- 1Department of Radiology, Fifth Affiliated Hospital of Sun Yat-sen University, Zhuhai, China
- 2School of Biomedical Engineering, Guangzhou Xinhua University, Guangzhou, China
Coronary heart disease (CHD) is the leading cause of mortality in the world. Early detection and treatment of CHD are crucial. Currently, coronary CT angiography (CCTA) has been the prior choice for CHD screening and diagnosis, but it cannot meet the clinical needs in terms of examination quality, the accuracy of reporting, and the accuracy of prognosis analysis. In recent years, artificial intelligence (AI) has developed rapidly in the field of medicine; it played a key role in auxiliary diagnosis, disease mechanism analysis, and prognosis assessment, including a series of studies related to CHD. In this article, the application and research status of AI in CCTA were summarized and the prospects of this field were also described.
Introduction
Coronary heart disease (CHD) has been a disease with the highest mortality worldwide (1), and early detection and treatment will be beneficial to controlling risk factors and reducing cardiovascular events (2). Currently, digital subtraction angiography (DSA) is the gold standard for diagnosing coronary artery disease (CAD) (3, 4). However, DSA is an invasive examination and possesses some defects, such as it can only display the shape of blood vessels but cannot analyze the composition and nature of the plaques and the cost of DSA is high (2). Coronary CT angiography (CCTA) could use prospective or retrospective ECG gating to collect the optimal phase to reconstruct the images at any heart rate, displaying the main branches of the coronary artery in multiple directions and analyzing the diseased vessels (5). Moreover, CCTA could also provide the basis for cardiovascular risk stratification and treatment decision-making and can be used to predict the occurrence of cardiac events (6). The advantages of CCTA include noninvasiveness, convenient examination, fast speed, and relatively low price, which make it the best choice for clinical screening of CHD (7). In recent years, the number of CCTA examinations has increased year by year and the cardiovascular imaging data have increased rapidly (8, 9). Moreover, because of the shortage of imaging diagnostic talents, the quality of CCTA examinations has declined, diagnostic reports have been delayed, and missed or misdiagnosed cases have also increased. As an emerging frontier technology, artificial intelligence (AI) has been developing rapidly in the medical field, including the field of cardiovascular CT imaging (10–12). In this article, the application and research status of AI in CCTA, including imaging technology and assisted diagnosis, and its future development, are reviewed. For this purpose, studies were searched mainly in the PubMed database, by using “Artificial intelligence” or “Machine learning” and “Coronary CT angiography” as keywords and cross-searched in citations; articles published in the last 6 years were retrieved.
Artificial Intelligence Technology
Artificial intelligence technology can be mainly divided into machine learning (ML) and intelligent computing. ML is the main technology of AI, which includes supervised learning, unsupervised learning, and deep learning (DL; Figure 1). Specifically, supervised learning includes artificial neural network (ANN), support vector machine (SVM), decision tree, Random Forest (RF), naive Bayes classifier, and K-nearest neighbor (k-NN) algorithm. Unsupervised learning mainly includes clustering algorithms and association rule algorithms. DL contains convolutional neural networks (CNNs), recurrent neural networks (RNNs), and deep neural networks (DNNs) (13, 14). AI technology differs in its applications and limitations for different data types. Therefore, the accurate diagnosis of coronary artery disease can only be achieved by finding an appropriate intelligent mathematical model to match the CCTA imaging data.
Status of AI Applications at CCTA
AI-Optimized CCTA Imaging Technology
Reduce the Radiation Dose of CCTA Examination
Coronary CT angiography (CCTA) has high sensitivity and specificity for detecting CAD, but for a patient who needs long-term follow-up, multiple CCTA examinations would inevitably lead to the accumulation of radiation doses and increase the probability of radiation injury. Researchers have been developing various technologies to reduce the dose level for patients and to achieve low-dose CCTA examinations (15–17). If we only focused on reducing radiation dose while ignoring image quality, the accuracy of our diagnostics would be affected. AI technology can be used to lower radiation doses without affecting image quality in patients (Table 1). Wolterink et al. (18) suppressed the image noise caused by low-dose CT through the combination of CNN and adversarial CNN. Yang et al. (24) proposed a method of generative adversarial networks (GANs) for visual perception, which could reduce the noise level of the image in low-dose CT and preserve the key details of the images. Brodoefel et al. (25) reported that body size was an independent factor affecting the quality of CCTA images. To obtain the same image quality, the patients with large body sizes required higher tube voltage and tube current than patients with normal body sizes. Nevertheless, increasing the tube voltage and tube current would undoubtedly increase the patients' radiation dose (26). AI reduces the radiation dose by learning from CT images in regular-dose phases to remove noise from low-dose phases while maintaining image details (19). In addition, several studies have used DL methods, the radiation dose of CCTA has been significantly reduced by using a low scanning voltage, and the degree of radiation dose reduction is 36%−55.65% (19–23).
Reduce Image Noise
By using DL-based image reconstruction (DLR) and iterative reconstruction (IR), Takatsugami compared the quality of CCTA images processed by DLR and IR and also measured the noise in the image of the ascending aorta, the left atrium, and the ventricular septum in all the images (27). A contrast-to-noise ratio (CNR) for the proximal coronary artery was calculated as well. The results indicate that the average image noise for DLR images is lower than that for IR images (18.5 ± 2.8 vs. 23.0 ± 4.6 HU, P < 0.01) and CNR increased significantly (P < 0.01). With the DL image reconstruction method, Dominik C. Benz reduced the image noise by about 43% in comparison with the IR method (28). In addition, Hong et al. (29) applied DL to the removal of image noise by using an improved U-Net-type CNN; the denoised image was finally obtained by predicting the low-dose noise that might occur in the original model and then subtracting the prediction noise from the original noise. Image clarity was measured by edge rise distance (ERD), and the quality of the images was subjectively rated by two physicians. The results showed that the average ERD of the denoised image was significantly less than that of the original image (0.98 ± 0.08 vs. 0.09±0.08, P<0.001). In terms of diagnostic accuracy, there was no significant difference between the paired comparison groups. The study confirmed that, combined with IR techniques, the DL method could significantly facilitate noise reduction performance and image quality (Table 2).
Reduce Motion Artifact of the Images
The coronary artery continuously supplies blood to the heart through regular contraction and relaxation. In patients with arrhythmia and high heart rates, the motion speed exceeds the scanning and the acquisition speed of CT equipment, resulting in motion artifacts in CT images, which influences the diagnostic accuracy and the reliability of coronary CT images and interferes with the evaluation of coronary lesions (30). In the branches of the coronary arteries, the motion direction of the right coronary artery is perpendicular to the CT scanning plane, which is more prone to motion artifacts. CT equipment with better hardware and higher resolution could be replaced for improvement, but the cost is too high. Thus, it is necessary to explore another approach to improve the image quality. Lossau et al. (31) believed that the time resolution of the CCTA images was limited by the angular range required for the reconstruction and the rotation time of the system. They modeled coronary motion artifact CT data to generate the data needed for AI and then used the trained CNN to iterate to an alternative path of a motion vector field (MVF) and motion compensated filtered back projection (MC-FBP), which effectively suppressed the artifacts caused by the angle in the image. After incorporating AI technology, the motion artifacts caused by heartbeat in CCTA images were significantly reduced, and the time resolution of the device was also made up.
