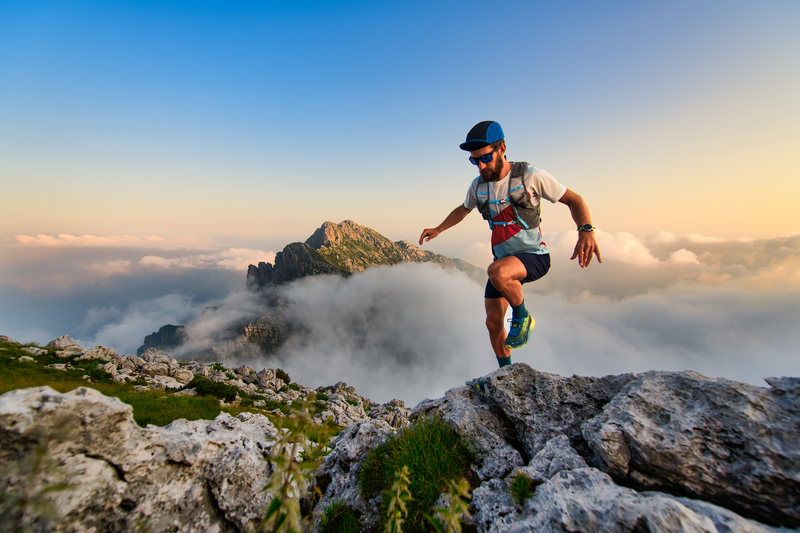
94% of researchers rate our articles as excellent or good
Learn more about the work of our research integrity team to safeguard the quality of each article we publish.
Find out more
ORIGINAL RESEARCH article
Front. Cardiovasc. Med. , 04 October 2022
Sec. Cardiovascular Metabolism
Volume 9 - 2022 | https://doi.org/10.3389/fcvm.2022.976844
This article is part of the Research Topic Novel Biomarkers and Risk Factors Associated with Cardiometabolic Dysfunction in Heart Failure View all 16 articles
Background: The risk factors for acute heart failure (AHF) vary, reducing the accuracy and convenience of AHF prediction. The most common causes of AHF are coronary heart disease (CHD). A short-term clinical predictive model is needed to predict the outcome of AHF, which can help guide early therapeutic intervention. This study aimed to develop a clinical predictive model for 1-year prognosis in CHD patients combined with AHF.
Materials and methods: A retrospective analysis was performed on data of 692 patients CHD combined with AHF admitted between January 2020 and December 2020 at a single center. After systemic treatment, patients were discharged and followed up for 1-year for major adverse cardiovascular events (MACE). The clinical characteristics of all patients were collected. Patients were randomly divided into the training (n = 484) and validation cohort (n = 208). Step-wise regression using the Akaike information criterion was performed to select predictors associated with 1-year MACE prognosis. A clinical predictive model was constructed based on the selected predictors. The predictive performance and discriminative ability of the predictive model were determined using the area under the curve, calibration curve, and clinical usefulness.
Results: On step-wise regression analysis of the training cohort, predictors for MACE of CHD patients combined with AHF were diabetes, NYHA ≥ 3, HF history, Hcy, Lp-PLA2, and NT-proBNP, which were incorporated into the predictive model. The AUC of the predictive model was 0.847 [95% confidence interval (CI): 0.811–0.882] in the training cohort and 0.839 (95% CI: 0.780–0.893) in the validation cohort. The calibration curve indicated good agreement between prediction by nomogram and actual observation. Decision curve analysis showed that the nomogram was clinically useful.
Conclusion: The proposed clinical prediction model we have established is effective, which can accurately predict the occurrence of early MACE in CHD patients combined with AHF.
Acute heart failure (AHF) is a life-threatening condition characterized by acute dyspnea and Systemic congestion caused by abnormal cardiac structure or function, including acute decompensated heart failure (ADHF) or new onset of AHF (1). AHF is the leading cause of cardiogenic shock and cardiac arrest in patients, and the case fatality rate in hospitals is as high as 3–13%, seriously threatening their life safety, and their prognosis is extremely poor (2). The most common causes of AHF are coronary ischemic disease, cardiomyopathy, valvular disease, infective endocarditis, hypertensive heart disease, pulmonary heart disease, renal failure, and metabolic disorders. A significant proportion of coronary heart disease (CHD) patients develop AHF, and CHD is the primary cause of heart failure(HF). Despite recent advances in AHF management, such as advances in pharmacological treatment, cardiac devices, and specific heart failure programs, mortality remains high. The in-hospital fatality rate and 5-year mortality rate of AHF were 3 and 60%, respectively, and the rate of 1-year emergency department visits and emergency re-hospitalization was 50% (3). Uncontrolled AHF complicates clinical treatment and threatens patient safety (4). Thus, an effective and simple prediction model for AHF is urgently needed.
