- 1Key Laboratory of Molecular Cardiovascular Sciences, Department of Cardiology, Institute of Vascular Medicine, Ministry of Education, Peking University Third Hospital, Beijing, China
- 2Department of Cardiology, Xinhua Hospital, Shanghai Jiaotong University School of Medicine, Shanghai, China
- 3Department of Cardiology, State Key Laboratory of Cardiovascular Disease, Fuwai Hospital, National Center for Cardiovascular Diseases, Chinese Academy of Medical Sciences and Peking Union Medical College, Beijing, China
- 4Graduate School of Peking Union Medical College, Chinese Academy of Medical Sciences and Peking Union Medical College, Beijing, China
- 5Department of Epidemiology and Biostatistics, School of Public Health, Peking University, Beijing, China
- 6Department of Global Health, School of Public Health, Peking University, Beijing, China
- 7Center for Intelligent Public Health, Institute for Artificial Intelligence, Peking University, Beijing, China
Background: Observational studies have shown inconsistent results of the associations between type 2 diabetes mellitus (T2DM) and blood lipid profiles, while there is also a lack of evidence from randomized controlled trials (RCTs) for the causal effects of T2DM on blood lipid profiles and lipoprotein subclasses.
Objectives: Our study aimed at investigating the causal effects of T2DM on blood lipid profiles and concentration of particle-size-determined lipoprotein subclasses by using the two-sample Mendelian randomization (MR) method.
Methods: We obtained genetic variants for T2DM and blood lipid profiles including high-density lipoprotein-cholesterol (HDL-C), low-density lipoprotein-cholesterol (LDL-C), triglycerides (TG), and total cholesterol (TC) from international genome-wide association studies (GWASs). Two-sample MR method was applied to explore the potential causal effects of genetically predicted T2DM on blood lipid profiles based on different databases, respectively, and results from each MR analysis were further meta-analyzed to obtain the summary results. The causal effects of genetically predicted T2DM on the concentration of different subclasses of lipoproteins that are determined by particle size were also involved in MR analysis.
Results: Genetically predicted 1-unit higher log odds of T2DM had a significant causal effect on a higher level of TG (estimated β coefficient: 0.03, 95% confidence interval [CI]: 0.00 to 0.06) and lower level of HDL-C (estimated β coefficient: −0.09, 95% CI: −0.11 to −0.06). The causality of T2DM on the level of TC or LDL-C was not found (estimated β coefficient: −0.01, 95% CI: −0.02 to 0.01 for TC and estimated β coefficient: 0.01, 95% CI: −0.01 to 0.02 for LDL-C). For different sizes of lipoprotein particles, 1-unit higher log odds of T2DM was causally associated with higher level of small LDL particles, and lower level of medium HDL particles, large HDL particles, and very large HDL particles.
Conclusion: Evidence from our present study showed causal effects of T2DM on the level of TG, HDL-C, and concentration of different particle sizes of lipoprotein subclasses comprehensively, which might be particularly helpful in illustrating dyslipidemia experienced by patients with T2DM, and further indicate new treatment targets for these patients to prevent subsequent excessive cardiovascular events from a genetic point of view.
Introduction
Type 2 diabetes mellitus (T2DM), a global serious condition with reduced quality of life and life expectancy, is expected to reach 550 million people by 2030 (1), and the estimated global health expenditure on T2DM will be $490 billion in 2030 (2). The mortality of cardiovascular disease (CVD) in patients with T2DM was 2–4 times higher compared with non-diabetic population (3, 4). These observational studies arouse the recognition of T2DM as an essential risk factor for CVD.
Dyslipidemia, one of the key cardiometabolic risk factors, was found to be associated with excessive CVD risk in patients with T2DM (5). Dyslipidemia included disorders of high-density lipoprotein-cholesterol (HDL-C), low-density lipoprotein-cholesterol (LDL-C), very low-density lipoprotein-cholesterol (VLDL-C), triglycerides (TG) and total cholesterol (TC). Among these blood lipid profiles, LDL-C was proved to be a major atherogenic lipoprotein which was also a key risk factor for CVD. Reducing the level of LDL-C of patients with T2DM showed significant benefit in CVD risk reduction according to previous randomized controlled trials (RCTs) (6, 7), while evidence supporting the benefits of control of other blood lipid profiles was inadequate. Only LDL-C lowering treatment was recommended by current guidelines to reduce the excessive risk of CVD among patients with T2DM (8–10). However, the Framingham Heart Study found no statistically significant difference in the level of LDL-C and TC in patients with T2DM compared to those without T2DM. Significantly higher level of TG and lower level of HDL-C were found among patients with T2DM compared to patients with non-diabetes instead (11), which were further supported by subsequent observational studies (12–15). The above studies indicated that the efficacy of lowering LDL-C to attenuate excessive CVD risk among patients with T2DM still needs to be reconfirmed, while potential effects of other types of lipid profiles on excessive CVD risk should be taken into consideration seriously. In addition, as the technology of lipoprotein profiling advanced in recent years, measuring the concentration of lipoprotein particles and sub-particles that are related to cardiometabolic risks might provide better predictors of CVD risks among patients with T2DM than traditional lipid panels (16–18). However, the previous observational studies only displayed the associations of T2DM with alterations of blood lipid profiles, without uncovering the causal effects of T2DM on various blood lipid profiles and different sizes of lipoproteins sub-particles remained largely unexamined.
Mendelian randomization (MR) is a genetic epidemiological method to explore the causality of an exposure on an outcome using genetic variants as instrumental variables (19). Unlike observational studies which are more prone to be biased by reverse causality, measurement error, and other confounding factors, the genetic variants used in MR were determined at conception which had lifelong influence and thus could largely avoid reverse causality and other confounding factors. MR could provide efficient and robust results that closely resemble those of RCTs as nature's randomized trials as there is currently a lack of well-conducted, high-quality RCTs through which to investigate the causal relationships of T2DM and blood lipid profiles.
Therefore, to provide more efficient and precise treatment strategies to reduce the excessive CVD risk in patients with T2DM, we conducted a two-sample MR study by analyzing genome-wide association studies (GWASs) summary statistics from international genetic consortia to further explore the potential causal effects of genetically predicted T2DM on genetically predicted various blood lipid profiles as well as genetically determined different particle sizes of lipoproteins.
Methods
Overall study design
First, we conducted a two-sample MR study by using genetic instrumental variables as proxies for T2DM and blood lipid profiles to investigate the potential causal effects of genetically predicted T2DM on genetically predicted blood lipid profiles according to different large GWAS data resources, respectively. Then, we performed an overall meta-analysis to obtain the summary results by meta-analyzing each MR result to determine those causal effects more comprehensively across different data sources. An overview of our study design is shown in Figure 1.