Segmenting Automatically Decreases Postprocessing Time
Coronary CT angiography (CCTA) can estimate the origin and variation of branch coronary artery, the location of stenosis site, and the degree of stenosis. However, the process is time-consuming and energy-consuming and requires experienced doctors to take part in the analysis (32, 33). The lack of radiologists caused a large number of images from the CCTA to not be processed in time. Multiple study centers reported that AI could be used to automatically recognize the images and could mark and measure the lesions in advance (34–37). A radiology doctor only needs to proofread the reports that are generated by AI, which drastically increases diagnostic efficiency and reduces the probability of misdiagnosis or missed diagnosis.
Coronary artery segmentation is an important content in image postprocessing and the data should be collected according to the relative stationary phase in the individual cardiac cycle as the optimal time window (38). An optimal cardiovascular structure model should be constructed so that the physicians could evaluate the anatomy of the coronary artery from multiple perspectives and analyze the lesions. Kong et al. (39) developed a fully convolutional network (FCN) with tree structure, which involved the architecture model of multiscale discriminant feature extraction and final prediction and a tree structure layer for constructing anatomical structure. The model was carried out on four large-scale three-dimensional CCTA datasets. The final experimental results illustrated that it was more accurate and efficient than other methods of coronary artery segmentation. In several comparative studies, the accuracy of AI in segmenting coronary vessels is close to the manual, but the speed is much faster than manual (Table 3) (40–42).
AI-Assisted Diagnosis of CCTA
Coronary Artery Calcium Score
Coronary artery calcium (CAC) is a manifestation of coronary atherosclerosis. The formation of CAC is an organic, complicated, and controllable process. The coronary artery calcium score (CACS) is usually detected and calculated by CCTA, which could predict the cardiac events of asymptomatic individuals (43). The CACS can guide lipid-lowering therapy and patients with CACS > 100 are most likely to benefit from lipid-lowering therapy, thereby reducing the incidence of atherosclerotic cardiovascular disease events (44).
The CACS calculation is a semi-automatic process that is required to draw the contour to obtain the region of interest (ROI) or click all the calcium-containing objects, which is usually time-consuming with manual intervention by the physicians (45). AI can accurately find and segment the vascular calcification through the algorithm and can automatically complete the calcification score, and then, the CACS was reviewed by the diagnostic physician; this prominently accelerates the process of the diagnostic system (Figure 2, Table 4). Wolterink et al. introduced a CNN algorithm, which could skip vessel segmentation and directly identify and quantify calcium. The results indicated that the CACS was very consistent with the reference quality score (46). June-Goo Lee demonstrated the high accuracy of AI on the CACS through a large sample study and used AI to perform risk stratification for CHD (47). D de Vos and van Assen (48, 49) used a CNN approach to accurately identify calcifications in cardiac and chest CT, extending automatic assessment of calcification scores to non-ECG-gated CT scans. AI can rapidly process CT images and calculate the CACS, which greatly alleviates the current shortage of medical talent. However, in reality, the CACS AI is still in its infancy, and it is only being piloted in a small number of hospitals. An important reason for this situation is the lack of large-scale clinical testing and validation of related AI software.
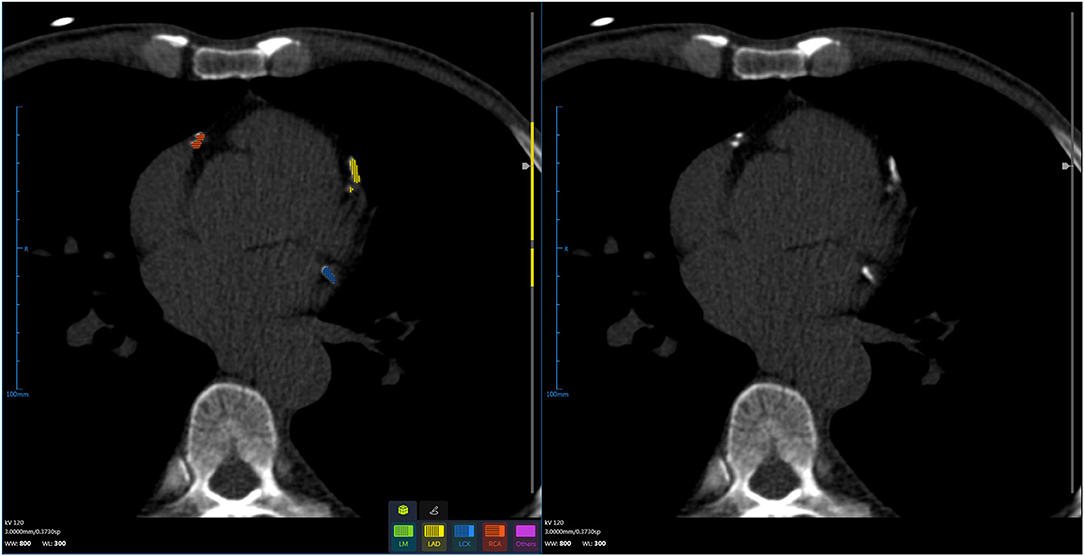
Figure 2. Using artificial intelligence, coronary artery calcification is identified, segmented, and scored.
Analyze Coronary Plaque and Assess Risk
Atherosclerotic plaque and coronary artery stenosis are causally related. When plaque is accumulated on the coronary artery wall, it causes blood flow obstruction. When plaque continues to accumulate, it would lead to lumen stenosis and even myocardial ischemia, eventually resulting in myocardial infarction (50). In addition to the clinically explained qualitative characteristics of the disease, the volume of plaque is also correlated with the severity, progression, and prognosis of CHD (51).
Clinically, plaques can be classified into calcified plaques, noncalcified plaques, and mixed plaques. Plaques of different types would also cause different degrees of stenosis, and treatment methods will vary from person to person. The identification of noncalcified and mixed plaques is not as good as that of calcified plaques. In the face of a large number of CT images, missed diagnosis and misdiagnosis might be caused by visual fatigue. To detect patients who may suffer from CHD from the CCTA images, it is necessary to visually evaluate the plaque and measure the stenosis; this is a tedious and time-consuming process (52). AI can quantify the underlying concepts of textures and structures, input certain characteristics into the machine learning model, and automatically complete the plaque analysis and stenosis rate assessment, therefore greatly reducing the actual burden of imaging workers (Figure 3) (49). Majd Zreik et al. (53) used the CNN method to detect and classify the types of coronary plaques with an accuracy of 0.77; according to the CNN method, coronary plaque detection and classification by automated methods are feasible. The detection of vulnerable plaque is the importance of CCTA examination. The instability of vulnerable plaque increases the incidence of adverse cardiovascular events (54). Plaque could be affected by external forces, such as dynamic pressure or shear stress. Meanwhile, not all unstable plaques would contribute to cardiovascular events (55). AI is capable of extracting quantitative information through the algorithm integration of imaging data and it would also facilitate extracting vulnerable plaques more quickly and automatically and provide accurate decision-making based on multiple specific features of an anatomical segment. Kolosvalay et al. (35) introduced radiomic parameters into 8 machine learning algorithms. A total of 75% of the data were employed to train the ML model, and the remaining 25% of the data were visually evaluated and histogram evaluated using the feature area under the curve (AUC) and compared with the ML model. It was found that the ML model was better than visual evaluation in the identification of advanced atherosclerotic lesions. Tesche and Rosendael compared ML risk scores with conventional CT risk scores using the AUC, namely, the Agatston calcium score and the segment involvement score (SIS); they demonstrate that the ML model could improve the accuracy of risk stratification in plaque-derived information (56, 57). Their results indicated that the AUC of the ML model was significantly higher than that of the conventional CT risk score and that there was good agreement between the unstable plaque measurements and clinical parameters (including the Framingham Risk Score). ML could identify all the plaque information from CCTA and provide a more precise risk assessment. Gudigar et al. (50) selected 122 works of literature; they analyzed and summarized the methods and performance indexes of ML and DL, compared the artificial plaque classification scheme, and concluded the application of AI algorithms in plaque deposition prediction, detection, and classification. Statistics indicated that AI algorithms could provide valuable information for treatment decision-making and that ML- and DL-based AI algorithms were outstanding in identifying plaque.
