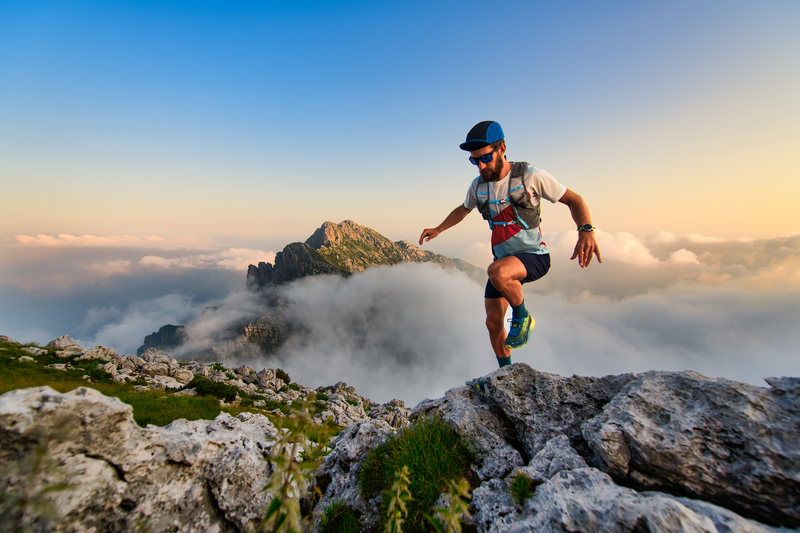
95% of researchers rate our articles as excellent or good
Learn more about the work of our research integrity team to safeguard the quality of each article we publish.
Find out more
ORIGINAL RESEARCH article
Front. Cardiovasc. Med. , 04 August 2022
Sec. General Cardiovascular Medicine
Volume 9 - 2022 | https://doi.org/10.3389/fcvm.2022.955838
This article is part of the Research Topic Abdominal Aortic Aneurysms: Advancements in diagnosis, biomarkers, drug therapeutics, surgical and endovascular treatment View all 21 articles
Background: Various studies have highlighted the role of circular RNAs (circRNAs) as critical molecular regulators in cardiovascular diseases, but its role in abdominal aortic aneurysm (AAA) is unclear. This study explores the potential molecular mechanisms of AAA based on the circRNA-microRNA (miRNA)-mRNA competing endogenous RNA (ceRNA) network and immune cell infiltration patterns.
Methods: The expression profiles of circRNAs (GSE144431) and mRNAs (GSE57691 and GSE47472) were obtained from the Gene Expression Omnibus (GEO). Then, the differentially expressed circRNAs (DEcircRNAs) and mRNAs (DEmRNAs) between AAA patients and healthy control samples, and the target miRNAs of these DEmRNAs and DEcircRNAs were identified. Based on the miRNA-DEmRNAs and miRNA-DEcircRNAs pairs, the ceRNA network was constructed. Furthermore, the proportion of the 22 immune cell types in AAA patients was assessed using cell type identification by estimating relative subsets of RNA transcripts (CIBERSORT) algorithm. The expressions of key genes and immune cell infiltration were validated using clinical specimens.
Results: A total of 214 DEmRNAs were identified in the GSE57691 and GSE47472 datasets, and 517 DEcircRNAs were identified in the GSE144431 dataset. The ceRNA network included 19 circRNAs, 36 mRNAs, and 68 miRNAs. Two key genes, PPARG and FOXO1, were identified among the hub genes of the established protein-protein interaction between mRNAs in the ceRNA network. Moreover, seven types of immune cells were differentially expressed between AAA patients and healthy control samples. Hub genes in ceRNA, such as FOXO1, HSPA8, and RAB5C, positively correlated with resting CD4 memory T cells or M1 macrophages, or both.
Conclusion: In conclusion, a ceRNA interaction axis was constructed. The composition of infiltrating immune cells was analyzed in the abdominal aorta of AAA patients and healthy control samples. This may help identify potential therapeutic targets for AAA.
Abdominal aortic aneurysm (AAA) is a progressive vascular accompanies the risk of rupturing the dilated aortic segment and is potentially lethal (1). However, the mechanism of AAA progression is unclear; hence it is essential to explore the underlying molecular causes of AAA that will help improve the diagnosis and treatment of AAA patients (2). Recent studies have shown the role of circular RNAs (circRNAs) in the pathogenesis of cardiovascular diseases (3, 4). circRNAs are a unique class of RNA molecules; they form a circular closed-loop structure by a covalent bond linkage by back-splicing linear RNA (5). It has been shown that circRNAs act as competitive endogenous RNA (ceRNA) and are involved in cardiovascular disease pathogenesis (6). In the ceRNA network, circRNAs compete with microRNAs (miRNAs) through miRNA response elements (MRE), thereby negatively regulating the mRNA expression of protein-coding genes (7). A unique circRNA-miRNA-mRNA interaction could be a potential mechanism for the development and progression of AAA.
The main pathological features of AAA include smooth muscle cell (SMC) dysfunction, inflammation, immune cell infiltration, and extracellular matrix remodeling (8). Studies have shown an association between circ-FNDC3B and angiotensin II (Ang II) induced SMC dysfunction, suggesting that circ-FNDC3B/miR-143-3p/ADAM10 axis may regulate AAA pathogenesis (9). Further investigation of circ-Sirt1/miR-132/212/SIRT1 in SMC phenotypic switching provided another perspective on the pathogenesis of AAA (10). Recently, Ma et al. showed the involvement of hsa_circ_0087352 in promoting the inflammatory response of macrophages in AAA. The target miRNAs and mRNAs were identified, and a ceRNA network of hsa_circ_0087352/ hsa-miR-149-5p/ IL-6 in AAA was constructed (11). Results suggest hsa_circ_0087352 promotes IL-6 transcription and secretion of inflammatory cytokine via endogenous hsa-miR-149-5p in macrophages thereby hsa_circ_0087352 could be potentially used in AAA therapeutics. These findings suggest that circRNA may have different targets and functions in other cells and tissues, and the circRNA-miRNA-mRNA network could play a significant role in AAA pathogenesis. Studies have proven the involvement of the infiltrating immune cells, such as neutrophils, macrophages, and T cells, in the occurrence and development of AAA (12). AAA is characterized by the infiltration of immune cells, suggesting that the immune system plays a critical role in AAA progression (13, 14). However, the ceRNA network and its association with infiltrating immune cells in AAA have not been thoroughly elucidated (15, 16).
This study aims to explore a novel circRNA-miRNA-mRNA ceRNA axis in AAA by analyzing microarray datasets from publicly available databases. Various patterns of immune cell infiltration in AAA were studied using the “cell type identification by estimating relative subsets of RNA transcripts (CIBERSORT)” algorithm. The co-expression patterns of immune cells and hub genes of the ceRNA network were also identified. Moreover, the target mRNAs of DEcircRNAs and immune infiltration were validated in healthy control samples and AAA patients. This study sheds light on the potential role of ceRNA in the pathogenesis of AAA and its underlying immune infiltration signature.