Acute heart failure is associated with a higher risk of death and re-hospitalization, and many researchers have attempted to develop different tools to predict adverse events in patients with AHF. Several researchers have developed risk score models to stratify HF patients, such as the Seattle Heart Failure Model (SHFM), the European Society of Cardiology (ESC) Model, and the American College of Cardiology/American Heart Association (ACC/AHA) Model (5–7). Although the predictive value of these models is widely recognized, some researchers report that these models do not necessarily predict the mortality of individual patients with HF (8). In addition to the high mortality rate, the high rate of re-hospitalization was due to major adverse cardiac events (MACE), which contributed to the poor prognostic outcomes of AHF (9, 10). According to the report (11), each AHF hospitalization resulted in cardiac dysfunction and a gradual decline in the patient’s clinical course, increasing the risk of re-hospitalization. Notably, its risk could be markedly increased in the short-term period after an AHF event (12–15). A significant proportion of AHF patients appear to be at an even higher risk of rapid HF progression and death following an acute event. Early, post-discharge follow-up and risk-tailored, intensive HF therapy may reduce AHF re-hospitalization and improve survival in these patients (16). At present, AHF prediction models are mostly used to predict mortality or long-term prognosis, while models for AHF after short-term are very rare. Evidence in the short-term prognosis prediction model of AHF demonstrates that early, coordinated, aggressive treatment reduces inpatient mortality (17). Recent several studies have shown that a number of risk factors are related to short-term mortality after discharge, including age, sex, ventricular function, management, and so on (18–21). Although some established algorithms are currently available, these studies have not been validated in Chinese populations. Therefore, an individualized prediction model is imperative for more accurate MACE prediction in AHF patients.
Nomogram is a new prognosis evaluation tool based on Cox proportional hazards regression model or logistic regression model that predicts individual disease risk graphically and is easily applied clinically. It mainly simplifies the prediction model by calculating a single estimated value of the probability of an event occurrence and provides a personalized prognosis assessment for individual patients to assist clinical decision-making (22). Compared with the traditional risk scoring system, a nomogram can integrate more risk factors, calculate the numerical probability of the target event, quantify the risk more accurately, and apply it more flexibly.
This study aimed to develop a clinical predictive model to predict the risk of short-term (1-year) adverse outcomes in CHD patients combined with AHF based on potential risk factors.
A total of 692 CHD patients combined with AHF admitted to Shunde Hospital of Southern Medical University between January 2020 and December 2020 were selected for this study. Inclusion criteria: (1)Patients with CHD who were diagnosed with coronary artery stenosis > 70%; (2) Patients with AHF met the Chinese Guidelines for the Diagnosis and Treatment of Heart Failure 2018 (23); (3) Patients aged ≥ 18 years; and (4) Patients were classified as having grade II–IV cardiac function, by the New York Heart Association (NYHA) classification (24). Exclusion criteria: (1) Patients with congenital heart disease, cardiomyopathy, or valvular disease; (2) Patients complicated with malignant tumors; (3) Patients with hematological system diseases or autoimmune diseases; (4) Patients with prior history of cerebrovascular accident or mental illness; and (5) Patients with clinical data that were incomplete or lost to follow-up.
Based on previous studies (25, 26), we selected 40 risk factors that may predict MACE in CHD patients combined with AHF 1 year after discharge from the hospital, including age, sex, hypertension, diabetes, smoking, HF history, chronic kidney disease history, atrial fibrillation history, NYHA grades, systolic blood pressure, diastolic blood pressure, lipoprotein-associated phospholipase A2 (Lp-PLA2), homocysteine (Hcy), serum creatine kinase isoenzyme MB (CK-MB), troponin T, N-terminal pro B-type natriuretic peptide (NT-proBNP), D-dimer, total cholesterol, low-density lipoprotein cholesterol, high-density lipoprotein cholesterol, Triglycerides, fibrinogen degradation product, serum creatinine, uric acid, fasting plasma glucose, C-reactive protein, white blood cell count, neutrophil count, lymphocyte count, neutrophil to lymphocyte ratio, hemoglobin levels, platelet count, and medication status (such as diuretics, beta-blockers, Statins, Mineralocorticoid receptor antagonists, Angiotensin receptor enkephalinase inhibitors, calcium channel blockers, angiotensin-converting enzyme inhibitors, and angiotensin receptor blockers).
Follow-up data were collected from our hospital’s electronic medical record system, patients’ outpatient records, and telephone conversations with patients or family members.
The 1-year adverse outcomes were defined as adverse cardiovascular events associated with stroke, Non-fatal myocardial infarction, cardiac death and re-hospitalization due to HF during the first 12 months of follow-up after discharge from the hospital.
Patients were divided into MACE and no MACE groups based on whether MACE occurred 1-year after discharge from the hospital.
