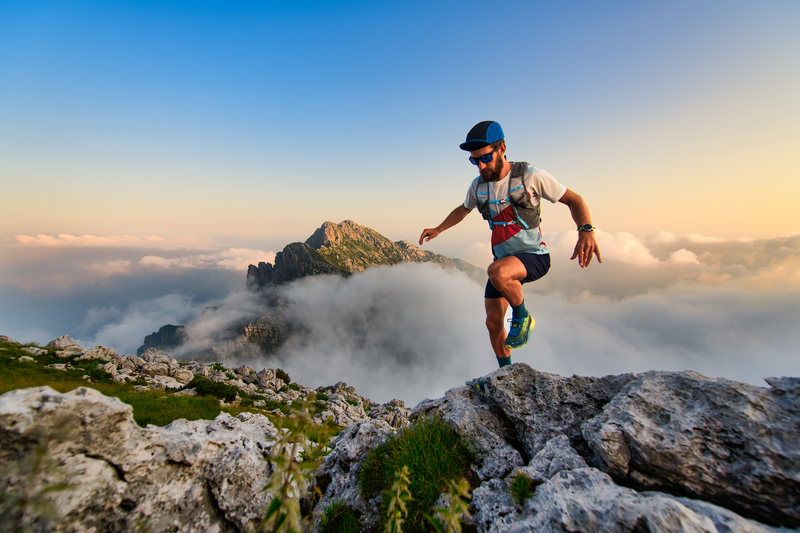
94% of researchers rate our articles as excellent or good
Learn more about the work of our research integrity team to safeguard the quality of each article we publish.
Find out more
ORIGINAL RESEARCH article
Front. Cardiovasc. Med. , 28 June 2022
Sec. Cardiovascular Metabolism
Volume 9 - 2022 | https://doi.org/10.3389/fcvm.2022.888062
This article is part of the Research Topic Mitophagy and Mitochondrial Proteostasis in Cardiovascular Diseases View all 8 articles
Objective: Atrial fibrillation (AF) is associated with both obesity and its metabolic consequences. However, there is a paucity of information on whether the dynamic change of metabolic health and obesity phenotypes affect the risk of AF. We aimed to prospectively examine the association between metabolic health and its change over time and AF risk across body mass index (BMI) categories.
Methods: A total of 58,483 participants without history of cancer, and cardiovascular diseases from the Kailuan study were included in the present study. Transition of metabolic phenotypes was evaluated between 1st survey (2006–2007) and the 2nd survey (2008–2009). The hazard ratios (HRs) and 95% confidence intervals (CIs) for AF were assessed by Cox proportional hazards regression.
Results: During a median follow-up of 3 years, we documented 580 cases of AF. Compared with metabolically healthy individuals with normal weight, the multivariable-adjusted hazard ratios for metabolically healthy and unhealthy overweight/obese were 1.27 (95% CI: 1.01, 1.59) and 1.37 (95% CI: 1.09, 1.72), respectively. However, when transition was taken into account, overweight/obese people who maintained metabolically healthy status were not associated with increased long-term risk (HR, 1.11;95% CI: 0.70, 1.78), whereas participants who converted from metabolically healthy overweight/obese status to an unhealthy phenotype had higher AF risk than those who maintained metabolically healthy normal weight (HR 1.59, 95% CI: 1.11, 2.26). When BMI and metabolically healthy status were updated over the course of the study, significant short-term elevations in AF risk were associated with individuals with stable MU-OW/OB status.
Conclusion: In this community-based cohort study, metabolically healthy overweight/obese individuals have increased risks of AF. Obesity remains a risk factor for AF independent of major metabolic factors. Our data further suggested that metabolic phenotype was a dynamic condition, and maintenance of metabolic health and normal weight might alleviate the risk of AF.
The increasing prevalence of atrial fibrillation (AF) is a growing global epidemic concern afflicting more than 33 million people worldwide in 2010, with estimated 5 million new cases arising annually (1–5). If this rapid growth continues, a dramatic increment in the health care costs is expected (6). It is therefore, the need for greater understanding of modifiable risk factors and implementing new strategies of prevention to manage this severe condition is urgent.
Overweight and obese are well-known risk factors associated with occurrence of incident AF (7). Overweight and obesity have a negative impact on the development of AF, which is mediated in part by metabolic abnormalities such as hypertension, hypercholesterolemia, and hyperglycemia (1, 8). However, individuals with overweight and obese have variation in metabolic disorders. It has been reported that there is an unique subset of obese individuals having a relatively favorable metabolic profile, termed as metabolically healthy obese (MHO) (9, 10). Contrary to some previous observations that MHO do not entail an increased risk for cardiovascular diseases (CVDs) compared with normal weight metabolically healthy individuals (11–13), recent studies have suggested that MHO phenotype was not a benign condition and associated with higher risk of chronic diseases such as CVD (14–16). However, only limited data were available for the relationship between AF and MHO and the results were conflicting (17–19). The inconsistency may due to the ethic difference and ignoring the dynamic nature of metabolic health status. Recent studies reported that MHO is a dynamic condition that changes over time and this metabolic transition may alter the risk of metabolic complications (20–23). To date, the information regarding the effects of transitions in metabolic health across the obesity status on AF risk are lacking.