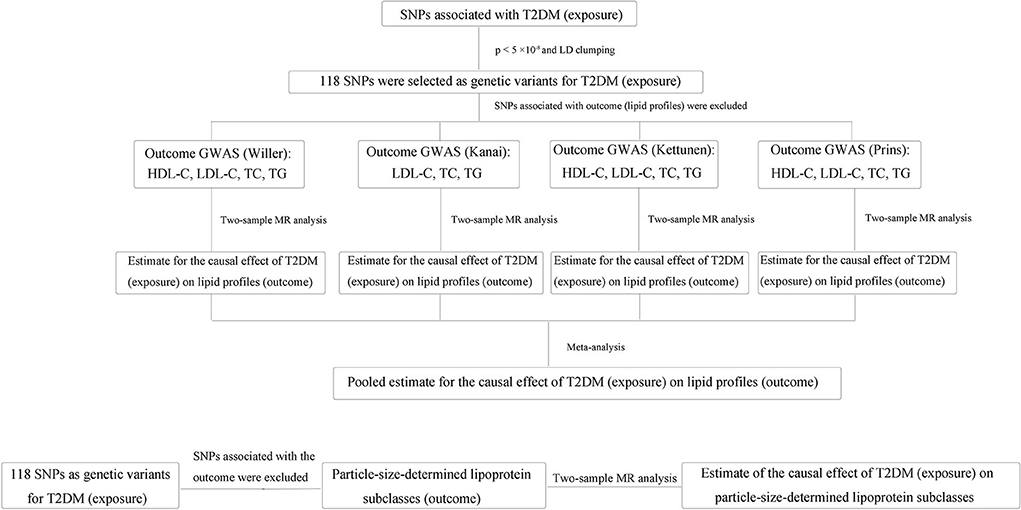
Figure 1. Flow diagram of the overview of our study design. First, we conducted a two-sample MR study to investigate the potential causal effects of genetically predicted T2DM on genetically predicted blood lipid profiles according to different large GWAS data resources, respectively. Then, we performed the overall meta-analysis to obtain the summary results by meta-analyzing each MR result to determine those causal effects more comprehensively across different data sources. In addition, the estimates of the causal effect of T2DM on each particle-size-determined lipoprotein subclass were also made via the two-sample MR method. SNP, single-nucleotide polymorphism; T2DM, type 2 diabetes mellitus; GWAS, genome-wide association study; HDL, high-density lipoprotein; LDL, low-density lipoprotein; TC, total cholesterol; TG, triglycerides; MR, Mendelian randomization.
Data sources
Genetic instrumental variable for T2DM
We searched GWAS summarized data and extracted 143 single-nucleotide polymorphisms (SNPs) from the latest analysis including 62,892 patients with T2DM and 596,424 controls of European ancestry (20). Among these SNPs, 118 were finally used for genetic variants for T2DM which met the standard of genome-wide significance (p-value <5 × 10−8) and not in linkage disequilibrium (r2 < 0.001). The analysis we selected was larger in scale than previous studies and explained 10% of the heritability of T2DM (20) which was almost two times than previous GWAS (21–23). Genetic variants used as proxies for T2DM in our study are shown in Supplementary Table 1.
GWAS summary statistics for blood lipid profiles
Genome-wide association studies' summary statistics for HDL-C, LDL-C, TC, and TG were obtained from various sources including: (1) a GWAS including 1,88,578 European ancestry individuals and 7,898 non-European ancestry individuals (24); (2) 24,925 European ancestry participants from a GWAS (25); (3) 9,961 European ancestry individuals from the UK Household Longitudinal Study (26); and (4) a GWAS including 1,62,255 Asian ancestry individuals (27). The GWAS including 24,925 individuals further categorized LDL and HDL according to the particle size of lipoproteins, and we took each category (e.g., small LDL, medium LDL, and large LDL) into subsequent subgroup analysis. Summary information of the GWAS sources for outcomes in our study are shown in Table 1.
Statistical analysis
All the analyses in our study were performed by using R version 4.0.3 (The R Foundation for Statistical Computing) through TwoSampleMR and Meta package.
Mendelian randomization
The MR method used in our study was based on three assumptions: (1) the genetic variants used as instrumental variables are associated with the exposure; (2) the genetic variants are not associated with other confounders; and (3) the genetic variants are only associated with the outcome through the exposure (28). In addition, estimated SNPs were used to calculate the causal effects of exposure (genetically predicted T2DM) on the outcome (genetically predicted level of blood lipid profiles and concentration of particle-size-determined lipoprotein subclasses) using an inverse-variance weighted (IVW) which combined the estimates from each SNP based on summarized GWAS database. For binary exposure (T2DM), MR estimates are odds ratios (ORs) per genetically predicted unit difference in log odds of having the relevant exposure (T2DM). Thus, causal estimates are presented as the association of one unit genetically predicted higher log odds of T2DM with genetically predicted levels of various blood lipid profiles and concentration of particle-size-determined lipoprotein subclasses. We scaled genetically predicted effect size of the genetic variant of exposure (T2DM) on the continuous outcome (levels of various blood lipid profiles and concentrations of particle-size-determined lipoprotein subclasses) as one copy addition of the effect allele of exposure on standard deviation units difference in outcome according to the database resources we referred to. IVW method was applied as the main analysis because of its efficiency, but it must satisfy all three assumptions for MR (29). Results of IVW were expressed as estimated β coefficient, standard error, 95% confidence interval (CI) keeping two decimals and p-value. P-value <0.05 indicates statistical significance. It is noteworthy that even one invalid genetic instrument may cause bias for IVW results (29). In addition, if the strength of genetic variants is not strong enough, this “weak instrument bias” may also cause bias. Besides, horizontal pleiotropy may also greatly influence the results of IVW. Therefore, we applied multiple sensitivity analyses to evaluate those possible biases.
MR sensitivity analysis
We performed various MR sensitivity analyses to testify whether our results were influenced by the violation of the three assumptions of MR. F-statistic for each SNP was calculated to examine whether weak instrument bias might influence IVW results. F-statistic larger than 10 indicates less prone to weak instrument bias (30). Simple median estimator and weighted median estimator analysis were also performed and the estimated results would be consistent with IVW if at least more than half of the genetic variants were valid. Briefly speaking, a simple median can be regarded as a certain situation that weighted median estimators have equal weights (31). We applied the weighted median method to explore whether the results of IVW were influenced by an invalid instrument. The p-value of both IVW and weighted median was <0.05, indicating that the result of IVW was not likely to be influenced by invalid instrument bias. MR-Egger regression and the intercept of MR-Egger estimates the horizontal pleiotropy which is the main source of bias for MR studies (32). The p-value of MR-Egger regression larger than 0.05 indicated horizontal pleiotropy, and the p-value of MR-Egger intercept suggested whether such pleiotropy was statistically significant. Mendelian randomization pleiotropy residual sum and outlier (MR-PRESSO) was designed to detect and correct for pervasive horizontal pleiotropy (33). A unique test in MR-PRESSO called “the distortion test” allows it to evaluate whether the difference between the causal estimate before and after the removal of outliers is significant. Whether our results were influenced by horizontal pleiotropy was carefully examined according to the results of MR-Egger regression, MR-Egger intercept, and MR-PRESSO. Besides, we still applied single-SNP analysis to identify the association between each genetic variant and the outcome and leave-one-out analysis to explore whether the causality of the exposure on the outcome is mainly credited to a certain genetic variant. All the MR analyses were performed in the “TwoSampleMR” package in R software.
Meta-analysis of the estimates from various outcome sources
We performed MR analyses to identify the causality of T2DM on each blood lipid profile according to each outcome source, respectively, and these results were further meta-analyzed to obtain the pooled estimates for the causality of T2DM on each blood lipid profile. I2 statistics were calculated to quantify the heterogeneity between estimates from different outcome sources. All meta-analyses were performed using the “Meta” package in R software.