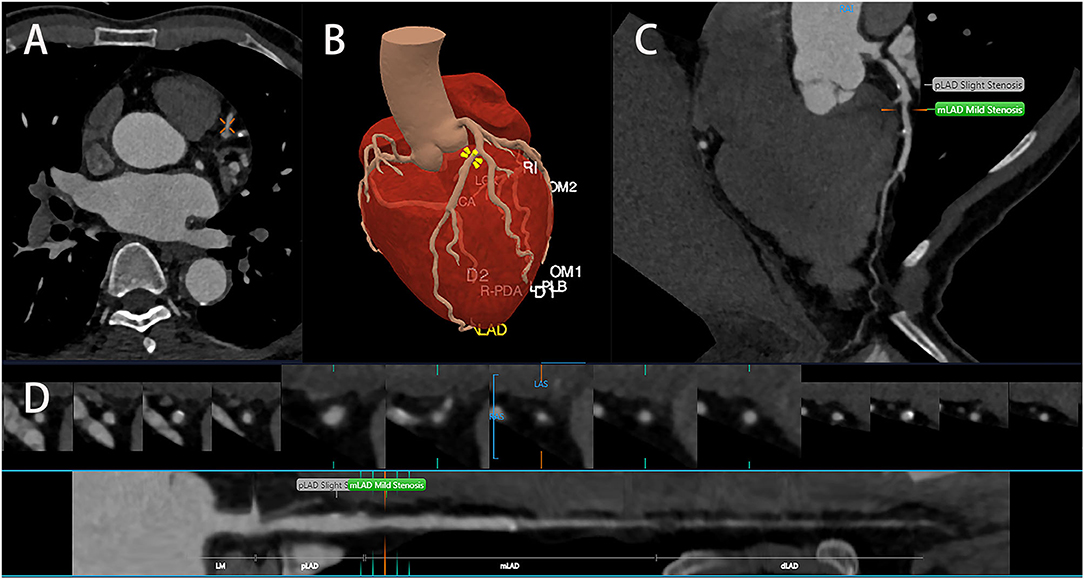
Figure 3. Artificial intelligence identifies coronary arteries (A) and segments them (B) accurately, identifies and classifies coronary plaques, and measures the severity of stenosis (C,D).
Assess the Severity of Coronary Artery Stenosis
Coronary artery stenosis is a chronic result of long-term atherosclerosis (AS) accumulation caused by multiple factors and pathways. As a result of certain inflammation factors, coronary intima hyperplasias and carotid intima-media and adventitia thickened and later fibrosis was developed. In the end, coronary arteries can be stenotic or occluded (58). Therefore, early detection of coronary artery stenosis is important.
In recent years, AI technology was used to detect coronary artery stenosis, which can assist and improve diagnostic efficiency and accuracy (Figure 3D, Table 5). Chen et al. (59) applied a DL model to CCTA, took DSA as the diagnostic standard, and compared the detection performance of the DL model and the reader model at the level of each patient, each vessel, and each segment through the AUC. From the perspective of each patient, it could be seen that the diagnostic performance of the DL model (AUC = 0.78) was better than the reader model (AUC = 0.74) and that the diagnostic time (0.47min) was significantly less than the average diagnostic time of reader model (29.65 ± 2.15min). From the perspective of each vessel and segment, the diagnostic performance of the reader model was slightly better than that of the DL model. Kang et al. also proposed an ML algorithm different from Arnoldi, Kelm, and Goldenberg, which could detect obstructive lesions (stenosis rate ≥ 50%) and nonobstructive lesions (stenosis rate 25%−50%) (33, 60–62). In these examinations, they detected lesions of the left anterior descending artery, the left circumflex artery, and the right coronary artery in 42 patients, and the results discussed by three highly qualified specialist physicians were also compared. It was found that the algorithm performed well in the automatic detection of obstructive and nonobstructive lesions by CCTA, with a sensitivity of 93%, a specificity of 95%, and an accuracy of 94%. In conclusion, AI possesses high accuracy and efficiency in detecting coronary artery stenosis.
CT-Derived Fractional Flow Reserve
The fractional flow reserve (FFR) is a tool conceived to assess the hemodynamic relevance of coronary plaques by measuring pressure differences across coronary stenosis (63). FFR functionally evaluates stenotic lesions. Nevertheless, the high cost and invasiveness of FFR are also the focus, which makes people more inclined to look for another inspection method. ML fractional flow reserve-CT (FFR-CT) is an emerging noninvasive functional examination for a combined anatomical and hemodynamic assessment of coronary lesions (Table 6). In the past, it used to take several hours to conduct computational fluid dynamics (CFD). In contrast, it took only a few minutes for the ML method, and the FFR-CT evaluation was in high consistency with traditional FFR examinations (68–70). Coenen et al. (64) took the FFR of coronary angiography as the reference standard; they recruited 351 patients and 525 vessels and compared the diagnostic performance of the FFR of CCTA by using the fluid dynamics method and ML methods. It was found that both the ML-based AUC (AUC=0.84) and CFD-based FFR-CT (AUC = 0.84) were better than the AUC of visual evaluation (AUC = 0.69, P < 0.001). The diagnostic accuracy of ML-based FFR-CT increased from 54%−63% to 75%−82%. In addition, Itu et al. (65) found that the evaluated FFR of the CCTA-based ML model was almost consistent with the CFD-based results, while the computational time of ML was reduced by 80 times. Tesche et al. (66) compared the technical performance of two methods to detect lesion-specific ischemia, namely, FFR derived from coronary CT angiography by computational fluid dynamics (FFRCFD) and FFR derived from coronary CT angiography by the machine learning algorithm (FFRML). Each lesion and patient were sensitive to FFRML at 79 and 90% for detecting lesion-specific ischemia and specific at 94 and 95%. Based on each lesion and each patient, FFRCFD produced a sensitivity of 79.0 and 89.0% and a specificity of 93.0 and 93.0%, respectively (P = 0.86 and 0.92). Compared with FFRCFD, FFRML had a significantly shorter processing time (40.5±6.3 vs. 43.4±7.1min; P = 0.042). Incorporating ML algorithms into CCTA not only improved the accuracy of diagnosis but also facilitated treatment decisions and outcome prediction. Coronary artery stenosis restricts the blood supply to the myocardium and might lead to ischemia and irreversible damage. The stenosis that significantly restricts blood flow should be treated invasively, whereas those that are minor should not be treated invasively (71).
However, there is a limitation in the ML algorithm. The quality of the image and coronary artery calcification would affect the diagnostic performance of ML-based FFR-CT. Coronary vessel segmentation is a crucial step in calculating FFR, and coronary calcification not only influences the accurate segmentation of vascular lumen but also overestimates the severity of vascular stenosis (72). Tesche et al. (67) investigated 314 patients (482 vessels in total) who first obtained the CACS, generated a patient-specific three-dimensional grid using the ML model, and calculated FFR-CT values throughout the coronary artery tree, using invasive FFR as a reference. It was reported that, with the increase in calcification score, the diagnostic accuracy of FFR-CT also decreased, but ML still had more prominent diagnostic advantages as compared to CCTA alone (69).
