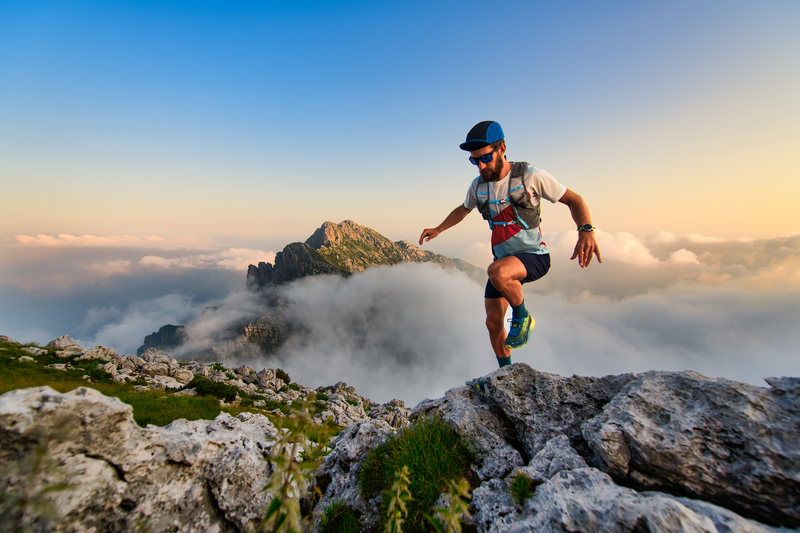
95% of researchers rate our articles as excellent or good
Learn more about the work of our research integrity team to safeguard the quality of each article we publish.
Find out more
ORIGINAL RESEARCH article
Front. Cardiovasc. Med. , 04 July 2022
Sec. Cardiac Rhythmology
Volume 9 - 2022 | https://doi.org/10.3389/fcvm.2022.885016
This article is part of the Research Topic Optimal Management of Atrial Fibrillation: Recent advances View all 11 articles
Background: Little is known regarding the risk of clinical outcomes depending on the clustering of lifestyle behaviors after atrial fibrillation (AF) diagnosis. This study evaluated the association between a cluster of healthy lifestyle behaviors and the risk of adverse outcomes in patients with AF.
Methods: Using the Korean National Insurance Service database, patients who were newly diagnosed with AF between 2009 and 2016 were included. A healthy lifestyle behavior score (HLS) was calculated by assigning 1 point each for non-current smoking, for non-drinking, and for performing regular exercise from the self-reported questionnaire in health examinations. The primary outcome was defined as major adverse cardiovascular event (MACE), including ischemic stroke, myocardial infarction, and hospitalization for heart failure.
Results: A total of 208,662 patients were included; 7.1% in HLS 0, 22.7% in HLS 1, 58.6% in HLS 2, and 11.6% in HLS 3 groups. Patients with HLS 1, 2, and 3 were associated with a lower risk of MACE than those with HLS 0 (adjusted hazard ratio [95% confidence interval (CI)]: 0.788 [0.762–0.855], 0.654 [0.604–0.708], and 0.579 [0.527–0.636], respectively). After propensity score weighting, consistent results were observed. The risk reduction of healthy lifestyle combinations was consistently observed in various subgroups, regardless of the CHA2DS2-VASc score and oral anticoagulant use.
Conclusion: Increased number of healthy lifestyle behaviors was significantly associated with lower MACE risk in patients with new-onset AF. These findings support the promotion of a healthy lifestyle to reduce the risk of adverse events in patients with AF.
Globally, the prevalence of atrial fibrillation (AF) is increasing with an aging population (1–3). AF is associated with an increased risk of stroke, heart failure, and death, and increases the overall healthcare burden (3–6). Therefore, the optimal management of AF, including AF burden reduction and stroke prevention, is crucial for improving outcomes and reducing the AF-related healthcare burden (7). Many studies have studied optimal oral anticoagulation treatment and better symptom care including rhythm and rate control in patients with AF; (8–10) however, lifestyle-related factors that play a role as modifiable risk factors in AF management are still generally underrecognized and understudied, especially in relation to clinical outcomes.