Results
Pooled estimate of the causal effect of T2DM on blood lipid profiles
Estimate of the causal effect of T2DM on each data source was made individually and details are shown in Supplementary Tables 2–16, and genetic association for the causal effect of T2DM on blood lipid profiles using the IVW method in each data source is shown in Table 2. Pooled β for HDL-C, LDL-C, TC, and TG per unit increase in log odds of T2DM was −0.09 (95% CI: −0.11 to −0.06, I2 = 62%, p-value = 0.07), 0.00 (95% CI: −0.03 to 0.02, I2 = 67%, p-value = 0.03), −0.01 (95% CI: −0.02 to 0.01, I2 = 35%, p-value = 0.20), and 0.06 (95% CI: −0.01 to 0.12, I2 = 80%, p-value < 0.01), respectively (Figure 2). Random-effect model meta-analyses were used due to the heterogeneity across databases in terms of LDL-C and TG when pooling the primary analyses results using the IVW method. Fix-effect model meta-analyses were used because the heterogeneity in terms of HDL-C and TC was minor across databases when the primary analyses were pooled using the IVW method.
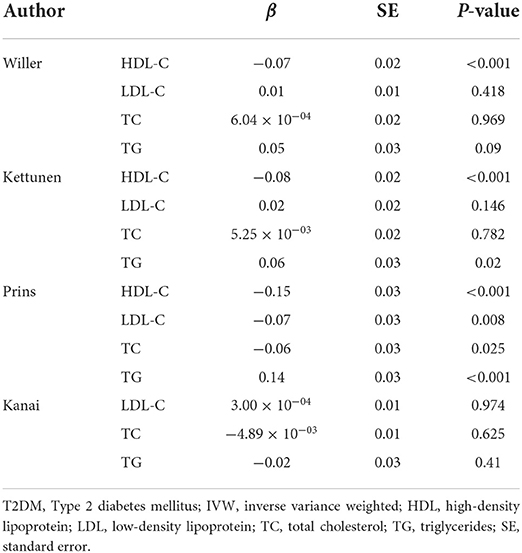
Table 2. Genetic association for the causal effect of T2DM on blood lipid profiles using IVW method in each data source.
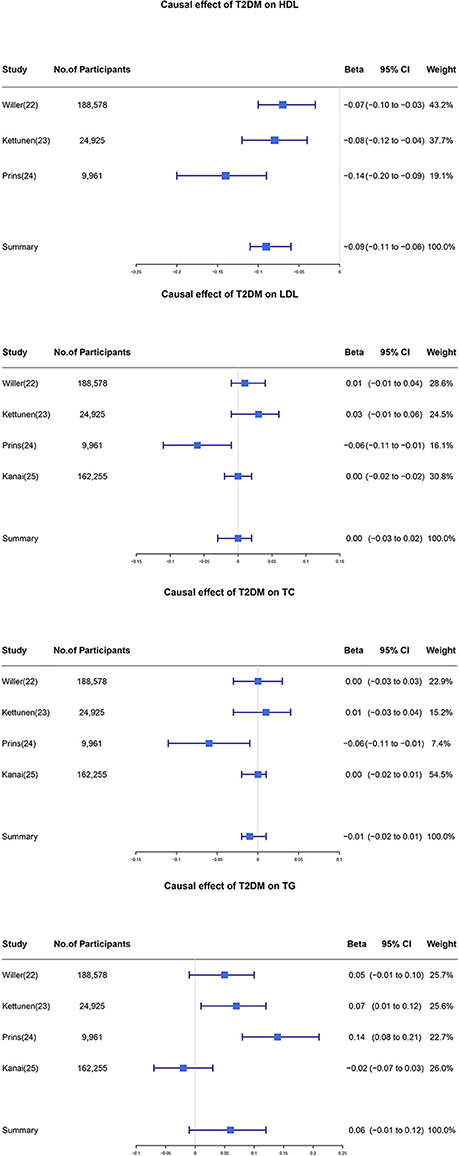
Figure 2. Individual and pooled estimate of the causal effect of T2DM on each blood lipid profile. Firstly we applied the two-sample MR method to estimate the causal effect of T2DM on each blood lipid profile. Then these individual estimates were further meta-analyzed to obtain the pooled results. T2DM, type 2 diabetes mellitus; HDL, high-density lipoprotein; LDL, low-density lipoprotein; TC, total cholesterol; TG, triglycerides; CI, confidence interval.
Sensitivity analysis for heterogeneity
We noticed that the I2 statistics for pooled β in regard to LDL-C and TG were larger than 50% with a p-value <0.05. After performing the senstivity analysis, we identified that the source of heterogeneity was mainly from the UK Household Longitudinal Study. After removing the data from the UK Household Longitudinal Study, pooled β for LDL-C and TG were 0.01 (95% CI: −0.01 to 0.02, I2 = 0%, p-value = 0.44) and 0.03 (95% CI: 0.00 to 0.06, I2 = 66%, p-value = 0.06), respectively (Figure 3). Overall, our results showed that one unit higher log odds of T2DM was associated with lower level of HDL-C and higher level of TG. Pooled β did not support the causality of T2DM on TC and LDL-C levels.
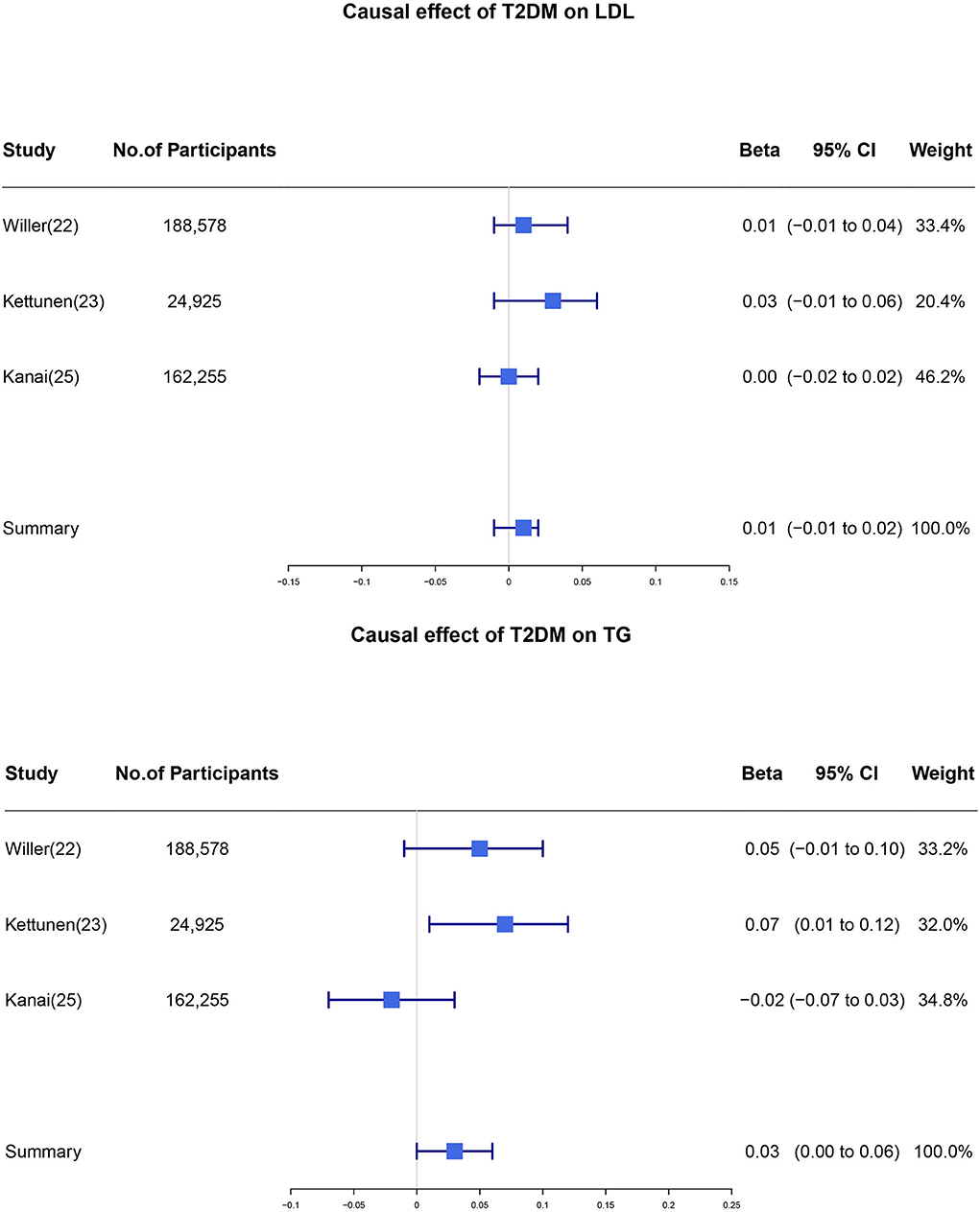
Figure 3. Individual and pooled estimate of the causal effect of T2DM on the level of LDL and TG after sensitivity analysis. We removed the study which generated heterogeneity in our meta-analysis and the individual and pooled estimates of the causal effect of T2DM on the level of LDL and TG were re-analyzed. T2DM, type 2 diabetes mellitus; LDL, low-density lipoprotein; TG, triglycerides; CI, confidence interval.
