Corrigendum: Analysis of time series gene expression and DNA methylation reveals the molecular features of myocardial infarction progression
- 1Shanghai Key Laboratory of Molecular Imaging, Zhoupu Hospital, Shanghai University of Medicine and Health Sciences, Shanghai, China
- 2School of Medical Instrument and Food Engineering, University of Shanghai for Science and Technology, Shanghai, China
- 3School of Nursing, Shanghai University of Traditional Chinese Medicine, Shanghai, China
- 4School of Basic Medical Sciences, Fudan University, Shanghai, China
- 5Seventh People's Hospital of Shanghai University of Traditional Chinese Medicine, Shanghai, China
- 6School of Pharmacy, Shanghai University of Medicine & Health Sciences, Shanghai, China
Myocardial infarction (MI) is one of the deadliest diseases in the world, and the changes at the molecular level after MI and the DNA methylation features are not clear. Understanding the molecular characteristics of the early stages of MI is of significance for the treatment of the disease. In this study, RNA-seq and MeDIP-seq were performed on heart tissue from mouse models at multiple time points (0 h, 10 min, 1, 6, 24, and 72 h) to explore genetic and epigenetic features that influence MI progression. Analysis based on a single point in time, the number of differentially expressed genes (DEGs) and differentially methylated regions (DMRs) increased with the time of myocardial infarction, using 0 h as a control group. Moreover, within 10 min of MI onset, the cells are mainly in immune response, and as the duration of MI increases, apoptosis begins to occur. Analysis based on time series data, the expression of 1012 genes was specifically downregulated, and these genes were associated with energy metabolism. The expression of 5806 genes was specifically upregulated, and these genes were associated with immune regulation, inflammation and apoptosis. Fourteen transcription factors were identified in the genes involved in apoptosis and inflammation, which may be potential drug targets. Analysis based on MeDIP-seq combined with RNA-seq methodology, focused on methylation at the promoter region. GO revealed that the downregulated genes with hypermethylation at 72 h were enriched in biological processes such as cardiac muscle contraction. In addition, the upregulated genes with hypomethylation at 72 h were enriched in biological processes, such as cell-cell adhesion, regulation of the apoptotic signaling pathway and regulation of angiogenesis. Among these genes, the Tnni3 gene was also present in the downregulated model. Hypermethylation of Tnni3 at 72 h after MI may be an important cause of exacerbation of MI.
Introduction
Myocardial infarction (MI) is a common cardiovascular disease worldwide. MI is caused mainly by the rupture of an atherosclerotic plaque leading to thrombus formation within the lumen of a coronary vessel, which in turn blocks blood flow to the distal myocardium (1), and the heart muscle is damaged by a lack of oxygen (2). Infarction leads to cardiac myocyte death and subsequent necrosis of the tissue in the infarcted area, causing inflammatory cells that phagocytose dead cells and debris within the infarcted area (3). The symptoms of MI are chest pain, which travels to the left arm or left side of the neck, shortness of breath, sweating, nausea, vomiting, abnormal heartbeat, anxiety, fatigue, and other phenomena (4). More than 7 million people worldwide experience a heart attack each year, and although the death rate from heart attacks has declined in recent years, it is still in the 10% range (5–7). MI is characterized by insignificant early symptoms, sudden onset and high mortality, and it is difficult to prevent, diagnose and treat this disease. Therefore, ideal biomarkers capable of rapid diagnosis and effective regulation are still needed in clinical practice.
MI occurs when myocardial cells are irreversibly damaged and necrotic due to hypoxia and decreased ATP supply (8). Oxidative stress, the inflammatory response and MI pathological processes have been confirmed by numerous studies to be closely related (9, 10). (ROS) generation activates the p53 and NF-κB signaling pathways, leading to upregulation of proinflammatory cytokine expression and impairment of glucose metabolism (11). Under pressure load, the expression of p53 increases, and the expression of ICAM1 increases, which lead to the inflammatory response of the heart, myocardial injury and systolic dysfunction. Moreover, in the inflammatory response after MI, IL-17A induces myocardial cell apoptosis mainly through the p38-p53-Bax signaling pathway, thus promoting ventricular remodeling and leading to heart failure (12).
With the development of sequencing technologies, transcriptomic changes in myocardial tissue have been studied through animal models at different times after MI (13, 14). However, single layer-omics data have limited ability to reveal complex molecular interactions between different layers. DNA methylation is a widely studied epigenetic modification that plays an essential role in regulating gene expression. A study by Agha et al. found that DNA methylation in blood was associated with coronary heart disease and MI (15). The experiment by Yousuf et al. (16) demonstrated that the hypermethylated state of the ABO gene promoter was associated with MI. Currently, only a few studies have focused on the relationship between DNA methylation and MI, and little is known about the modification and function of DNA methylation in myocardial tissue in early MI.
In this study, RNA sequencing (RNA-Seq) and Methylated DNA immunoprecipitation sequencing (MeDIP-Seq) were used to determine transcriptome profiles and DNA methylation of myocardial cells from mice at six time points after MI. We intend to study the characteristics of gene expression at different time points after MI and the genes with specific expression trends at different time points. First, transcriptome data at 0 h served as the control group, and differentially expressed genes (DEGs) at 10 min, 1, 6, 24, and 72 h were obtained. Subsequently, 0, 1, and 72 h expression data were selected for Short Time-series Expression Miner (STEM) analysis, and time-dependent gene expression profiles with statistical significance were screened, and transcription factors in the module were found. The TRRUST database was used to predict the target genes of transcription factors. The DrugBank database was used to identify drug targets among transcription factors. The methylation data were combined with the transcriptome data and the methylation of gene promoters at each time point was analyzed. The results can help us better understand the occurrence and development of disease after MI, and will provide new insights into the mechanism of MI at the epigenetic level.
Materials and Methods
Animal
Eighteen 12-week-old male C57BL/6JR mice were included in the experiment. The experiment was divided into 6 groups. Each group contained 3 mice, 5 groups of which required surgery to make models, called the operation group, and the other group was the sham operation group (0 h). The operation group was divided into 10 min, 1, 6, 24, and 72 h subgroups after MI. The mice were anesthetized with sodium pentobarbital (50 mg/kg, intraperitoneally) and placed on the operating table. The mice in the surgery group were intubated, and the left anterior descending coronary artery was ligated to induce MI, separately. The mice in the control group received the same operation, sutured through the inferior artery, but not ligated (17). Complete anesthesia of mice with 1–3% isoflurane after specific time of MI, followed by euthanasia by cervical dislocation. Total RNA was isolated from myocardial region of infarcted left ventricle.
The Acquisition of RNA-Seq and MeDIP-Seq Data
First, total RNA was extracted from the samples and the quality of the RNA was tested. Then, mRNA was purified from the total RNA using oligo dT magnetic beads. Fragmentation of mRNA was performed under high temperature conditions using divalent cations in NEBNext First Strand Synthesis Reaction Buffer (5X). Synthesis of first-strand cDNA using random hexamer primer and M-MuLV Reverse Transcriptase (RNase H) as the template for mRNA. Second strand cDNA synthesis was carried out with DNA polymerase I and RNase H. The remaining overhangs were transformed to blunt-ended by exonuclease/polymerase activity. The 3′ end of the DNA fragment was adenylated and then the NEBNext adapters with a hairpin loop structure were attached in preparation for hybridization. Library fragments were purified using the Ampoule XP system (Beverly Beckman Coulter, USA) to prioritize cDNA fragments of 250–300 bp in length. The cDNA was ligated using 3 μl of USER Enzyme (NEB, USA) for 15 min at 37°C using an adapter of the selected size, then used for 5 min at 95°C, followed by PCR. PCR was then conducted using Phusion High Fidelity DNA polymerase, universal PCR primers and index (X) Primer. Next, the PCR products were purified (Ampoule XP system) and the library quality was evaluated on the Agilent Bioanalyzer 2100 system. Finally, the library preparation was sequenced on the Illumina Novaseq platform and 150 bp paired-end reads were generated.
Genome-wide DNA methylation sequencing was performed using MeDIP-Seq, which was carried out by Shanghai Bohol Biotech, China. To summarize, the genomic DNA was sheared to a peak of 250bp fragments using the Covaris S2 ultrasound system. Next, the end repair and adenylation of the 3' end processes were carried out. After the connector ligation reaction, fragment selection was performed using beads. Then, immunoprecipitation of the methylated DNA was performed and the eluted DNA was amplified by PCR to generate the final sequencing library. The Agilent Bioanalyzer 2100 was used to purify the PCR products and assess the library quality. Finally, the illumina Novoseq 6000 was used to perform paired-end sequencing.
RNA-Seq Data Analysis
The cutadapt (V1.18) (18) was used to remove adapter sequences, low quality bases, and read parameters of <50 bases “-a AGATCGGAAGAGC-a AGATCGGAAGC-trim-n-m 50-q 20,20.” The clean data were mapped to the mm10 reference genome using Hisat2 (v2.1.0) (19) to obtain BAM files. The featureCounts software (20) was used to obtain the number of reads of genes from the BAM files in each sample and the fragments per kilobase million (FPKM) values in genes was calculated by R software. The annotated information of lncRNA was downloaded from GENECODE (https://www.gencodegenes.org/), lncRNAs were removed from the obtained expression data, and the remaining mRNAs were used for subsequent analysis. The differentially expressed genes between each sample group (10 min, 1, 6, 24, and 72 h) and the control group(0h) were calculated using the DESeq2 package in R software (version 4.1.1). The threshold was set as a p-value < 0.05 and a | log2FC| >1.