The microarray data of AAA were retrieved from the National Center for Biotechnology Information Gene Expression Omnibus (NCBI_GEO) database (https://www.ncbi.nlm.nih.gov/geo/). The species type as “Homo sapiens.” was set as a filter and the results obtained included three datasets GSE47472, GSE57691, and GSE144431. The data of circRNA expression (GSE144431) was ncRNA profiling by array, and the data of the microarray platform by 074301 Arraystar Human ncRNA microarray V2 (platform: GPL21825), the mRNA expression datasets (GSE47472 and GSE57691) by expression profiling by array, and the data of the microarray platform by Illumina HumanHT-12 V4.0 expression BeadChip (platform: GPL10558). Each dataset includes data from patients with AAA and normal aorta (which will be referred to as the healthy control group). Series matrix files and expressive data were retrieved from the GEO database.
The differentially expressed mRNAs (DEmRNAs) and circRNAs (DEcircRNAs) between the AAA patients and the healthy control group were analyzed and compared using the “Linear Models for Microarray Data (limma)” R package. mRNAs and circRNAs were considered as DEmRNAs and DEcircRNAs if they met the criteria: |log2 fold change (FC)| > 1 and false discovery rate (FDR) adjusted p-value < 0.05. The differential analysis results were presented as volcano plots and heat maps, and related tabular information was derived.
Kyoto Encyclopedia of Genes and Genomes (KEGG) enrichment analysis and Gene Ontology (GO) were used to explore the biological functions of DEmRNAs in AAA. The KEGG enrichment analysis and GO analysis included biological processes (BP), molecular functions (MF), and cellular components (CC), and the analysis was performed using the “clusterProfiler” package of the R software. FDR adjusted p-value < 0.05 was considered statistically significant.
The DEcircRNAs (GSE144431) and DEmRNAs (GSE57691 and GSE47472) between AAA patients and healthy control samples were identified, and the target miRNAs of the key DEmRNAs and DEcircRNAs were predicted. The DEmRNA-miRNA pairs were predicted using the target miRNA information from the databases like miRcode, miRDB, TargetScan, miRmap, and miRanda databases (17–21). The DEcircRNA-miRNA pairs were determined using the starBase database (http://starbase.sysu.edu.cn/index.php). DEcircRNA-miRNA pairs and DEmRNA-miRNA pairs were used to construct the ceRNA network and visualized using cytoHubba in Cytoscape 3.4.0 software.
The Search Tool for the Retrieval of Interacting Genes (STRING; version 11.0) was used to explore the protein-protein interactions between mRNAs in the ceRNA network (22). The PPI network of the DEmRNAs with combined score >0.4 in STRING was considered as a functional link. The PPI network was visualized by Cytoscape 3.4.0. The cytoHubba plugin of the Cytoscape software calculates the dense relationship through the degree, betweenness centrality, and closeness centrality algorithms. The hub genes of the PPI network were confirmed by cytoHubba.
The mRNA microarray dataset GSE57691 was analyzed to study the proportion of 22 infiltrating immune cells in the tissues between AAA patients and the healthy control samples using the CIBERSORT algorithm (23). The significantly different cell types (p < 0.05) between AAA patients and the healthy control samples were filtered out in the CIBERSORT analysis. Subsequently, the Wilcoxon rank-sum test was applied to assess differentially infiltrating immune cells between patients with AAA and controls, visualized by “heatmap” and “Violin plot” packages of the R software.
The study was approved by the Institutional Review Board of the Shanghai Jiaotong University School of Medicine, Renji Hospital (No. KY2021–168). All experiments were conducted in accordance with the Declaration of Helsinki. Written informed consent was obtained from all patients or their families before the collection of the biological specimens. The presence of an aortic aneurysm was confirmed prior to the echocardiography (ECG) or computed tomography (CT)/magnetic resonance imaging (MRI). The clinical diagnosis was established before surgery by expert clinicians. Healthy control aortic samples were obtained from organ donors.
The abdominal aortas were harvested and fixed using 4% paraformaldehyde (PFA), embedded in paraffin, and serial sections of five μm thickness were prepared on poly-l-lysine coated slides. The sections were dewaxed, rehydrated, and permeabilized using 0.1% Triton X-100. The sections were then blocked using 1% goat serum for 1 h at room temperature and further incubated with anti-rabbit CD68 antibody (1:200, ab213363, Abcam, MA, USA) and anti-rabbit CD86 antibody (1:200, ab239075, Abcam, USA) at 4°C overnight. The following day, slides containing sections were incubated with fluorescent-conjugated secondary antibodies diluted in blocking buffer for 1 h at room temperature and mounted using 4′,6-diamidino-2-phenylindole (DAPI, Vector, ZsBio, Beijing, China). Imaging was done using a confocal microscope (Leica-SP8, Wetzlar, Germany).
Total RNA from tissue samples was isolated using TRIzol (15596018, Invitrogen, Carlsbad, CA) per the manufacturer's instructions. Complementary DNA was synthesized using 500 ng of total RNA using the Reverse Transcription Kit (EZBioscience, MN, USA) per the manufacturer's instructions. qRT-PCR was performed using SYBR Green qPCR Master Mix (EZBioscience, MN, USA). Relative gene expression was calculated using the 2−ΔΔCT method. PCR was performed using three biological and technical replicates and normalized using the housekeeping gene glyceraldehyde 3-phosphate dehydrogenase. Primer sequences are listed in Supplementary Table S1.
The statistical analysis was carried out using Statistical Package for Social Sciences (SPSS, Chicago, Illinois) version 22.0. The data are expressed as the mean ± standard deviation or percentage. The variables between the two groups were compared using Student's t-test. Spearman's rank correlation analysis was performed to study the correlation between the hub genes of the ceRNA network and differentially expressed infiltrating immune cells. p < 0.05 was accepted as statistically significant.
The schematic diagram in Figure 1 illustrates the course of this study. A total of 1,135 upregulated mRNA and 2830 downregulated mRNAs were identified in the GSE47472 dataset. The GSE57691 had 2497 DEmRNAs, of which 247 mRNAs were upregulated, and 2250 mRNAs were downregulated. DEmRNAs were visualized by hierarchical clustering heatmap analysis (Figures 2A,B) and volcano plots analysis (Figures 2C,D). In the GSE14431 dataset, 225 upregulated and 292 downregulated circRNAs were visualized by hierarchical clustering heat map analysis (Figure 2E) and volcano plot analysis (Figure 2F). The most significant differentially expressed DEmRNAs and DEcircRNAs were shown in Tables 1, 2.
