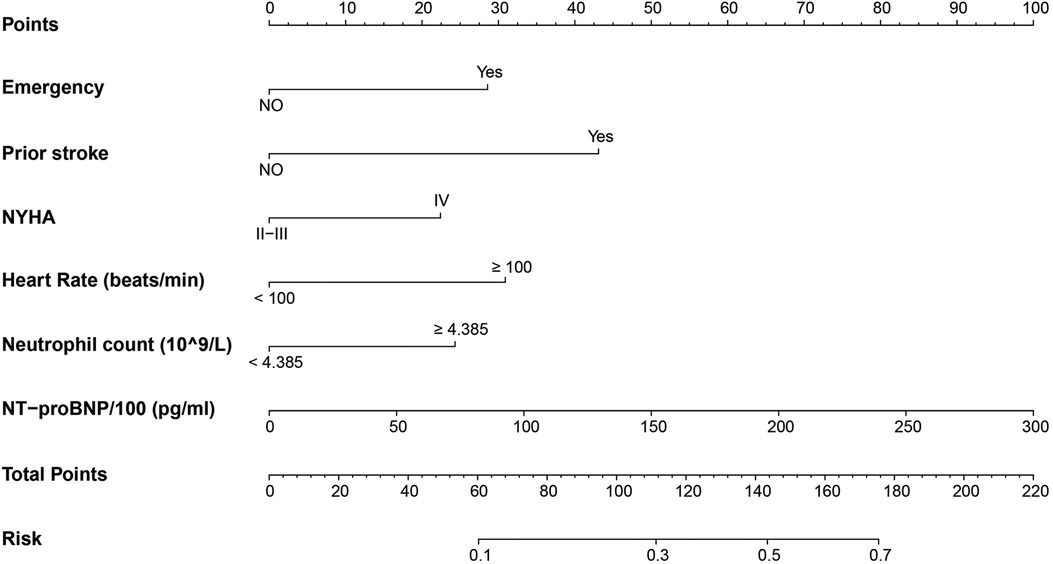
Figure 3. Nomogram to predict the risk of unplanned ICU admission in DCM inpatients. Points were assigned for each variable by drawing a line upward from the corresponding values to the “points line.” The “total points” was calculated as the sum of the individual score of each of the six variables included in the nomogram. We can estimate the risk of unplanned ICU admission for this patient by the probability corresponding to the “total points.” ICU, intensive care unit; DCM, dilated cardiomyopathy; NYHA, New York Heart Association; NT-proBNP, N-terminal pro b-type natriuretic peptide.
Nomogram evaluation and validation
The discriminatory ability of the nomogram was evaluated by calculating the C-statistic as 0.76 (95% CI: 0.72–0.80) in the training group. The corrected C-statistic from bootstrap resampling showed good internal validation with a value of 0.75. The model proved to be accurate in predicting unplanned ICU admissions of DCM patients with an AUC of 0.76 (95% CI: 0.72–0.80, Figure 4A). The Hosmer–Lemeshow test showed that the model has good calibration (χ2 = 14.40, P = 0.07), and the calibration curves similarly showed good calibration between the predicted and actual risk of unplanned ICU admissions for DCM patients (Figure 5A).
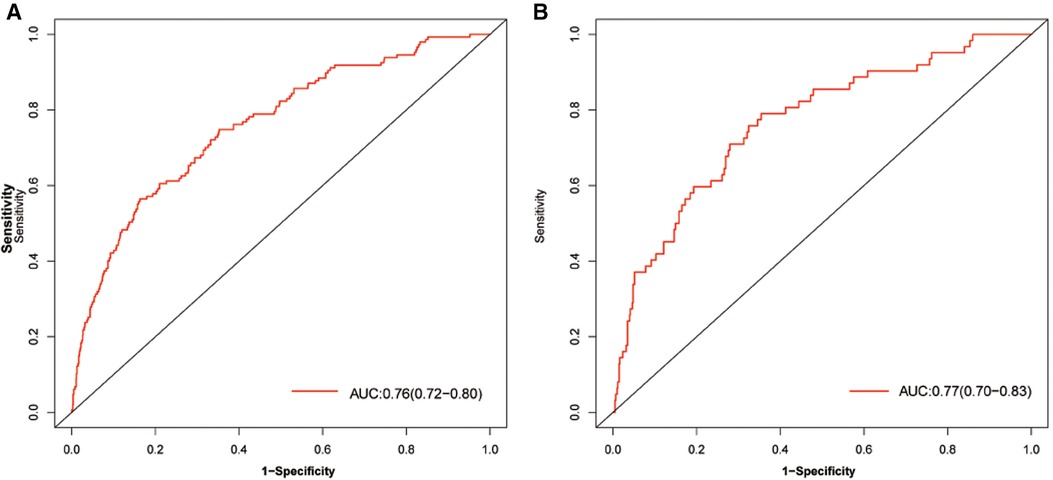
Figure 4. AUC of the model for predicting unplanned ICU admission of DCM patients. A, Development group. B, Validation group. Red curve shown the receiver operating characteristic curve (ROC) for nomogram. AUC, area under curve.