Gene Set Enrichment Analysis
To identify the biological signaling pathways involved in gene expression at different time points, normalized gene expression data obtained from five different time groups were used to perform GSEA analysis against controls for MI using the Gene Set Enrichment Analysis (GSEA) tool (v4.1) (21, 22), respectively. A subset of C2 (Mm.c2.cp.kegg.v7.1.entrez.rds) from mice was downloaded from the database (https://bioinf.wehi.edu.au/software/MSigDB/) as the reference gene set for GSEA analysis. In each analysis, 1000 permutations of the genome were performed to identify significantly different pathways. The normalized enrichment score (NES) indicates the extent to which the genome is over-represented at the top or bottom of the gene ranking list in the expression dataset. The p-value < 0.05 was set as the cut-off criteria. The GSEA analysis results were visualized using the ggbarplot function in ggpubr package.
Trend Analysis and Function Annotation
To obtain the obvious variation trend in gene expression, the gene expression data at 0, 1, and 72 h were analyzed using Short Time-series Expression Miner (STEM) software (23). The maximum number of model profiles was set to 8, and the maximum unit change in the model profiles between time points was set to 1. Gene expression values were transformed to log ratios relative to the expression value at 0 h. The parameter maximum–minimum was set as 1. If the maximum change in the converted expression value between the three time points was <1, the gene was filtered out. The algorithm assigned each gene to the best matched expression profile model on the basis of the correlation coefficient. The boxes in the figures were colored if the profiles were statistically significant and boxes of the same color with high similarity could be analyzed together.
Gene Ontology (GO) and kyoto encyclopedia of genes and genomes (KEGG) pathway analyses of significant profiles were performed using the clusterProfiler package in R software. A p-value < 0.05 was considered statistically significant.
Mining Transcription Factors, Drug Target Prediction
In this section, we focused on the genes from profile #4 and profile #7 enriched in apoptosis and inflammation related pathways. The transcription factor database (TRRUST, https://www.grnpedia.org/trrust/) contains 6,552 pieces of reported regulatory information on TF-targets in humans and mice were used to identify transcription factors in these genes. Drug target prediction using the DrugBank database (https://go.drugbank.com/targets).
MeDIP-Seq Data Analysis
The reads with low overall quality, containing sequencing primers, and with low end quality were filtered using FASTX (http://hannonlab.cshl.edu/fastx_toolkit/index.html). The reads from each MeDIP sample were mapped to the mouse (mm10) genome using Bowtie2 (24) and default parameters to obtain BAM files. We apply MACS2 (25) to the bam file obtained in the above step to find the peak enrichment region and obtain the enrichment region of the sample peak. Then, annotation of the peaks was performed. The distribution of the peaks on the chromosomes (promoter, 5'UTR, CDS, 3'UTR, Intron and TTR, where promoter is 2000 bp upstream of the transcription start site and TTR is 5000 bp downstream of the transcription termination site) was obtained according to the information on the location of the peaks. On CpG Island, the CpG shore is located 2000 bp downstream of CpG Island and the CpG shelf is located within 2000 bp to 4000 bp downstream of CpG Island. Differential analysis was performed on the peaks of the control and other MI groups using the R package MEDIPS (26) with parameters set to |log2FC| >1 and p-value < 0.05. Differentially methylated regions (DMRs) were annotated using the same procedure as for peak annotation above.
Comprehensive Analysis of Methylation and Transcriptome Data
MeDIP-Seq and RNA-Seq data were interpreted in an integrated way to identify epigenetically regulated genes that affect the progression of MI. We calculated the intersection of DMRs and DEGs as differentially methylated and expressed genes (DMEGs), and classified them into four distinct groups: HypoUp, HypoDown, HyperUp, and HyperDown. A fold change > 2 and p-value < 0.05 were used as filtering criteria. We focused on DMEGs in the promoter region. Due to the large number of DMEGs in the 72 h promoter region, we focused on genes with downregulated hypermethylation and upregulated hypomethylation, and conducted GO enrichment analysis by using the clusterProfiler package in R software.
Results
Transcriptome Changes of DEGs
Figure 1 shows the flow diagram for study enrolment. Unsupervised hierarchical clustering of the cardiac transcriptome indicated that mRNA expression signature distinguished 6 different time points of MI (Figure 2A). DESeq2 packages were used to identify differentially expressed genes at each MI time point compared with the control group (0 h). There were 151, 203, 1570, 2174 and 5513 DEGs (|log2FC | > 1, p-value < 0.05) in the 10 m vs. 0 h, 1 vs. 0 h, 6 vs. 0 h, 24 vs. 0 h and 72 vs. 0 h (Figures 2B–F). The upregulation and downregulation of DEGs at each time point are shown in Supplementary Figure 1.
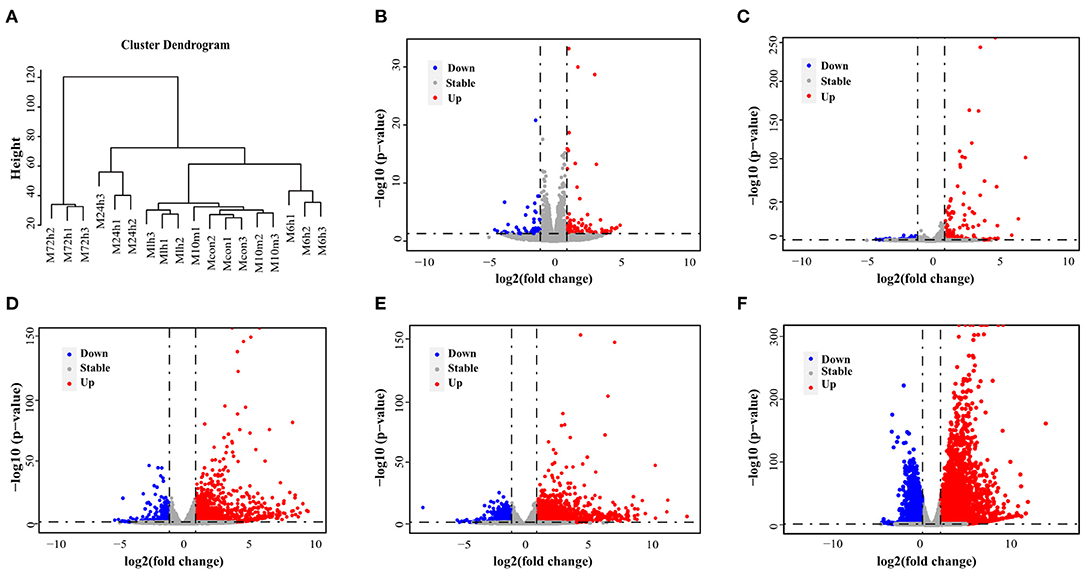
Figure 2. DEGs in mouse model of MI derived by RNA-Seq. (A) An unsupervised cluster analysis of MI at 6 different time points. (B–F) Volcano plot showing DEGs in 10 min, 1, 6, 24, and 72 h post-MI. Red dots indicate significantly upregulated mRNAs, and blue dots indicate downregulated mRNAs.
GSEA Identifies Key Pathways in the Progression of MI
To identify the signaling pathways involved in the progression of MI (10 min, 1, 6, 24, and 72 h) over the control group, GSEA was performed on the whole set of expressed genes and ranked by their differential expression at each MI time point vs. the control group. Figures 3A–E displays the results of GSEA for each time point with KEGG gene sets from MSigDB using the GSEA tool. The results show that inflammatory and immune signaling pathways (e.g., B cell receptor signaling pathway, T cell receptor signaling pathway, chemokine signaling pathway) were among the top overrepresented pathways in the 10 min post MI and were continuously activated until 24 h post MI. Apoptosis signaling, such as the p53 signaling pathway, ECM receptor interaction, and apoptosis, was also significantly activated at different time points after MI. Oxidative phosphorylation in downregulated gene-enriched pathways lasted from 10 min after MI to 72 h.