Figure 1. Flow diagram of the analytic process. The difference expression of three datasets (circRNA dataset and mRNA dataset) was analyzed. Construction of ceRNA network, protein-protein interaction network, functional enrichment analysis, co-expression analysis of essential genes and the immune infiltration, and validation of gene expression and immune infiltration.
Figure 2. DEmRNAs and DEcircRNAs in aortic tissues between patients with AAA and normal controls. (A) The heat map of GSE47472, mRNAs. (B) The heat map of GSE57691, mRNAs. (C) The volcano plot of GSE47472, mRNAs. (D) The volcano plot of GSE57691, mRNAs. (E) The heat map of GSE144431, circRNAs. (F) The volcano plot of GSE144431, circRNAs. mRNAs, messenger RNAs; circRNAs, circular RNAs; DEmRNAs, differentially expressed messenger RNAs; DEcircRNAs, differentially expressed circular RNAs.
The Venn diagram showed 214 overlapping DEmRNAs, of which 11 mRNAs were upregulated, and 203 mRNA were downregulated between GSE47472 and GSE57691 (Figure 3A). GO analysis of these DEmRNAs indicated that BP was significantly enriched in the circulatory system development, negative regulation of cell population proliferation, and response to insulin (Figure 3B; Supplementary Figure S1). The CC ontology cellular processes that were enriched were related to the intracellular membrane-bounded organelle, cytoplasmic ribonucleoprotein granule, and ribonucleoprotein granule (Figure 3C; Supplementary Figure S2). In the MF ontology, enriched processes included snRNA binding, double-stranded RNA binding, and ligand-binding domain (LBD) domain binding (Figure 3D; Supplementary Figure S3). Additional GO analysis was shown in (Supplementary Figure S4. Furthermore, KEGG pathway analysis revealed that these DEmRNAs are mainly involved in the spliceosome, non-alcoholic fatty liver disease (NAFLD), oxidative phosphorylation, and tumor necrosis factor (TNF) signaling pathway (Figure 3E; Supplementary Figure S5). GO and KEGG enrichment analyses are presented in Supplementary Tables S2, S3.
Figure 3. Venn diagram analysis and GO/KEGG analysis of 214 DEmRNAs coregulation modes in the two datasets (A) The intersection of downregulated and upregulated DEmRNAs (GSE57691 and GSE47472). (B) Biological process of 214 DEmRNAs by GO analysis. (C) Cellular component of 214 DEmRNAs by GO analysis. (D) Molecular function of 214 DEmRNAs by GO analysis. (E) KEGG enrichment analysis of DEmRNAs. GO, Gene Ontology; KEGG, Kyoto Encyclopedia of Genes and Genomes; DEmRNAs, differentially expressed messenger RNAs; BP, biological process; CC, cellular component; MF, molecular function.
The starBase predicted and identified 311 miRNA targets of the DEcircRNAs from the GSE144431 dataset. The interactions between 311 miRNAs and 214 DEmRNAs were analyzed using the miRcode, miRDB, TargetScan, miRmap, and miRanda. The circRNA-miRNA-mRNA ceRNA network was constructed based on DEcircRNA-miRNA and DEmRNA-miRNA pairs, including 68 miRNAs, 19 circRNAs, and 36 mRNAs (Figure 4).
Figure 4. The ceRNA network of circRNA-miRNA-mRNA. The round represents mRNAs, square shapes represent miRNAs, triangles represent circRNAs, and connection represents interaction. ceRNA, competing endogenous RNA; circRNAs, circular RNAs; miRNAs, microRNAs; mRNAs, messenger RNAs.
The PPI network was constructed using the STRING database based on the mRNAs from the ceRNA network. After excluding the genes that failed to interact, PPI network consisted of 25 nodes and 27 edges (Figure 5). Subsequently, nine hub genes were identified; among the genes that were upregulated were SOCS3 (suppressor of cytokine signaling 3) and PTGS2 (prostaglandin-endoperoxide synthase 2). The downregulated genes were NOTCH2 (notch receptor 2), RAB5C (RAB5C, member RAS oncogene family), HSP90AA1 (heat shock protein 90 alpha family class A member 1), HSPA8 [heat shock protein family A (Hsp70) member 8] and PIK3R1 (phosphoinositide-3-kinase regulatory subunit 1), PPARG (peroxisome proliferator-activated receptor gamma), and FOXO1 (forkhead box O1). Among them, two key genes were identified: PPARG and FOXO1.
Figure 5. The PPI network of mRNAs from ceRNA network. (A) The results of the STRING database showed the protein-protein interaction network. Yellow line, interactions form textmining; blue-green line, known interactions from curated databases; dark blue, predicted interactions form gene co-occurrence; black line, interactions form co-expression; purple line, known interactions from experimentally determined; light blue, interactions form protein homology. (B) Square shapes represent genes, and lines represent interaction relationships. PPI, protein-protein interaction; mRNAs, messenger RNAs; ceRNA, competing endogenous RNA.
The proportions and composition of 22 infiltrating immune cells were compared between the aortic tissues of AAA patients and healthy control samples by CIBERSORT algorithm (Figures 6A,B). The relationships between the abundance of 22 immune cells cells are shown in Figure 6C. The correlation analysis revealed a positive correlation between follicular helper T cells and naive B cells (R = 0.54) and a negative correlation between activated mast cells and resting mast cells (R = −0.53). Other immune cell subpopulations were weak to moderately correlated. Other immune cell subpopulations were weak to moderately correlated.
Figure 6. Composition of infiltrating immune cells in aortic tissues. (A) Distribution of immune cell types in each sample. (B) Heat map of infiltrating immune cells. (C) The correlation among infiltrating immune cells. (D) Violin plot of infiltrating immune cells.
The relative proportions of infiltrating immune cells between AAA patients and healthy control samples were analyzed by Wilcoxon rank-sum test. Compared with the controls, activated dendritic cells (p = 0.006), monocytes (p = 0.016), neutrophils (p = 0.009), activated CD4 memory T cells (p = 0.043), and regulatory T cells (p = 0.002) were significantly enriched in AAA patients (Figure 6D; Supplementary Figure S6). The M1 macrophages (p = 0.04) and resting CD4 memory T cells (p = 0.004) were significantly less enriched in AAA samples compared with the controls (Figure 6D; Supplementary Figure S6).