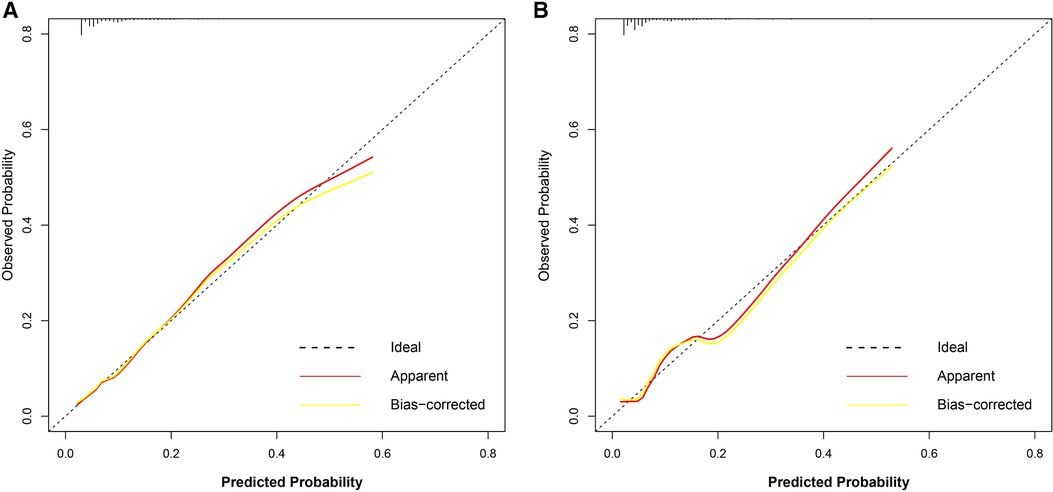
Figure 5. Calibration curve of the nomogram for the development group (A) and the validation group (B). The dotted line represents the ideal prediction, while the red line represents the actual calibration curve of the nomogram. The yellow line meanwhile represents the internal corrected calibration curve of the nomogram.
The C-index also reached 0.78 in the validation group and the AUC was 0.77 (95% CI: 0.70–0.83), as shown in Figure 4B. The calibration curves of the nomogram also suggested a good agreement between the actual and the predicted outcomes (Figure 5B).
To estimate the clinical utility of the nomogram, DCA and CIC were used. The results of DCA are presented in Figure 6, showing that the use of this model for making clinical decisions has more benefit than the “no intervention” or “all intervention” scenarios when the unplanned ICU admission threshold probability was between 0 and 0.75 in the training group and was generally between 0.0 and 1 in the validation group. Also, CIC analysis showed the clinical efficiency of the nomogram, when the threshold probability was greater than 65%; the prediction model determined that the population at high risk for unplanned ICU admission highly matched the population experiencing unplanned ICU admissions (Figure 7).
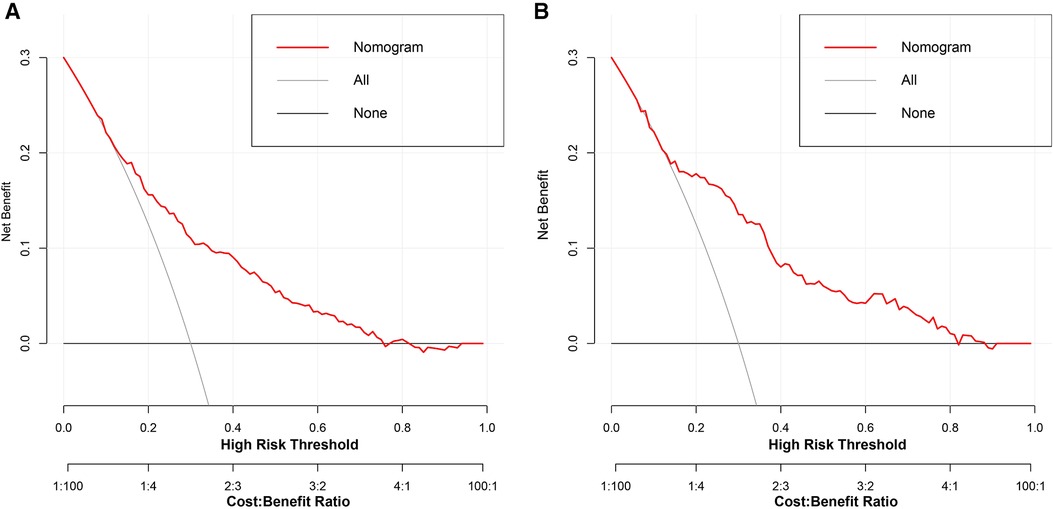
Figure 6. Decision curve analysis of the nomogram for the development group (A) and the validation group (B). The black line indicates that for extreme cases, the model predicts that all DCM patients have low-ICU+ probability and the clinical net benefit is 0. The gray curve indicates that for extreme cases, the model predicts that all DCM patients have moderate or high ICU+ probability and the clinical net benefit is the negative slope. The red line indicates that the model has clinical net benefit. The red line is higher than the gray and black lines, indicating that patients can benefit from the model. ICU, intensive care unit; DCM, dilated cardiomyopathy.