All statistical calculations were computed using R software. The data were randomly divided into a training cohort (n = 484) and a validation cohort (n = 208) at about 7:3. Predictors of 1-year outcomes were analyzed using logistic regression based on the training cohort. Step-wise regression based on the Akaike information criterion was used to further select significant variables. The receiver operating characteristic curve (ROC) and its area under the curve (AUC) were used to evaluate the step-wise regression on both the training and validation cohorts. A nomogram was formulated based on the results of logistic regression. The nomogram is based on proportionally transforming the regression coefficient into a 0–100 point scale. The sum of all variables’ points could be interpreted as a probability of belonging to a class. The predictive performance of the nomogram was measured using AUC and resampling model calibration, accompanied by the Hosmer-Lemeshow test (a significant test statistic implies that the model does not calibrate perfectly). Decision curve analysis (DCA) was used to determine the clinical usefulness of the models by calculating the net benefits at different threshold probabilities in the combined training and validation datasets. The “rms” package was used for nomogram formulation and calibration. The DCA was performed by using the “rmda” package. P-value < 0.05 was considered to be statistically significant.
The mean age of the 692 patients was 67.13 ± 15.55 years, with 400 (57.8%) males and 292 (42.2%) females. Three hundred seven-nine (54.8%) patients were diagnosed with ADHF, and 313 (45.2%) patients were diagnosed with new onset of AHF. Two hundred fifty-nine (37.4%) patients were followed up with MACE, including 23 (3.3%) patients with cardiac death and 236 (34.1%) patients with re-hospitalization cause by stroke, Non-fatal myocardial infarction, or HF. Finally, 484 patients were assigned to the training cohort, which included 181 (37.4%) patients with MACE, and 208 patients were assigned to the validation cohort, which included 78 (37.5%) patients with MACE.
Clinical data from the training and validation cohorts revealed no statistically significant differences in age, sex, disease history, laboratory tests, NYHA grades, medication status, or MACE incidence (P > 0.05) (Table 1).
Table 2 compares patients and clinical characteristics in the training cohort between the MACE and No MACE groups.
Six predictors, including diabetes, HF history, NYHA ≥ 3, NT-proBNP, Lp-PLA2, and Hcy, were selected by step-wise regression based on the Akaike information criterion (Table 3). The above independent predictors were incorporated into the nomogram (Figure 1). The nomogram for 1-year MACE prognosis prediction indicated an AUC of 0.847 [95% confidence interval (CI): 0.811–0.882], a sensitivity of 0.740, a specificity of 0.815, and an accuracy of 0.787 in the training cohort (Figure 2A and Table 4). Relatively, in the validation cohort, the nomogram showed an AUC of 0.839 (95% CI: 0.780–0.893), a sensitivity of 0.705, a specificity of 0.877, and an accuracy of 0.812 (Figure 2B and Table 4). Figures 3A,B depicts the nomogram calibration curve, demonstrating good agreement between prediction by nomogram and actual observation in the training and validation cohorts. The Hosmer-Lemeshow test produced a non-significant statistic (χ2 = 6.522, P = 0.589 in the training cohort and χ2 = 5.648, P = 0.687 in the validation cohort, respectively), indicating no perfect fit deviation.
Figure 1. Nomogram for 1-year MACE prognosis in coronary heart disease (CHD) patients combined with AHF. The nomogram was developed in the primary cohort, with the Diabetes, HF history, NYHA ≥ 3, NT-proBNP, Lp-PLA2, and Hcy incorporated.
Figure 2. The ROC of nomogram in panels (A,B) training and validation cohorts. ROC, receiver operating characteristic curve.
Figure 3. The calibration curve and decision curve of the nomogram in training and validation cohorts. The calibration curves of the nomogram in the training cohort (A) and validation cohort (B) are reported. The x-axis is the nomogram predicted probability and the y-axis is the actual probability. The prediction performance can be measured by the difference of the fitted curve and slope 1 line (diagonal 45-degree line). The diagonal dotted line represents a perfect prediction by an ideal model. The solid line represents the performance of the nomogram, of which a closer fit to the diagonal dotted line represents a better prediction. Decision curve analysis for the nomogram in the training cohort (C) and validation cohort (D) are showed. The y-axis measures the net benefit. The red line represents the nomogram. The blue line represents all patients had MACE. The orange line represents the assumption that no patients had MACE. The decision curve showed that if the threshold probability of a patient uses the nomogram offered a net benefit over the “happen-all” or “happen-none” strategy at a threshold range form 8–88 to 8–92%, respectively in the training cohort and validation cohort.
In the training and validation cohort DCAs, the nomogram offered a net benefit over the “happen-all” or “happen-none” strategies at a threshold of 8–88 and 8–92%, respectively (Figures 3C,D), demonstrating that our nomogram was clinically useful. For example, in a training cohort with a threshold probability of 40%, using the clinical nomogram could provide an additional net benefit of 0.2 over the “happen-all” or “happen-none” strategy.
We included six independent factors associated with 1–year prognosis in CHD patients combined with AHF, including diabetes, HF history, NYHA ≥ 3, NT-proBNP, Lp-PLA2, and Hcy. The developed clinical nomogram model achieved good predictive performance in the training cohort (AUC = 0.847, 95% CI: 0.811–0.882) and validation cohort (AUC = 0.839, 95% CI: 0.780–0.893). The clinical predictive model has been clinically validated.