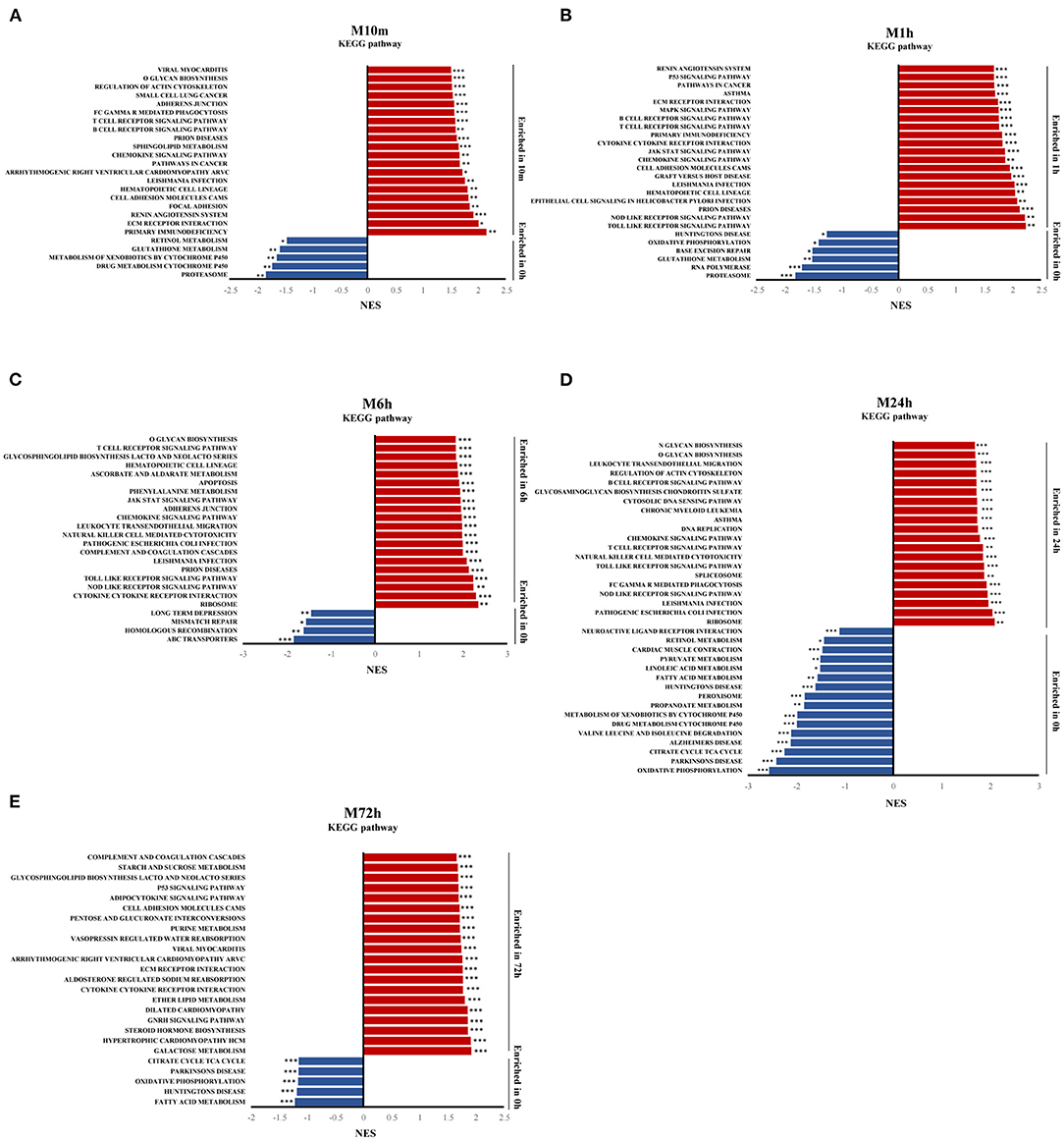
Figure 3. Gene expression analysis by GSEA. (A–E) Gene set enrichment analysis (GSEA) of KEGG gene sets from the Molecular Signatures Database (MSigDB), showing the most significantly enriched gene sets in MI, and their normalized enrichment scores. *p-value < 0.05, **p-value < 0.01, ***p-value < 0.001.
Trend Analysis of mRNA and Function Annotation
7581 genes were considered by STEM to have undergone expression changes between 0, 1, and 72 h. These genes were clustered into 8 profiles (Figure 4A). We identified 3 significant clusters, and the profile boxes display the gene expression patterns over three time points. The expression of mRNAs in profile #4 remained temporally stable from 0 to 1 h but rapidly increased at 72 h (Figure 4B). Moreover, 459 genes in profile #7 continued to increase from 0 to 72 h (Figure 4B). Profile #4 and profile #7 were in a highly similar, which indicates that the upregulation of the gene expression in the two modules over time has an impact on the development of the disease. The expression of mRNAs in profile #3 remained temporally stable from 0 to 1 h but rapidly declined at 72 h (Figure 4C). This group of mRNAs may be involved in the process of myocardial contraction, because with the development of MI, myocardial contractility decreases.
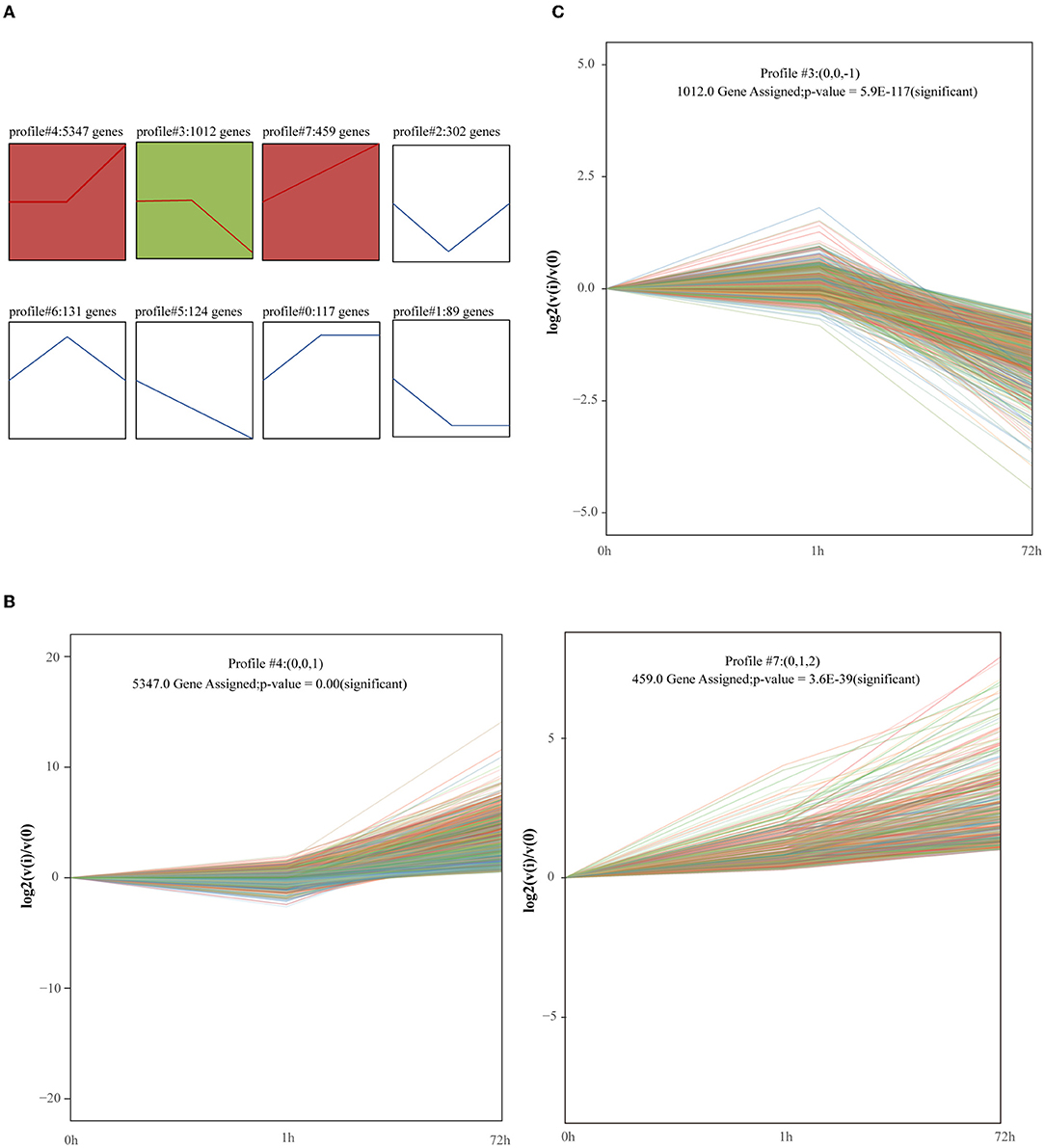
Figure 4. Short Time-Series Expression Miner (STEM) analysis of gene expression profile. Squares of the same color indicate that the correlation is >0.7. (A) Trend analysis of genes. Gene expression was normalized in the STEM software and genes with maximal values minus minimal values not <1 were included in the analysis. The 7581 genes from 0, 1, and 72 h after MI were clustered into 8 profiles. (B,C) Significantly enriched trend analysis of profile#3, profile#4, and profile#7.
Finally, two expression patterns were identified: type I profiles (profiles #4 and #7) with upregulation modes, and type II profile (profile #3) with downregulation modes. The genes included in the two patterns were shown in Supplementary Table 1.
To further investigate the function of the two profiles above, we performed GO and KEGG pathway analyses to determine a significant enrichment pathway for each expression profile (p-value < 0.05). For the type I profiles (profiles #4 and #7), the top 20 items of GO analysis and the top 30 items of KEGG analysis are shown (Figures 5A,B). The GO items included biological processes associated with immunity and inflammation, such as regulation of the immune effector process, cell activation involved in immune response and leukocyte chemotaxis. Moreover, KEGG items such as ECM-receptor interaction, apoptosis and the TNF signaling pathway are related to the immune response and apoptosis. The top 20 items of GO analysis and the top 30 items of KEGG analysis of the type II profile (profile #3) are shown in Figures 5C,D. The genes in this profile were associated primarily with energy metabolism and muscle contraction; GO analysis, such as ATP metabolic process, oxidative phosphorylation, regulation of heart contraction and nucleotide metabolic process; and KEGG analysis, such as PPAR signaling pathway, citrate cycle (TCA cycle) and cardiac muscle contraction.