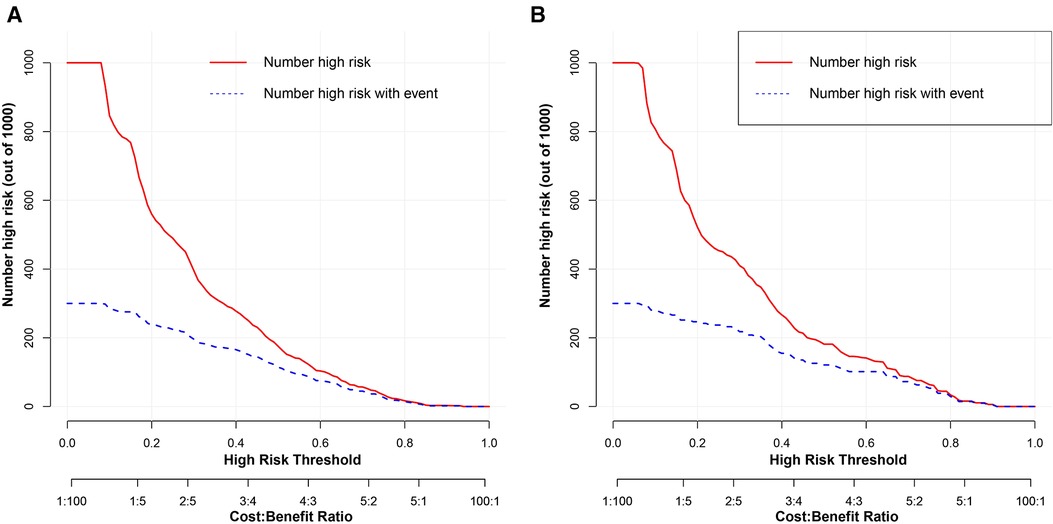
Figure 7. Clinical impact curve analysis of the nomogram for development group (A) and the validation group (B). The red curve indicates the number of people classified as positive (high risk) by the model at each threshold probability, and the blue curve is the number of true positives.
Performance of the GWTG-HF score of DCM inpatients
The data showed that the Get With the Guidelines-Heart Failure (GWTG-HF) score was an independent risk factor for unplanned ICU admission in DCM patients (OR: 1.04; 95% CI: 1.02–1.06; P < 0.001). In parallel, we evaluated the ability of the GWTG-HF score to assess the risk of unplanned transfer to the ICU for DCM inpatients. However, the diagnostic power of the GWTG-HF score was general, and the AUC of unplanned ICU admission was only 0.58 (95% CI: 0.53–0.63). The DeLong test suggested a statistically significant difference between the nomogram model and the GWTG-HF score in the ability to differentiate patients with high risk of unplanned ICU admission (P < 0.001, Figure 8).
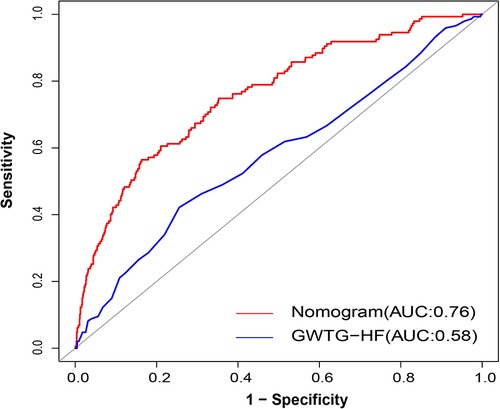
Figure 8. AUC of nomogram and GWTG-HF. Red and blue curves shown the ROC for nomogram and GWTG-HF. AUC, area under curve; GWTG-HF, Get With the Guidelines-Heart Failure; ROC, receiver operating characteristic curve.