HF is one of the most common acute and severe diseases in internal medicine, as well as the final stage of the progression of various cardiovascular disorders. It has been reported that a significant number of patients with AHF are re-hospitalized after discharge due to recurrent symptoms or die within months of being discharged from the hospital (27). Our study showed that during 1-year post-discharge follow-up, 259 patients (37.4%) were followed up with MACE, including 23 (3.3%) patients with cardiac death and 236 (34.1%) patients with re-hospitalization cause by stroke, Non-fatal myocardial infarction, or HF. This result is consistent with the findings of the European Heart Organization and the American Heart Association (28–30). They concluded that AHF represented high-risk patients with higher mortality and likelihood of re-hospitalization during the same follow-up period than chronic stable HF. The treatment goal of HF is not only to improve symptoms and quality of life but also to prevent and delay the development of cardiac remodeling by targeting the mechanism of cardiac remodeling to reduce the mortality and hospitalization rate of patients with HF (31). Delayed diagnosis of AHF worsens prognosis by increasing the time to initiate initial treatment, and this delay may be associated with increased morbidity and mortality (32). That is why the individualized prediction of AHF is critical.
According to the 2018 Guidelines for the Diagnosis and Treatment of Heart Failure in China, the associated factors for poor prognosis of patients with HF are decreased left ventricular ejection fraction, continuously increased natriuretic peptide levels, deterioration of NYHA cardiac function grading, hyponatremia, decreased hematocrit value, chronic hypotension, resting tachycardia, and renal insufficiency (33). However, studies on identifying short-term (1-year) prognosis predictors of CHD patients combined with AHF are scarce. Inspired by previous studies, our nomogram model is based on the available clinical high performance in predicting the 1-year prognosis of CHD patients combined with AHF, including diabetes, HF history, NYHA ≥ 3, NT-proBNP, Lp-PLA2, and Hcy. Diabetes can increase the mortality rate in patients with HF by 50–100% (34, 35). Mebazaa et al. have shown that hyperglycemia has a poor short-term prognosis for AHF and can exacerbate its progression. Lassus et al. suggested that both all-cause mortality and cardiovascular mortality are lower in patients with new-onset AHF than in patients with acutely decompensated chronic heart failure (ADCHF) (36, 37). Studies have shown that patients with ADCHF have a longer course of CHD and more significant cardiac remodeling. ADCHF will further aggravate the damage to myocardial cells and extracellular matrix damage, resulting in aggravated cardiac remodeling and deterioration of cardiac function (36, 38). NT-proBNP is a bioactive lysis product of brain natriuretic peptide (BNP), produced and released by ventricular myocytes under ventricular wall stress (39). Causes of BNP release during AHF include myocardial cell extension, tissue ischemia, and myocardial remodeling (40, 41). Many experiments confirmed that NT-probNP is a predictor of the prognosis of HF. And our studies showed that NT-probNP is an independent risk factor for short-term prognosis. Moreover, the higher the NT-proBNP index of AHF patients, the more severe the symptoms of HF, and the worse the short-term and long-term prognosis (42). Belkin et al. suggested that NYHA ≥ III is a risk factor for AHF (43). BNP levels and NT-proBNP concentrations of AHF patients increased gradually as their NYHA cardiac classification increased, and the differences between the III/IV and II groups were statistically significant. Sheng et al. have suggested that LP-PLA2 is an independent predictor of vascular endothelial injury, which is associated with the occurrence and prognosis of both ischemic and non-ischemic heart failure (44). High levels of Hcy are independent risk factors for HF induced by CHD (45). Studies showed that the 5- or 3-year mortality rate of HF patients with high levels of Hcy is significantly higher than that of patients with normal levels of Hcy, and Hcy levels may be an independent predictor of the long-term prognosis of patients with HF (46, 47). Our study suggests that LP-PLA2 and Hcy were independent factors for 1-year prognosis of AHF. However, the effect of Hcy and LP-PLA2 levels on the short-term prognosis of HF has not been widely reported. Thus, larger sample size studies are required to confirm the findings of this study.