Estimate of the causal effect of T2DM on different sizes of HDL and LDL particles
Our study further categorized HDL particles and LDL particles into subgroups to investigate whether T2DM had different causal effects on the levels of different particle sizes. Results showed that T2DM had causal effects on the level of medium HDL particles (β: −0.04, 95% CI: −0.08 to −0.01, p-value = 0.019), large HDL particles (β: −0.09, 95% CI: −0.13 to −0.05, p-value < 0.001), and very large HDL particles (β: −0.05, 95% CI: −0.08 to −0.01, p-value = 0.016). In addition, the causality of T2DM on the level of small LDL particles was also found (β: 0.04, 95% CI: 0.00 to 0.08, p-value = 0.048). However, the causal effects of T2DM on the level of small HDL particles or large LDL or medium LDL-C particles were not found (p-value > 0.05) (Figure 4).
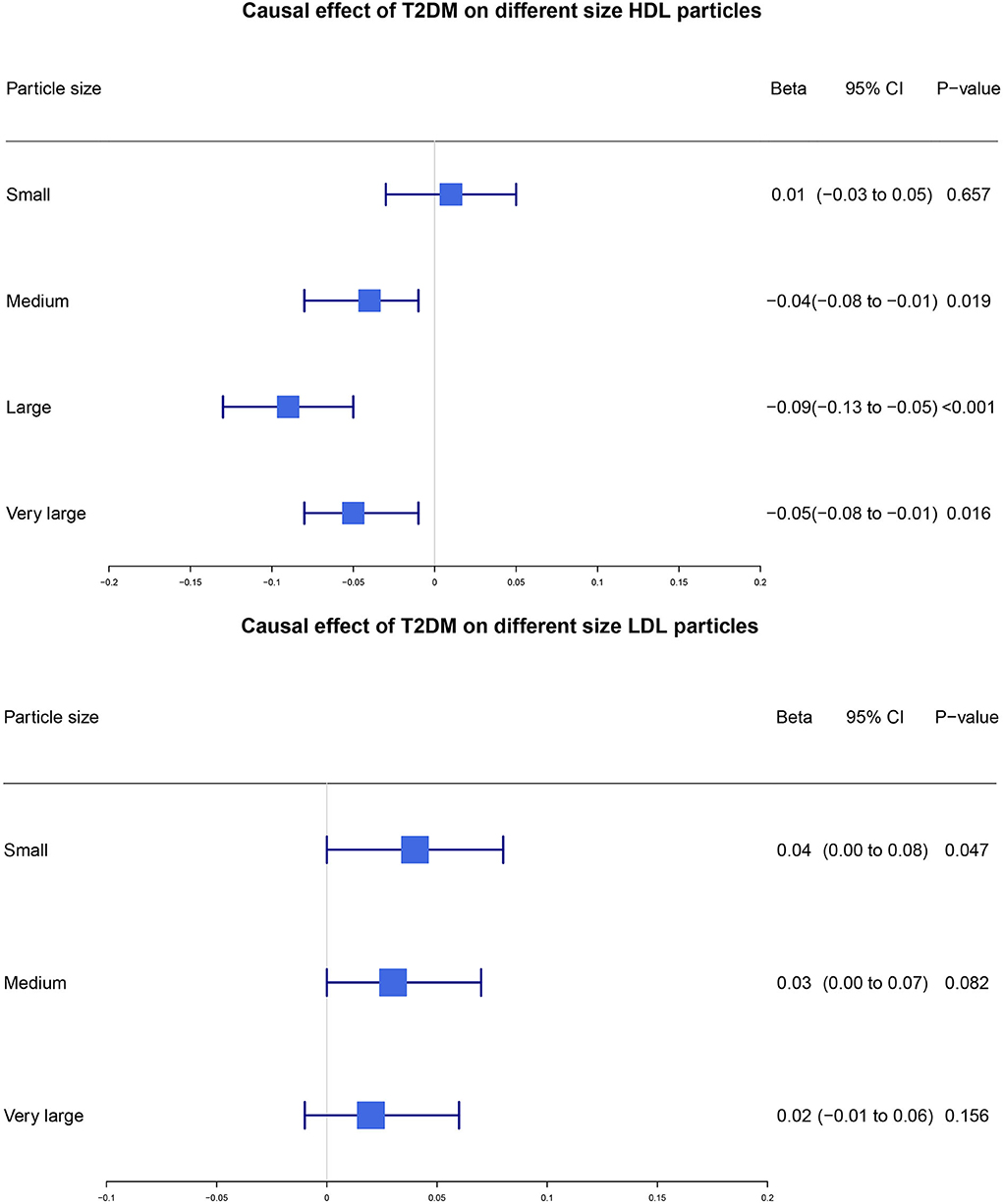
Figure 4. Estimate of the causal effect of T2DM on different sizes of HDL and LDL particles. We used the two-sample MR method to estimate the potential causal effect of T2DM on different sizes of HDL and LDL particles. T2DM, type 2 diabetes mellitus; HDL, high-density lipoprotein; LDL, low-density lipoprotein; CI, confidence interval.
MR sensitivity analyses
In MR sensitivity analyses, F-statistics of genetic variants for T2DM in our study was larger than 10 which indicated that genetic variants included in our study were strong enough to avoid weak instrument bias.