Discussion
Early identification of DCM inpatients at high risk of unplanned ICU admission provides an important opportunity to assess deterioration and make timely changes in the treatment strategy. To overcome this practical need, for the first time we developed and validated a nomogram for DCM inpatients to predict the risk of unplanned ICU admissions. Emergency admission, previous stroke, NYHA class, heart rate, neutrophil count, and NT-proBNP were demonstrated to be predictors of elevated risk for unplanned ICU admissions.
Previous studies have reported that, in general internal inpatients, unplanned ICU admissions contribute to 14%–28% of ICU admissions (12, 26). From our data, the percentage of unplanned ICU admissions occurring is roughly 9.44% (209/2214) among DCM inpatients, and most of these inpatients were admitted through ED (47.85%, 100/209). In our study, the admission pathway was a valuable predictor of deterioration (OR: 2.13, 95% CI: 1.48–3.06, P < 0.001). Numerous studies have also confirmed that patients transferred from the general unit to ICU for intensive care management have higher in-hospital mortality than those admitted directly from ED (7, 9). Therefore, we considered that advance risk assessment and management of DCM patients with emergency admissions might be an effectively managed approach. Currently, the Medical Emergency Team (MET) system could be widely applied and extended to assess the risk of an emergency patient. Efficient use of the MET system can reduce the incidence of unplanned ICU admissions and is independently associated with reduced hospital mortality (27, 28).
Of note, some studies have been conducted on the risk assessment of unplanned ICU admissions. At present, the EWS has been used internationally and is widely promoted (29, 30). The NEWS, established by the Royal College of Physicians of London in 2012, is one of the scoring systems used to assess the severity of acute illnesses (13). The NEWS has proven to be a very effective tool for assessing the risk of in-hospital adverse events such as unplanned ICU admissions and in-hospital deaths. The predictors included in the NEWS were respiration rate, SpO2, any supplemental oxygen, temperature, SBP, heart rate, and level of consciousness, among which respiration rate, SpO2, and heart rate affect unplanned ICU admissions. Also, heart rate was the most important predictor in our nomogram model, emphasizing the importance of vital signs in assessing the risk of unplanned ICU admissions. Lindgren et al. showed the causal relationship between increased heart rate and myocardial systolic dysfunction, and fast heart rate in adolescents was strongly associated with the development of DCM-related heart failure (31). The TRED-HF study also found that increased heart rate might be a valid indicator of worsening cardiac function and relapse in patients recovering from DCM (32). Unfortunately, due to the missing arterial blood gas and temperature data, we were unable to assess the performance of the NEWS in DCM patients.
As we know, composite clinical endpoints, including unplanned ICU admission, cardiac arrest, and in-hospital mortality, were the observed outcomes for prior scores (32–35), and most of the study information was based on health records of general inpatients (33–35). Generalizing these scores to risk stratification of cardiovascular disease, therefore, may be somewhat limited. In view of the following, we formulated a new nomogram model to assess the potential risk of unplanned ICU admissions for DCM inpatients based on clinical information and laboratory characteristics. The C-index of our nomogram in the training group and the validation group were 0.76 and 0.78, respectively, indicating that the model had high discriminative power. Moreover, the calibration curve also suggested good agreement between the actual probabilities and the predicted probabilities in the training and validation groups. Our nomogram model still has a number of unique advantages. First, the population enrolled in this study differs from previous studies, which have mostly studied patients in the emergency department. In contrast, our study focused on inpatients with DCM. Second, we used LASSO regression to effectively avoid multivariate multicollinearity and overfitting in the variable selection procedure (36).
It also requires attention that exacerbation of heart failure is an important driver of deterioration in DCM patients. NYHA class and NT-proBNP on admission had previously been confirmed to be associated with an increased risk of major adverse cardiovascular events (MACE) in DCM patients (37, 38). They are widely used by several models of heart failure to stratify risk and predict prognosis. For this reason, NT-proBNP and NYHA class need to be considered, as we did in our study. In our nomogram model, NYHA class and NT-proBNP were independent predictors of unplanned ICU admissions.
In our study, we found that the level of neutrophils was high in DCM inpatients who were unplanned for ICU admission. Previous studies have shown that inflammation plays a very important role in the development of DCM (39), and neutrophils as an index of inflammation was closely associated with the severity of heart failure in DCM patients. Evidence of inflammatory infiltration has also been found in myocardial biopsy samples from DCM patients (40). Moreover, neutrophils activation may accelerate disease progression in DCM by promoting fibrosis in the myocardium (41). In this study, we found that elevated neutrophil count was an important marker of deterioration and unplanned ICU admission in DCM inpatients. Similarly, risk stratification in NEWS was improved by including the neutrophil count measured at hospital admission, and these improvements were replicated across several different studies (42). Redfern et al. recommended routinely collected blood tests combined with vital signs to assess unplanned ICU admissions (43). Therefore, we included neutrophils in the prediction model we constructed, which was a feature of our model.