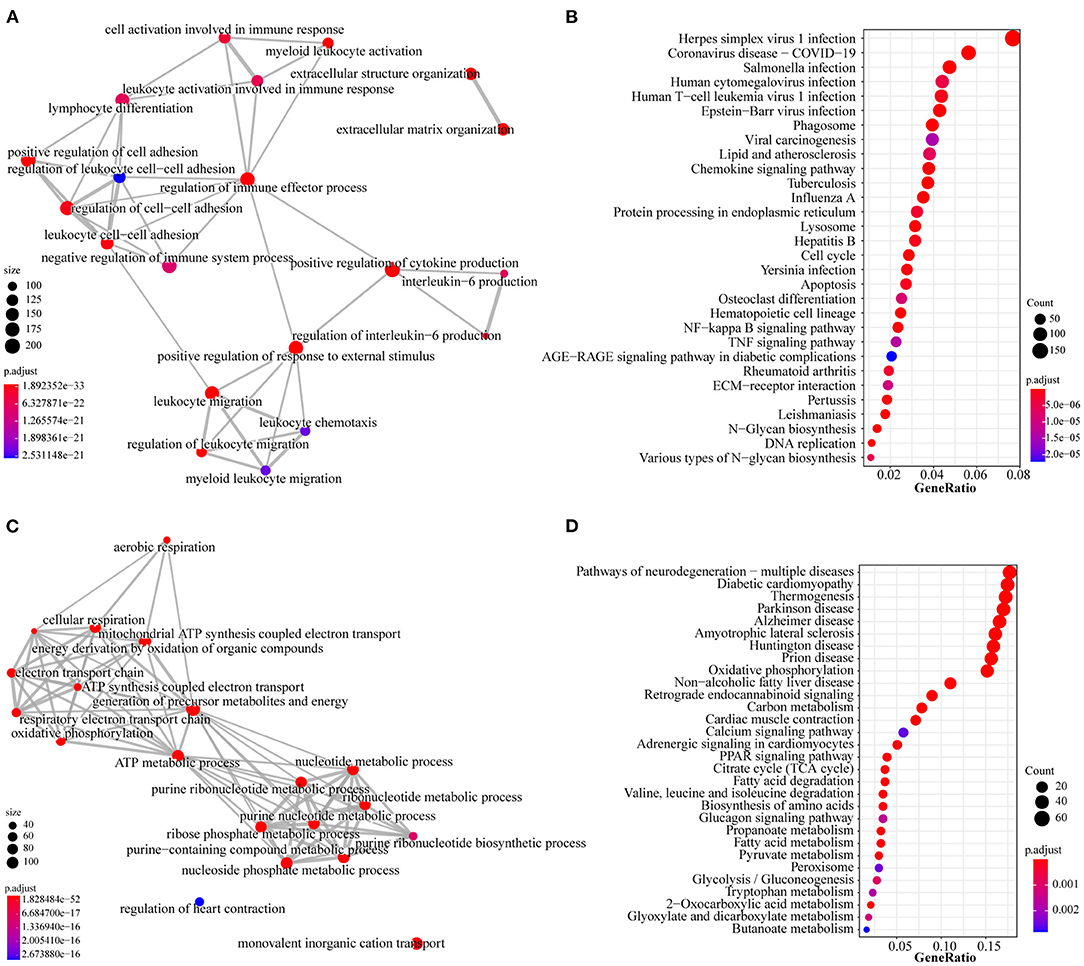
Figure 5. GO and KEGG analysis of type I profiles (profiles#4 and #7) and type II profile (profile #3). (A) Top 20 items for GO analysis of type I profiles. (B) Top 30 items for KEGG pathway analysis of type I profiles. (C) Top 20 items for GO analysis of type II profile. (D) Top 30 items for KEGG pathway analysis of type II profile. Colors represent adjusted p-value, the size of the dots represents the number of genes enriched into this biological process. The thickness of the line represents how many genes are enriched in common between the two biological processes.
Screening of Transcription Factors in Type I Profiles (Profiles #4 and #7) and Identification of Drug Targets
For the type I profiles (profiles #4 and #7), we selected genes enriched in apoptosis and inflammation related pathways (Figure 6A) as the focus of the following study. In addition, the cardiac muscle contractile pathway in the type II profile was given particular attention, and a heatmap of genes enriched in this pathway is shown in Figure 6B.
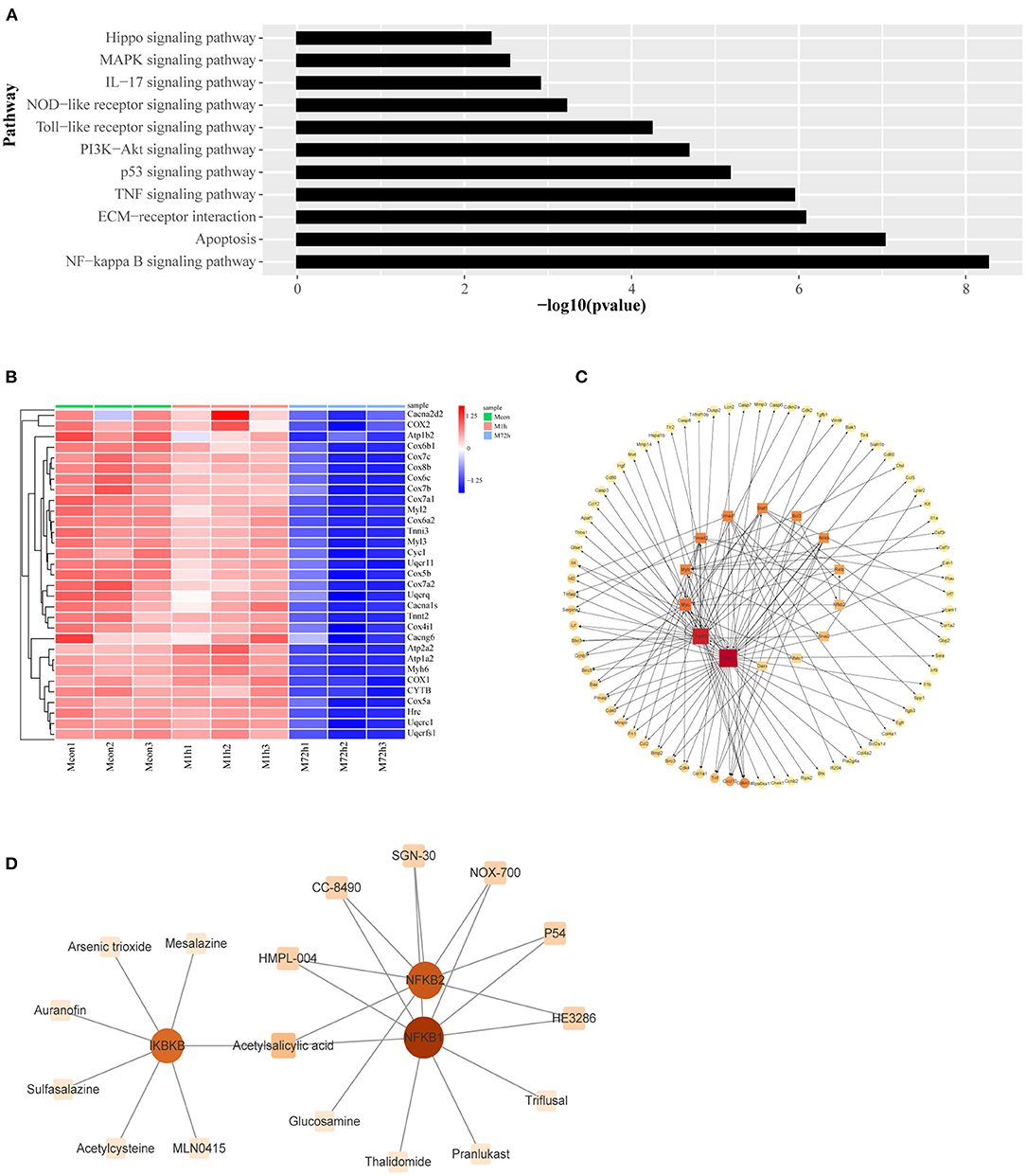
Figure 6. (A) Signaling pathways associated with apoptosis and inflammation in type I profiles (profiles #4 and #7). (B) Heatmap of genes associated with cardiac muscle contraction. (C) The transcriptional regulatory network of genes related to apoptosis and inflammation. The square nodes represent the transcription factors, the circular nodes represent the target genes, the node size represents node connectivity, and the arrow represents the regulatory direction. (D) Protein–drug interactions. The square nodes represent the drugs, the circular nodes represent the genes.
We identified transcription factors and constructed the transcriptional regulatory network of the type I profiles (profiles #4 and #7) by combining data with those in the TRRUST database. Regulatory network of transcription factors and their corresponding regulated target genes are shown in Figure 6C. Protein–drug interactions that may influence these transcription factors have been identified. The relationship of protein and drug is obtained from the DrugBank database. The results are shown in Figure 6D.