For the estimate of the causality of T2DM on the level of HDL-C (data source: Kettunen et.al), the MR-Egger intercept was non-zero with a p-value larger than 0.05, and the p-value for the distortion test of MR-PRESSO was larger than 0.05, which both indicated that the influence of horizontal pleiotropy was not statistically significant, and the difference between before and after removing outliers which caused horizontal pleiotropy was also not statistically significant either. In addition, the p-value for weighted median was <0.05 which was also consistent with the IVW method. Thus, we reckon that the estimate of the causal effect of T2DM on the level of HDL-C (data source: Kettunen) based on IVW results was robust.
Likewise, for the estimate of the causality of T2DM on the level of LDL-C (data source: Kanai, Kettunen, Willer), TC (data source: Kanai, Kettunen, Willer), TG (data source:Kanai, Willer), results of MR sensitivity analyses showed no horizontal pleiotropy, while for the estimate of the causality of T2DM on the level of LDL-C (data source: Prins), TC (data source: Prins), TG (data source: Kettunen, Prins), and MR sensitivity analyses indicated that the influence of horizontal pleiotropy on our results was not statistically significant either.
Results of single-SNP analyses and leave-one-out analyses were also consistent with our main analyses. The only exception was the estimate of the causality of T2DM on the level of HDL-C (data source: Prins), for the p-value of MR-Egger regression and distortion test in MR-PRESSO was both <0.05, thus we reckon that IVW results might be influenced by horizontal pleiotropy which we further discussed in the limitation part. Details of MR sensitivity analyses are shown in Supplementary Tables 24–69.
Discussion
In the present study, we explored the causal effect of T2DM on blood lipid profiles and found that one additional unit of log odds of T2DM was causally associated with a 4% higher level of TG, 8% lower level of HDL-C, and also had significant causality on the concentration of different sizes of HDL and LDL lipoprotein particles. The above blood lipid profiles causally influenced by T2DM might be more specifically responsible for the excessive CVD risk among patients with T2DM, and new treatments may be developed to target these blood lipid alterations to further reduce secondary CVD risk among patients with T2DM.
As MR studies that explored the potential causal effects of T2DM on blood lipid profiles were lacking and RCTs were also scarce due to the difficulties in design and ethics, thus previous research which illustrated the relationship between T2DM and blood lipid profiles was mainly observational. Results of our study showed that T2DM had a positive causal effect on TG, and a negative causal effect on HDL-C, which were consistent with previous studies (11, 12, 15, 34–36). The causal relationship between T2DM and TC level was not found in our study which was also proved by the Framingham study (11), but there were also opposite results from comparative studies with 8-year follow-up claiming that TC levels of patients with T2DM were higher than non-diabetic participants (men: 5.84 ± 0.18 mmol/L, women: 5.84 ± 0.18 mmol/L vs. men: 5.55 ± 0.05 mmol/L, women: 5.20 ± 0.05 mmol/L, p-value = 0.002) (12). However, this difference may be due to the limited number of participants (17 males and 26 females). Most previous studies showed normal LDL-C levels among patients with T2DM (14, 36–38). A more recent study “PROCAM” also found little difference in the occurrence of high LDL levels between patients with T2DM and non-diabetic participants (22.7 vs. 21.7%) (15).
It is particularly noteworthy that the increasing number of studies in recent years illustrated that there were little differences in LDL-C levels between diabetic and non-diabetic population; however, the level of small dense LDL particles was found to increase instead (39–44) which were in accordance with our MR results. As the increasing number of small dense LDL is the consequence of lipolysis of increasing TG-rich VLDL particles (44), it is rational to observe both the increase of small LDL particles and TG levels in our study. Recent studies have shown that increasing levels of small LDL particles were closely associated with increased risk of CVD (45, 46) and a statement also suggested that cardiovascular disease risk may be more closely related to the number of atherogenic lipoprotein particles than to LDL cholesterol (47). Thus, our MR results which further indicated significant causal effects of genetically predicted T2DM on the subclass of lipoprotein particles could provide more robust evidence for better predictors of future CVD risks than the traditional lipid panel, especially in subjects with cardiometabolic diseases such as T2DM when traditional lipid panel is relatively normal. In addition, previous observational studies also showed that medium and large HDL particles were associated with T2DM (44, 48) which is consistent with the estimated β coefficient for genetically predicted medium, large, and very large HDL particles in our study. Due to the close relationship of atherogenic lipoprotein subclass particles with non-alcoholic fatty liver disease (NAFLD) and subsequent CVD events (49–51), our results may explain the excessive CVD risk among patients with T2DM.
Including data from the UK Household Longitudinal Study in meta-analyzing pooled β for TG and LDL-C could originate significant heterogeneity in our study. After comparing with other GWAS, we found patients taking statins and other lipid-lowering treatments were excluded except for the UK Household Longitudinal Study. Therefore, it might partly explain the lower estimated β coefficient for HDL-C and higher for TG using data from the UK Household Longitudinal Study, and lower estimated β coefficient for LDL-C might be due to statin intake.
Strengths
First, to the best of our knowledge, the present study is the first MR study to identify the potential causality of T2DM on blood lipid profiles. MR studies can largely avoid bias from reverse causation, measurement error, and other unknown confoundings which are responsible for less conclusive results from observational studies. Besides, MR studies are less time-consuming, less expensive, easier to design, and with lifelong exposure compared with RCTs. Therefore, the uniqueness of MR studies makes them an effective method to explore the possible causal effect. Our MR study illustrated that T2DM had causal effects on certain types of blood lipid profiles which might provide help in exploring the complex mechanism of dyslipidemia in patients with T2DM.
Second, using MR-based statistics, we further conducted a meta-analysis to integrate different causal effects of T2DM on blood lipid profiles across distinct data resources to provide a more consolidated and desirable conclusion.
Third, the number of genetic variants used as proxies for each blood lipid profile in the present study is considerable and sensitivity analyses have confirmed the results of MR main analyses are less prone to be biased by weak instruments and less significant influence by horizontal pleiotropy, thus adding to the robustness of MR estimates. In addition, genetic variants for T2DM in the present study were identified from the latest GWAS which was larger in scale and also explained double heritability of T2DM than previous GWAS. Besides, data sources for outcomes were thoroughly searched and carefully checked for consistency, which further contributes to less heterogeneity and more reliability for final pooled MR estimates.
In addition, we further involved MR analysis for genetically predicted HDL and LDL into different subgroups according to the genetically determined size of lipoprotein particles. MR results showed the causal relationship between genetically predicted T2DM with a higher level of small LDL particles, and lower level of medium, large and very large HDL particles, which shed new light on the treatment targets to reduce the CVD risk of patients with T2DM despite the relatively normal level of LDL-C.
Last but not least, the present study design is different from normal MR studies that our individual estimates of the estimated β coefficient for each blood lipid profile were subsequently meta-analyzed to obtain a pooled estimated β coefficient from various data sources which made our results more persuasive.