The GWTG-HF risk score is a risk assessment tool commonly used to predict in-hospital mortality in patients with heart failure (44). In our patients, the GWTG-HF risk score is also useful as a tool for estimating unplanned ICU admissions in DCM patients (OR: 1.04; 95% CI: 1.02–1.06; P < 0.001). We compared the diagnostic efficacy of our nomogram and GWTG-HF risk score using ROC curve analysis. Ultimately, our model performs much better (Figure 8). Hence, it is reasonable to believe that our model has the potential to be a useful tool for evaluating unplanned ICU admissions for DCM inpatients.
Our study also has some limitations. First, this is a single-center and retrospective study, and some patients with incomplete data were also excluded, leading to selective bias. Therefore, multicenter and prospective studies are still necessary to improve the accuracy and applicability of the model. Second, we lacked data needed to externally validate our risk prediction model, so external validation of other clinical research centers is still needed to verify the predictive effect of our nomogram. Third, the population of our study was restricted to DCM inpatients, which also limited its application. A risk assessment study of unplanned ICU admissions for outpatients with DCM will be our next task. In addition, the course of DCM is a dynamic evolutionary process, and a single cross-sectional analysis cannot comprehensively assess the prognosis of DCM, which requires close observation and long-term follow-up, as well as timely adjustment of the treatment plan.
Conclusion
We developed and validated a new nomogram to predict the risk for unplanned ICU admission in DCM patients, based on six easily accessible independent risk variables. The nomogram may assist physicians in identifying individuals at high unplanned ICU admission risk for DCM inpatients.
Data availability statement
The raw data supporting the conclusions of this article will be made available by the authors, without undue reservation.
Ethics statement
The studies involving human participants were reviewed and approved by the Ethics Committees of the First Affiliated Hospital of Xinjiang Medical University. Written informed consent for participation was not required for this study in accordance with the national legislation and the institutional requirements.
Author contributions
Y-TM, AA (Adila Azhati), XL (Xiao-Lei Li), and DA: conception and design of the study, data collecting and analysis, and drafting of article. QZ, AA (Aibibanmu Aizezi), MK, and Y-PL: data collection and data analysis. FL, XM, and XL (Xiao-Mei Li): critical revision of article. All authors contributed to the article and approved the submitted version.
Funding
This study was supported by grants from the Central Guide on Local Science and Technology Development Fund of the Xinjiang Province (ZYYD2022A01) and the National Natural Science Foundation of China (91957208 and 81960046).
Acknowledgments
Thanks to Xian Wei for her contribution in the data collection and analysis.
Conflict of interest
The authors declare that the research was conducted in the absence of any commercial or financial relationships that could be construed as a potential conflict of interest.
Publisher's note
All claims expressed in this article are solely those of the authors and do not necessarily represent those of their affiliated organizations, or those of the publisher, the editors and the reviewers. Any product that may be evaluated in this article, or claim that may be made by its manufacturer, is not guaranteed or endorsed by the publisher.