Therefore, the present study aimed to examine the associations of BMI categories and metabolic health status and their transition over time with AF in a large Chinese cohort study.
The study population was obtained from the Kailuan Study, which is an ongoing cohort study conducted in a community-based population in Tangshan City, China. A detailed description of the study design and procedures has been published previously (24, 25). Briefly, 101,510 participants aged 18 years or above were enrolled between 2006 and 2007 at 11 hospitals affiliated with the Kailuan community. All of the participants were clinically follow-up approximately every 2 years to obtain information via face-to-face interviews with medical staff. For the present study, participants who underwent 1st (2006–2007) and 2nd (2008–2009) survey were included (n = 75,801). Of these remaining subjects, those with missing parameters (e.g., use of lipid-lowering, antidiabetic, or antihypertensive drugs, body mass index, HDL-C, triglycerides, blood pressure, history of diabetes and hypertension), or with previous history of cancer and CVD, or with physician diagnosed AF, or underweight were excluded. A total of 58,483 participants were included in the final analyses (Figure 1). This study was approved by the Ethics Committees of the Kailuan General Hospital), and informed consent was provided.
Height and weight were measured by trained staffs while the participants were barefoot and in light clothing. Blood pressure was measured twice from the left arm with participants in a seated position after at least 15 min of rest, using a mercury sphygmomanometer. Venous blood samples were obtained and transfused into vacuum tubes containing EDTA in the morning after participants fasted for at least 8 h. Fasting blood glucose (FBG), triglyceride (TG), total cholesterol, low-density lipoprotein cholesterol (LDL-C), and high-density lipoprotein cholesterol (HDL-C) were measured using an auto-analyzer (Hitachi747; Hitachi, Tokyo, Japan). Serum levels of C-reactive protein (CRP) were determined by an immunoturbidimetry assay (Kanto Chemical Co Inc., Tokyo, Japan), with a lower limit of detection of 0.1 mg/L. High hs-CRP levels were defined as serum hs-CRP >3 mg/L.
We classified participants into BMI categories based on recommendations of the Working Group on Obesity in China (26): normal weight (BMI 18.5–23.9 kg/m2), overweight (BMI 24.0–27.9 kg/m2), and obese (BMI ≥ 28 kg/m2). Individuals with none or one of the following disorders were deemed metabolically healthy (27): (1) elevated SBP (≥130 mmHg) and/or DBP (≥85 mmHg), or the use of antihypertensive drugs, or self-reported physician-diagnosed hypertension; (2) high FPG (≥5.6 mmol/l), or the use of antidiabetic drugs, or self-reported physician-diagnosed diabetes; (3) low HDL-C (<1.04 mmol/l in men and <1.29 mmol/l in women); and (4) high triacylglycerols (≥1.7 mmol/l) or the use of lipid-lowering drugs.
Based on the combination of BMI categories and metabolic health status, participants were then categorized into four groups: metabolically healthy normal weight (MH-NW); metabolically healthy overweight/obese (MH-OW/OB); metabolically unhealthy normal weight (MU-NO); metabolically unhealthy overweight/obese (MU-OW/OB). Transition in the metabolic health across BMI categories were examined at the follow-up biannual health examination between 1st and 2nd survey. Transitions in metabolic health yielded six phenotypes: stable MH-NW; MH-NW to MU (metabolically unhealthy); MU-NW; stable MH-OW/OB; MH-OW/OB to MU-OW/OB; MU-OW/OB (Supplementary Table 1).
Information on age, sex, smoking status (yes or no), alcohol consumption (yes or no), and physical activity level (sedentary or active) were obtained via standardized questionnaires. Smoker was defined as those who had a history of smoking. Drinker was defined as those who had a history of drinking. Physical activity was classified into two categories: sedentary/active activity for aerobic exercise <3 times/week, and aerobic exercise ≥3 times/week.
The primary endpoint was newly diagnosed AF during the follow-up period. AF diagnoses were retrieved from discharge registers at the municipal social insurance that cover all of the Kailuan study participants and resting ECGs of each survey. At each biennial survey, a 12-lead electrocardiogram performed with participants in the supine position, after a 5-min or longer rest in the quite room. The ECG and diagnosis were completed by two professional electrocardiologists according to the European Society of Cardiology guideline (28).