Distribution of Differentially Methylated Regions
The genomic distribution of differentially methylated regions (DMRs) across different genomic regions was investigated (Figures 7A–E), and the DMRs were found not to be randomly distributed across the genome. Most of the DMRs were located in the intron, CDS, and exon, whereas only a few of the DMRs were located in the promoter, 5'UTR, 3'UTR and TTR. In general, at 10 min, 1, 6, and 24 h after MI, the number of hypomethylated regions was higher than the number of hypermethylated regions, and at 72 h, the number of hypermethylated regions was higher than the number of hypomethylated regions. The distribution density of DMRs on different chromosomes is shown in Supplementary Figure 2.
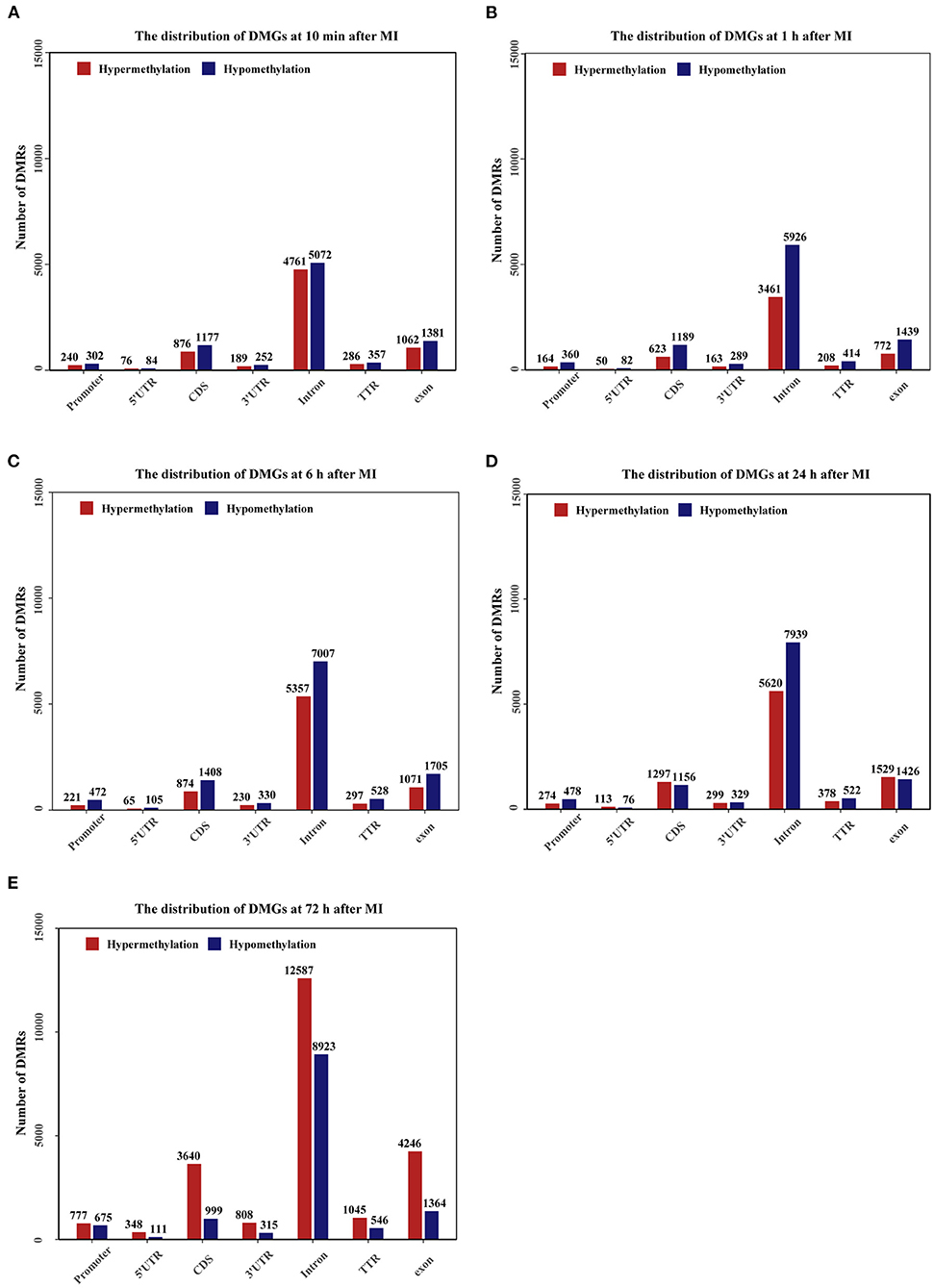
Figure 7. Bar plots showing the numbers of DMGs in promoter (2000bp upstream of the transcription start site), 5'UTR, CDS, 3'UTR, Intron, TTR (5000bp downstream of the transcription termination site), exon regions. The number of DMRs is given at the top of each graph bar. (A–E) The bar plots of DMGs distribution at 10 min, 1, 6, 24, and 72 h after MI.
Integrated Analysis of DMGs and DEGs
The subset of genes regulated by DNA methylation was identified by integrating MeDIP-Seq and RNA-Seq data. The distribution pattern of the genes with both differential methylation and expression (DMEGs) were shown (Supplementary Figure 3). Next, we analyzed the distribution of DMEGs in different gene elements. Due to the small number of DMEGs obtained at 10 min and 1 h, only the following three time points are displayed in bar plots. Bidirectional gene expression patterns were found in various genomic regions (Figures 8A–F). Of the methylated regions, methylation at the promoter region is considered the most important (27), so we focused on DMEGs in the promoter region. DNA methylation is often inversely proportional to the transcriptional activity of genes, so the degree of DNA methylation is bound to affect the expression level of genes, and then affect the function of genes (28). DMEGs at 10 min, 1, 6, and 24 h located in the promoter region with expression changes opposite to the methylation state are shown in Supplementary Table 2. We conducted GO enrichment analysis, and the results are shown (Figures 8G,H). The hypermethylated and downregulated genes were enriched in cardiac muscle contraction, and heart contraction, the hypomethylated and upregulated genes were enriched in regulation of the apoptotic signaling pathway, and regulation of cell-cell adhesion. The methylation of some important DMEGs in promoter region is shown in Table 1.
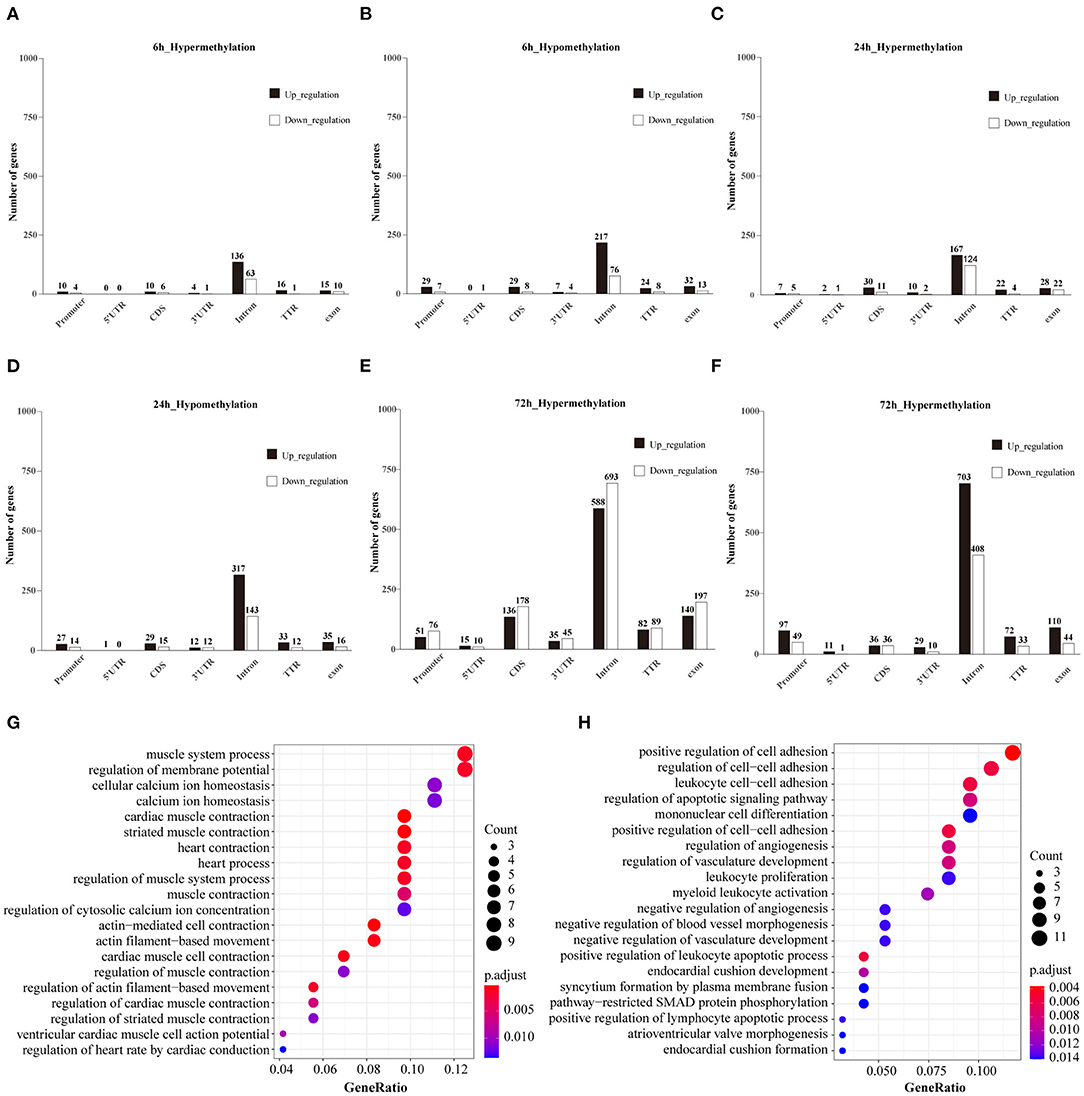
Figure 8. (A–F) Bidirectional expression patterns of differentially hypermethylated genes in different genomic elements. The number of genes is given at the top of each graph bar. (G) Top 20 items for GO analysis of hypermethylated and down-regulated genes at 72 h. (H) Top 20 items for GO analysis of hypomethylated and up-regulated genes at 72 h.