References
1. Pinto YM, Elliott PM, Arbustini E, Adler Y, Anastasakis A, Bohm M, et al. Proposal for a revised definition of dilated cardiomyopathy, hypokinetic non-dilated cardiomyopathy, and its implications for clinical practice: a position statement of the ESC working group on myocardial and pericardial diseases. Eur Heart J. (2016) 37:1850–8. doi: 10.1093/eurheartj/ehv727
2. Seferovic PM, Polovina M, Bauersachs J, Arad M, Ben Gal T, Lund LH, et al. Heart failure in cardiomyopathies: a position paper from the Heart Failure Association of the European Society of Cardiology. Eur J Heart Fail. (2019) 21:553–76. doi: 10.1002/ejhf.1461
3. Kitaoka H, Tsutsui H, Kubo T, Ide T, Chikamori T, Fukuda K, et al. JCS/JHFS 2018 guideline on the diagnosis and treatment of cardiomyopathies. Circ J. (2021) 85:1590–689. doi: 10.1253/circj.CJ-20-0910
4. Mozaffarian D, Benjamin EJ, Go AS, Arnett DK, Blaha MJ, Cushman M, et al. Heart disease and stroke statistics—2016 update: a report from the American Heart Association. Circulation. (2016) 133:e38–360. doi: 10.1161/CIR.0000000000000350
5. Halliday BP, Gulati A, Ali A, Newsome S, Lota A, Tayal U, et al. Sex- and age-based differences in the natural history and outcome of dilated cardiomyopathy. Eur J Heart Fail. (2018) 20:1392–400. doi: 10.1002/ejhf.1216
6. Chioncel O, Ambrosy AP, Filipescu D, Bubenek S, Vinereanu D, Petris A, et al. Patterns of intensive care unit admissions in patients hospitalized for heart failure: insights from the RO-AHFS registry. J Cardiovasc Med (Hagerstown). (2015) 16:331–40. doi: 10.2459/JCM.0000000000000030
7. Frost SA, Alexandrou E, Bogdanovski T, Salamonson Y, Parr MJ, Hillman KM. Unplanned admission to intensive care after emergency hospitalisation: risk factors and development of a nomogram for individualising risk. Resuscitation. (2009) 80:224–30. doi: 10.1016/j.resuscitation.2008.10.030
8. Jennerich AL, Hobler MR, Sharma RK, Engelberg RA, Curtis JR. Unplanned admission to the ICU: a qualitative study examining family member experiences. Chest. (2020) 158:1482–9. doi: 10.1016/j.chest.2020.05.554
9. Reese J, Deakyne SJ, Blanchard A, Bajaj L. Rate of preventable early unplanned intensive care unit transfer for direct admissions and emergency department admissions. Hosp Pediatr. (2015) 5:27–34. doi: 10.1542/hpeds.2013-0102
10. Essay P, Balkan B, Subbian V. Decompensation in critical care: early prediction of acute heart failure onset. JMIR Med Inform. (2020) 8:e19892. doi: 10.2196/19892
11. Grieve R, O'Neill S, Basu A, Keele L, Rowan KM, Harris S. Analysis of benefit of intensive care unit transfer for deteriorating ward patients: a patient-centered approach to clinical evaluation. JAMA Netw Open. (2019) 2:e187704. doi: 10.1001/jamanetworkopen.2018.7704
12. Fu LH, Schwartz J, Moy A, Knaplund C, Kang MJ, Schnock KO, et al. Development and validation of early warning score system: a systematic literature review. J Biomed Inform. (2020) 105:103410. doi: 10.1016/j.jbi.2020.103410
13. Smith GB, Prytherch DR, Meredith P, Schmidt PE, Featherstone PI. The ability of the national early warning score (NEWS) to discriminate patients at risk of early cardiac arrest, unanticipated intensive care unit admission, and death. Resuscitation. (2013) 84:465–70. doi: 10.1016/j.resuscitation.2012.12.016
14. Blackwell JN, Keim-Malpass J, Clark MT, Kowalski RL, Najjar SN, Bourque JM, et al. Early detection of in-patient deterioration: one prediction model does not fit all. Crit Care Explor. (2020) 2:e0116. doi: 10.1097/CCE.0000000000000116
15. Chinese Society of Cardiology, China Collaborative Group on myocarditis and cardiomyopathy. Chinese guidelines for the diagnosis and treatment of dilated cardiomyopathy. J Clin Cardiol. (2018) 34:421–34. doi: 10.13201/j.issn.1001-1439.2018.05.001
16. Williams B, Mancia G, Spiering W, Agabiti Rosei E, Azizi M, Burnier M, et al. 2018 ESC/ESH guidelines for the management of arterial hypertension: the task force for the management of arterial hypertension of the European Society of Cardiology and the European Society of Hypertension. J Hypertens. (2018) 36:1953–2041. doi: 10.1097/HJH.0000000000001940
17. Cosentino F, Grant PJ, Aboyans V, Bailey CJ, Ceriello A, Delgado V, et al. 2019 ESC guidelines on diabetes, pre-diabetes, and cardiovascular diseases developed in collaboration with the EASD. Eur Heart J. (2020) 41:255–323. doi: 10.1093/eurheartj/ehz486
18. Sacco RL, Kasner SE, Broderick JP, Caplan LR, Connors JJ, Culebras A, et al. An updated definition of stroke for the 21st century: a statement for healthcare professionals from the American Heart Association/American Stroke Association. Stroke. (2013) 44:2064–89. doi: 10.1161/STR.0b013e318296aeca
19. Hindricks G, Potpara T, Dagres N, Arbelo E, Bax JJ, Blomstrom-Lundqvist C, et al. 2020 ESC guidelines for the diagnosis and management of atrial fibrillation developed in collaboration with the European Association for Cardio-Thoracic Surgery (EACTS): the task force for the diagnosis and management of atrial fibrillation of the European Society of Cardiology (ESC) developed with the special contribution of the European Heart Rhythm Association (EHRA) of the ESC. Eur Heart J. (2021) 42:373–498. doi: 10.1093/eurheartj/ehaa612
20. Mirza S, Clay RD, Koslow MA, Scanlon PD. COPD guidelines: a review of the 2018 GOLD report. Mayo Clin Proc. (2018) 93:1488–502. doi: 10.1016/j.mayocp.2018.05.026
21. Levey AS, Eckardt KU, Dorman NM, Christiansen SL, Hoorn EJ, Ingelfinger JR, et al. Nomenclature for kidney function and disease: report of a kidney disease: improving global outcomes (KDIGO) consensus conference. Kidney Int. (2020) 97:1117–29. doi: 10.1016/j.kint.2020.02.010
22. National Institutes of Health/National Cancer Institute Common Terminology Criteria for Adverse Events (CTCAE) Version 4.03. 2009. [Accessed June 27, 2014]. Available from: http://www.eortc.be/services/doc/ctc/.