Baseline characteristics are presented as mean ± SD for normally distributed data, or as median (inter quartile ranges) for not normally distributed data and n (%) for categorical variables. Comparisons were made using chi-square tests for categorical variables and one-way ANOVA test for continuous variables. Cox proportional hazards model was used to examine the relationship of different phenotypes and incident AF. The risk of incident AF was first analyzed according to the baseline metabolic health and BMI categories without considering their transition, with the MH-NW phenotype as the reference. Next, we further assessed the association between the transitions in metabolic health from 2006–2007 to 2010–2011 and the risk of AF incidence during the follow-up (after 2010–2011). All Cox proportional hazards regression models were adjusted for the following covariates: age, sex, physical activity, smoke status, drink status, LDL-C, and C-reactive protein. The proportional hazard assumptions for the main Cox models were tested and no violations have been observed. To investigate the relationship between the short term risk of transitions in metabolic health status and subsequent risk of incident AF, we also constructed time-varying Cox models where BMI categories and metabolic health status were updated at each follow up and the most recent BMI measurement was used to estimate risk in the following time period. In multivariable-adjusted models, other covariates were updated at various time points, and if data were missing at a given time point, the last observation was carried forward. As recommended by Knol and VanderWeele (29, 30), additive interaction of comorbidity and hip fracture was calculated based on the relative excess risk due to interaction (RERI) compared to no hip fracture and CCI = 0, while multiplicative interaction was based on the ratio of relative risks.
To assess the robustness of our findings, we performed several sensitivity analyses:
(1) We excluded outcome events occurring within the first year of follow-up to address the potential reverse causation; (2) We excluded the participants developing CVD during follow-up; (3) We further adjusted for the weight change. In time-varying cox model, weight changes were calculated for each subject as the differences in weight from visit 2 to baseline (visit 1), from visit 3 to visit 2, from visit 4 to visit 3, and in the same way afterward. In Cox proportional hazards model, weight change calculated as between baseline and 2nd survey; (4) We repeated our analyses of incident AF using Fine-Gray models instead, accounting for the competing risk of death; Moreover, we analyzed the data of participants who were initially eligible at first survey (2006–2007) to assessing the risk of incident atrial fibrillation cross-classified by metabolic health status and obesity at baseline. All statistical analyses were conducted using SAS 9.3 (SAS Institute, Cray, NC, United States). Tests were two-sided, and a p-value < 0.05 was considered statistically significant.
Table 1 presents the participants’ baseline characteristics stratified by BMI categories and metabolic health status. Among 58,483 participants, 34.3% (n = 20,083) and 24.1% (n = 14,080) were classified as MH-NW and MU-OW/OB, respectively.
Table 1. Characteristics of participants according to metabolic health and body mass index categories.
The risk of CVD for participants cross-classified by BMI categories and metabolic health were shown in Table 2. During 5.3 million person-years of follow-up, 580 patients with new-onset AF were identified. After adjustments for clinical variables, participants with MH-OW/OB and MU-OW/OB were associated with 1.27-fold (95% CI: 1.01, 1.59) and 1.37-fold (95% CI: 1.09, 1.72) increased AF risk, respectively, compared with participants with MH-NW. We found no significant multiplicative and additive interaction between BMI and metabolic healthiness [p for additive interaction > 0.05; RERI = 0.52 (95% CI: −0.48, 1.53); p for multiplicative interaction > 0.05].
Table 2. Hazard ratios for incident atrial fibrillation classified by weight categories and metabolic health status.
We next examined the dynamic association between BMI and AF by updating measures of BMI and metabolic health status, and participants with MH-OW/OB and MU-OW/OB were associated with a significant elevation in the risk of AF. Even after adjustment for updated measures of potential confounders, such as physical activity, smoke status, drink status, LDL-C, and C-reactive protein, MH-OW/OB and MU-OW/OB status remained significantly associated with short-term elevations in AF risk.
We further investigated the effect of changes in metabolic health status during follow-up. Among participants with metabolic health, 14.3% (n = 8,390) MH-NW and 21.2% (n = 12,402) MH-OW/OB converting to metabolically unhealthy phenotype. Compared with stable MH-NW individuals, overweight/obese participants who were metabolically unhealthy at baseline or transitioned to metabolically unhealthy phenotype were at increased risk of AF (HR: 1.59; 95% CI: 1.11, 1.26 for MH-OW/OB to MU; HR: 1.63; 95% CI: 1.16, 2.31 for MU-OW/OB). However, the long-term AF risk was not significantly elevated among stable MHO individuals after multivariable adjustment. In time-varying models introducing transition in metabolic health status, BMI, and confounders as time-varying covariates, participant who maintained stable MH-OW/OB and transitioned from MH-OW/OB to unhealthy metabolic status had 1.35-fold (95% CI: 1.06, 1.73) and 1.52-fold (95% CI: 1.18, 1.95) higher short-term risk of AF than participant with stable MH-NW, respectively (Table 3).