Discussion
Worldwide, 20% of surviving MI patients have a second cardiovascular event in the first year, and ~50% of major coronary events occur in patients previously discharged with a diagnosis of ischemic heart disease (29). AMI has a high mortality rate worldwide, but fast and reliable diagnosis can reduce mortality (30). Although some biomarkers have been used in the clinical diagnosis of MI, biomarkers that meet the definition of ideal cardiac biomarkers have not been discovered (30, 31). Therefore, exploring more effective biomarkers will be helpful for the diagnosis and understanding the underlying mechanism of MI.
In our research, we analyzed the gene expression profile data and DNA methylation data of mice at 0 h, 10 min, 1, 6, 24, and 72 h after myocardial infarction. Based on the single time point analysis, the 5 time points after MI were compared with 0 h, and significant changes were found in the transcriptome and DNA methylation levels at each time point. Based on time series analysis, the genes in the type I profiles are associated with apoptosis and inflammation, and the genes in the type II profile are associated with energy metabolism and muscle contraction. Fourteen transcription factors were screened among genes associated with apoptosis and inflammation in the type I profiles, including Smad1, Stat1, Bcl3, Ibkbk, Relb, Nfkb2, Snai2, Nfatc1, Daxx, Nfkb1, Trp53, Myc, Myb and Smad3. Among these transcription factors, Ikbkb, Nfkb1 and Nfkb2 have been used as drug targets for the treatment of heart disease. Among DMEGs, we found that the Thbs1 gene hypomethylated and highly expressed at multiple time points after MI. Hypomethylated upregulated genes at 72 h were involved mainly in apoptosis, angiogenesis and cell adhesion, while hypermethylated downregulated genes at 72 h were involved mainly in the biological processes of muscle contraction and cardiac contraction.
In the GSEA of the genes at each time point, we found that there were pathways related to inflammation and apoptosis at 5 time points after MI, such as the B cell receptor signaling pathway, T cell receptor signaling pathway, ECM receptor interaction, NOD-like receptor signaling pathway and p53 signaling pathway. Acute myocardial ischemia, when it occurs, will trigger the initial proinflammatory response, the purpose of which is to remove necrotic cell debris in the myocardial infarction area (32). However, molecular evidence suggests that excessive or persistent proinflammatory states after AMI may worsen poor cardiac remodeling after myocardial infarction, which is related to poor clinical outcomes (32, 33). The dysregulated proinflammatory response leads to increased expression of cytokines and proteases, thereby inducing cardiomyocyte apoptosis, and thereby inhibiting myocardial function (32).
This study obtained 17 drugs that target the transcription factors we identified. Auranofin (34, 35), triflusal (36), and acetylsalicylic acid (37) have been found to have a potential role in the treatment of cardiovascular diseases. Therefore, further investigation of these drugs for the treatment of MI is worthwhile. Transcription factor deregulation has contributed to the pathogenesis of a large number of human diseases, from diabetes, inflammatory diseases and cardiovascular disease to many cancers, and therefore these proteins have great therapeutic potential (38). Among the 14 transcription factors screened, only Ikbkb, Nfkb1 and Nfkb2 are potential targets for cardiovascular drugs and the remaining transcription factors are also valuable to be studied. We hope that our results will provide a new direction for cardiovascular drug development.
DNA methylation plays an important role in gene modification in cardiovascular aging and disease (39). There is growing evidence that MI is associated with epigenetic changes, including DNA methylation. For example, using animal models of MI, abnormal hypermethylation of the CpG site in the upstream sequence of the ALDH2 promoter has been shown to be associated with myocardial ischemic injury (40). The Thbs1 gene is hypomethylated and highly expressed at 10 min, 24, and 72 h after MI. Thbs1 can maintain the movement and growth of vascular smooth muscle cells (VSMCs). Short-term stimulation of VSMCs with FGF-2 can cause a transient increase in TSP-1 (Thbs1) expression, which may be one of the ways FGF-2 promotes atherosclerosis (41). When overexpressed, Thbs1 instead of Thbs2, Thbs3, or Thbs4 has been shown by research to be able to cause fatal heart atrophy (42). Mechanistically, Thbs1 binds to and activates the endoplasmic reticulum stress effector PERK, induces its downstream transcription factor ATF4, and causes fatal autophagy-mediated cardiac atrophy (42). In tumors, the expression of THBS1 is induced by the hypoxic tumor microenvironment and may be regulated by the TP53 pathway (43). Most downregulation of THBS1 in cancers is epigenetic, resulting from promoter hypermethylation, altered expression of regulatory non-coding RNAs, or altered levels of oncogenic transcription factors (44, 45). In our study, the Thbs1 gene, as a target gene of Trp53, was hypomethylated and highly expressed at multiple time points. Therefore, the increase in Thbs1 expression may be the cause of both hypomethylation and Trp53 activation. Thbs1 (TSP-1) is an important extracellular matrix component that affects the functions of vascular smooth muscle cells, endothelial cells, fibroblasts and inflammatory cells, and is of great significance to cardiovascular diseases (46). Increasing the understanding of the role of Thbs1 in cardiovascular diseases may provide new directions for the treatment of cardiovascular diseases.
In the results of GO analysis of hypomethylation upregulated genes at 72 h, regulation of apoptotic signaling pathway, regulation of cell-cell adhesion, positive regulation of cell-cell adhesion, regulation of angiogenesis, and regulation of vasculature development were in the GO enrichment pathway. We found that Tgfb2 (TGF-β2) was enriched in the regulation of the apoptotic signaling pathway, regulation of angiogenesis and regulation of vasculature development. Transforming growth factor (TGF)-β family members play a key role in the inflammation and repair response after MI. TGF-β can regulate the survival response of cardiomyocytes, and mediate both angiogenic and angiostatic effects (47). The experiment showed that miR-323-3p overexpression can inhibit oxidative stress and cardiomyocyte apoptosis by regulating the TGF-β2/JNK pathway (48). Moreover, miR-19b in endothelial MPs (EMPs) induced by hypoxia was shown by a study to be able to reduce endothelial cell migration and angiogenesis by downregulating TGF-β2 expression, which may have inhibited the progression of atherosclerosis (49), indicating that Tgfb2 acts as an intermediate gene to mediate the apoptosis of cardiomyocytes or the progression of atherosclerotic disease. However, the mediating effect of TGF-β is highly dependent on the environment, with both beneficial and harmful effects (47). In our research, the Tgfb2 gene was highly expressed and hypomethylated, indicating that the high expression of Tgfb2 may be due to both upstream regulatory factors and epigenetics. The role of Tgfb2 methylation in myocardial infarction has not been reported in the literature. The mechanism of Tgfb2 action in myocardial infarction needs further experimental verification.
The GO analysis of hypermethylated downregulated genes at 72 h suggested that these genes are involved multiple biological processes related to muscle contraction and heart contraction, such as muscle system process, cardiac muscle contraction, and heart contraction. Interestingly, the Tnni3 gene appears in these biological processes. Tnni3 is expressed only in myocardial tissue, encoding mainly cardiac troponin I (cTnI), which together with cardiac troponin T (cTnT) and cTnC form a troponin complex, that regulates the contraction and relaxation of the heart. Clinically, after myocardial ischemia-reperfusion injury, plasma cTnI is significantly increased, accompanied by a large area of cTnI loss in myocardial tissue, and plasma cTnI level will increase with the prolonged ischemia time (50), and the missing area will increase with the time of myocardial ischemia or infarction (51). The prolonged time and increase suggest that plasma cTnI content is negatively correlated with myocardial tissue cTnI expression. Cardiac troponin I and T are essential proteins for cardiac function and they are very sensitive markers for detecting myocardial injury (52). In our time series analysis, we also found Tnni3, which is present in the type II profile and is enriched in the cardiac muscle contraction pathway. With the development of MI, the expression of Tnni3 gradually decreased. Interestingly, the hypermethylation state of the promoters of Tnni3 did not always exist, but hypermethylation began to appear after 72 h. Hypermethylation of the promoter of Tnni3 may be one of the reasons for the aggravation of the disease. There is no report on the methylation of Tnni3 in cardiovascular diseases, and the specific impact needs further experimental verification.
Several limitations exist in our study as well. Our study was limited to the early stages of myocardial infarction, and further studies are needed on the performance of these results in the longer term after MI. Furthermore, the genetic and methylation features we obtained that influence the disease process need to be validated by more experiments.