The correlation between hub genes of the ceRNA network and differentially expressed infiltrating immune cells was estimated using Spearman's rank correlation analysis (Figures 7A–F). The RAB5C gene was positively correlated with resting CD4 memory T cells and M1 macrophages (R = 0.55 and R = 0.54, respectively). HSPA8 gene was positively correlated with resting CD4 memory T cells (R = 0.60). The FOXO1 gene was positively correlated with resting CD4 memory T cells and M1 macrophages (R = 0.58 and R = 0.50, respectively). The correlation between hub genes (FOXO1, RAB5C, and HSPA8) and M1 macrophages was estimated using Spearman's rank correlation analysis (Figure 7G). The expression levels of M1 macrophages and hub genes are illustrated using the heatmap (Figure 7H).
Figure 7. Co-expression patterns of infiltrating immune cells and key genes. (A) The correlation between hub genes of ceRNA network and differentially expressed infiltrating immune cells. (B–F) The significantly correlated pairs with correlation coefficient > 0.5 and p < 0.001. (G) The correlation between key genes (FOXO1, RAB5C, and HSPA8) and differentially expressed infiltrating immune cells. (H) Heat map of key genes (FOXO1, RAB5C, and HSPA8) and differentially expressed infiltrating immune cells. mRNAs, messenger RNAs; ceRNA, competing endogenous RNA.
Resting CD4 memory T cells are important cell types of the immune system and play a crucial role in resistance to pathogens. They are the reservoir for human immunodeficiency virus-1, rendering unrealistic hopes of virus eradication with current antiretroviral regimens (24). Few studies show the involvement of resting CD4 memory T cells in biological processes related to AAA. M1 macrophages circulate in the peripheral blood and reside in specific tissues, thereby conferring protection by attacking foreign substances such as bacteria and engulfing them by phagocytosis. We validated the expression of the M1 macrophage marker in clinical specimens, i.e., abdominal aortas, from AAA patients and healthy control samples. The immunofluorescence staining of CD68 and CD86 showed the infiltrating M1 macrophages were downregulated in large AAA samples (abdominal aorta diameter >55 mm), upregulated in both small AAA samples (abdominal aorta diameter ≤ 55 mm) and ruptured AAA samples compared to the healthy aorta (Figure 8A).
Figure 8. The results of preliminary clinical specimen validation. (A) Immunofluorescence staining of CD68 (red), CD86 (green) and DAPI (blue) in tissue samples of patients with AAA and controls. Scale bar = 100 μm. *p < 0.05, **p < 0.01, Student's t-test. (B) The expression levels of RAB5C, HSPA8, FOXO1, PPARG genes in tissue samples of AAA vs. controls. n = 10 in each group, *p < 0.05, **p < 0.01, Student's t-test. (C) The expression levels of RAB5C, HSPA8, FOXO1, PPARG genes in tissue samples of non-rupture AAA vs. rupture AAA. n = 10 in each group, *p < 0.05, Student's t-test. (D) Correlation curves between the levels of M1 macrophages and key genes in tissue samples of patients with AAA and controls. Total n = 20. (E) Quantification of the relative expression levels of hsa_circ_0002722, hsa_circ_0001837, hsa_circ_0000941 in tissue samples of AAA vs. controls. n = 3 in each group, *p < 0.05, **p < 0.01, Student's t-test. (F) Quantification of the relative expression levels of miR-130a-3p, miR-130b-3p, miR-135a-5p, miR-135b-5p in tissue samples of AAA vs. controls. n = 3 in each group, *p < 0.05, **p < 0.01, Student's t-test.
The mRNA levels of FOXO1, RAB5C, HSPA8, and PPARG were significantly decreased in AAA than in healthy control samples (Figure 8B). Significantly lower levels of HSPA8 and higher levels of FOXO1 and RAB5C in ruptured AAA than in unruptured AAA samples were observed (Figure 8C). The correlation between hub genes (FOXO1, RAB5C, HSPA8, and PPARG) and M1 macrophages in the clinical specimens was estimated using Spearman's rank correlation analysis (Figure 8D). The M1 macrophages positively correlated with FOXO1 and RAB5C (R = 0.61 and R = 0.56, respectively). In addition, RNA expression of FOXO1 and PPARG in clinical specimens were detected using RT-qPCR, confirming that hsa_circ_0002722-miR-130a/b-3p-PPARG and hsa_circ_0001837/hsa_circ_0000941-miR-135a/b-5p-FOXO1 were associated with the pathogenesis of AAA (Figures 8E,F).
AAA is a chronic vascular disease with potentially fatal outcomes (25). Microarray-based screening methods helped identify new diagnostic and therapeutic targets. Evidence suggests the role of circRNAs in the pathogenesis of AAA (4). Unlike traditional linear RNA, circRNA has a unique covalent closed circular structure, which confers high stability and tolerance to exonuclease activity (26). According to the ceRNA hypothesis, circRNAs act as miRNA sponges, which affects and inhibits miRNA activity and alters the expression of miRNA target genes (27). To understand and explore the effect of circRNA on gene expression via miRNA, in this study, the ceRNA network was constructed, and immune infiltration in AAA was analyzed using bioinformatics tools.
We integrated mRNAs with the same regulated trend in the NCBI_GEO database (GSE47472 and GSE57691) and obtained 214 DEmRNAs. GO analysis revealed that these DEmRNAs were significantly enriched in the circulatory system development and negative regulation of cell population proliferation. These processes play a key role in the crucial lesion formation in vascular diseases like AAA. Consistent with the results from Batra et al., KEGG analysis revealed that the oxidative phosphorylation and TNF signaling pathway are closely related to AAA pathogenesis (28, 29). After screening out the DEcircRNAs in the NCBI_GEO database (GSE144431), we predicted the DEcircRNA-miRNA and miRNA-DEmRNA pairs that construct the ceRNA network. Hub genes play a central role in the biological mechanisms underlying the potential pathogenesis of AAAs. Two key genes, namely, PPARG and FOXO1, among hub genes in the PPI network were identified.
PPARG are transcription factors of the nuclear hormone receptor family that binds to peroxisome proliferators. On activation by a ligand, the nuclear receptor binds to DNA-specific PPARG response elements and regulates the transcription of its target genes (30). Studies in the Ang II-induced AAA mouse model reveal that PPARG upregulates the expression of anti-inflammatory cytokines such as IL-10, thereby slowing the process of AAA development and rupture (31). The activators of PPARG, such as rosiglitazone and pioglitazone, have been shown to alleviate the development and rupture of Ang II-induced aneurysms in mouse models (32–34). Besides, this study also found that PPARG may exert its impacts through hsa_circ_0002722-miR-130a/b-3p. The downregulation of PPARG in SMC increases pro- inflammatory factors such as MMP and OPN, thereby promoting SMC proliferation, migration, and vascular remodeling, which is associated with atherosclerosis (35, 36). Additionally, PPARG can also act via hsa_circ_0002722-miR-130a/b-3p. miR-130a promotes inflammation which accelerates disease progression in atherosclerosis by downregulating the expression of PPARG (37). Similarly, previous studies show upregulation of miR-130b, specifically in AAA tissue (38). Further reports suggest polymorphisms in PPARG are associated with the development and progression of AAA (39). Therefore, the changes in PPARG expression can alter the inflammatory response and SMC function, making it a potential therapeutic target for AAA.