Table 3. Hazard ratios for incident atrial fibrillation according to maintenance or transition of phenotypes in MHO group.
In the sensitivity analyses, the association between MHO phenotype and its transitions with AF development was not altered by excluding participants with AF occurring within the first years of the follow-up (Supplementary Tables 2, 3), or excluding the participants developing CVD during follow-up (Supplementary Tables 4, 5) or additional adjustment for weight change (Supplementary Table 6). To account for the competing risk of mortality, we conducted a competing risk model and the results remains similar to main results (Supplementary Tables 7, 8). Furthermore, we analyzed the data of participants who were initially eligible at first survey (2006–2007) to assessing the risk of incident atrial fibrillation cross-classified by metabolic health status and obesity at baseline (Supplementary Table 9).
In this large community-based cohort study, we explore the association between the metabolic health status and its transition over time and AF risk across the BMI categories. We found that the individuals with MH-OW/OB had higher risk of developing AF, compared with those with MH-NW. Our results further supported the notion that metabolic health was a transient state. Particularly, metabolic status converting from healthy to unhealthy further increased the risk of AF among overweight/obese individuals.
Overweight and obesity are well-established risk factor for CVDs (7). One hypothesis explains this negative association is that overweight and obesity are associated with elevations in cardiometabolic traits (31). Although observational data from some independent studies reported that obesity with metabolic healthy was not at an increased risk for cardiovascular complications (9, 32), the association between CVD risk and MHO phenotype is still controversial. However, scarce evidence is available regarding whether individuals with MH-OW/OB without an increased AF risk. To date, only four studies have investigated the associations between metabolically healthy obesity and AF risk, but the results were conflicting. The HUNT study and a Swedish study reported that compared with the MH-NW, those with healthy and unhealthy obesity associated with similar AF risk, which is consistent with our results (17, 19). On the contrary, results of French and Korean study shown that MHO was associated with a higher risk of incident AF, compared with the MH-NW (18, 33). The inconsistency may due to the lack of a consensus definition of “metabolically healthy” and the different specificity of an AF diagnosis. In our study, a stricter definition of metabolic health (an absence of all metabolic abnormalities) was adopted, an adverse effect of obesity or overweight with a metabolically healthy status on incident AF was revealed. This supports the notion that obesity and overweight itself was associated with the risk of incident AF independent of metabolic comorbidities.
More recent evidence suggested that MHO phenotype might be a transient in nature. The percentage of individuals converting from MHO to MUO was reported to vary between 30 and 50% during 4–10 years (11, 34–36). Besides, latest findings from the Nurses’ Health Study (NHS) and a Chinese study shown that over 80 and 50% of the participants with metabolically healthy obesity at baseline converted to a metabolically unhealthy phenotype during the follow-up (14, 22). In line with previous study, 23.1% original MH-OW/OB converted to metabolic unhealthy status over a period of 3 years. Thus, these findings imply that single point determination of metabolic health may be not sufficient to predict AF risk.
Several recent studies have reported that the transition from MHO to MUO increased the risk of adverse health outcomes, such as atherosclerosis, chronic kidney disease, and CVD events (14, 20, 35, 37–40). However, whether the transition of metabolic health status could affect the risk of AF development among different obesity status remains unknown. In the present study, we adopted two approaches to address the change in metabolic health status over time. First, we investigate the long-term effect of transition of metabolic health on AF development, by following up the metabolic health status. We found that having MH-OW/OB at baseline conferred high risk of AF on individuals who experienced a transition to metabolically unhealthy status later, but the participants remain stable MH-OW/OB had non-significant long-term risk of AF. Second, we utilized updated measures to account for changes in MHO phenotype over time and to characterize the short term impact MHO phenotype has on AF risk. Our results utilizing updated BMI and metabolic health status are consistent with data from other population based cohort studies utilizing single time point determination of metabolic health status (18, 33). MH-OW/OB and MU-OW/OB were at higher AF risk. In addition, individuals with stable MH-OW/OB was associated with significant short-term elevations in AF risk, compared with persistent MH-NO.
Although the exact causal mechanism has not been completely understood, the present findings are biologically plausible. Accumulating evidence suggests that individuals who are initially metabolically unhealthy or convert so during follow-up have less adequate subcutaneous adipose tissue expansion, leading to less subcutaneous, more visceral fat mass, and a higher ectopic fat deposition in the liver than those who remained metabolically healthy (41–44), which have been associated with higher levels of inflammation, insulin resistance. All these pathophysiological changes were the major triggers for CVDs, which may result in atrial fibrillation (45–49).