Epicardial Adipose Tissue and Perivascular Adipose Tissue
Pericardial fat contains pericardial adipose tissue (PAT), epicardial adipose tissue (EAT), and perivascular adipose tissue (PVAT). Among them, EAT and PVAT are close to the coronary artery and act directly on coronary atherosclerosis by the local release of inflammatory factors (73). Many studies have demonstrated that EAT and PVAT are independent predictors of adverse cardiovascular events (74–76). EAT deposits in the atrioventricular and ventricular sulcus, especially in the coronary subcutaneous vessel, and it is directly in contact with the coronary artery and its branches (77). The change in EAT thickness, therefore, may be associated with coronary artery disease in people with obesity (78, 79). In conclusion, the quantitative assessment of EAT contributes significantly to assessing coronary artery disease risk. However, quantitative analysis of EAT is obtained by manual measurement, which is very onerous. Commandeur et al. (80) proposed a fully automated quantitative tool to rapidly identify the pericardium and segment the epicardial and thoracic adipose tissues (TAT) from coronary calcium CT. Its results were more prominent compared to the improved version of the CNN with slice classification supervision (81). Commandeur combined two CNNs; they first segmented the heart and adipose tissues by a multitask CNN and then combined CNN and Statistical Shape Model (SSM) to detect the pericardium. The results showed that the agreement between expert and automatic quantification was good, with the median EAT volume of 78.03 cm3 [interquartile range (IQR): 57.08–105.79] and 78.64 cm3 (IQR: 54.48–106.58), respectively, and the correlation was 0.926 (P < 0.00001). This model is based on a deep CNN that improves the clinical guidance of EAT quantification for the diagnosis of CAD and improves the risk assessment for CAD. The PVAT can be considered as the adipose tissue around the blood vessels, and its attenuation changes can be measured by the fat attenuation index (FAI) (82). FAI reflects the differences in the peripheral coronary fat decay gradient and allows for direct visualization and quantification of coronary inflammation. When coronary artery inflammation occurs, PVAT changes its components to release proinflammatory cytokines and promote the hardening of the diseased vessel wall (83). Therefore, PVAT has an important clinical guiding value for CHD risk stratification and treatment (84). Antoniades et al. (85) proposed an AI-based image analysis method that captures perivascular attenuation gradients and reflects changes in PVAT biology caused by vascular inflammation; this method can improve the predictive ability of traditional risk stratification. Coronary artery inflammation can change due to the effects of drugs; FAI measurements are needed continuously to detect changes in perivascular adipose tissue composition, which is impossible (85). Crewe et al. (86) described fibrosis and vascular distribution based on specific texture patterns in PVAT radiomic profiles; this reflected the changes in the adipose tissues caused by chronic coronary inflammation and this algorithm significantly improved risk prediction of adverse clinical events. By combining FAI and FRP, Oikonomou et al. (83) detected adverse structural changes associated with PVAT fibrosis and microvascular remodeling. The results showed that FRP was not only significantly increased in patients with acute myocardial infarction (AMI) and that FRP remained unchanged 6 months after the event, while FAI decreased significantly after AMI. This indicates that FAI is a more dynamic measure of inflammatory biomarker and that FRP can capture more static changes. ML can also distinguish patients with acute myocardial infarction, patients with chronic CAD, or patients without CAD. Lin et al. (87) found that patients with acute myocardial infarction had significant peripheral adipose tissue radiological phenotype differences in patients with chronic coronary syndrome or without CHD; ML helps to identify patients with acute myocardial infarction.
Artificial intelligence can be more timely and can aid in the rapid analysis of the epicardial adipose tissue and the perivascular fat tissue with adverse fibrosis and distribution characteristics; AI can track the trend of coronary inflammation; AI also provides a more time-saving and more intelligent method for accurate assessment of dynamic CAD; this would help patients to reduce the incidence of adverse heart events (Table 7).
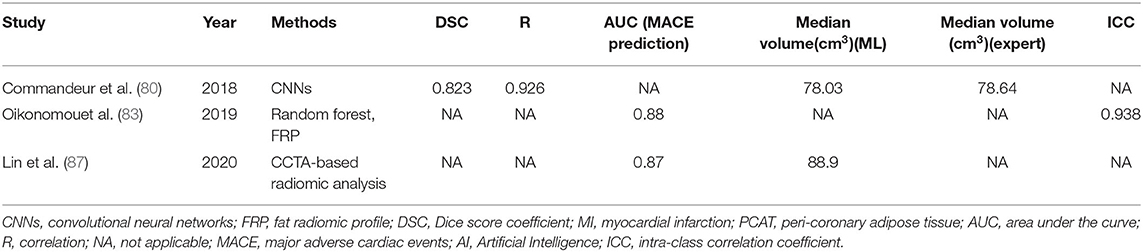
Table 7. Application of artificial intelligence in epicardial adipose tissue and perivascular adipose tissue.
Prognostic Evaluation of AI on Coronary Artery Disease
Early detection and treatment of CAD are essential to avoid cardiovascular events. In recent years, CCTA, as the main means of a prognostic examination of CHD (8), has provided important prognostic information for CAD (88). Unfortunately, due to the differences in the clinical experience of diagnostic imaging physicians, there is a certain degree of subjectivity in the evaluation study and the characteristic information and details provided by CCTA would probably be missed. Consequently, the advantages of various AI algorithms are more obvious. AI can improve decision paths, risk stratification, and outcome prediction in a more objective, repeatable, and reasonable manner. These algorithms were learned from large training datasets and were then applied to task-specific prediction and intelligent decision-making of new untrained data (89). Patel et al. (90) evaluated the prognostic value of FFR-CT on myocardial ischemia using CCTA-derived parameters. The results indicated that, compared with patients possessing abnormal FFR-CT values, the patients with normal FFR-CT had a lower incidence of myocardial ischemia, less vascular remodeling, and a significantly lower risk of cardiovascular death or myocardial infarction.
Johnson et al. (91) collected CCTA data from 6,892 patients and then compared it with the Coronary Artery Disease Reporting and Data System (CAD-RADS) scores after evaluating the prognosis by ML methods. Based on all-cause mortality, the AUC of ML was 0.77, while that of the CAD-RADS was 0.72. Based on CHD mortality, the AUC of ML was 0.85, while that of the CAD-RADS was 0.79. The AUC of ROC of ML was higher than that of the CAD-RADS score. As an automatic analysis and diagnosis tool, AI can more acutely capture the prognostic information provided by CCTA and better improve the prognostic evaluation of CHD.
Challenges and Prospects
As the main basis for disease diagnosis and treatment, huge amounts of data support the establishment of the medical image AI model. In the current status, the medical image AI system is still in the trial stage in cardiovascular diseases. The data provided for ML and modeling must be accurate, while it requires experienced doctors to label. As a result, relevant data resources are very scarce. In addition, there are deviations in the diagnostic standards of CAD in different medical institutions, and it is impossible to unify the quality and standards of data. Coupled with the issues such as data sharing and the lack of gold standards, the combination of AI and medical imaging has been hindered. In response to these problems, various medical research centers and relevant supervision departments should keep close contact to formulate data specifications and provide more important data support for the implementation of the AI automated auxiliary diagnosis system.