FOXO1 is a transcription factor that acts as a master switch to regulate the apoptosis of multiple cell types, including cardiomyocytes, pulmonary artery SMC, and endothelial cells (40). FOXO1-mediated SMC apoptosis has been reported to regulate plaque instability in advanced atherosclerotic lesions, providing new ideas for AAA formation, as SMC apoptosis is a classic pathological feature of AAA (41). FOXO1 is also involved in cell migration, invasion, proliferation, and physiological processes such as inflammation and autophagy (42, 43). It has been shown to promote the migration, invasion, and inflammatory response of human umbilical vein endothelial cells, leading to the development and progression of a cerebral aneurysm (44). Hou et al. discovered that FOXO1 promoted the proliferation of SMC, which contributes to the progression of cerebral aneurysm and atherosclerosis (45, 46). Moreover, FOXO1-mediated cellular autophagy plays a vital role in a variety of diseases, such as liver steatosis, cancer, cerebral ischemia/reperfusion injury, diabetic kidney disease, and oxidative damage (47–51). Reports suggest metformin acts as an inhibitor of autophagy and reduces the Ang II-induced AAA in mouse models, suggesting that FOXO1 may regulate AAA pathogenesis (52). Further, results from this study reveal, FOXO1 may exert its impacts through hsa_circ_0001837/hsa_circ_0000941-miR-135a/b-5p. It has been shown that the expression of miR-135a/b was upregulated in AAAs, and the mechanism by which they function in AAA remains to be investigated (38). A recent study suggests that miR-135a-5p inhibits SMC proliferation and migration by inactivating FOXO1, which is consistent with our finding (53). Although FOXO1 has been extensively studied in cardiovascular diseases, the role of FOXO1 in AAA requires further investigation.
AAA is caused by multiple factors, such as immune cell infiltration, inflammation, and extracellular matrix remodeling (54). This study identified the proportion of infiltrating immune cells in aortic tissues with differentially expressed immune cells, including M1 macrophages. As M1 macrophage polarization is key in promoting AAA formation, it has been widely accepted to play a part in AAA pathogenesis (4). In the Ang II-induced AAA mouse model, the Chemokine C-C motif ligand contributes to the AAA development and pathogenesis by promoting the M1 polarization of macrophages (55). Furthermore, it has been noted that miR-144-5p is a novel regulator of AAA pathology, inhibiting the M1 polarization of macrophages (56). Our results show the downregulation of M1 macrophages in AAA patients samples compared with healthy control samples. The majority of AAAs in GSE57691 were large AAAs, showing downregulation of M1 macrophages compared to controls. To validate the expression of M1 macrophages in different stages of AAA, we used clinical samples for validation. Compared to the healthy aorta, the expression of the M1 macrophages was downregulated in large-diameter AAA aorta samples. However, it was upregulated in small diameter and ruptured AAA aorta samples. This may be because AAA is a dynamic vascular disease in which M1 macrophages are initially recruited to the injured aorta early in the pathogenesis to induce inflammation. The infiltration of M1 macrophages in AAAs is associated with the long-term effects of multiple factors (4). As the AAA progresses, M1 macrophages cause chronic inflammation, which prevents the repair of the injured aorta. In large aorta AAAs, the M2 polarization of macrophages reduces inflammation, contributing to wound healing, followed by the downregulation of M1 macrophages (57, 58). Our result demonstrates that the M1 polarization of macrophages may change over time in AAA formation, and the massive infiltration of M1 macrophages may be associated with AAA rupture.
To explore the potential regulatory mechanisms of genes in infiltration immune cells, we performed the correlation analysis between hub genes and differentially expressed immune cells in aortic tissues of patients with AAA. It has been shown that hub genes, such as FOXO1, RAB5C, and HSPA8, were positively correlated with M1 macrophages or resting CD4 memory T cells. The expression of FOXO1, RAB5C, and HSPA8 in aortic samples was downregulated in AAA compared to the healthy control samples Furthermore, HSPA8 levels were low, and FOXO1 and RAB5C were higher in ruptured AAA samples compared to unruptured AAA samples. Macrophage polarization plays an important role in various diseases, such as atherosclerosis, tissue inflammation, and abdominal aortic aneurysm. However, the role of resting CD4 memory T cells in AAA has not yet been reported. A previous study shows the involvement of FOXO1 in macrophage polarization; hence the overexpression of FOXO1 drives macrophages to M1 phenotypes (59). FOXO1-mediated M1 polarization has been demonstrated in gastric cancer, periodontal bone loss, and NAFLD and can be investigated as potential pathogenesis of AAA (60, 61). It is tempting to postulate that the correlation analysis provides new insights into the mechanisms of the immune system of AAA. Further in-depth investigation is required to establish concrete conclusions.
Some limitations include that the study was conducted on a small sample size, and the data were obtained from only three microarray datasets. However, it is important to note the challenges of obtaining AAA samples. Only 22 immune cells were included, which fails to incorporate the complexities of the immune microenvironment and requires further investigation. Finally, the underlying regulatory mechanisms of the ceRNA network and their relationship with immune cell infiltration were not elucidated, and other functional experiments are required.
In summary, PPARG, FOXO1, RAB5C, and HSPA8 likely play significant roles in AAA. Besides, M1 macrophages and resting CD4 memory T cells could be involved in AAA formation. A ceRNA network was established, hsa_circ_0002722-miR-130a/b-3p-PPARG and hsa_circ_0001837/hsa_circ_0000941-miR-135a/b-5p-FOXO1, may be associated with the pathogenesis of AAA. Although the expression of FOXO1, RAB5C, HSPA8, PPARG, and M1 macrophages were verified using clinical specimens, further studies are required to establish a correlation between DEmRNAs and differentially expressed infiltrating immune cells. This study enhances our understanding of the biological role of ceRNA and infiltrating immune cells in the pathogenesis of AAA. This may provide potential therapeutic insights for AAA, which requires further validation.
The datasets presented in this study can be found in online repositories. The names of the repository/repositories and accession number(s) can be found in the article/Supplementary material.
The studies involving human participants were reviewed and approved by Institutional Review Board of the Shanghai Jiaotong University School of Medicine, Renji Hospital. The patients/participants provided their written informed consent to participate in this study.
LC and SY draft the article and contribute to the conception, design, acquisition, and interpretation of data. LC, SW, ZW, YL, and YX contribute to acquisition and analysis of data. SY and GX revise the manuscript critically for important intellectual content and make final approval of the version to be published. All authors contributed to the article and approved the submitted version.