The present study expanded the current body of knowledge on the impact of MHO phenotype on risk of AF. Several findings from the current analysis bear mention. First, results of our study suggested that overweight/obese was associated with AF risk regardless of metabolic status. Second, we confirmed that MHO phenotype was a dynamic condition and participants with overweight/obese converting from metabolically healthy to unhealthy status further increased the risk of AF. In addition, we also revealed that conversion rate from metabolically healthy to metabolically unhealthy status in overweight/obesity individuals was higher than normal weight individuals, which may imply that obesity is an accelerating factor for metabolic abnormalities. Therefore, findings of our study support the public health policy that early management of body weight should be encouraged for the public and with aim to prevent the development of metabolic syndrome, which can be accomplished by physical activity, exercise training, and incorporating dietary strategies of reduced caloric intake (50).
Strengths of the present study include its prospective design, large sample size, updated measures of MHO phenotype, and long-term follow-up. Moreover, to the best of our knowledge, this is the first study to explore the association between transition of MHO phenotype and AF risk. Several limitations to our work should also be acknowledged. First, as the study population consisted of only Chinese, the results may not be generalizable to other ethnicities. Second, detailed data regarding the subtypes of AF, which may or may not have influenced the future development of AF, were not collected. Third, although we adjusted for covariates with either known or suspected relationships with AF risk, residual confounding always remains a possibility. Fourth, given the observational nature of the present study, the finding is not sufficient for a causal relationship. Fifth, detailed data on the potential comorbidities such as COPD OSAHS, which may have influenced the future development of AF, were also not collected.
In the present study, we suggested that overweight and obesity remains the risk factors of AF, regardless of the metabolic health status. Moreover, with repeated-measured of MHO phenotype, significant elevations in AF risk persisted in MH-OW/OB and its transition to a metabolically unhealthy status. Our findings highlight the need to maintain healthy weight and metabolic status.
The data that support the findings of this study are available from Kailuan study but restrictions apply to the availability of these data, which were used under license for the current study, and so are not publicly available. Data are however available from the corresponding author upon reasonable request and with permission of corresponding author.
This study was approved by the Ethics Committees of Kailuan General Hospital. The patients/participants provided their written informed consent to participate in this study.
YL, HX, SW, and MZ designed the research. SW and MZ conducted the research. MZ and WD wrote the manuscript. MZ and CW analyzed the data. SY, QZ, YC, BL, ZX, ZF, NZ, XC, MW, and XL edited the manuscript. YL and MZ had primary responsibility for final content. All authors read and approved the final manuscript.
This work was supported by the National Nature Science Foundation of China (82070328 and 81870249).
The authors declare that the research was conducted in the absence of any commercial or financial relationships that could be construed as a potential conflict of interest.
All claims expressed in this article are solely those of the authors and do not necessarily represent those of their affiliated organizations, or those of the publisher, the editors and the reviewers. Any product that may be evaluated in this article, or claim that may be made by its manufacturer, is not guaranteed or endorsed by the publisher.
We thank all the survey teams of the Kailuan Study Group for their contribution and the study participants who contributed their information.
The Supplementary Material for this article can be found online at: https://www.frontiersin.org/articles/10.3389/fcvm.2022.888062/full#supplementary-material
1. Fuster V, Rydén LE, Cannom DS, Crijns HJ, Curtis AB, Ellenbogen KA, et al. ACC/AHA/ESC 2006 guidelines for the management of patients with atrial fibrillation: a report of the American College of Cardiology/American Heart Association Task Force on Practice Guidelines and the European Society of Cardiology Committee for Practice Guidelines (writing committee to revise the 2001 guidelines for the management of patients with atrial fibrillation): developed in collaboration with the European Heart Rhythm Association and the Heart Rhythm Society. Circulation. (2006) 114:e257–354. doi: 10.1161/CIRCULATIONAHA.106.177292
2. Stewart S, Hart CL, Hole DJ, McMurray JJ. A population-based study of the long-term risks associated with atrial fibrillation: 20-year follow-up of the Renfrew/Paisley study. Am J Med. (2002) 113:359–64. doi: 10.1016/s0002-9343(02)01236-6
3. Thrall G, Lane D, Carroll D, Lip GY. Quality of life in patients with atrial fibrillation: a systematic review. Am J Med. (2006) 119:.448.e1–19. doi: 10.1016/j.amjmed.2005.10.057