23. Shiloh AL, Ari Eisen L, Savel RH. The unplanned intensive care unit admission. J Crit Care. (2015) 30:419–20. doi: 10.1016/j.jcrc.2014.12.010
24. Faggiano A, Avallone C, Gentile D, Provenzale G, Toriello F, Merlo M, et al. Echocardiographic advances in dilated cardiomyopathy. J Clin Med. (2021) 10(23):5518. doi: 10.3390/jcm10235518
25. Marrow BA, Cook SA, Prasad SK, McCann GP. Emerging techniques for risk stratification in nonischemic dilated cardiomyopathy: JACC review topic of the week. J Am Coll Cardiol. (2020) 75:1196–207. doi: 10.1016/j.jacc.2019.12.058
26. Bapoje SR, Gaudiani JL, Narayanan V, Albert RK. Unplanned transfers to a medical intensive care unit: causes and relationship to preventable errors in care. J Hosp Med. (2011) 6:68–72. doi: 10.1002/jhm.812
27. Satyavolu R, Ruknuddeen MI, Soar N, Edwards SM. Dosage and clinical outcomes of medical emergency team and conventional referral mediated unplanned intensive care admissions. J Intensive Care Soc. (2022) 0:1–8. doi: 10.1177/17511437211060157
28. Lee J, Shin Y, Choi E, Choi S, Son J, Jung YK, et al. Impact of hospitalization duration before medical emergency team activation: a retrospective cohort study. PLoS One. (2021) 16:e0247066. doi: 10.1371/journal.pone.0247066
29. Liu VX, Lu Y, Carey KA, Gilbert ER, Afshar M, Akel M, et al. Comparison of early warning scoring systems for hospitalized patients with and without infection at risk for in-hospital mortality and transfer to the intensive care unit. JAMA Netw Open. (2020) 3:e205191. doi: 10.1001/jamanetworkopen.2020.5191
30. Kim SH, Choi HS, Jin ES, Choi H, Lee H, Lee SH, et al. Predicting severe outcomes using national early warning score (NEWS) in patients identified by a rapid response system: a retrospective cohort study. Sci Rep. (2021) 11:18021. doi: 10.1038/s41598-021-97121-w
31. Lindgren M, Robertson J, Adiels M, Schaufelberger M, Aberg M, Toren K, et al. Elevated resting heart rate in adolescent men and risk of heart failure and cardiomyopathy. ESC Heart Fail. (2020) 7:1178–85. doi: 10.1002/ehf2.12726
32. Halliday B, Vazir A, Owen R, Gregson J, Wassall R, Lota AS, et al. Heart rate as a marker of relapse during withdrawal of heart failure therapy in patients with recovered dilated cardiomyopathy: an analysis from TRED-HF. Eur Heart J. (2020) 41:1057. doi: 10.1093/eurheartj/ehaa234
33. Watkinson PJ, Pimentel MAF, Clifton DA, Tarassenko L. Manual centile-based early warning scores derived from statistical distributions of observational vital-sign data. Resuscitation. (2018) 129:55–60. doi: 10.1016/j.resuscitation.2018.06.003
34. Dziadzko MA, Novotny PJ, Sloan J, Gajic O, Herasevich V, Mirhaji P, et al. Multicenter derivation and validation of an early warning score for acute respiratory failure or death in the hospital. Crit Care. (2018) 22:286. doi: 10.1186/s13054-018-2194-7
35. Churpek MM, Adhikari R, Edelson DP. The value of vital sign trends for detecting clinical deterioration on the wards. Resuscitation. (2016) 102:1–5. doi: 10.1016/j.resuscitation.2016.02.005
36. Chen S, Chen L, Saguner AM, Chen K, Akdis D, Gasperetti A, et al. Novel risk prediction model to determine adverse heart failure outcomes in arrhythmogenic right ventricular cardiomyopathy. J Am Heart Assoc. (2022) 11:e024634. doi: 10.1161/JAHA.121.024634
37. McDonagh TA, Metra M, Adamo M, Gardner RS, Baumbach A, Bohm M, et al. 2021 ESC guidelines for the diagnosis and treatment of acute and chronic heart failure. Eur Heart J. (2021) 42:3599–726. doi: 10.1093/eurheartj/ehab368