In previous studies, each component of unhealthy lifestyle behaviors such as smoking, alcohol consumption, and lack of regular exercise were individually associated with an increased risk of AF burden, thromboembolic events, and all-cause death (11–15). Although unhealthy or healthy lifestyle behaviors tend to be clustered, studies on the risk of clinical outcomes depending on how lifestyle behaviors are managed after AF diagnosis remain limited (16–19).
This study aimed to evaluate the clustering of healthy lifestyle behaviors in patients who were newly diagnosed with AF and the impact of such accumulation of multiple healthy lifestyle behaviors on the risk of AF-related adverse clinical outcomes.
The National Medical Claims Database, linked with the National Health Screening Examination Database established by the Korean National Health Insurance Service (NHIS) was used (20, 21). Briefly, the Korean NHIS provides universal, comprehensive, and mandatory medical coverage for the entire Korean population (approximately 50 million). The Korean NHIS database includes all the information about enrollees’ medical use, including demographic information, diagnoses, examinations, prescription records, procedures, and operations for inpatient and outpatient services. Diagnoses were coded based on the International Classification of Diseases, Tenth Revision, Clinical Modification codes. The Korean government provides a national health screening examination and recommends that all Korean adults receive examinations every 1 or 2 years. The health examination included anthropometric measurements, physical examinations, regular blood tests, and questionnaires on lifestyle behaviors and medical history.
Among the patients who were newly diagnosed with non-valvular AF between 1 January 2009 and 31 December 2016 (n = 576,077), we included patients who underwent a national health screening examination within 2 years after their AF diagnosis (n = 209,880) (Figure 1). After excluding patients aged < 20 years and those with missing values among health screening examinations, 208,662 patients were finally included in this analysis.
This study was exempt from review by the Seoul National University Hospital Institutional Review Board (E-2103-006-1200). All data and materials have been made publicly available at the National Health Insurance Sharing Service and can be accessed at http://nhiss.nhis.or.kr/bd/ab/bdaba000eng.do.
Lifestyle behaviors were assessed using self-reported questionnaires during health screening examinations. Each lifestyle was classified as follows: (1) smoking status as currently smoking or not; (2) alcohol consumption as a current drinker or non-drinker; and (3) regular exercise as performing moderate physical activity ≥ 5 times per week or vigorous physical activity ≥ 3 times per week and lack of regular exercise in the absence of regular exercise (19, 22). The frequency and intensity of physical activity were assessed using questionnaires. Moderate intensity of physical activity was defined as ≥ 30 min per day of brisk walking, dancing, or gardening, and vigorous intensity of physical activity was defined as ≥ 20 min per day of running fast, cycling, or aerobics (23).
To estimate the impact of each unhealthy lifestyle behavior on the risk of MACE, we evaluated the association between each unhealthy lifestyle behavior (with various dose or intensity) and the risk of MACE in the study population using multivariable Cox analysis (Supplementary Table 1). Based on the results of preliminary analyses, current smoker, current drinker, and non-regular exerciser were defined as patients with unhealthy lifestyle behaviors who were at significantly higher risk of MACE. Thus, in the present study, a healthy lifestyle behavior score (HLS) was calculated by assigning 1 point each for non-current smokers, non-drinkers, and performing regular exercise. The study population was categorized into four groups according to HLS from patients with HLS 0 who did not have any healthy lifestyle behaviors to patients with HLS 3 who met all three healthy lifestyle behaviors.