This work was supported by the National Natural Science Foundation of China (Grants No. 81873526).
The authors show great acknowledgments to all participants involved in the study.
The authors declare that the research was conducted in the absence of any commercial or financial relationships that could be construed as a potential conflict of interest.
All claims expressed in this article are solely those of the authors and do not necessarily represent those of their affiliated organizations, or those of the publisher, the editors and the reviewers. Any product that may be evaluated in this article, or claim that may be made by its manufacturer, is not guaranteed or endorsed by the publisher.
The Supplementary Material for this article can be found online at: https://www.frontiersin.org/articles/10.3389/fcvm.2022.955838/full#supplementary-material
1. Sakalihasan N, Limet R, Defawe OD. Abdominal aortic aneurysm. Lancet. (2005) 365:1577–89. doi: 10.1016/S0140-6736(05)66459-8
2. Golledge J, Muller J, Daugherty A, Norman P. Abdominal aortic aneurysm: pathogenesis and implications for management. Arterioscler Thromb Vasc Biol. (2006) 26:2605–13. doi: 10.1161/01.ATV.0000245819.32762.cb
3. Huang S, Li X, Zheng H, Si X, Li B, Wei G, et al. Loss of super-enhancer-regulated circrna nfix induces cardiac regeneration after myocardial infarction in adult mice. Circulation. (2019) 139:2857–76. doi: 10.1161/CIRCULATIONAHA.118.038361
4. Song H, Yang Y, Sun Y, Wei G, Zheng H, Chen Y, et al. Circular Rna Cdyl promotes abdominal aortic aneurysm formation by inducing M1 macrophage polarization and M1-Type inflammation. Mol Ther. (2022) 30:915–31. doi: 10.1016/j.ymthe.2021.09.017
5. Chen L, Wang C, Sun H, Wang J, Liang Y, Wang Y, et al. The bioinformatics toolbox for circrna discovery and analysis. Brief Bioinform. (2021) 22:1706–28. doi: 10.1093/bib/bbaa001
6. Gu X, Jiang YN, Wang WJ, Zhang J, Shang DS, Sun CB, et al. Comprehensive circrna expression profile and construction of circrna-related cerna network in cardiac fibrosis. Biomed Pharmacother. (2020) 125:109944. doi: 10.1016/j.biopha.2020.109944
7. Karreth FA, Pandolfi PP. Cerna cross-talk in cancer: when ce-bling rivalries go awry. Cancer Discov. (2013) 3:1113–21. doi: 10.1158/2159-8290.CD-13-0202
8. Quintana RA, Taylor WR. Cellular mechanisms of aortic aneurysm formation. Circ Res. (2019) 124:607–18. doi: 10.1161/CIRCRESAHA.118.313187
9. Liu Y, Zhong Z, Xiao L, Li W, Wang Z, Duan Z, et al. Identification of Circ-Fndc3b, an overexpressed circrna in abdominal aortic aneurysm, as a regulator of vascular smooth muscle cells. Int Heart J. (2021) 62:1387–98. doi: 10.1536/ihj.21-186
10. Kong P, Yu Y, Wang L, Dou YQ, Zhang XH, Cui Y, et al. Circ-Sirt1 controls Nf-kappab activation via sequence-specific interaction and enhancement of Sirt1 expression by binding to Mir-132/212 in vascular smooth muscle cells. Nucleic Acids Res. (2019) 47:3580–93. doi: 10.1093/nar/gkz141
11. Ma X, Xu J, Lu Q, Feng X, Liu J, Cui C, et al. Hsa_Circ_0087352 promotes the inflammatory response of macrophages in abdominal aortic aneurysm by adsorbing Hsa-Mir-149-5p. Int Immunopharmacol. (2022) 107:108691. doi: 10.1016/j.intimp.2022.108691
12. Li H, Bai S, Ao Q, Wang X, Tian X, Li X, et al. Modulation of immune-inflammatory responses in abdominal aortic aneurysm: emerging molecular targets. J Immunol Res. (2018) 2018:7213760. doi: 10.1155/2018/7213760
13. Horimatsu T, Blomkalns AL, Ogbi M, Moses M, Kim D, Patel S, et al. Niacin protects against abdominal aortic aneurysm formation via Gpr109a independent mechanisms: role of Nad+/Nicotinamide. Cardiovasc Res. (2020) 116:2226–38. doi: 10.1093/cvr/cvz303
14. Davis FM, Tsoi LC, Melvin WJ, denDekker A, Wasikowski R, Joshi AD, et al. Inhibition of macrophage histone demethylase Jmjd3 protects against abdominal aortic aneurysms. J Exp Med. (2021) 218:e20201839. doi: 10.1084/jem.20201839
15. Tian L, Hu X, He Y, Wu Z, Li D, Zhang H. Construction of Lncrna-Mirna-Mrna networks reveals functional lncrnas in abdominal aortic aneurysm. Exp Ther Med. (2018) 16:3978–86. doi: 10.3892/etm.2018.6690
16. Li T, Wang T, Yan L, Ma C. Identification of potential novel biomarkers for abdominal aortic aneurysm based on comprehensive analysis of Circrna-Mirna-Mrna networks. Exp Ther Med. (2021) 22:1468. doi: 10.3892/etm.2021.10903
17. Jeggari A, Marks DS, Larsson E. Mircode: A map of putative microrna target sites in the long non-coding transcriptome. Bioinformatics. (2012) 28:2062–3. doi: 10.1093/bioinformatics/bts344
18. Chen Y, Wang X. Mirdb: An online database for prediction of functional microrna targets. Nucleic Acids Res. (2020) 48:D127–D31. doi: 10.1093/nar/gkz757
19. Agarwal V, Bell GW, Nam JW, Bartel DP. Predicting effective microrna target sites in mammalian mrnas. Elife. (2015) 4:e05005. doi: 10.7554/eLife.05005
20. Vejnar CE, Zdobnov EM. Mirmap: comprehensive prediction of microrna target repression strength. Nucleic Acids Res. (2012) 40:11673–83. doi: 10.1093/nar/gks901
21. Enright AJ, John B, Gaul U, Tuschl T, Sander C, Marks DS. Microrna targets in drosophila. Genome Biol. (2003) 5:R1. doi: 10.1186/gb-2003-5-1-r1
22. Szklarczyk D, Gable AL, Lyon D, Junge A, Wyder S, Huerta-Cepas J, et al. String V11: Protein-protein association networks with increased coverage, supporting functional discovery in genome-wide experimental datasets. Nucleic Acids Res. (2019) 47:D607–D13. doi: 10.1093/nar/gky1131