38. Heidenreich PA, Bozkurt B, Aguilar D, Allen LA, Byun JJ, Colvin MM, et al. 2022 AHA/ACC/HFSA guideline for the management of heart failure: a report of the American College of Cardiology/American Heart Association Joint Committee on Clinical Practice Guidelines. J Am Coll Cardiol. (2022) 79:e263–421. doi: 10.1016/j.jacc.2021.12.012
39. Schultheiss HP, Fairweather D, Caforio ALP, Escher F, Hershberger RE, Lipshultz SE, et al. Dilated cardiomyopathy. Nat Rev Dis Primers. (2019) 5:32. doi: 10.1038/s41572-019-0084-1
40. Noutsias M, Rohde M, Goldner K, Block A, Blunert K, Hemaidan L, et al. Expression of functional T-cell markers and T-cell receptor Vbeta repertoire in endomyocardial biopsies from patients presenting with acute myocarditis and dilated cardiomyopathy. Eur J Heart Fail. (2011) 13:611–8. doi: 10.1093/eurjhf/hfr014
41. Baci D, Bosi A, Parisi L, Buono G, Mortara L, Ambrosio G, et al. Innate immunity effector cells as inflammatory drivers of cardiac fibrosis. Int J Mol Sci. (2020) 21:7165. doi: 10.3390/ijms21197165
42. Carr E, Bendayan R, Bean D, Stammers M, Wang W, Zhang H, et al. Evaluation and improvement of the national early warning score (NEWS2) for COVID-19: a multi-hospital study. BMC Med. (2021) 19:23. doi: 10.1186/s12916-020-01893-3
43. Redfern OC, Pimentel MAF, Prytherch D, Meredith P, Clifton DA, Tarassenko L, et al. Predicting in-hospital mortality and unanticipated admissions to the intensive care unit using routinely collected blood tests and vital signs: development and validation of a multivariable model. Resuscitation. (2018) 133:75–81. doi: 10.1016/j.resuscitation.2018.09.021
44. Peterson PN, Rumsfeld JS, Liang L, Albert NM, Hernandez AF, Peterson ED, et al. A validated risk score for in-hospital mortality in patients with heart failure from the American Heart Association get with the guidelines program. Circ Cardiovasc Qual Outcomes. (2010) 3:25–32. doi: 10.1161/CIRCOUTCOMES.109.854877
Keywords: dilated cardiomyopathy, unplanned ICU admission, prognosis, nomogram, prediction model
Citation: Li X, Adi D, Zhao Q, Aizezi A, Keremu M, Li Y, Liu F, Ma X, Li X, Azhati A and Ma Y (2023) Development and validation of nomogram for unplanned ICU admission in patients with dilated cardiomyopathy. Front. Cardiovasc. Med. 10:1043274. doi: 10.3389/fcvm.2023.1043274
Received: 13 September 2022; Accepted: 22 February 2023;
Published: 16 March 2023.
Edited by:
Tomasz Zieliński, National Institute of Cardiology, PolandReviewed by:
Bruno Pinamonti, University Health Organization Giuliano Isontina (ASU GI), ItalyJie Weng, Second Affiliated Hospital and Yuying Children's Hospital of Wenzhou Medical University, China
© 2023 Li, Adi, Zhao, Aizezi, Keremu, Li, Liu, Ma, Li, Azhati and Ma. This is an open-access article distributed under the terms of the Creative Commons Attribution License (CC BY). The use, distribution or reproduction in other forums is permitted, provided the original author(s) and the copyright owner(s) are credited and that the original publication in this journal is cited, in accordance with accepted academic practice. No use, distribution or reproduction is permitted which does not comply with these terms.
*Correspondence: Yi-Tong Ma bXl0LXhqQDE2My5jb20= Adila Azhati YWRsbmRsQDE2My5jb20=
†These authors have contributed equally to this work
Specialty Section: This article was submitted to Heart Failure and Transplantation, a section of the journal Frontiers in Cardiovascular Medicine