Conclusion
The present study provided comprehensive mRNA and methylation expression profiling in the heart. A single time point analysis found that the inflammatory response after MI continued within 72 h. Time series analysis identified two expression patterns. In type I profiles, 14 transcription factors related to inflammation and apoptosis were screened out and 17 drugs that target these transcription factors were identified. Some drugs have been reported to be effective in the treatment of cardiovascular diseases. Integrative analysis of the transcriptome and methylation screened out genes that reverse changes in methylation and gene expression, represented by Thbs1, Tgfb2 and Tnni3. As one of the target genes of Trp53, Thbs1 is in a state of hypomethylation and high expression at multiple time points, and its expression changes may be the dual role of upstream regulatory factors and methylation. However, more experiments in the near future are needed to verify these conclusions.
Data Availability Statement
The datasets presented in this study can be found in online repositories. The name of the repository is GEO database and accession number is GSE206282.
Ethics Statement
We retrieved all data from publicly available resources and we required no ethical approvals for dissemination of this purely academic information.
Author Contributions
YH: conceptualization, methodology, and writing- original draft preparation. BD, YL, and XG: writing- reviewing and editing and supervision. XW and JW: conceptualization, methodology, and software. YZ: data curation and supervision. YG and XC: visualization and investigation. CL: supervision. All authors contributed to the article and approved the submitted version.
Funding
This work was supported by the National Natural Science Foundation of China (81772829 and 81830052), the Special Program for Collaborative Innovation, the Construction Project of Shanghai Key Laboratory of Molecular Imaging (18DZ2260400), the Shanghai Municipal Commission of Health and Family Planning (202150007), the Discipline Leaders Training Program of Pudong New District Health Bureau (No. PWRd2020-07) and Top-100 Talent Cultivation Plan of Shanghai University of Medicine and Health Sciences.
Conflict of Interest
The authors declare that the research was conducted in the absence of any commercial or financial relationships that could be construed as a potential conflict of interest.
Publisher's Note
All claims expressed in this article are solely those of the authors and do not necessarily represent those of their affiliated organizations, or those of the publisher, the editors and the reviewers. Any product that may be evaluated in this article, or claim that may be made by its manufacturer, is not guaranteed or endorsed by the publisher.
Supplementary Material
The Supplementary Material for this article can be found online at: https://www.frontiersin.org/articles/10.3389/fcvm.2022.912454/full#supplementary-material
Supplementary Figure 1. Bar chart of the number of up-regulated and down-regulated differential genes at different time points.
Supplementary Figure 2. Rainfall plots for differentially methylated regions (DMR) and their genomic densities. In the plot, red corresponds to hypermethylated DMRs (gain of methylation) and blue corresponds to hypomethylated DMRs (loss of methylation). (A–E) The rainfall plots of DMRs distribution at 10 min, 1, 6, 24, and 72 h after MI.
Supplementary Figure 3. Venn diagram of DEGs and DMGs. Each group was further divided into up-regulation group and down-regulation group. The number of genes is given in the middle of each figure section. (A–E) The venn diagram at 10 min, 1, 6, 24, and 72 h after MI.
Supplementary Table 1. The genes included in profile #3, profile #4 and profile #7 and their FPKM expression values at 0, 1, and 72 h.
Supplementary Table 2. The genes localized in the promoter region at 10 min, 1, 6, and 24 h and with expression changes opposite to the methylation status.
Abbreviations
MI, myocardial infarction; RNA-seq, RNA sequencing; MeDIP-seq, methylated DNA immunoprecipitation sequencing; DEGs, differentially expressed genes; DMRs, differential methylation regions; FPKM, fragments per kilobase million; ES, Enrichment score; STEM, Short Time-series Expression Miner; GO, Gene Ontology; KEGG, Kyoto Encyclopedia of Genes and Genomes; TF, transcription factor; MSigDB, Molecular Signatures Database; DMEGs, differentially methylated and expressed genes.
References
1. Jennings RB, Murry CE, Steenbergen CJr, Reimer KA. Development of cell injury in sustained acute ischemia. Circulation. (1990) 82:II2–12.
2. Lu L, Liu M, Sun R, Zheng Y, Zhang P. Myocardial infarction: symptoms and treatments. Cell Biochem Biophys. (2015) 72:865–7. doi: 10.1007/s12013-015-0553-4
3. Swirski FK, Nahrendorf M. Leukocyte behavior in atherosclerosis, myocardial infarction, and heart failure. Science. (2013) 339:161–6. doi: 10.1126/science.1230719
4. Kosuge M, Kimura K, Ishikawa T, Ebina T, Hibi K, Tsukahara K, et al. Differences between men and women in terms of clinical features of ST-segment elevation acute myocardial infarction. Circ J. (2006) 70:222–6. doi: 10.1253/circj.70.222
5. Pedersen F, Butrymovich V, Kelbæk H, Wachtell K, Helqvist S, Kastrup J, et al. Short- and long-term cause of death in patients treated with primary PCI for STEMI. J Am Coll Cardiol. (2014) 64:2101–8. doi: 10.1016/j.jacc.2014.08.037
6. Smolina K, Wright FL, Rayner M, Goldacre MJ. Determinants of the decline in mortality from acute myocardial infarction in England between 2002 and 2010: linked national database study. BMJ. (2012) 344:d8059. doi: 10.1136/bmj.d8059
7. White HD, Chew DP. Acute myocardial infarction. Lancet. (2008) 372:570–84. doi: 10.1016/S0140-6736(08)61237-4
8. Kajstura J, Cheng W, Reiss K, Clark WA, Sonnenblick EH, Krajewski S, et al. Apoptotic and necrotic myocyte cell deaths are independent contributing variables of infarct size in rats. Lab Invest. (1996) 74:86–107.
9. Gupta S, Sodhi S, Mahajan V. Correlation of antioxidants with lipid peroxidation and lipid profile in patients suffering from coronary artery disease. Expert Opin Ther Targets. (2009) 13:889–94. doi: 10.1517/14728220903099668
10. Reed GW, Rossi JE, Cannon CP. Acute myocardial infarction. Lancet. (2017) 389:197–210. doi: 10.1016/S0140-6736(16)30677-8
11. Shimizu I, Yoshida Y, Katsuno T, Minamino T. Adipose tissue inflammation in diabetes and heart failure. Microbes and infection. (2013) 15:11–7. doi: 10.1016/j.micinf.2012.10.012
12. Zhou S-F, Yuan J, Liao M-Y, Xia N, Tang T-T, Li J-J, et al. IL-17A promotes ventricular remodeling after myocardial infarction. J Mol Med. (2014) 92:1105–16. doi: 10.1007/s00109-014-1176-8
13. Liu Z, Ma C, Gu J, Yu M. Potential biomarkers of acute myocardial infarction based on weighted gene co-expression network analysis. Biomed Eng Online. (2019) 18:9. doi: 10.1186/s12938-019-0625-6
14. Sun X, Han Q, Luo H, Pan X, Ji Y, Yang Y, et al. Profiling analysis of long non-coding RNAs in early postnatal mouse hearts. Sci Rep. (2017) 7:43485. doi: 10.1038/srep43485
15. Agha G, Mendelson MM, Ward-Caviness CK, Joehanes R, Huan T, Gondalia R, et al. Blood leukocyte DNA methylation predicts risk of future myocardial infarction and coronary heart disease. Circulation. (2019) 140:645–57. doi: 10.1161/CIRCULATIONAHA.118.039357
16. Yousuf FA, Kazmi K, Iqbal J, Ahmed N, Iqbal MP. Higher DNA methylation of ABO gene promoter is associated with acute myocardial infarction in a hospital-based population in Karachi. Pak J Med Sci. (2020) 36:505–10. doi: 10.12669/pjms.36.3.1406
17. Daskalopoulos EP, Hermans KCM, Debets J, Strzelecka A, Leenders P, Vervoort-Peters L, et al. The beneficial effects of UM206 on wound healing after myocardial infarction in mice are lost in follow-up experiments. Front Cardiovasc Med. (2019) 6:118. doi: 10.3389/fcvm.2019.00118
18. Martin M. Cutadapt removes adapter sequences from high-throughput sequencing reads. EMBnet. (2011) 17:10–12. doi: 10.14806/ej.17.1.200
19. Kim D, Langmead B, Salzberg SL. HISAT: a fast spliced aligner with low memory requirements. Nat Methods. (2015) 12:357–60. doi: 10.1038/nmeth.3317