23. Newman AM, Liu CL, Green MR, Gentles AJ, Feng W, Xu Y, et al. Robust enumeration of cell subsets from tissue expression profiles. Nat Methods. (2015) 12:453–7. doi: 10.1038/nmeth.3337
24. Pierson T, McArthur J, Siliciano RF. Reservoirs for Hiv-1: mechanisms for viral persistence in the presence of antiviral immune responses and antiretroviral therapy. Annu Rev Immunol. (2000) 18:665–708. doi: 10.1146/annurev.immunol.18.1.665
25. Nordon IM, Hinchliffe RJ, Loftus IM, Thompson MM. Pathophysiology and epidemiology of abdominal aortic aneurysms. Nat Rev Cardiol. (2011) 8:92–102. doi: 10.1038/nrcardio.2010.180
26. Cheng Z, Yu C, Cui S, Wang H, Jin H, Wang C, et al. Circtp63 functions as a cerna to promote lung squamous cell carcinoma progression by upregulating Foxm1. Nat Commun. (2019) 10:3200. doi: 10.1038/s41467-019-11162-4
27. Wang J, Zhao X, Wang Y, Ren F, Sun D, Yan Y, et al. Circrna-002178 act as a cerna to promote Pdl1/Pd1 expression in lung adenocarcinoma. Cell Death Dis. (2020) 11:32. doi: 10.1038/s41419-020-2230-9
28. Jeong SJ, Cho MJ, Ko NY, Kim S, Jung IH, Min JK, et al. Deficiency of peroxiredoxin 2 exacerbates angiotensin ii-induced abdominal aortic aneurysm. Exp Mol Med. (2020) 52:1587–601. doi: 10.1038/s12276-020-00498-3
29. Batra R, Suh MK, Carson JS, Dale MA, Meisinger TM, Fitzgerald M, et al. Il-1beta (Interleukin-1beta) and Tnf-Alpha (Tumor Necrosis Factor-Alpha) impact abdominal aortic aneurysm formation by differential effects on macrophage polarization. Arterioscler Thromb Vasc Biol. (2018) 38:457–63. doi: 10.1161/ATVBAHA.117.310333
30. Ma S, Zhou B, Yang Q, Pan Y, Yang W, Freedland SJ, et al. A transcriptional regulatory loop of master regulator transcription factors, pparg, and fatty acid synthesis promotes esophageal adenocarcinoma. Cancer Res. (2021) 81:1216–29. doi: 10.1158/0008-5472.CAN-20-0652
31. Wang WD, Sun R, Chen YX. Pparγ agonist rosiglitazone alters the temporal and spatial distribution of inflammation during abdominal aortic aneurysm formation. Mol Med Rep. (2018) 18:3421–8. doi: 10.3892/mmr.2018.9311
32. Golledge J, Cullen B, Rush C, Moran CS, Secomb E, Wood F, et al. Peroxisome proliferator-activated receptor ligands reduce aortic dilatation in a mouse model of aortic aneurysm. Atherosclerosis. (2010) 210:51–6. doi: 10.1016/j.atherosclerosis.2009.10.027
33. Jones A, Deb R, Torsney E, Howe F, Dunkley M, Gnaneswaran Y, et al. Rosiglitazone reduces the development and rupture of experimental aortic aneurysms. Circulation. (2009) 119:3125–32. doi: 10.1161/CIRCULATIONAHA.109.852467
34. Que Y, Shu X, Wang L, Wang S, Li S, Hu P, et al. Inactivation of Serca2 Cys(674) accelerates aortic aneurysms by suppressing Pparγ. Br J Pharmacol. (2021) 178:2305–23. doi: 10.1111/bph.15411
35. Meredith D, Panchatcharam M, Miriyala S, Tsai YS, Morris AJ, Maeda N, et al. Dominant-negative loss of ppargamma function enhances smooth muscle cell proliferation, migration, and vascular remodeling. Arterioscler Thromb Vasc Biol. (2009) 29:465–71. doi: 10.1161/ATVBAHA.109.184234
36. Halabi CM, Beyer AM, de Lange WJ, Keen HL, Baumbach GL, Faraci FM, et al. Interference with Ppar gamma function in smooth muscle causes vascular dysfunction and hypertension. Cell Metab. (2008) 7:215–26. doi: 10.1016/j.cmet.2007.12.008
37. Liu F, Liu Y, Du Y, Li Y. Mirna-130a promotes inflammation to accelerate atherosclerosis via the regulation of proliferator-activated receptor Γ (Pparγ) expression. Anatol J Cardiol. (2021) 25:630–7. doi: 10.5152/AnatolJCardiol.2021.56721
38. Kin K, Miyagawa S, Fukushima S, Shirakawa Y, Torikai K, Shimamura K, et al. Tissue- and plasma-specific microrna signatures for atherosclerotic abdominal aortic aneurysm. J Am Heart Assoc. (2012) 1:e000745. doi: 10.1161/JAHA.112.000745
39. Moran CS, Clancy P, Biros E, Blanco-Martin B, McCaskie P, Palmer LJ, et al. Association of Ppargamma allelic variation, osteoprotegerin and abdominal aortic aneurysm. Clin Endocrinol (Oxf). (2010) 72:128–32. doi: 10.1111/j.1365-2265.2009.03615.x
40. Alikhani M, Alikhani Z, Graves DT. Foxo1 functions as a master switch that regulates gene expression necessary for tumor necrosis factor-induced fibroblast apoptosis. J Biol Chem. (2005) 280:12096–102. doi: 10.1074/jbc.M412171200
41. Jia G, Aggarwal A, Tyndall SH, Agrawal DK. Tumor necrosis factor-alpha regulates p27 kip expression and apoptosis in smooth muscle cells of human carotid plaques via forkhead transcription factor O1. Exp Mol Pathol. (2011) 90:1–8. doi: 10.1016/j.yexmp.2010.11.001
42. Stöhr R, Kappel BA, Carnevale D, Cavalera M, Mavilio M, Arisi I, et al. Timp3 interplays with apelin to regulate cardiovascular metabolism in hypercholesterolemic mice. Mol Metab. (2015) 4:741–52. doi: 10.1016/j.molmet.2015.07.007
43. Malik S, Sadhu S, Elesela S, Pandey RP, Chawla AS, Sharma D, et al. Transcription factor Foxo1 Is essential for Il-9 induction in T helper cells. Nat Commun. (2017) 8:815. doi: 10.1038/s41467-017-00674-6
44. Wang RK, Sun YY Li GY, Yang HT, Liu XJ Li KF, et al. Microrna-124-5p delays the progression of cerebral aneurysm by regulating foxo1. Exp Ther Med. (2021) 22:1172. doi: 10.3892/etm.2021.10606
45. Hou WZ, Chen XL, Wu W, Hang CH. Microrna-370-3p inhibits human vascular smooth muscle cell proliferation via targeting Kdr/Akt signaling pathway in cerebral aneurysm. Eur Rev Med Pharmacol Sci. (2017) 21:1080–7.