Demographic information including age and sex, comorbidities including hypertension, diabetes mellitus, dyslipidaemia, heart failure, prior ischemic stroke, prior myocardial infarction, peripheral artery disease, chronic obstructive pulmonary disease, cancer, and chronic kidney disease were assessed based on the diagnoses of medical claims and health screening examination database. The CHA2DS2-VASc score was calculated based on comorbidities (7). Detailed definitions of the comorbidities are presented in Supplementary Table 2 (2, 21, 24). Prescription records of claims database obtained medications including oral anticoagulants (warfarin or non-vitamin K antagonist oral anticoagulant), antiplatelet agents, and statins. Body mass index (BMI) was calculated as body weight in kilograms divided by the square of height in meters (kg/m2). Systolic and diastolic blood pressure, fasting glucose, and glomerular filtration rate were obtained from the health screening examination. Low income was defined as household income lower than 25%.
The primary outcome was the first occurrence of major adverse cardiovascular events (MACE), including ischemic stroke, myocardial infarction, and hospitalization for heart failure. Secondary outcomes included the individual components of MACE and all-cause deaths. Detailed definitions of the clinical outcomes are presented in Supplementary Table 2 (21, 24, 25). Patients were followed up starting from the index date (at the health screening examination date) until the occurrence of the study outcomes, death, or 31 December 2017 whichever came first.
Continuous variables were presented as mean and standard deviation and categorical variables were presented as number and percentage. Baseline characteristics across patient groups were compared using the Cochran-Armitage trend test for categorical variables and a linear trend test using a generalized linear model for continuous variables. The number of events was calculated as the incidence rate during the follow-up period divided by 100 person-years at risk. The risk of clinical outcomes with HLS was analyzed using the Cox proportional hazards regression model. The hazard ratio (HR) and 95% confidence intervals (CIs) for primary and secondary outcomes were analyzed using the HLS 0 group as the reference group. Model 1 was unadjusted; model 2 was adjusted for age and sex; and model 3 was further adjusted for hypertension, diabetes mellitus, dyslipidaemia, heart failure, prior ischemic stroke, prior myocardial infarction, peripheral artery disease, chronic obstructive pulmonary disease, cancer, chronic kidney disease, CHA2DS2-VASc score, use of oral anticoagulants, antiplatelet agents, and statins, BMI, and low income. The proportional hazards assumption was graphically evaluated with a log minus log graph and confirmed with Schoenfeld residuals for Cox models. Parallel log minus log survival curves and random patterns in Schoenfeld residuals were found, indicating no major deviation from the proportionality assumption, and the test was not statistically significant. The variance inflation factor (VIF) was used to assess multi-collinearity. Between the covariates, there was no significant collinearity (VIF = 1.005–2.492).
To provide complementary analyses for balancing among patient groups with different HLS, we performed inverse probability of treatment weighting (IPTW) using stabilized weights calculated from the propensity scores as a sensitivity analysis (26). The covariates included in model 3 were used for the propensity score calculation. We evaluated the maximum absolute standardized difference (ASD) of covariates to confirm the balance of the different groups. A maximum ASD of > 0.1 (10%) indicates an imbalance in a covariate (27, 28). The weighted incidence rate (per 100 person-years) and weighted cumulative incidence curves using the Kaplan–Meier method and log-rank test for primary and secondary outcomes were evaluated. The risk for primary and secondary outcomes of the different HLS groups was evaluated using weighted Cox proportional hazards models with IPTW. When the maximum ASD was > 0.1, the covariate was included in the Cox proportional hazard model for further adjustment.
For the primary outcome, subgroup analyses were performed for age (< 65, 65 to < 75, and ≥ 75 years), sex, CHA2DS2-VASc score (< 3 and ≥ 3), presence of prior history of ischemic stroke, and use of oral anticoagulants.
All analyses were two-tailed, and statistical significance was defined as P < 0.05. Statistical analyses were conducted using SAS (version 9.4; ASA Institute Inc., Cary, NC, United States).