4. Soliman EZ, Safford MM, Muntner P, Khodneva Y, Dawood FZ, Zakai NA, et al. Atrial fibrillation and the risk of myocardial infarction. JAMA Intern Med. (2014) 174:107–14. doi: 10.1001/jamainternmed.2013.11912
5. Chugh SS, Havmoeller R, Narayanan K, Singh D, Rienstra M, Benjamin EJ, et al. Worldwide epidemiology of atrial fibrillation: a Global Burden of Disease 2010 Study. Circulation. (2014) 129:837–47. doi: 10.1161/CIRCULATIONAHA.113.005119
6. Wong CX, Lau DH, Sanders P. Atrial fibrillation epidemic and hospitalizations: how to turn the rising tide. Circulation. (2014) 129:2361–3. doi: 10.1161/CIRCULATIONAHA.114.010073
7. Feng T, Vegard M, Strand LB, Laugsand LE, Mørkedal B, Aune D, et al. Weight and weight change and risk of atrial fibrillation: the HUNT study. Eur Heart J. (2019) 40:2859–66. doi: 10.1093/eurheartj/ehz390
8. Lovren F, Teoh H, Verma S. Obesity and atherosclerosis: mechanistic insights. Can J Cardiol. (2015) 31:177–83. doi: 10.1016/j.cjca.2014.11.031
9. Stefan N, Häring HU, Schulze MB. Metabolically healthy obesity: the low-hanging fruit in obesity treatment. Lancet Diabetes Endocrinol. (2018) 6:249–58. doi: 10.1016/S2213-8587(17)30292-9
10. Caleyachetty R, Thomas GN, Toulis KA, Mohammed N, Gokhale KM, Balachandran K, et al. Metabolically healthy obese and incident cardiovascular disease events among 3.5 million men and women. J Am Coll Cardiol. (2017) 70:1429–37. doi: 10.1016/j.jacc.2017.07.763
11. Appleton SL, Seaborn CJ, Visvanathan R, Hill CL, Gill TK, Taylor AW, et al. Diabetes and cardiovascular disease outcomes in the metabolically healthy obese phenotype: a cohort study. Diabetes Care. (2013) 36:2388–94. doi: 10.2337/dc12-1971
12. Hosseinpanah F, Barzin M, Sheikholeslami F, Azizi F. Effect of different obesity phenotypes on cardiovascular events in Tehran Lipid and Glucose Study (TLGS). Am J Cardiol. (2011) 107:412–6. doi: 10.1016/j.amjcard.2010.09.034
13. Mørkedal B, Vatten LJ, Romundstad PR, Laugsand LE, Janszky I. Risk of myocardial infarction and heart failure among metabolically healthy but obese individuals: HUNT (Nord-Trøndelag Health Study), Norway. J Am Coll Cardiol. (2014) 63:1071–8. doi: 10.1016/j.jacc.2013.11.035
14. Eckel N, Li Y, Kuxhaus O, Stefan N, Hu FB, Schulze MB. Transition from metabolic healthy to unhealthy phenotypes and association with cardiovascular disease risk across BMI categories in 90 257 women (the Nurses’ Health Study): 30 year follow-up from a prospective cohort study. Lancet Diabetes Endocrinol. (2018) 6:714–24. doi: 10.1016/S2213-8587(18)30137-2
15. Mirzababaei A, Djafarian K, Mozafari H, Shab-Bidar S. The long-term prognosis of heart diseases for different metabolic phenotypes: a systematic review and meta-analysis of prospective cohort studies. Endocrine. (2019) 63:439–62. doi: 10.1007/s12020-019-01840-0
16. Hinnouho GM, Czernichow S, Dugravot A, Nabi H, Brunner EJ, Kivimaki M, et al. Metabolically healthy obesity and the risk of cardiovascular disease and type 2 diabetes: the Whitehall II cohort study. Eur Heart J. (2015) 36:551–9. doi: 10.1093/eurheartj/ehu123
17. Nyström PK, Carlsson AC, Leander K, de Faire U, Hellenius ML, Gigante B. Obesity, metabolic syndrome and risk of atrial fibrillation: a Swedish, prospective cohort study. PLoS One. (2015) 10:e0127111. doi: 10.1371/journal.pone.0127111
18. Lee H, Choi EK, Lee SH, Han KD, Rhee TM, Park CS, et al. Atrial fibrillation risk in metabolically healthy obesity: a nationwide population-based study. Int J Cardiol. (2017) 240:221–7. doi: 10.1016/j.ijcard.2017.03.103
19. Feng T, Vegard M, Strand LB, Laugsand LE, Mørkedal B, Aune D, et al. Metabolically healthy obesity and risk for atrial fibrillation: the HUNT study. Obesity. (2019) 27:332–8. doi: 10.1002/oby.22377
20. Cho YK, Kang YM, Yoo JH, Lee J, Park JY, Lee WJ, et al. Implications of the dynamic nature of metabolic health status and obesity on risk of incident cardiovascular events and mortality: a nationwide population-based cohort study. Metabolism. (2019) 97:50–6. doi: 10.1016/j.metabol.2019.05.002
21. Soriguer F, Gutiérrez-Repiso C, Rubio-Martín E, García-Fuentes E, Almaraz MC, Colomo N, et al. Metabolically healthy but obese, a matter of time? Findings from the prospective Pizarra study. J Clin Endocrinol Metab. (2013) 98:2318–25. doi: 10.1210/jc.2012-4253
22. Wu S, Song L, Wang L, Chen S, Wu M, Wang Y, et al. Transitions in metabolic health and associations with arterial stiffness progression across body mass index categories. Hypertension. (2021) 78:1270–7. doi: 10.1161/HYPERTENSIONAHA.121.17735
23. Bi J, Song L, Wang L, Su B, Wu M, Li D, et al. Transitions in metabolic health status over time and risk of heart failure: a prospective study. Diabetes Metab. (2022) 48:101266. doi: 10.1016/j.diabet.2021.101266
24. Zhao M, Song L, Sun L, Wang M, Wang C, Yao S, et al. Associations of type 2 diabetes onset age with cardiovascular disease and mortality: the Kailuan study. Diabetes Care. (2021). [Epub ahead of print]. doi: 10.2337/DC20-2375
25. Zhang Q, Zhou Y, Gao X, Wang C, Zhang S, Wang A, et al. Ideal cardiovascular health metrics and the risks of ischemic and intracerebral hemorrhagic stroke. Stroke. (2013) 44:2451–6. doi: 10.1161/STROKEAHA.113.678839
26. Zhou BF. Predictive values of body mass index and waist circumference for risk factors of certain related diseases in Chinese adults–study on optimal cut-off points of body mass index and waist circumference in Chinese adults. Biomed Environ Sci. (2002) 15:83–96.