Limitations
There are several limitations of our study. First of all, the number of GWAS included for each blood lipid profile is not large enough and the sample size of some GWAS was also moderate. For example, the largest GWAS included in the present study recruited 1,88,578 participants, while the sample size of the UK Household Longitudinal Study was <10,000 and the sample size of another GWAS included was merely over 20,000. Second, although the number of genetic variants used in the present study was from the latest GWAS and was far more than previous studies, they could only explain a small proportion of T2DM heritability, leaving almost 90% unexplained. Besides, MR studies can avoid most biases affecting observational studies, but bias from horizontal pleiotropy should always be considered. Horizontal pleiotropy was generated when there were other pathways for the exposure to influence the outcome and the assumption of MR studies was violated, thus resulting in bias for MR main analysis. We have examined that most of our results were robust, while the estimate of the causal effect of T2DM on the level of HDL-C (data source: Prins) might be biased. In addition, restricted by the contradiction between the format of our data sources and the R software codes, we did not perform MR analyses that could detect correlated horizontal pleiotropy including Genome-wide mR Analysis under Pervasive PLEiotropy (GRAPPLE) (52) or Causal Analysis Using Summary Effect Estimates (CAUSE) (53). Future investigators are encouraged to apply more sensitivity analyses to detect both correlated and uncorrelated horizontal pleiotropy in order to minimize bias. Lastly, inspite of the large sample sizes of GWAS included in our study, the effect size of LDL-C and TC was rather small; thus, we could not completely rule out the causality of T2DM on LDL-C and TC. More large-scale GWAS and well-conducted observational studies are expected in future to provide more supportive evidence.
Clinical and public implications
The causal effect of T2DM on LDL-C is still unclear and results from observational studies indicated an increase of small dense LDL-C in patients with T2DM. Results of our study were in accordance with previous studies and also found lower level of very large HDL, large HDL, and medium HDL, which might be indicative of the excessvie CVD risk of patients with T2DM. Besides, statin treatment for lowering LDL-C is widely recommended by various dyslipidemia guidelines for patients with T2DM. The 2016 ESC/EAS Guidelines for the Management of Dyslipidaemias regarded LDL-C as the primary target of lipid-lowering treatment among patients with T2DM (54). However, whether treatment for diabetic patients with higher TG and lower HDL-C is beneficial for CVD risk reduction remains controversial. These might be ascribable to the negative effects on reducing major cardiovascular events by using fenofibrate on patients with T2DM reported by FIELD and ACCORD studies (55, 56). The present study which was conducted in an MR framework indicated that patients with T2DM were more inclined to experience higher level of TG and lower level of HDL-C despite the relatively normal level of LDL-C. Moreover, our MR analysis also uncovered the causal effects of genetically predicted T2DM on genetically predicted atherogenic lipoprotein sub-particles. As the atherogenic lipoprotein sub-particles were closely related to cardiometabolic diseases such as NAFLD and increased subsequent risk of CVD, our results might provide new ideas for future RCTs to evaluate the impact of targeting the concentration of atherogenic lipoproteins sub-particles to attenuate CVD risk among patients with T2DM.
Conclusion
Evidence from our study supported higher level of TG and lower level of HDL-C among patients with T2DM. Moreover, T2DM was also causally associated with higher level of small LDL particles, and lower level of medium, large, and very large HDL particles. New evidence from a genetic point of view for the causality of T2DM on these blood lipid profiles and lipoprotein subclass particles based on our study might be constructive for developing new therapeutic strategies to reduce the excessive CVD risk experienced by patients with T2DM.
Data availability statement
The datasets presented in this study can be found in online repositories. The names of the repository/repositories and accession number(s) can be found in the article/Supplementary material.
Author contributions
KC and JZ contributed equally to this manuscript, performed data analysis, and wrote the manuscript. KC and Y-DT designed this study, also took responsibility for the integrity, accuracy of data analysis in this study, and are the guarantors. CS, QZ, JY, TH, and Y-DT reviewed and revised the manuscript. All authors had access to data in this study and contributed to statistical analysis and reviewing of the manuscript. All listed authors meet authorship criteria. All authors contributed to the article and approved the submitted version.
Funding
This work was supported by (1) National Key R&D Program of China (2020YFC2004700). (2) Research Unit of Medical Science Research Management/Basic and Clinical Research of Metabolic Cardiovascular Diseases from Chinese Academy of Medical Sciences (2021RU003). (3) National Natural Program (81825003). (4) Science and Technology Project of Xicheng District Finance (XCSTS-SD2021-01). (5) Basic research funds of the Central Public Welfare Research Institutes of the Chinese Academy of Medical Sciences (2018PT32032, 2018RC320014-2, and 3332019044).
Conflict of interest
The authors declare that the research was conducted in the absence of any commercial or financial relationships that could be construed as a potential conflict of interest.
Publisher's note
All claims expressed in this article are solely those of the authors and do not necessarily represent those of their affiliated organizations, or those of the publisher, the editors and the reviewers. Any product that may be evaluated in this article, or claim that may be made by its manufacturer, is not guaranteed or endorsed by the publisher.
Supplementary material
The Supplementary Material for this article can be found online at: https://www.frontiersin.org/articles/10.3389/fcvm.2022.965995/full#supplementary-material
References
1. Zimmet PZ, Magliano DJ, Herman WH, Shaw JE. Diabetes: a 21st century challenge. Lancet Diabetes Endocrinol. (2014) 2:56–64. doi: 10.1016/S2213-8587(13)70112-8
2. Zhang P, Zhang X, Brown J, Vistisen D, Sicree R, Shaw J, et al. Global healthcare expenditure on diabetes for 2010 and 2030. Diabetes Res Clin Pract. (2010) 87:293–301. doi: 10.1016/j.diabres.2010.01.026
3. Sarwar N, Gao P, Seshasai SR, Gobin R, Kaptoge S, Di Angelantonio E, et al. Diabetes mellitus, fasting blood glucose concentration, and risk of vascular disease: a collaborative meta-analysis of 102 prospective studies. Lancet. (2010) 375:2215–22. doi: 10.1016/S0140-6736(10)60484-9
4. Coutinho M, Gerstein HC, Wang Y, Yusuf S. The relationship between glucose and incident cardiovascular events. A metaregression analysis of published data from 20 studies of 95,783 individuals followed for 124 years. Diabetes Care. (1999) 22:233–40. doi: 10.2337/diacare.22.2.233
5. Lu W, Resnick HE, Jablonski KA, Jones KL, Jain AK, Howard WJ, et al. Non-HDL cholesterol as a predictor of cardiovascular disease in type 2 diabetes: the strong heart study. Diabetes Care. (2003) 26:16–23. doi: 10.2337/diacare.26.1.16
6. Costa J, Borges M, David C, Vaz Carneiro A. Efficacy of lipid lowering drug treatment for diabetic and non-diabetic patients: meta-analysis of randomised controlled trials. BMJ. (2006) 332:1115–24. doi: 10.1136/bmj.38793.468449.AE
7. Kearney PM, Blackwell L, Collins R, Keech A, Simes J, Peto R, et al. Efficacy of cholesterol-lowering therapy in 18,686 people with diabetes in 14 randomised trials of statins: a meta-analysis. Lancet. (2008) 371:117–25. doi: 10.1016/S0140-6736(08)60104-X