As an intuitive expression of the analysis results of a statistical model, a nomogram is more concise and effective in quantifying risks. Studies have confirmed that the nomogram has a good application effect in predicting the risk of acute kidney injury in patients with acute myocardial infarction after percutaneous coronary intervention (48) and in identifying the risk of HF in patients with CHD (49). However, no studies on the development of 1-year prognosis risk in CHD patients combined with AHF have been published. In our study, 6 independent risk factors affecting the occurrence of MACE in CHD patients combined with AHF after discharge from the hospital were screened out by step-wise regression, and a personalized nomogram prediction model was constructed. The AUC values for the training and validation cohorts were 0.847 (95% CI: 0.811–0.882) and 0.839 (95% CI: 0.780–0.893), with sensitivity and specificity of 0.740 and 0.705 and 0.815 and 0.877, respectively, suggesting that the nomogram had good prediction ability. It is better than the prediction model reported by Kadoglou et al., which 1-year prediction model has an AUC value of 0.698 (25). The Hosmer-Lemeshow test confirmed that the deviation between the risk prediction value of the nomogram and the actual observed value was not statistically significant (χ2 = 6.522, P = 0.589 in the training cohort and χ2 = 5.648, P = 0.687 in the validation cohort, respectively). Moreover, the calibration curve shows the mean absolute error of the internal verification of the nomogram, which is 0.008 and 0.024, respectively, indicating that the nomogram has good calibration and pretest uniformity. DCA curve analysis showed that the nomogram had good clinical applicability. Meanwhile, the prediction indexes required for constructing the nomogram are all derived from the clinical data of patients during hospitalization, which is easy to obtain and does not require complex calculation transformation. In conclusion, the nomogram for predicting the risk of 1-year MACE in CHD patients combined with AHF after discharge from the hospital has a high predictive value and clinical application value, and targeted preventive measures can be formulated for patients to reduce the occurrence of MACE in CHD patients combined with AHF.
This study has several limitations. Firstly, this was a single-center and retrospective study with a small sample size. The training and validation cohorts’ data in this study were based on the researcher’s location. Therefore, the 1-year MACE clinical predictive model must be verified in additional regional databases. Secondly, we did not analyze the occurrence of MACE in patients in the two subgroups of newly developed AHF and ADCHF due to the smaller sample size and heterogeneity of baseline data, which would lead to decreased accuracy of results. Finally, the clinical relevance or applicability of the nomogram we constructed should be validated in a prospective cohort of patients.
In conclusion, this study identified 6 predictors of 1-year prognosis of MACE in CHD patients combined with AHF. A clinical predictive model is established based on the predictors to identify who will experience short-term MACE, to enhance more effective clinical intervention for CHD patients combined with AHF. DCA confirmed the clinical usefulness of the nomogram.
The raw data supporting the conclusions of this article will be made available by the authors, without undue reservation.
Written informed consent was obtained from the individual(s) for the publication of any potentially identifiable images or data included in this article.
XH, SY, JL, BG, FO, and QH: conception and design. XH, QZ, XC, JP, JC, SL, and FO: acquisition of data. XH, SY, BG, FO, LD, YD, JC, and QH: analysis and interpretation of data. XH, SY, BG, and JL: drafting or revising the article. All authors contributed to the article and approved the submitted version.
This research was funded by the key medical talents training project of Shunde District, Foshan. This research was supported by grants of Foshan Self-funded Science and Technology Project (2020001005216); the Scientific Research Foundation for Clinical Research of Shunde Hospital, Southern Medical University (CRSP2022005); the Scientific Research Foundation for the Younger Researchers of Southern Medical University (PY2018N116); and the Guangdong Medical Science and Technology Research Fund (A2020395, A2020089, and A2021483).
The authors declare that the research was conducted in the absence of any commercial or financial relationships that could be construed as a potential conflict of interest.
All claims expressed in this article are solely those of the authors and do not necessarily represent those of their affiliated organizations, or those of the publisher, the editors and the reviewers. Any product that may be evaluated in this article, or claim that may be made by its manufacturer, is not guaranteed or endorsed by the publisher.
1. Pranata R, Tondas AE, Yonas E, Vania R, Yamin M, Chandra A, et al. Differences in clinical characteristics and outcome of de novo heart failure compared to acutely decompensated chronic heart failure-systematic review and meta-analysis. Acta Cardiol. (2021) 76:410-420. doi: 10.1080/00015385.2020.1747178
2. Bahit MC, Kochar A, Granger CB. Post-myocardial infarction heart failure. JACC Heart Fail. (2018) 6:179–86. doi: 10.1016/j.jchf.2017.09.015
3. Olchanski N, Vest AR, Cohen JT, DeNofrio D. Two-year outcomes and cost for heart failure patients following discharge from the hospital after an acute heart failure admission. Int J Cardiol. (2020) 307:109–13. doi: 10.1016/j.ijcard.2019.10.033
4. Hao Y, Xie Y, Li H, Bao C. The safety and effffect of small doses of perindopril on the patients of CHD with heart failure and low-normal blood pressure. J Hypertens. (2018) 36(Suppl):3. doi: 10.1097/01.hjh.0000548471.26053.cc
5. Granger CB, Goldberg RJ, Dabbous O, Pieper KS, Eagle KA, Cannon CP, et al. Predictors of hospital mortality in the global registry of acute coronary events. Arch Intern Med. (2003) 163:2345–53. doi: 10.1001/archinte.163.19.2345
6. Collet J, Thiele H, Barbato E, Barthélémy O, Bauersachs J, Bhatt DL, et al. 2020 esc guidelines for the management of acute coronary syndromes in patients presenting without persistent st-segment elevation. Eur Heart J. (2021) 42:1289–367.