A total of 208,662 patients with AF who were available for national health screening exam data were included in this analysis (Figure 1). The mean duration between AF diagnosis and the national health screening exam was 333 ± 203 days. The proportions of patients with 0, 1, 2, and 3 HLS were 7.1%, 22.7%, 58.6%, and 11.6%, respectively, and the baseline characteristics according to HLS are presented in Table 1. The baseline characteristics of the study population are summarized in Supplementary Table 3.
Patients in the HLS 0 group who had a cluster of three unhealthy lifestyles, including current smoking, current drinking, and lack of regular exercise, were younger, more likely to be men, had lower CHA2DS2-VASc scores, and had a lower prevalence of comorbidities such as hypertension, diabetes, dyslipidaemia, heart failure, prior ischemic stroke, prior myocardial infarction, peripheral artery disease, chronic obstructive pulmonary disease, cancer, and chronic kidney disease than those in the HLS 3 group who had a cluster of three healthy lifestyles. The HLS 0 group showed a lower proportion of patients with obesity (BMI ≥ 25 kg/m2) than the HLS 3 group. The proportion of patients receiving oral anticoagulation treatment was higher in the HLS 1, 2, and 3 groups than in the HLS 0 group.
During a median of 3.5-year follow-up (interquartile range 1.7–5.6), ischemic stroke, myocardial infarction, hospitalization for heart failure, MACE, and all-cause death occurred in 7,110 (3.4%), 1,460 (0.7%), 4,378 (2.1%), 12,298 (16.4%), and 18,318 (8.8%), respectively. Supplementary Table 4 and Figure 2 show the unadjusted and adjusted HRs for clinical outcomes according to HLS groups.
Figure 2. The risk of primary and secondary outcomes according to healthy lifestyle behavior scores: a multivariable-adjusted Cox analysis. Model 3 was adjusted for age, sex, hypertension, diabetes mellitus, dyslipidemia, heart failure, prior ischemic stroke, prior myocardial infarction, peripheral artery disease, chronic obstructive pulmonary disease, cancer, chronic kidney disease, CHA2DS2-VASc score, use of oral anticoagulant, antiplatelet agent, and statin, BMI, and low income. Major adverse cardiovascular events was defined as the composite outcomes of ischemic stroke, myocardial infarction, and hospitalization for heart failure. BMI, body mass index; CI, confidence interval; HR, hazard ratio.
After multivariable adjustment (model 3), patients with HLS 1, 2, and 3 were associated with a lower risk of MACE by 21%, 35%, and 42%, respectively, than those with HLS 0. Consistent results were observed for secondary outcomes. HLS 1, 2, and 3 groups were associated with lower risks of ischemic stroke by 15, 38, and 42%, respectively, compared to the HLS 0 group. The HLS 3 group showed a statistically significant risk reduction for myocardial infarction compared to the HLS 1 group. Compared to the HLS 0 group, the HLS 1, 2, and 3 groups were associated with lower risks of hospitalization for heart failure by 27%, 30%, and 41%, respectively. An increased number of healthy lifestyle behaviors was associated with a lower risk of all-cause death.
Since the baseline characteristics of each group stratified by HLS were significantly different, we performed a sensitivity analysis to compare HLS groups for the risk of clinical outcomes using the IPTW method. The baseline covariates were well balanced among the different HLS groups after IPTW (Table 1). Figure 3 and Supplementary Figure 1 reveal weighted Kaplan–Meier curves for clinical outcomes, and Figure 4 shows the weighted incidence rates and HRs for clinical outcomes after IPTW. The results were largely consistent with those of the multivariable Cox analysis (Model 3).
Figure 4. The risk or primary and secondary outcomes according to healthy lifestyle behavior scores: an IPTW analysis. Major adverse cardiovascular events was defined as the composite outcomes of ischemic stroke, myocardial infarction, and hospitalization for heart failure. CI, confidence interval; HR, hazard ratio; IPTW, inverse probability of treatment weighting; IR, incidence rate; PY, person-years.