46. Li X, Li L, Dong X, Ding J, Ma H, Han W. Circ_Grn promotes the proliferation, migration, and inflammation of vascular smooth muscle cells in atherosclerosis through Mir-214-3p/Foxo1 axis. J Cardiovasc Pharmacol. (2021) 77:470–9. doi: 10.1097/FJC.0000000000000982
47. Zhang J, Ng S, Wang J, Zhou J, Tan SH, Yang N, et al. Histone deacetylase inhibitors induce autophagy through Foxo1-dependent pathways. Autophagy. (2015) 11:629–42. doi: 10.1080/15548627.2015.1023981
48. Zhang L, Zhang Z, Li C, Zhu T, Gao J, Zhou H, et al. S100a11 promotes liver steatosis via foxo1-mediated autophagy and lipogenesis. Cell Mol Gastroenterol Hepatol. (2021) 11:697–724. doi: 10.1016/j.jcmgh.2020.10.006
49. Mei ZG, Huang YG, Feng ZT, Luo YN, Yang SB, Du LP, et al. Electroacupuncture ameliorates cerebral ischemia/reperfusion injury by suppressing autophagy via the Sirt1-Foxo1 signaling pathway. Aging. (2020) 12:13187–205. doi: 10.18632/aging.103420
50. Ren H, Shao Y, Wu C, Ma X, Lv C, Wang Q. Metformin alleviates oxidative stress and enhances autophagy in diabetic kidney disease via Ampk/Sirt1-Foxo1 pathway. Mol Cell Endocrinol. (2020) 500:110628. doi: 10.1016/j.mce.2019.110628
51. Shen M, Cao Y, Jiang Y, Wei Y, Liu H. Melatonin protects mouse granulosa cells against oxidative damage by inhibiting foxo1-mediated autophagy: implication of an antioxidation-independent mechanism. Redox Biol. (2018) 18:138–57. doi: 10.1016/j.redox.2018.07.004
52. Wang Z, Guo J, Han X, Xue M, Wang W, Mi L, et al. Metformin represses the pathophysiology of Aaa by suppressing the activation of Pi3k/Akt/Mtor/Autophagy pathway in Apoe(-/-) Mice. Cell Biosci. (2019) 9:68. doi: 10.1186/s13578-019-0332-9
53. Li D, An Y. Mir-135a-5p inhibits vascular smooth muscle cells proliferation and migration by inactivating foxo1 and Jak2 signaling pathway. Pathol Res Pract. (2021) 224:153091. doi: 10.1016/j.prp.2020.153091
54. Yang H, Zhou T, Stranz A, DeRoo E, Liu B. Single-cell Rna sequencing reveals heterogeneity of vascular cells in early stage murine abdominal aortic aneurysm-brief report. Arterioscler Thromb Vasc Biol. (2021) 41:1158–66. doi: 10.1161/ATVBAHA.120.315607
55. Xie C, Ye F, Zhang N, Huang Y, Pan Y, Xie X. Ccl7 contributes to angiotensin ii-induced abdominal aortic aneurysm by promoting macrophage infiltration and pro-inflammatory phenotype. J Cell Mol Med. (2021) 25:7280–93. doi: 10.1111/jcmm.16757
56. Shi X, Ma W, Li Y, Wang H, Pan S, Tian Y, et al. Mir-144-5p limits experimental abdominal aortic aneurysm formation by mitigating m1 macrophage-associated inflammation: suppression of Tlr2 and Olr1. J Mol Cell Cardiol. (2020) 143:1–14. doi: 10.1016/j.yjmcc.2020.04.008
57. Pope NH, Salmon M, Davis JP, Chatterjee A, Su G, Conte MS, et al. D-series resolvins inhibit murine abdominal aortic aneurysm formation and increase M2 macrophage polarization. FASEB J. (2016) 30:4192–201. doi: 10.1096/fj.201600144RR
58. Dale MA, Xiong W, Carson JS, Suh MK, Karpisek AD, Meisinger TM, et al. Elastin-derived peptides promote abdominal aortic aneurysm formation by modulating M1/M2 macrophage polarization. J Immunol. (2016) 196:4536–43. doi: 10.4049/jimmunol.1502454
59. Liu XL, Pan Q, Cao HX, Xin FZ, Zhao ZH, Yang RX, et al. Lipotoxic hepatocyte-derived exosomal microrna 192-5p activates macrophages through Rictor/Akt/Forkhead box transcription factor O1 signaling in nonalcoholic fatty liver disease. Hepatology. (2020) 72:454–69. doi: 10.1002/hep.31050
60. Ren J, Han X, Lohner H, Liang R, Liang S, Wang H. Serum- and glucocorticoid-inducible kinase 1 promotes alternative macrophage polarization and restrains inflammation through Foxo1 and Stat3 signaling. J Immunol. (2021) 207:268–80. doi: 10.4049/jimmunol.2001455
Keywords: abdominal aortic aneurysm, circular RNA, competing endogenous RNA network, immune cell infiltration, bioinformatic analysis
Citation: Chen L, Wang S, Wang Z, Liu Y, Xu Y, Yang S and Xue G (2022) Construction and analysis of competing endogenous RNA network and patterns of immune infiltration in abdominal aortic aneurysm. Front. Cardiovasc. Med. 9:955838. doi: 10.3389/fcvm.2022.955838
Received: 29 May 2022; Accepted: 12 July 2022;
Published: 04 August 2022.
Edited by:
Zhenjie Liu, The Second Affiliated Hospital of Zhejiang University School of Medicine, ChinaReviewed by:
Tao Han, Northern Theater General Hospital, ChinaCopyright © 2022 Chen, Wang, Wang, Liu, Xu, Yang and Xue. This is an open-access article distributed under the terms of the Creative Commons Attribution License (CC BY). The use, distribution or reproduction in other forums is permitted, provided the original author(s) and the copyright owner(s) are credited and that the original publication in this journal is cited, in accordance with accepted academic practice. No use, distribution or reproduction is permitted which does not comply with these terms.
*Correspondence: Shuofei Yang, ZG9jdG9yX3lhbmdzaHVvZmVpQDE2My5jb20=; Guanhua Xue, Z3Vhbmh1YXh1ZTIwMThAMTYzLmNvbQ==
Disclaimer: All claims expressed in this article are solely those of the authors and do not necessarily represent those of their affiliated organizations, or those of the publisher, the editors and the reviewers. Any product that may be evaluated in this article or claim that may be made by its manufacturer is not guaranteed or endorsed by the publisher.
Research integrity at Frontiers
Learn more about the work of our research integrity team to safeguard the quality of each article we publish.