7. Jneid H, Addison D, Bhatt DL, Fonarow GC, Gokak S, Grady KL, et al. 2017 aha/acc clinical performance and quality measures for adults with stelevation and non-st-elevation myocardial infarction. J Am Coll Cardiol. (2017) 70:2048–90. doi: 10.1016/j.jacc.2017.06.032
8. Allen LA, Matlock DD, Shetterly SM, Xu S, Levy WC, Portalupi LB, et al. Use of risk models to predict death in the next year among individual ambulatory patients with heart failure. JAMA Cardiol. (2017) 2:435–41. doi: 10.1001/jamacardio.2016.5036
9. Krumholz HM, Merrill AR, Schone EM, Schreiner GC, Chen J, Bradley EH, et al. Patterns of hospital performance in acute myocardial infarction and heart failure 30-day mortality and readmission. Circ Cardiovasc Qual Outcomes. (2009) 2:407–13. doi: 10.1161/CIRCOUTCOMES.109.883256
10. Desai AS, Stevenson LW. Rehospitalization for heart failure: Predict or prevent? Circulation. (2012) 126:501–6. doi: 10.1161/CIRCULATIONAHA.112.125435
11. Ali D, Banerjee P. Inpatient monitoring of decompensated heart failure: What is needed? Curr Heart Fail Rep. (2017) 14:393–7. doi: 10.1007/s11897-017-0352-x
12. Ezekowitz JA, Kaul P, Bakal JA, Armstrong PW, Welsh RC, McAlister FA, et al. Declining in-hospital mortality and increasing heart failure incidence in elderly patients with first myocardial infarction. J Am Coll Cardiol. (2009) 53:13–20. doi: 10.1016/j.jacc.2008.08.067
13. Mcmanus DD, Piacentine SM, Lessard D, Gore JM, Yarzebski J, Spencer FA, et al. Thirty-year (1975 to 2005) trends in the incidence rates, clinical features, treatment practices, and short-term outcomes of patients <55 years of age hospitalized with an initial acute myocardial infarction. Am J Cardiol. (2011) 108:477–82. doi: 10.1016/j.amjcard.2011.03.074
14. Gerber Y, Weston SA, Berardi C, McNallan SM, Jiang R, Redfield MM, et al. Contemporary trends in heart failure with reduced and preserved ejection fraction after myocardial infarction: a community study. Am J Epidemiol. (2013) 178:1272–80. doi: 10.1093/aje/kwt109
15. Gerber Y, Weston SA, Enriquez-sarano M, Berardi C, Chamberlain AM, Manemann SM, et al. Mortality associated with heart failure after myocardial infarction: a contemporary community perspective. Circ Heart Fail. (2016) 9:e002460. doi: 10.1161/CIRCHEARTFAILURE.115.002460
16. Gayat E, Arrigo M, Littnerova S, Sato N, Parenica J, Ishihara S, et al. Heart failure oral therapies at discharge are associated with better outcome in acute heart failure: a propensity-score matched study. Eur J Heart Fail. (2018) 20:345–54. doi: 10.1002/ejhf.932
17. Ong MK, Romano PS, Edgington S, Aronow HU, Auerbach AD, Black JT, et al. Effectiveness of remote patient monitoring after discharge of hospitalized patients with heart failure: the Better Effectiveness After Transition-Heart Failure (BEAT-HF) randomized clinical trial. JAMA Intern Med. (2016) 176:310–310. doi: 10.1001/jamainternmed.2015.7712
18. Lupón J, Domingo M, de Antonio M, Zamora E, Santesmases J, Díez-Quevedo C, et al. Aging and heart rate in heart failure: clinical implications for long-term mortality. Mayo Clin Proc. (2015) 90:765–72. doi: 10.1016/j.mayocp.2015.02.019
19. Marui A, Nishiwaki N, Komiya T, Hanyu M, Tanaka S, Kimura T, et al. Comparison of 5-year outcomes after coronary artery bypass grafting in heart failure patients with versus without preserved left ventricular ejection fraction (from the CREDO-Kyoto CABG Registry Cohort-2). Am J Cardiol. (2015) 116:580–6. doi: 10.1016/j.amjcard.2015.05.020
20. Donal E, Lund LH, Oger E, Hage C, Persson H, Reynaud A, et al. New echocardiographic predictors of clinical outcome in patients presenting with heart failure and a preserved left ventricular ejection fraction: a subanalysis of the Ka (Karolinska) Ren (Rennes) Study. Eur J Heart Fail. (2015) 17:680–8. doi: 10.1002/ejhf.291
21. George M, Rajaram M, Shanmugam E, VijayaKumar TM. Novel drug targets in clinical development for heart failure. Eur J Clin Pharmacol. (2014) 70:765–74.