20. Liao Y, Smyth GK, Shi W. featureCounts: an efficient general purpose program for assigning sequence reads to genomic features. Bioinformatics. (2014) 30:923–30. doi: 10.1093/bioinformatics/btt656
21. Lai D, Tan L, Zuo X, Liu D, Jiao D, Wan G, et al. (2021). Prognostic ferroptosis-related lncRNA signatures associated with immunotherapy and chemotherapy responses in patients with stomach cancer. Front Genet. 12:798612. doi: 10.3389/fgene.2021.798612
22. Subramanian A, Tamayo P, Mootha VK, Mukherjee S, Ebert BL, Gillette MA, et al. Gene set enrichment analysis: a knowledge-based approach for interpreting genome-wide expression profiles. Proc Natl Acad Sci U S A. (2005) 102:15545–50. doi: 10.1073/pnas.0506580102
23. Ernst J, Bar-Joseph Z. STEM: a tool for the analysis of short time series gene expression data. BMC Bioinformatics. (2006) 7:191. doi: 10.1186/1471-2105-7-191
24. Langmead B, Salzberg SL. Fast gapped-read alignment with Bowtie 2. Nat Methods. (2012) 9:357–9. doi: 10.1038/nmeth.1923
25. Zhang Y, Liu T, Meyer CA, Eeckhoute J, Johnson DS, Bernstein BE, et al. Model-based analysis of ChIP-Seq (MACS). Genome Biol. (2008) 9:R137. doi: 10.1186/gb-2008-9-9-r137
26. Lienhard M, Grimm C, Morkel M, Herwig R, Chavez L. MEDIPS: genome-wide differential coverage analysis of sequencing data derived from DNA enrichment experiments. Bioinformatics. (2014) 30:284–6. doi: 10.1093/bioinformatics/btt650
27. Goodrich JA, Tjian R. Unexpected roles for core promoter recognition factors in cell-type-specific transcription and gene regulation. Nat Rev Genet. (2010) 11:549–58. doi: 10.1038/nrg2847
28. Moore LD, Le T, Fan G. DNA methylation and its basic function. Neuropsychopharmacology. (2013) 38:23–38. doi: 10.1038/npp.2012.112
29. Piepoli MF, Corrà U, Dendale P, Frederix I, Prescott E, Schmid JP, et al. Challenges in secondary prevention after acute myocardial infarction: a call for action. Eur J Prev Cardiol. (2016) 23:1994–2006. doi: 10.1177/2047487316663873
30. Aydin S, Ugur K, Aydin S, Sahin I, Yardim M. Biomarkers in acute myocardial infarction: current perspectives. Vasc Health Risk Manag. (2019) 15:1–10. doi: 10.2147/VHRM.S166157
31. Reichlin T, Irfan A, Twerenbold R, Reiter M, Hochholzer W, Burkhalter H, et al. Utility of absolute and relative changes in cardiac troponin concentrations in the early diagnosis of acute myocardial infarction. Circulation. (2011) 124:136–45. doi: 10.1161/CIRCULATIONAHA.111.023937
32. Cheng JB, Sedgewick AJ, Finnegan AI, Harirchian P, Lee J, Kwon S, et al. Transcriptional programming of normal and inflamed human epidermis at single-cell resolution. Cell Rep. (2018) 25:871–83. doi: 10.1016/j.celrep.2018.09.006
33. Cohn JN, Ferrari R, Sharpe N. Cardiac remodeling–concepts and clinical implications: a consensus paper from an international forum on cardiac remodeling. behalf of an international forum on cardiac remodeling. J Am Coll Cardiol. (2000) 35:569–82. doi: 10.1016/S0735-1097(99)00630-0
34. Hu M, Zhang Z, Liu B, Zhang S, Chai R, Chen X, et al. Deubiquitinase inhibitor auranofin attenuated cardiac hypertrophy by blocking NF-κB activation. Cell Physiol Biochem. (2018) 45:2421–30. doi: 10.1159/000488230
35. Yang CF, Chen YY, Singh JP, Hsu SF, Liu YW, Yang CY, et al. Targeting protein tyrosine phosphatase PTP-PEST (PTPN12) for therapeutic intervention in acute myocardial infarction. Cardiovasc Res. (2020) 116:1032–46. doi: 10.1093/cvr/cvz165
36. Alvarez-Sabín J, Quintana M, Santamarina E, Maisterra O. Triflusal and aspirin in the secondary prevention of atherothrombotic ischemic stroke: a very long-term follow-up. Cerebrovasc Dis. (2014) 37:181–7. doi: 10.1159/000357662
37. Cruz-Fernández JM, López-Bescós L, García-Dorado D, López García-Aranda V, Cabadés A, Martín-Jadraque L, et al. Randomized comparative trial of triflusal and aspirin following acute myocardial infarction. Eur Heart J. (2000) 21:457–65. doi: 10.1053/euhj.1999.1874
38. Papavassiliou KA, Papavassiliou AG. Transcription factor drug targets. J Cell Biochem. (2016) 117:2693–6. doi: 10.1002/jcb.25605
39. Zhang W, Song M, Qu J, Liu GH. Epigenetic modifications in cardiovascular aging and diseases. Circ Res. (2018) 123:773–86. doi: 10.1161/CIRCRESAHA.118.312497
40. Wang P, Shen C, Diao L, Yang Z, Fan F, Wang C, et al. Aberrant hypermethylation of aldehyde dehydrogenase 2 promoter upstream sequence in rats with experimental myocardial infarction. Biomed Res Int. (2015) 2015:503692. doi: 10.1155/2015/503692
41. Barillari G, Iovane A, Bonuglia M, Albonici L, Garofano P, Di Campli E, et al. Fibroblast growth factor-2 transiently activates the p53 oncosuppressor protein in human primary vascular smooth muscle cells: implications for atherogenesis. Atherosclerosis. (2010) 210:400–6. doi: 10.1016/j.atherosclerosis.2010.01.006
42. Vanhoutte D, Schips TG, Vo A, Grimes KM, Baldwin TA, Brody MJ, et al. Thbs1 induces lethal cardiac atrophy through PERK-ATF4 regulated autophagy. Nat Commun. (2021) 12:3928. doi: 10.1038/s41467-021-24215-4
43. Alvarez Secord A, Bernardini MQ, Broadwater G, Grace LA, Huang Z, Baba T, et al. TP53 Status is associated with thrombospondin1 expression in vitro. Front Oncol. (2013) 3:269. doi: 10.3389/fonc.2013.00269
44. Italiano A, Thomas R, Breen M, Zhang L, Crago AM, Singer S, et al. The miR-17-92 cluster and its target THBS1 are differentially expressed in angiosarcomas dependent on MYC amplification. Genes Chromosomes Cancer. (2012) 51:569–78. doi: 10.1002/gcc.21943
45. Yang QW, Liu S, Tian Y, Salwen HR, Chlenski A, Weinstein J, et al. Methylation-associated silencing of the thrombospondin-1 gene in human neuroblastoma. Cancer Res. (2003) 63:6299–310.
46. Krishna SM, Golledge J. The role of thrombospondin-1 in cardiovascular health and pathology. Int J Cardiol. (2013) 168:692–706. doi: 10.1016/j.ijcard.2013.04.139
47. Frangogiannis NG. The role of transforming growth factor (TGF)-β in the infarcted myocardium. J Thorac Dis. (2017) 9:S52–63. doi: 10.21037/jtd.2016.11.19
48. Shi CC, Pan LY, Zhao YQ, Li Q, Li JG. MicroRNA-323-3p inhibits oxidative stress and apoptosis after myocardial infarction by targeting TGF-β2/JNK pathway. Eur Rev Med Pharmacol Sci. (2020) 24:6961–70. doi: 10.26355/eurrev_202006_21688
49. Liang HZ, Li SF, Zhang F, Wu MY, Li CL, Song JX, et al. Effect of endothelial microparticles induced by hypoxia on migration and angiogenesis of human umbilical vein endothelial cells by delivering MicroRNA-19b. Chin Med J. (2018) 131:2726–33. doi: 10.4103/0366-6999.245271
50. Chocron S, Alwan K, Toubin G, Kantelip B, Clement F, Kantelip JP, et al. Effects of myocardial ischemia on the release of cardiac troponin I in isolated rat hearts. J Thorac Cardiovasc Surg. (1996) 112:508–13. doi: 10.1016/S0022-5223(96)70279-9
51. Fishbein MC, Wang T, Matijasevic M, Hong L, Apple FS. Myocardial tissue troponins T and I. an immunohistochemical study in experimental models of myocardial ischemia. Cardiovasc Pathol. (2003) 12:65–71. doi: 10.1016/S1054-8807(02)00188-6
Keywords: myocardial infarction, time series, MeDIP-seq, RNA-seq, DNA methylation
Citation: Han Y, Duan B, Wu J, Zheng Y, Gu Y, Cai X, Lu C, Wu X, Li Y and Gu X (2022) Analysis of Time Series Gene Expression and DNA Methylation Reveals the Molecular Features of Myocardial Infarction Progression. Front. Cardiovasc. Med. 9:912454. doi: 10.3389/fcvm.2022.912454
Received: 04 April 2022; Accepted: 25 May 2022;
Published: 24 June 2022.
Edited by:
Emma Louise Robinson, University of Colorado, United StatesReviewed by:
Simona Greco, IRCCS San Donato Polyclinic, ItalyNarasimman Gurusamy, University of Tennessee Health Science Center (UTHSC), United States
Copyright © 2022 Han, Duan, Wu, Zheng, Gu, Cai, Lu, Wu, Li and Gu. This is an open-access article distributed under the terms of the Creative Commons Attribution License (CC BY). The use, distribution or reproduction in other forums is permitted, provided the original author(s) and the copyright owner(s) are credited and that the original publication in this journal is cited, in accordance with accepted academic practice. No use, distribution or reproduction is permitted which does not comply with these terms.
*Correspondence: Xuefeng Gu, guxf@sumhs.edu.cn; Yanfei Li, liyf@sumhs.edu.cn; Xubo Wu, wuxubo320@163.com
†These authors have contributed equally to this work