The realization of the combination of AI and medical imaging requires the sharing of data and the collection of these data needs the provision of basic personal information. Setting an encrypted entrance could not guarantee that the patient's privacy would not be disclosed as anyone might steal the patient's information and use it elsewhere. Therefore, the related functional departments are supposed to clarify the boundaries of medical ethics and pay close attention to the work of supervision; the personal information of the patients could be legally and compliantly managed.
Artificial intelligence has made a series of progress in CCTA image quality control, auxiliary diagnosis, and prognostic analysis, but it is still in the primary stage. The application of AI in CCTA will be more extensive and the diagnostic performance will be further facilitated. AI can further improve its value in auxiliary diagnosis, clinical prediction, and auxiliary decision-making, therefore achieving more accurate medical treatment, providing the patients with better individualized medical services, and promoting the development of cardiovascular medicine.
Conclusion
Artificial intelligence has been used to automate the CCTA workflow, such as assessing coronary artery calcium, segmenting automatically, identifying plaques, and calculating the severity of stenosis. AI will play a greater role in the accurate assessment and prognosis analysis of CHD. However, before AI is widely used in clinical practice, there must be adequate measures done to ensure data security, data standardization, and so on.
Author Contributions
JL and LH: conceived and designed the review and wrote the article. MQ: collected the data. BC: conceived and designed the review, contributed to the analysis of literature data, and wrote the article. GW: conceived and designed the review, contributed to the analysis of literature data, wrote the article, and acquired funding. All authors contributed to the article and approved the submitted version.
Funding
This study was supported by the Guangdong Medical Science and Technology Research Foundation (A2021449).
Conflict of Interest
The authors declare that the research was conducted in the absence of any commercial or financial relationships that could be construed as a potential conflict of interest.
Publisher's Note
All claims expressed in this article are solely those of the authors and do not necessarily represent those of their affiliated organizations, or those of the publisher, the editors and the reviewers. Any product that may be evaluated in this article, or claim that may be made by its manufacturer, is not guaranteed or endorsed by the publisher.
References
1. Maragna R, Giacari CM, Guglielmo M, Baggiano A, Fusini L, Guaricci AI, et al. artificial intelligence based multimodality imaging: a new frontier in coronary artery disease management. Front Cardiovasc Med. (2021) 8:736223. doi: 10.3389/fcvm.2021.736223
2. Rubin GD. Emerging and evolving roles for CT in screening for coronary heart disease. J Am Coll Radiol. (2013) 10:943–8. doi: 10.1016/j.jacr.2013.09.018
3. Pijls NH, Fearon WF, Tonino PA, Siebert U, Ikeno F, Bornschein B, et al. Fractional flow reserve versus angiography for guiding percutaneous coronary intervention in patients with multivessel coronary artery disease: 2-year follow-up of the FAME (Fractional Flow Reserve Versus Angiography for Multivessel Evaluation) study. J Am Coll Cardiol. (2010) 56:177–84. doi: 10.1016/j.jacc.2010.04.012
4. Tonino PA, Fearon WF, De Bruyne B, Oldroyd KG, Leesar MA, Ver Lee PN, et al. Angiographic versus functional severity of coronary artery stenoses in the FAME study fractional flow reserve versus angiography in multivessel evaluation. J Am Coll Cardiol. (2010) 55:2816–21. doi: 10.1016/j.jacc.2009.11.096
5. Nicol ED, Norgaard BL, Blanke P, Ahmadi A, Weir-McCall J, et al. The future of cardiovascular computed tomography: advanced analytics and clinical insights. JACC Cardiovasc Imaging. (2019) 12:1058–72. doi: 10.1016/j.jcmg.2018.11.037
6. Schoepf UJ, Tesche C. Oracle of our time: machine learning for predicting cardiovascular events. Radiology. (2019) 292:363–4. doi: 10.1148/radiol.2019191165
7. Alizadehsani R, Abdar M, Roshanzamir M, Khosravi A, Kebria PM, Khozeimeh F, et al. Machine learning-based coronary artery disease diagnosis: a comprehensive review. Comput Biol Med. (2019) 111:103346. doi: 10.1016/j.compbiomed.2019.103346
8. Knuuti J, Wijns W, Saraste A, Capodanno D, Barbato E, Funck-Brentano C, et al. 2019 ESC Guidelines for the diagnosis and management of chronic coronary syndromes. Eur Heart J. (2020) 41:407–77. doi: 10.1093/eurheartj/ehz425
9. Obermeyer Z, Emanuel EJ. Predicting the future - big data, machine learning, and clinical medicine. N Engl J Med. (2016) 375:1216–9. doi: 10.1056/NEJMp1606181
10. Jiang B, Guo N, Ge Y, Zhang L, Oudkerk M, Xie X. Development and application of artificial intelligence in cardiac imaging. Br J Radiol. (2020) 93:20190812. doi: 10.1259/bjr.20190812
11. Antman EM, Loscalzo J. Precision medicine in cardiology. Nat Rev Cardiol. (2016) 13:591–602. doi: 10.1038/nrcardio.2016.101
12. Morris MA, Saboury B, Burkett B, Gao J, Siegel EL. Reinventing radiology: big data and the future of medical imaging. J Thorac Imaging. (2018) 33:4–16. doi: 10.1097/RTI.0000000000000311
13. Betancur J, Commandeur F, Motlagh M, Sharir T, Einstein AJ, Bokhari S, et al. Deep learning for prediction of obstructive disease from fast myocardial perfusion SPECT: a multicenter study. JACC Cardiovasc Imaging. (2018) 11:1654–63. doi: 10.1016/j.jcmg.2018.01.020
14. Zreik M, Lessmann N, van Hamersvelt RW, Wolterink JM, Voskuil M, Viergever MA, et al. Deep learning analysis of the myocardium in coronary CT angiography for identification of patients with functionally significant coronary artery stenosis. Med Image Anal. (2018) 44:72–85. doi: 10.1016/j.media.2017.11.008
15. The ALARA (as low as reasonably achievable) concept in pediatric CT intelligent dose reduction. Multidisciplinary conference organized by the Society of Pediatric Radiology. August 18-19, 2001. Pediatr Radiol. (2002) 32:217–313. doi: 10.1007/s00247-002-0665-z
16. SCOT-HEART Investigators, Newby DE, Adamson PD, Berry C, Boon NA, Dweck MR, et al. Coronary CT angiography and 5-year risk of myocardial infarction. N Engl J Med. (2018) 379:924–33. doi: 10.1056/NEJMoa1805971
17. Clerc OF, Kaufmann BP, Possner M, Liga R, Vontobel J, Mikulicic F, et al. Long-term prognostic performance of low-dose coronary computed tomography angiography with prospective electrocardiogram triggering. Eur Radiol. (2017) 27:4650–60. doi: 10.1007/s00330-017-4849-1