8. Detection E. And treatment of high blood cholesterol in adults (Adult Treatment Panel III). Jama. (2001) 285:2486–97. doi: 10.1001/jama.285.19.2486
9. Graham I, Atar D, Borch-Johnsen K, Boysen G, Burell G, Cifkova R, et al. European guidelines on cardiovascular disease prevention in clinical practice: executive summary: Fourth joint task force of the european society of cardiology and other societies on cardiovascular disease prevention in clinical practice (constituted by representatives of nine societies and by invited experts). Eur Heart J. (2007) 28:2375–414. doi: 10.1093/eurheartj/ehm316
10. Rydén L, Standl E, Bartnik M, Van den Berghe G, Betteridge J, de Boer MJ, et al. Guidelines on diabetes, pre-diabetes, and cardiovascular diseases: executive summary. The task force on diabetes and cardiovascular diseases of the European society of cardiology (ESC) and of the European association for the study of diabetes (EASD). Eur Heart J. (2007) 28:88–136. doi: 10.1093/eurheartjehl260
11. Kannel WB, McGee DL. Diabetes and glucose tolerance as risk factors for cardiovascular disease: the Framingham study. Diabetes Care. (1979) 2:120–6. doi: 10.2337/diacare.2.2.120
12. Haffner SM, Stern MP, Hazuda HP, Mitchell BD, Patterson JK. Cardiovascular risk factors in confirmed prediabetic individuals. Does the clock for coronary heart disease start ticking before the onset of clinical diabetes? Jama. (1990) 263:2893–8. doi: 10.1001/jama.1990.03440210043030
13. Tan CE, Chew LS, Chio LF, Tai ES, Lim HS, Lim SC, et al. Cardiovascular risk factors and LDL subfraction profile in type 2 diabetes mellitus subjects with good glycaemic control. Diabetes Res Clin Pract. (2001) 51:107–14. doi: 10.1016/S0168-8227(00)00211-4
14. Taskinen MR. Quantitative and qualitative lipoprotein abnormalities in diabetes mellitus. Diabetes. (1992) 41 Suppl 2:12–7. doi: 10.2337/diab.41.2.S12
15. Assmann G, Cullen P, Schulte H. Simple scoring scheme for calculating the risk of acute coronary events based on the 10-year follow-up of the prospective cardiovascular Münster (PROCAM) study. Circulation. (2002) 105:310–5. doi: 10.1161/hc0302.102575
16. Cromwell WC, Otvos JD, Keyes MJ, Pencina MJ, Sullivan L, Vasan RS, et al. LDL particle number and risk of future cardiovascular disease in the framingham offspring study—implications for LDL management. J Clin Lipidol. (2007) 1:583–92. doi: 10.1016/j.jacl.2007.10.001
17. Ai M, Otokozawa S, Asztalos BF, Ito Y, Nakajima K, White CC, et al. Small dense LDL cholesterol and coronary heart disease: results from the Framingham Offspring Study. Clin Chem. (2010) 56:967–76. doi: 10.1373/clinchem.2009.137489
18. Koba S, Hirano T, Ito Y, Tsunoda F, Yokota Y, Ban Y, et al. Significance of small dense low-density lipoprotein-cholesterol concentrations in relation to the severity of coronary heart diseases. Atherosclerosis. (2006) 189:206–14. doi: 10.1016/j.atherosclerosis.2005.12.002
19. Lawlor DA, Harbord RM, Sterne JA, Timpson N, Davey Smith G. Mendelian randomization: using genes as instruments for making causal inferences in epidemiology. Stat Med. (2008) 27:1133–63. doi: 10.1002/sim.3034
20. Xue A, Wu Y, Zhu Z, Zhang F, Kemper KE, Zheng Z, et al. Genome-wide association analyses identify 143 risk variants and putative regulatory mechanisms for type 2 diabetes. Nat Commun. (2018) 9:2941. doi: 10.1038/s41467-018-04951-w
21. Ahmad OS, Morris JA, Mujammami M, Forgetta V, Leong A, Li R, et al. A Mendelian randomization study of the effect of type-2 diabetes on coronary heart disease. Nat Commun. (2015) 6:7060. doi: 10.1038/ncomms8060
22. Larsson SC, Scott RA, Traylor M, Langenberg CC, Hindy G, Melander O, et al. Type 2 diabetes, glucose, insulin, BMI, and ischemic stroke subtypes: Mendelian randomization study. Neurology. (2017) 89:454–60. doi: 10.1212/WNL.0000000000004173
23. Ross S, Gerstein HC, Eikelboom J, Anand SS, Yusuf S, Paré G. Mendelian randomization analysis supports the causal role of dysglycaemia and diabetes in the risk of coronary artery disease. Eur Heart J. (2015) 36:1454–62. doi: 10.1093/eurheartj/ehv083
24. Willer CJ, Schmidt EM, Sengupta S, Peloso GM, Gustafsson S, Kanoni S, et al. Discovery and refinement of loci associated with lipid levels. Nat Genet. (2013) 45:1274–83. doi: 10.1038/ng.2797
25. Kettunen J, Demirkan A, Würtz P, Draisma HH, Haller T, Rawal R, et al. Genome-wide study for circulating metabolites identifies 62 loci and reveals novel systemic effects of LPA. Nat Commun. (2016) 7:11122. doi: 10.1038/ncomms11122
26. Prins BP, Kuchenbaecker KB, Bao Y, Smart M, Zabaneh D, Fatemifar G, et al. Genome-wide analysis of health-related biomarkers in the UK Household Longitudinal Study reveals novel associations. Sci Rep. (2017) 7:11008. doi: 10.1038/s41598-017-10812-1
27. Kanai M, Akiyama M, Takahashi A, Matoba N, Momozawa Y, Ikeda M, et al. Genetic analysis of quantitative traits in the Japanese population links cell types to complex human diseases. Nat Genet. (2018) 50:390–400. doi: 10.1038/s41588-018-0047-6
28. Burgess S, Thompson SG. Interpreting findings from Mendelian randomization using the MR-Egger method. Eur J Epidemiol. (2017) 32:377–89. doi: 10.1007/s10654-017-0255-x
29. Burgess S, Butterworth A, Thompson SG. Mendelian randomization analysis with multiple genetic variants using summarized data. Genet Epidemiol. (2013) 37:658–65. doi: 10.1002/gepi.21758
30. Burgess S, Thompson SG. Avoiding bias from weak instruments in Mendelian randomization studies. Int J Epidemiol. (2011) 40:755–64. doi: 10.1093/ije/dyr036
31. Bowden J, Davey Smith G, Haycock PC, Burgess S. Consistent estimation in Mendelian randomization with some invalid instruments using a weighted median estimator. Genet Epidemiol. (2016) 40:304–14. doi: 10.1002/gepi.21965
32. Bowden J, Davey Smith G, Burgess S. Mendelian randomization with invalid instruments: effect estimation and bias detection through Egger regression. Int J Epidemiol. (2015) 44:512–25. doi: 10.1093/ije/dyv080
33. Verbanck M, Chen CY, Neale B, Do R. Detection of widespread horizontal pleiotropy in causal relationships inferred from Mendelian randomization between complex traits and diseases. Nat Genet. (2018) 50:693–8. doi: 10.1038/s41588-018-0099-7
34. Monami M, Vitale V, Ambrosio ML, Bartoli N, Toffanello G, Ragghianti B, et al. Effects on lipid profile of dipeptidyl peptidase 4 inhibitors, pioglitazone, acarbose, and sulfonylureas: meta-analysis of placebo-controlled trials. Adv Ther. (2012) 29:736–46. doi: 10.1007/s12325-012-0045-5
35. Duvillard L, Pont F, Florentin E, Galland-Jos C, Gambert P, Vergès B. Metabolic abnormalities of apolipoprotein B-containing lipoproteins in non-insulin-dependent diabetes: a stable isotope kinetic study. Eur J Clin Invest. (2000) 30:685–94. doi: 10.1046/j.1365-2362.2000.00702.x
36. Taskinen MR, Nikkilä EA, Ollus A. Serum lipids and lipoproteins in insulin-dependent diabetic subjects during high-carbohydrate, high-fiber diet. Diabetes Care. (1983) 6:224–30. doi: 10.2337/diacare.6.3.224
37. BV H. Lipoprotein metabolism in diabetes mellitus. J Lipid Res. (1987) 28:613–28. doi: 10.1016/S0022-2275(20)38659-4
38. GM. R. Abnormal lipoprotein metabolism in non-insulin-dependent diabetes mellitus. Pathogenesis and treatment. Am J Med. (1987) 83:31-40. doi: 10.1016/0002-9343(87)90801-1
39. Garvey WT, Kwon S, Zheng D, Shaughnessy S, Wallace P, Hutto A, et al. Effects of insulin resistance and type 2 diabetes on lipoprotein subclass particle size and concentration determined by nuclear magnetic resonance. Diabetes. (2003) 52:453–62. doi: 10.2337/diabetes.52.2.453
40. Siegel RD, Cupples A, Schaefer EJ, Wilson PW. Lipoproteins, apolipoproteins, and low-density lipoprotein size among diabetics in the Framingham offspring study. Metabolism. (1996) 45:1267–72.