22. Balachandran VP, Gonen M, Smith JJ, DeMatteo RP. Nomograms in oncology: more than meets the eye. Lancet Oncol. (2015) 16:173–80. doi: 10.1016/S1470-2045(14)71116-7
23. Heart Failure Group of Chinese Society of Cardiology of Chinese Medical Association, Chinese Heart Failure Association of Chinese Medical Doctor Association, Editorial Board of Chinese Journal of Cardiology. [Chinese guidelines for the diagnosis and treatment of heart failure 2018]. Zhonghua Xin Xue Guan Bing Za Zhi. (2018) 46:760–89.
24. Shah B. Two Year Mortality of New York Heart Association (NYHA) Functional Class IIand III Congestive Heart Failurep. J Heart Health. (2017) 1.
25. Kadoglou NPE, Parissis J, Karavidas A, Kanonidis I, Trivella M. Assessment of acute heart failure prognosis: the promising role of prognostic models and biomarkers. Heart Fail Rev. (2022) 27:655–63. doi: 10.1007/s10741-021-10122-9
26. Jia Q, Wang YR, Ping HE, Huang XL, Yan W, Mu Y, et al. Prediction model of in-hospital mortality in elderly patients with acute heart failure based on retrospective study. J Geriatr Cardiol. (2017) 14:669–78.
27. Ibanez B, James S, Agewall S, Antunes MJ, Bucciarelli-Ducci C, Bueno H, et al. 2017 ESC Guidelines for the management of acute myocardial infarction in patients presenting with ST-segment elevation: the task force for the management of acute myocardial infarction in patients presenting with ST-segment elevation of the European Society of Cardiology (ESC). Eur Heart J. (2018) 39:119–77.
28. Hassanein M, Abdelhamid M, Ibrahim B, Elshazly A, Aboleineen MW, Sobhy H, et al. Clinical characteristics and management of hospitalized and ambulatory patients with heart failure-results from ESC heart failure long-term registry – Egyptian cohort. ESC Heart Fail. (2015) 2:159–67. doi: 10.1002/ehf2.12046
29. McDonagh TA, Metra M, Adamo M, Gardner RS, Baumbach A, Böhm M, et al. 2021 ESC Guidelines for the diagnosis and treatment of acute and chronic heart failure. Eur Heart J. (2021) 42:3599–3726.
30. Lee DS, Austin PC, Rouleau JL, Liu PP, Naimark D, Tu JV, et al. Predicting mortality among patients hospitalized for heart failure: derivation and validation of a clinical model. JAMA. (2003) 290:2581–7. doi: 10.1001/jama.290.19.2581
31. Gao L, Moodie M. Modelling the lifetime cost-effectiveness of catheter ablation for atrial fibrillation with heart failure. BMJ Open. (2019) 9:e031033. doi: 10.1136/bmjopen-2019-031033
32. Santoro GM, Carrabba N, Migliorini A, Parodi G, Valenti R. Acute heart failure in patients with acute myocardial infarction treated with primary percutaneous coronary intervention. Eur J Heart Fail. (2008) 10:780–5. doi: 10.1016/j.ejheart.2008.06.004
33. Heart Failure Group of Chinese Cardiology Association, Heart Failure Professional Committee of Chinese Medical Doctor Association, Editorial Board of Chinese Journal of Cardiology. Clinical guidelines for the diagnosis and treatment of heart failure in China. Chin J Cardiol. (2018) 46:760-789.
34. Targher G, Dauriz M, Laroche C, Temporelli PL, Hassanein M, Seferovic PM, et al. In-hospital and 1-year mortality associated with diabetes in patients with acute heart failure:results from the ESC-HFA heart failure long-term registry. Eur J Heart Fail. (2017) 19:54–65. doi: 10.1002/ejhf.679
35. Mebazaa A, Gayat E, Lassus J, Meas T, Mueller C, Maggioni A, et al. Association between elevated blood glucose and outcome in acute heart failure results from an international observational cohort. J Am Coll Cardiol. (2013) 61:820–9. doi: 10.1016/j.jacc.2012.11.054
36. Younis A, Mulla W, Goldkorn R, Klempfner R, Peled Y, Arad M, et al. Differences in mortality of new-onset (De-Novo) acute heart failure versus acute decompensated chronic heart failure. Am J Cardiol. (2019) 124:554-559. doi: 10.1016/j.amjcard.2019.05.031
37. Senni M, Gavazzi A, Oliva F, Mortara A, Urso R, Pozzoli M, et al. In-hospital and 1-year outcomes of acute heart failure patients according to presentation (de novo vs. worsening) and ejection fraction. results from IN-HF outcome registry. Int J Cardiol. (2014) 173:163-169. doi: 10.1016/j.ijcard.2014.02.018
38. Degoricija V, Trbušiæ M, Potoènjak I, Raduloviæ B, Terešak SD, Pregartner G, et al. Acute Heart Failure developed as worsening of Chronic Heart Failure is associated with increased mortality compared to de novo cases. Sci Rep. (2018) 8:9587. doi: 10.1038/s41598-018-28027-3