27. Alberti KG, Eckel RH, Grundy SM, Zimmet PZ, Cleeman JI, Donato KA, et al. Harmonizing the metabolic syndrome: a joint interim statement of the International Diabetes Federation Task Force on Epidemiology and Prevention; National Heart, Lung, and Blood Institute; American Heart Association; World Heart Federation; International Atherosclerosis Society; and International Association for the Study of Obesity. Circulation. (2009) 120:1640–5. doi: 10.1161/CIRCULATIONAHA.109.192644
28. Kirchhof P, Benussi S, Kotecha D, Ahlsson A, Atar D, Casadei B, et al. 2016 ESC Guidelines for the management of atrial fibrillation developed in collaboration with EACTS. Eur Heart J. (2016) 37:2893–962. doi: 10.1093/eurheartj/ehw210
29. VanderWeele TJ, Vansteelandt S. Invited commentary: some advantages of the relative excess risk due to interaction (RERI)–towards better estimators of additive interaction. Am J Epidemiol. (2014) 179:670–1. doi: 10.1093/aje/kwt316
30. Hosmer DW, Lemeshow S. Confidence interval estimation of interaction. Epidemiology. (1992) 3:452–6. doi: 10.1097/00001648-199209000-00012
31. Lavie CJ, De Schutter A, Parto P, Jahangir E, Kokkinos P, Ortega FB, et al. Obesity and Prevalence of Cardiovascular Diseases and Prognosis-The Obesity Paradox Updated. Prog Cardiovasc Dis. (2016) 58:537–47. doi: 10.1016/j.pcad.2016.01.008
32. Stefan N, Häring HU, Hu FB, Schulze MB. Metabolically healthy obesity: epidemiology, mechanisms, and clinical implications. Lancet Diabetes Endocrinol. (2013) 1:152–62. doi: 10.1016/S2213-8587(13)70062-7
33. Fauchier G, Bisson A, Bodin A, Herbert J, Semaan C, Angoulvant D, et al. Metabolically healthy obesity and cardiovascular events: a nationwide cohort study. Diabetes Obes Metab. (2021) 23:2492–501. doi: 10.1111/dom.14492
34. Mongraw-Chaffin M, Foster MC, Anderson C, Burke GL, Haq N, Kalyani RR, et al. Metabolically healthy obesity, transition to metabolic syndrome, and cardiovascular risk. J Am Coll Cardiol. (2018) 71:1857–65. doi: 10.1016/j.jacc.2018.02.055
35. Kouvari M, Panagiotakos DB, Yannakoulia M, Georgousopoulou E, Critselis E, Chrysohoou C, et al. Transition from metabolically benign to metabolically unhealthy obesity and 10-year cardiovascular disease incidence: the ATTICA cohort study. Metabolism. (2019) 93:18–24. doi: 10.1016/j.metabol.2019.01.003
36. Echouffo-Tcheugui JB, Short MI, Xanthakis V, Field P, Sponholtz TR, Larson MG, et al. Natural history of obesity subphenotypes: dynamic changes over two decades and prognosis in the framingham heart study. J Clin Endocrinol Metab. (2019) 104:738–52. doi: 10.1210/jc.2018-01321
37. Lin L, Zhang J, Jiang L, Du R, Hu C, Lu J, et al. Transition of metabolic phenotypes and risk of subclinical atherosclerosis according to BMI: a prospective study. Diabetologia. (2020) 63:1312–23. doi: 10.1007/s00125-020-05116-5
38. Guo X, Li Z, Zhou Y, Yu S, Yang H, Sun G, et al. The effects of transitions in metabolic health and obesity status on incident cardiovascular disease: insights from a general Chinese population. Eur J Prev Cardiol. (2021) 28:1250–8. doi: 10.1177/2047487320935550
39. Cho YK, Lee J, Kim HS, Park JY, Lee WJ, Kim YJ, et al. Impact of transition in metabolic health and obesity on the incident chronic kidney disease: a Nationwide cohort study. J Clin Endocrinol Metab. (2020) 105:dgaa033. doi: 10.1210/clinem/dgaa033
40. Bae YS, Choi S, Lee K, Son JS, Lee H, Cho MH, et al. Association of concurrent changes in metabolic health and weight on cardiovascular disease risk: a nationally representative cohort study. J Am Heart Assoc. (2019) 8:e011825. doi: 10.1161/JAHA.118.011825