18. Wolterink JM, Leiner T, Viergever MA, Isgum I. Generative adversarial networks for noise reduction in low-dose CT. IEEE Trans Med Imaging. (2017) 36:2536–45. doi: 10.1109/TMI.2017.2708987
19. Kang E, Koo HJ, Yang DH, Seo JB, Ye JC. Cycle-consistent adversarial denoising network for multiphase coronary CT angiography. Med Phys. (2019) 46:550–62. doi: 10.1002/mp.13284
20. Benz DC, Ersözlü S, Mojon FLA, Messerli M, Mitulla AK, Ciancone D, et al. Radiation dose reduction with deep-learning image reconstruction for coronary computed tomography angiography. Eur Radiol. (2022) 32:2620–8. doi: 10.1007/s00330-021-08367-x
21. Liu P,Wang M, Wang Y, Yu M, Wang Y, Liu Z, et al. Impact of deep learning-based optimization algorithm on image quality of low-dose coronary CT angiography with noise reduction: a Prospective Study. Acad Radiol. (2020) 27:1241–8. doi: 10.1016/j.acra.2019.11.010
22. Li W, Diao K, Wen Y, Shuai T, You Y, Zhao J, et al. High-strength deep learning image reconstruction in coronary CT angiography at 70-kVp tube voltage significantly improves image quality and reduces both radiation and contrast doses. Eur Radiol. (2022) 32:2912–20. doi: 10.1007/s00330-021-08424-5
23. Sun J, Li H, Li J, Cao Y, Zhou Z, Li M, et al. Performance evaluation of using shorter contrast injection and 70 kVp with deep learning image reconstruction for reduced contrast medium dose and radiation dose in coronary CT angiography for children: a pilot study. Quant Imaging Med Surg. (2021) 11:4162–71. doi: 10.21037/qims-20-1159
24. Yang Q, Yan P, Zhang Y, Yu H, Shi Y, Mou X, et al. Low-dose CT image denoising using a generative adversarial network with Wasserstein distance and perceptual loss. IEEE Trans Med Imaging. (2018) 37:1348–57. doi: 10.1109/TMI.2018.2827462
25. Brodoefel H, Tsiflikas I, Burgstahler C, Reimann A, Thomas C, Schroeder S, et al. Cardiac dual-source computed tomography: effect of body mass index on image quality and diagnostic accuracy. Invest Radiol. (2008) 43:712–8. doi: 10.1097/RLI.0b013e318180bae1
26. Lee SM, Lee W, Chung JW, Park EA, Park JH. Effect of kVp on image quality and accuracy in coronary CT angiography according to patient body size: a phantom study. Int J Cardiovasc Imaging. (2013) 29(Suppl 2):83–91. doi: 10.1007/s10554-013-0298-3
27. Tatsugami F, Higaki T, Nakamura Y, Yu Z, Zhou J, Lu Y, et al. Deep learning-based image restoration algorithm for coronary CT angiography. Eur Radiol. (2019) 29:5322–9. doi: 10.1007/s00330-019-06183-y
28. Benz DC, Benetos G, Rampidis G, von Felten E, Bakula A, Sustar A, et al. Validation of deep-learning image reconstruction for coronary computed tomography angiography: Impact on noise, image quality and diagnostic accuracy. J Cardiovasc Comput Tomogr. (2020) 14:444–51. doi: 10.1016/j.jcct.2020.01.002
29. Hong JH, Park EA, Lee W, von Felten E, Bakula A, Sustar A, et al. Incremental image noise reduction in coronary CT angiography using a deep learning-based technique with iterative reconstruction. Korean J Radiol. (2020) 21:1165–77. doi: 10.3348/kjr.2020.0020
30. Husmann L, Leschka S, Desbiolles L, Schepis T, Gaemperli O, Seifert B, et al. Coronary artery motion and cardiac phases: dependency on heart rate – implications for CT image reconstruction. Radiology. (2007) 245:567–76. doi: 10.1148/radiol.2451061791
31. Lossau Née Elss T, Nickisch H, Wissel T, Bippus R, Schmitt H, Morlock M, et al. Motion estimation and correction in cardiac CT angiography images using convolutional neural networks. Comput Med Imaging Graph. (2019) 76:101640. doi: 10.1016/j.compmedimag.2019.06.001
32. Pugliese F, Hunink MG, Gruszczynska K, Alberghina F, Malagó R, et al. Learning curve for coronary CT angiography: what constitutes sufficient training? Radiology. (2009) 251:359–68. doi: 10.1148/radiol.2512080384
33. Kang D, Dey D, Slomka PJ, Arsanjani R, Nakazato R, et al. Structured learning algorithm for detection of nonobstructive and obstructive coronary plaque lesions from computed tomography angiography. J Med Imaging. (2015) 2:014003. doi: 10.1117/1.JMI.2.1.014003
34. Kolossváry M, De Cecco CN, Feuchtner G, Maurovich-Horvat P. Advanced atherosclerosis imaging by CT: radiomics, machine learning, and deep learning. J Cardiovasc Comput Tomogr. (2019) 13:274–80. doi: 10.1016/j.jcct.2019.04.007
35. Kolossváry M, Karády J, Kikuchi Y, Ivanov A, Schlett CL, et al. Radiomics versus visual and histogram-based assessment to identify atheromatous lesions at coronary CT angiography: an ex vivo study. Radiology. (2019) 293:89–96. doi: 10.1148/radiol.2019190407
36. Podgorsak AR, Sommer KN, Reddy A, Iyer V, Wilson MF, et al. Initial evaluation of a convolutional neural network used for noninvasive assessment of coronary artery disease severity from coronary computed tomography angiography data. Med Phys. (2020) 47:3996–4004. doi: 10.1002/mp.14339
37. Matsumoto H, Watanabe S, Kyo E, Tsuji T, Ando Y, et al. Standardized volumetric plaque quantification and characterization from coronary CT angiography: a head-to-head comparison with invasive intravascular ultrasound. Eur Radiol. (2019) 29:6129–39. doi: 10.1007/s00330-019-06219-3
38. Leipsic J, Abbara S, Achenbach S, Cury R, Earls JP, Mancini GJ, et al. SCCT guidelines for the interpretation and reporting of coronary CT angiography: a report of the Society of Cardiovascular Computed Tomography Guidelines Committee. J Cardiovasc Comput Tomogr. (2014) 8:342–58. doi: 10.1016/j.jcct.2014.07.003
39. Kong B, Wang X, Bai J, Lu Y, Gao F, et al. Learning tree-structured representation for 3D coronary artery segmentation. Comput Med Imaging Graph. (2020) 80:101688. doi: 10.1016/j.compmedimag.2019.101688
40. Huang W, Huang L, Lin Z, Huang S, Chi Y, et al. Coronary artery segmentation by deep learning neural networks on computed tomographic coronary angiographic images. Annu Int Conf IEEE Eng Med Biol Soc. (2018) 2018:608–11. doi: 10.1109/EMBC.2018.8512328
41. Han D, Liu J, Sun Z, Cui Y, He Y, Yang Z. Deep learning analysis in coronary computed tomographic angiography imaging for the assessment of patients with coronary artery stenosis. Comput Methods Programs Biomed. (2020) 196:105651. doi: 10.1016/j.cmpb.2020.105651
42. Wan T, Shang X, Yang W, Chen J, Li D, Qin Z. Automated coronary artery tree segmentation in X-ray angiography using improved Hessian-based enhancement and statistical region merging. Comput Methods Programs Biomed. (2018) 157:179–90. doi: 10.1016/j.cmpb.2018.01.002