41. Festa A, Williams K, Hanley AJ, Otvos JD, Goff DC, Wagenknecht LE, et al. Nuclear magnetic resonance lipoprotein abnormalities in prediabetic subjects in the Insulin Resistance Atherosclerosis Study. Circulation. (2005) 111:3465–72. doi: 10.1161/CIRCULATIONAHA.104.512079
42. Goff DC, D'Agostino RB, Haffner SM, Otvos JD. Insulin resistance and adiposity influence lipoprotein size and subclass concentrations results from the insulin resistance atherosclerosis study. Metabolism. (2005) 54:264–70. doi: 10.1016/j.metabol.2004.09.002
43. Hodge AM, Jenkins AJ, English DR, O'Dea K, Giles GG. NMR-determined lipoprotein subclass profile predicts type 2 diabetes. Diabetes Res Clin Pract. (2009) 83:132–9. doi: 10.1016/j.diabres.2008.11.007
44. Mora S, Otvos JD, Rosenson RS, Pradhan A, Buring JE, Ridker PM. Lipoprotein particle size and concentration by nuclear magnetic resonance and incident type 2 diabetes in women. Diabetes. (2010) 59:1153–60. doi: 10.2337/db09-1114
45. Duran EK, Aday AW, Cook NR, Buring JE, Ridker PM, Pradhan AD. Triglyceride-rich lipoprotein cholesterol, small dense LDL cholesterol, and incident cardiovascular disease. J Am Coll Cardiol. (2020) 75:2122–35. doi: 10.1016/j.jacc.2020.02.059
46. Rizvi AA, Stoian AP, Janez A, Rizzo M. Lipoproteins and cardiovascular disease: an update on the clinical significance of atherogenic small, dense LDL and new therapeutical options. Biomedicines. (2021) 9:1579. doi: 10.3390/biomedicines9111579
47. Mora S, Otvos JD, Rifai N, Rosenson RS, Buring JE, Ridker PM. Lipoprotein particle profiles by nuclear magnetic resonance compared with standard lipids and apolipoproteins in predicting incident cardiovascular disease in women. Circulation. (2009) 119:931–9. doi: 10.1161/CIRCULATIONAHA.108.816181
48. Delgado-Velandia M, Gonzalez-Marrachelli V, Domingo-Relloso A, Galvez-Fernandez M, Grau-Perez M, Olmedo P, et al. Healthy lifestyle, metabolomics and incident type 2 diabetes in a population-based cohort from Spain. Int J Behav Nutr Phys Act. (2022) 19:8. doi: 10.1186/s12966-021-01219-3
49. Ekstedt M, Franzén LE, Mathiesen UL, Thorelius L, Holmqvist M, Bodemar G, et al. Long-term follow-up of patients with NAFLD and elevated liver enzymes. Hepatology. (2006) 44:865–73. doi: 10.1002/hep.21327
50. Siddiqui MS, Sterling RK, Luketic VA, Puri P, Stravitz RT, Bouneva I, et al. Association between high-normal levels of alanine aminotransferase and risk factors for atherogenesis. Gastroenterology. 2013;145:1271-9.e1–3. doi: 10.1053/j.gastro.2013.08.036
51. Siddiqui MS, Fuchs M, Idowu MO, Luketic VA, Boyett S, Sargeant C, et al. Severity of nonalcoholic fatty liver disease and progression to cirrhosis are associated with atherogenic lipoprotein profile. Clin Gastroenterol Hepatol. (2015) 13:1000-8.e3. doi: 10.1016/j.cgh.2014.10.008
52. Wang J, Zhao Q, Bowden J, Hemani G, Davey Smith G, Small DS, et al. Causal inference for heritable phenotypic risk factors using heterogeneous genetic instruments. PLoS Genet. (2021) 17:e1009575. doi: 10.1371/journal.pgen.1009575
53. Morrison J, Knoblauch N, Marcus JH, Stephens M, He X. Mendelian randomization accounting for correlated and uncorrelated pleiotropic effects using genome-wide summary statistics. Nat Genet. (2020) 52:740–7. doi: 10.1038/s41588-020-0631-4
54. Catapano AL, Graham I, De Backer G, Wiklund O, Chapman MJ, Drexel H, et al. 2016 ESC/EAS guidelines for the management of dyslipidaemias. Eur Heart J. (2016) 37:2999–3058. doi: 10.1093/eurheartj/ehw272
55. Ginsberg HN, Elam MB, Lovato LC, Crouse JR. 3rd, Leiter LA, Linz P, et al. Effects of combination lipid therapy in type 2 diabetes mellitus. New Eng J Med. (2010) 362:1563–74. doi: 10.1056/NEJMoa1001282
Keywords: type-2 diabetes, dyslipidemia, cardiovascular disease, Mendelian randomization, triglyceride, high-density lipoprotein (HDL)
Citation: Chen K, Zheng J, Shao C, Zhou Q, Yang J, Huang T and Tang Y-D (2022) Causal effects of genetically predicted type 2 diabetes mellitus on blood lipid profiles and concentration of particle-size-determined lipoprotein subclasses: A two-sample Mendelian randomization study. Front. Cardiovasc. Med. 9:965995. doi: 10.3389/fcvm.2022.965995
Received: 10 June 2022; Accepted: 20 September 2022;
Published: 13 October 2022.
Edited by:
Wei Liu, The University of Manchester, United KingdomReviewed by:
Giuseppe Mandraffino, University of Messina, ItalyJin Liu, Duke-NUS Medical School, Singapore
Copyright © 2022 Chen, Zheng, Shao, Zhou, Yang, Huang and Tang. This is an open-access article distributed under the terms of the Creative Commons Attribution License (CC BY). The use, distribution or reproduction in other forums is permitted, provided the original author(s) and the copyright owner(s) are credited and that the original publication in this journal is cited, in accordance with accepted academic practice. No use, distribution or reproduction is permitted which does not comply with these terms.
*Correspondence: Yi-Da Tang, dGFuZ3lpZGEmI3gwMDA0MDtiam11LmVkdS5jbg==
†These authors have contributed equally to this work and share first authorship