41. Stefan N, Kantartzis K, Machann J, Schick F, Thamer C, Rittig K, et al. Identification and characterization of metabolically benign obesity in humans. Arch Intern Med. (2008) 168:1609–16. doi: 10.1001/archinte.168.15.1609
42. Tran TT, Yamamoto Y, Gesta S, Kahn CR. Beneficial effects of subcutaneous fat transplantation on metabolism. Cell Metab. (2008) 7:410–20. doi: 10.1016/j.cmet.2008.04.004
43. Gray SL, Vidal-Puig AJ. Adipose tissue expandability in the maintenance of metabolic homeostasis. Nutr Rev. (2007) 65:S7–12. doi: 10.1111/j.1753-4887.2007.tb00331.x
44. Ordovas JM, Corella D. Metabolic syndrome pathophysiology: the role of adipose tissue. Kidney Int Suppl. (2008) 111:S10–4. doi: 10.1038/ki.2008.517
45. Neeland IJ, Poirier P, Després JP. Cardiovascular and metabolic heterogeneity of obesity: clinical challenges and implications for management. Circulation. (2018) 137:1391–406. doi: 10.1161/CIRCULATIONAHA.117.029617
46. Liu J, Musani SK, Bidulescu A, Carr JJ, Wilson JG, Taylor HA, et al. Fatty liver, abdominal adipose tissue and atherosclerotic calcification in African Americans: the Jackson Heart Study. Atherosclerosis. (2012) 224:521–5. doi: 10.1016/j.atherosclerosis.2012.07.042
47. Neeland IJ, Ayers CR, Rohatgi AK, Turer AT, Berry JD, Das SR, et al. Associations of visceral and abdominal subcutaneous adipose tissue with markers of cardiac and metabolic risk in obese adults. Obesity. (2013) 21:E439–47. doi: 10.1002/oby.20135
48. Kulkarni N, Gukathasan N, Sartori S, Baber U. Chronic kidney disease and atrial fibrillation: a contemporary overview. J Atr Fibrillation. (2012) 5:448. doi: 10.4022/jafib.448
49. Zhang X, Lerman LO. The metabolic syndrome and chronic kidney disease. Transl Res. (2017) 183:14–25. doi: 10.1016/j.trsl.2016.12.004
Keywords: atrial fibrillation, obesity, metabolic health, transition, body mass index
Citation: Zhao M, Du W, Zhao Q, Chen Y, Li B, Xie Z, Fu Z, Zhang N, Cheng X, Li X, Yao S, Wang M, Wang C, Wu S, Xue H and Li Y (2022) Transition of Metabolic Phenotypes and Risk of Atrial Fibrillation According to BMI: Kailuan Study. Front. Cardiovasc. Med. 9:888062. doi: 10.3389/fcvm.2022.888062
Received: 02 March 2022; Accepted: 13 May 2022;
Published: 28 June 2022.
Edited by:
Valeria Hirschler, University of Buenos Aires, ArgentinaReviewed by:
Giulio Francesco Romiti, Sapienza University of Rome, ItalyCopyright © 2022 Zhao, Du, Zhao, Chen, Li, Xie, Fu, Zhang, Cheng, Li, Yao, Wang, Wang, Wu, Xue and Li. This is an open-access article distributed under the terms of the Creative Commons Attribution License (CC BY). The use, distribution or reproduction in other forums is permitted, provided the original author(s) and the copyright owner(s) are credited and that the original publication in this journal is cited, in accordance with accepted academic practice. No use, distribution or reproduction is permitted which does not comply with these terms.
*Correspondence: Shouling Wu, drwusl@163.com; Hao Xue, xuehaoxh301@163.com; Yang Li, liyangbsh@163.com
†These authors have contributed equally to this work
Disclaimer: All claims expressed in this article are solely those of the authors and do not necessarily represent those of their affiliated organizations, or those of the publisher, the editors and the reviewers. Any product that may be evaluated in this article or claim that may be made by its manufacturer is not guaranteed or endorsed by the publisher.
Research integrity at Frontiers
Learn more about the work of our research integrity team to safeguard the quality of each article we publish.