43. Raggi P, Cooil B, Shaw LJ, Aboulhson J, Takasu J, et al. Progression of coronary calcium on serial electron beam tomographic scanning is greater in patients with future myocardial infarction. Am J Cardiol. (2003) 92:827–9. doi: 10.1016/S0002-9149(03)00892-0
44. Gallone G, Elia E, Bruno F, Angelini F, Franchin L, Bocchino PP, et al. Impact of lipid-lowering therapies on cardiovascular outcomes according to coronary artery calcium score. A systematic review and meta-analysis. Rev Esp Cardiol. (2021) 75(6):506–14. doi: 10.1016/j.rec.2021.08.002
45. Fischer AM, Eid M, De Cecco CN, Gulsun MA, van Assen M, et al. Accuracy of an artificial intelligence deep learning algorithm implementing a recurrent neural network with long short-term memory for the automated detection of calcified plaques from coronary computed tomography angiography. J Thorac Imaging. (2020) 35(Suppl 1):S49–57. doi: 10.1097/RTI.0000000000000491
46. Wolterink JM, Leiner T, de Vos BD, van Hamersvelt RW, Viergever MA, Išgum I. Automatic coronary artery calcium scoring in cardiac CT angiography using paired convolutional neural networks. Med Image Anal. (2016) 34:123–36. doi: 10.1016/j.media.2016.04.004
47. Lee JG, Kim H, Kang H, Koo HJ, Kang JW, et al. Fully automatic coronary calcium score software empowered by artificial intelligence technology: validation study using three CT cohorts. Korean J Radiol. (2021) 22:1764–76. doi: 10.3348/kjr.2021.0148
48. de Vos BD, Wolterink JM, Leiner T, de Jong PA, Lessmann N, Isgum I. Direct automatic coronary calcium scoring in cardiac and chest CT. IEEE Trans Med Imaging. (2019) 38:2127–38. doi: 10.1109/TMI.2019.2899534
49. van Assen M, Martin SS, Varga-Szemes A, Rapaka S, Cimen S, et al. Automatic coronary calcium scoring in chest CT using a deep neural network in direct comparison with non-contrast cardiac CT: a validation study. Eur J Radiol. (2021) 134:109428. doi: 10.1016/j.ejrad.2020.109428
50. Gudigar A, Nayak S, Samanth J, Raghavendra U, Ashwal AJ, Barua PD, et al. Recent trends in artificial intelligence-assisted coronary atherosclerotic plaque characterization. Int J Environ Res Public Health. (2021) 18:10003. doi: 10.3390/ijerph181910003
51. Kolossváry M, Jávorszky N, Karády J, Vecsey-Nagy M, Dávid TZ, et al. Effect of vessel wall segmentation on volumetric and radiomic parameters of coronary plaques with adverse characteristics. J Cardiovasc Comput Tomogr. (2021) 15:137–45. doi: 10.1016/j.jcct.2020.08.001
52. Cau R, Flanders A, Mannelli L, Politi C, Faa G, et al. Artificial intelligence in computed tomography plaque characterization: a review. Eur J Radiol. (2021) 140:109767. doi: 10.1016/j.ejrad.2021.109767
53. Zreik M, van Hamersvelt RW, Wolterink JM, Leiner T, Viergever MA, Isgum I, et al. Recurrent CNN for automatic detection and classification of coronary artery plaque and stenosis in coronary CT angiography. IEEE Trans Med Imaging. (2019) 38:1588–98. doi: 10.1109/TMI.2018.2883807
54. Opincariu D, Benedek T, Chiţu M, Raţ N, Benedek I. From CT to artificial intelligence for complex assessment of plaque-associated risk. Int J Cardiovasc Imaging. (2020) 36:2403–27. doi: 10.1007/s10554-020-01926-1
55. Stefanadis C, Antoniou CK, Tsiachris D, Pietri P. Coronary atherosclerotic vulnerable plaque: current perspectives. J Am Heart Assoc. (2017) 6:e005543. doi: 10.1161/JAHA.117.005543
56. Tesche C, Bauer MJ, Baquet M, Hedels B, Straube Fm, et al. Improved long-term prognostic value of coronary CT angiography-derived plaque measures and clinical parameters on adverse cardiac outcome using machine learning. Eur Radiol. (2021) 31:486–93. doi: 10.1007/s00330-020-07083-2
57. van Rosendael AR, Maliakal G, Kolli KK, Beecy A, Al'Aref SJ, et al. Maximization of the usage of coronary CTA derived plaque information using a machine learning-based algorithm to improve risk stratification; insights from the CONFIRM registry. J Cardiovasc Comput Tomogr. (2018) 12:204–9. doi: 10.1016/j.jcct.2018.04.011
58. Bechman K, Gopalan D, Nihoyannopoulos P, Mason JC. A cohort study reveals myocarditis to be a rare and life-threatening presentation of large vessel vasculitis. Semin Arthritis Rheum. (2017) 47:241–6. doi: 10.1016/j.semarthrit.2017.03.023
59. Chen M, Wang X, Hao G, Cheng X, Ma C, Guo N, et al. Diagnostic performance of deep learning-based vascular extraction and stenosis detection technique for coronary artery disease. Br J Radiol. (2020) 93:20191028. doi: 10.1259/bjr.20191028
60. Arnoldi E, Gebregziabher M, Schoepf UJ, Goldenberg R, Ramos-Duran L, et al. Automated computer-aided stenosis detection at coronary CT angiography: initial experience. Eur Radiol. (2010) 20:1160–7. doi: 10.1007/s00330-009-1644-7
61. Kelm BM, Mittal S, Zheng Y, Tsymbal A, Bernhardt D, et al. Detection, grading and classification of coronary stenoses in computed tomography angiography. Med Image Comput Comput Assist Interv. (2011) 14(Pt 3):25–32. doi: 10.1007/978-3-642-23626-6_4
62. Goldenberg R, Eilot D, Begelman G, Walach E, Ben-Ishai E, Peled N. Computer-aided simple triage (CAST) for coronary CT angiography (CCTA). Int J Comput Assist Radiol Surg. (2012) 7:819–27. doi: 10.1007/s11548-012-0684-7
63. Mehta SR, Cannon CP, Fox KA, Wallentin L, Boden WE, et al. Routine vs selective invasive strategies in patients with acute coronary syndromes: a collaborative meta-analysis of randomized trials. JAMA. (2005) 293:2908–17. doi: 10.1001/jama.293.23.2908
64. Coenen A, Kim YH, Kruk M, Tesche C, De Geer J, et al. Diagnostic accuracy of a machine-learning approach to coronary computed tomographic angiography-based fractional flow reserve: result from the MACHINE Consortium. Circ Cardiovasc Imaging. (2018) 11:e007217. doi: 10.1161/CIRCIMAGING.117.007217
65. Itu L, Rapaka S, Passerini T, Georgescu B, Schwemmer C, et al. A machine-learning approach for computation of fractional flow reserve from coronary computed tomography. J Appl Physiol. (2016) 121:42–52. doi: 10.1152/japplphysiol.00752.2015
66. Tesche C, De Cecco CN, Baumann S, Renker M, McLaurin TW, et al. Coronary CT angiography-derived fractional flow reserve: machine learning algorithm versus computational fluid dynamics modeling. Radiology. (2018) 288:64–72. doi: 10.1148/radiol.2018171291
67. Tesche C, Otani K, De Cecco CN, Coenen A, De Geer J, et al. Influence of coronary calcium on diagnostic performance of machine learning CT-FFR: results from MACHINE Registry. JACC Cardiovasc Imaging. (2020) 13:760–70. doi: 10.1016/j.jcmg.2019.06.027
68. Xu PP Li JH, Zhou F, Jiang MD, Zhou CS, Lu MJ, et al. The influence of image quality on diagnostic performance of a machine learning-based fractional flow reserve derived from coronary CT angiography. Eur Radiol. (2020) 30:2525–34. doi: 10.1007/s00330-019-06571-4