39. Maisel A, Mueller C, Adams K, Anker SD, Aspromonte N, Cleland JG, et al. State of the art: using natriuretic peptide levels in clinical practice. Eur J Heart Fail. (2008) 10:824–39. doi: 10.1016/j.ejheart.2008.07.014
40. Goetze JP, Gore A, Møller CH, Steinbrüchel DA, Rehfeld JF, Nielsen LB, et al. Acute myocardial hypoxia increases BNP gene expression. FASEB J. (2004) 18:1928–30. doi: 10.1096/fj.03-1336fje
41. Koch M, Haastert B, Kohnle M, Rump LC, Kelm M, Trapp R, et al. Peritoneal dialysis relieves clinical symptoms and is well tolerated in patients with refractory heart failure and chronic kidney disease. Eur J Heart Fail. (2012) 14:530–9. doi: 10.1093/eurjhf/hfs035
42. Patton KK, Ellinor PT, Heckbert SR, Christenson RH, DeFilippi C, Gottdiener JS, et al. N-terminal pro-B-type natriuretic peptide is a major predictor of the development of atrial fibrillation: the Cardiovascular Health Study. Circulation. (2009) 120:1768–74. doi: 10.1161/CIRCULATIONAHA.109.873265
43. Belkin M, Wussler D, Kozhuharov N, Strebel I, Walter J, Michou E, et al. Discordance in prognostic ability between physician assessed NYHA classification and self-reported health status in patients with acute heart failure. Eur Heart J. (2021) 42(Suppl. 1):ehaa946.1186. doi: 10.1093/eurheartj/ehab724.1030
44. Sheng G, Shen Y, Wu X, Zhu JH, Qin XT, Gou L, et al. Association of Lp-PLA2 mass and heart failure in a cohort of Nantong population. Int J Clin Exp Med. (2017) 10:14605–13.
45. Agoston-Coldea L, Mocan T, Gatfosse M, Lupu S, Dumitrascu DL. Plasma homocysteine and the severity of heart failure in patientswith previous myocardial infarction. Cardiol J. (2011) 18:55–62.
46. Naruszewicz M, Jankowska EA, Zymlinski R, Bukowska H, Millo B, Banasiak W, et al. Hyperhomocysteinemia in patients with symptomatic chronic heartfailure: prevalence and prognostic importance-pilot study. Atherosclerosis. (2007) 194:408–14. doi: 10.1016/j.atherosclerosis.2006.08.014
47. Fournier P, Fourcade J, Roncalli J, Salvayre R, Galinier M, Caussé E, et al. Homocysteine in chronic heart failure. Clin Lab. (2015) 61: 1137–45. doi: 10.7754/Clin.Lab.2015.141238
48. Guo BL, Ouyang FS, Yang SM, Liu ZW, Lin SJ, Meng W, et al. Development of a preprocedure nomogram for predicting contrast-induced acute kidney injury after coronary angiography or percutaneous coronary intervention. Oncotarget. (2017) 8:75087–93. doi: 10.18632/oncotarget.20519
Keywords: acute heart failure, major adverse cardiac events, prognosis, clinical predictive model, coronary heart disease
Citation: Huang X, Yang S, Chen X, Zhao Q, Pan J, Lai S, Ouyang F, Deng L, Du Y, Chen J, Hu Q, Guo B and Liu J (2022) Development and validation of a clinical predictive model for 1-year prognosis in coronary heart disease patients combine with acute heart failure. Front. Cardiovasc. Med. 9:976844. doi: 10.3389/fcvm.2022.976844
Received: 23 June 2022; Accepted: 22 August 2022;
Published: 04 October 2022.
Edited by:
Jie Yu, University of New South Wales, AustraliaReviewed by:
Suhua Li, Third Affiliated Hospital of Sun Yat-sen University, ChinaCopyright © 2022 Huang, Yang, Chen, Zhao, Pan, Lai, Ouyang, Deng, Du, Chen, Hu, Guo and Liu. This is an open-access article distributed under the terms of the Creative Commons Attribution License (CC BY). The use, distribution or reproduction in other forums is permitted, provided the original author(s) and the copyright owner(s) are credited and that the original publication in this journal is cited, in accordance with accepted academic practice. No use, distribution or reproduction is permitted which does not comply with these terms.
*Correspondence: Baoliang Guo, dG9tY2F0Y2Nrc0AxNjMuY29t; Jiemei Liu, amllbWVpbGl1MjAyMkAxNjMuY29t
†These authors have contributed equally to this work and share first authorship
Disclaimer: All claims expressed in this article are solely those of the authors and do not necessarily represent those of their affiliated organizations, or those of the publisher, the editors and the reviewers. Any product that may be evaluated in this article or claim that may be made by its manufacturer is not guaranteed or endorsed by the publisher.
Research integrity at Frontiers
Learn more about the work of our research integrity team to safeguard the quality of each article we publish.