69. Tang CX, Wang YN, Zhou F, Schoepf UJ, Assen MV, et al. Diagnostic performance of fractional flow reserve derived from coronary CT angiography for detection of lesion-specific ischemia: a multi-center study and meta-analysis. Eur J Radiol. (2019) 116:90–7. doi: 10.1016/j.ejrad.2019.04.011
70. Tesche C, De Cecco CN, Albrecht MH, Duguay TM, Bayer RR 2nd, et al. Coronary CT angiography-derived fractional flow reserve. Radiology. (2017) 285:17–33. doi: 10.1148/radiol.2017162641
71. Zreik M, van Hamersvelt RW, Khalili N, Wolterink JM, Voskuil M, Viergever MA, et al. Deep learning analysis of coronary arteries in cardiac CT angiography for detection of patients requiring invasive coronary angiography. IEEE Trans Med Imaging. (2020) 39:1545–57. doi: 10.1109/TMI.2019.2953054
72. Dey D, Lin A. Machine-learning CT-FFR, and extensive coronary calcium: overcoming the Achilles heel of coronary computed tomography angiography. JACC Cardiovasc Imaging. (2020) 13:771–3. doi: 10.1016/j.jcmg.2019.08.011
73. Gorter PM, van Lindert AS, de Vos AM, Meijs MF, van der Graaf Y, et al. Quantification of epicardial and peri-coronary fat using cardiac computed tomography; reproducibility and relation with obesity and metabolic syndrome in patients suspected of coronary artery disease. Atherosclerosis. (2008) 197:896–903. doi: 10.1016/j.atherosclerosis.2007.08.016
74. Yerramasu A, Dey D, Venuraju S, Anand DV, Atwal S, et al. Increased volume of epicardial fat is an independent risk factor for accelerated progression of sub-clinical coronary atherosclerosis. Atherosclerosis. (2012) 220:223–30. doi: 10.1016/j.atherosclerosis.2011.09.041
75. Mahabadi AA, Massaro JM, Rosito GA, Levy D, Murabito JM, et al. Association of pericardial fat, intrathoracic fat, and visceral abdominal fat with cardiovascular disease burden: the Framingham Heart Study. Eur Heart J. (2009) 30:850–6. doi: 10.1093/eurheartj/ehn573
76. Oikonomou EK, Marwan M, Desai MY, Mancio J, Alashi A, et al. Non-invasive detection of coronary inflammation using computed tomography and prediction of residual cardiovascular risk (the CRISP CT study): a post hoc analysis of prospective outcome data. Lancet. (2018) 392:929–39. doi: 10.1016/S0140-6736(18)31114-0
77. Jeong JW, Jeong MH, Yun KH, Oh SK, Park EM, et al. Echocardiographic epicardial fat thickness, and coronary artery disease. Circ J. (2007) 71:536–9. doi: 10.1253/circj.71.536
78. Talman AH, Psaltis PJ, Cameron JD, Meredith IT, Seneviratne SK, Wong DT. Epicardial adipose tissue: far more than a fat depot. Cardiovasc Diagn Ther. (2014) 4:416–29. doi: 10.3978/j.issn.2223-3652.2014.11.05
79. Shimabukuro M, Hirata Y, Tabata M, Dagvasumberel M, Sato H, et al. Epicardial adipose tissue volume and adipocytokine imbalance are strongly linked to human coronary atherosclerosis. Arterioscler Thromb Vasc Biol. (2013) 33:1077–84. doi: 10.1161/ATVBAHA.112.300829
80. Commandeur F, Goeller M, Betancur J, Cadet S, Doris M, et al. Deep learning for quantification of epicardial and thoracic adipose tissue from non-contrast CT. IEEE Trans Med Imaging. (2018) 37:1835–46. doi: 10.1109/TMI.2018.2804799
81. Zunair H, Ben Hamza A. Sharp U-Net: depthwise convolutional network for biomedical image segmentation. Comput Biol Med. (2021) 136:104699. doi: 10.1016/j.compbiomed.2021.104699
82. Elnabawi YA, Oikonomou EK, Dey AK, Mancio J, Rodante JA, et al. Association of biologic therapy with coronary inflammation in patients with psoriasis as assessed by perivascular fat attenuation Index. JAMA Cardiol. (2019) 4:885–91. doi: 10.1001/jamacardio.2019.2589
83. Oikonomou EK, Williams MC, Kotanidis CP, Desai MY, Marwan M, Antonopoulos AS, et al. A novel machine learning-derived radiotranscriptomic signature of perivascular fat improves cardiac risk prediction using coronary CT angiography. Eur Heart J. (2019) 40:3529–43. doi: 10.1093/eurheartj/ehz592
84. O'Donoghue ML, Marston NA. The role of adipose tissue in cardiovascular disease: decoding its secret language. J Am Coll Cardiol. (2021) 77:2514–6. doi: 10.1016/j.jacc.2021.04.017
85. Antoniades C, Kotanidis CP, Berman DS. State-of-the-art review article. Atherosclerosis affecting fat: what can we learn by imaging perivascular adipose tissue? J Cardiovasc Comput Tomogr. (2019) 13:288–96. doi: 10.1016/j.jcct.2019.03.006
86. Crewe C, An YA, Scherer PE. The ominous triad of adipose tissue dysfunction: inflammation, fibrosis, and impaired angiogenesis. J Clin Invest. (2017) 127:74–82. doi: 10.1172/JCI88883
87. Lin A, Kolossváry M, Yuvaraj J, Cadet S, McElhinney PA, et al. Myocardial infarction associates with a distinct pericoronary adipose tissue radiomic phenotype: a Prospective Case-Control Study. JACC Cardiovasc Imaging. (2020) 13:2371–83. doi: 10.1016/j.jcmg.2020.06.033
88. Bogaty P, Brophy J. Coronary CT angiography and subsequent risk of myocardial infarction. N Engl J Med. (2019) 380:298–9. doi: 10.1056/NEJMc1816189
89. Brandt V, Emrich T, Schoepf UJ, Dargis DM, Bayer RR, et al. Ischemia and outcome prediction by cardiac CT-based machine learning. Int J Cardiovasc Imaging. (2020) 36:2429–39. doi: 10.1007/s10554-020-01929-y
90. Patel MR, Nørgaard BL, Fairbairn TA, Nieman K, Akasaka T, et al. 1-year impact on medical practice and clinical outcomes of FFRCT: the ADVANCE Registry. JACC Cardiovasc Imaging. (2020) 13:97–105. doi: 10.1016/j.jcmg.2019.03.003
Keywords: coronary heart disease, artificial intelligence, coronary CT angiography, deep learning, machine learning
Citation: Liao J, Huang L, Qu M, Chen B and Wang G (2022) Artificial Intelligence in Coronary CT Angiography: Current Status and Future Prospects. Front. Cardiovasc. Med. 9:896366. doi: 10.3389/fcvm.2022.896366
Received: 15 March 2022; Accepted: 18 May 2022;
Published: 17 June 2022.
Edited by:
Umberto Morbiducci, Politecnico di Torino, ItalyReviewed by:
Ovidio De Filippo, University Hospital of the City of Health and Science of Turin, ItalyGeorgios Benetos, National and Kapodistrian University of Athens, Greece
Copyright © 2022 Liao, Huang, Qu, Chen and Wang. This is an open-access article distributed under the terms of the Creative Commons Attribution License (CC BY). The use, distribution or reproduction in other forums is permitted, provided the original author(s) and the copyright owner(s) are credited and that the original publication in this journal is cited, in accordance with accepted academic practice. No use, distribution or reproduction is permitted which does not comply with these terms.
*Correspondence: Binghui Chen, chenbhui@mail.sysu.edu.cn; Guojie Wang, wanggj5@mail.sysu.edu.cn
†These authors have contributed equally to this work