The results of subgroup analyses were consistent with the main analysis. For the primary outcome, there was no significant interaction between each subgroup and the risk of MACE according to HLS (Table 2), except for the age subgroups. Although p for interaction was 0.019 in the age subgroup analyses and the HRs were slightly different in different age subgroups, the trends and directionality of HRs of HLS 1, 2, and 3 compared to HLS 0 were consistent in all age subgroups.
In this analysis of a large-scale nationwide population-based cohort study, we sought to determine whether the accumulation of healthy lifestyle behaviors was associated with a lower risk of MACE. Our principal findings were as follows: (1) although the study patients were newly diagnosed with AF, 7% of patients had a cluster of three unhealthy lifestyles, including current smoking, current drinking, and lack of regular exercise, whereas 12% of patients had all three healthy lifestyle behaviors; (2) healthy lifestyle behaviors as a composite score were associated with a substantially lower risk of MACE, including ischemic stroke, myocardial infarction, and hospitalization for heart failure, and all-cause death; and (3) there was an inverse dose-response relationship in which an increased number of healthy lifestyle behaviors were associated with lower risks of MACE.
Considering that healthy lifestyle factors are not isolated but often occur in a cluster with other healthy lifestyle factors, we suggest that an integrated approach to lifestyle modification is needed to improve the clinical outcomes in patients with AF.
Traditionally, the management of patients with AF has been particularly focused on the prevention of stroke with anticoagulation and control rate and rhythm for symptom relief. A more integrated care approach that addresses modifiable risk factors, including coexisting cardiovascular comorbidities and unhealthy lifestyle behaviors, has been promoted in recent guidelines (7, 29). A recent scientific statement from the American Heart Association comprehensively summarizes the modifiable risk factors for primary and secondary prevention of AF, similar to the statement from the European Heart Rhythm Association (29, 30). Moderate exercise, smoking cessation, and alcohol intake reduction are recommended to reduce the risk of AF occurrence and reduce the AF burden (29).
Several previous observational studies have established that healthy lifestyle behaviors are associated with reducing the AF burden and lower risks of adverse clinical outcomes (11, 12, 14, 15, 31, 32). Subsequently, well-designed randomized controlled trials (RCTs) have strengthened previous findings in observational studies (13, 33). Although an RCT could not be conducted for smoking, several observational studies have shown that smoking was associated with an increased risk of stroke and death, and smoking cessation after AF diagnosis might reduce the risk of stroke and MACE (11, 12, 32). Alcohol is a well-known risk factor for the development of AF (34) and is associated with an increased risk of thromboembolic events, including ischemic stroke and AF hospitalization (14). Indeed, a recent RCT further confirmed the impact of abstinence from alcohol on reducing AF recurrence and its burden (13). In patients with AF, performing regular exercise is associated with a lower risk of all-cause death (15). In addition, higher physical activity and cardiorespiratory fitness have been associated with a lower long-term risk of death from cardiovascular disease (a composite of myocardial infarction, heart failure, and stroke) and all-cause death (31). Of note, cardiorespiratory fitness was also closely related to AF recurrence risk, with a significant dose-response relationship (33).
It must be emphasized that much of the evidence in previous studies has focused on an isolated component of lifestyle behaviors (11–15, 31–33). However, healthy (or unhealthy) lifestyle behaviors tend to cluster (16–19, 35, 36). Two RCTs have shown that intensive implementation of integrated care for modifiable risk factors significantly reduced AF recurrence (37, 38). Aggressive risk factor management, including blood pressure control, weight management, lipid management, glycemic control, sleep-disordered breathing management, smoking cessation, and alcohol reduction by the physician-directed clinic after AF catheter ablation reduced AF recurrence and symptom severity with left atrial reverse remodeling (37). In patients with early persistent AF and mild-to-moderate heart failure, optimal medical therapy combined with cardiac rehabilitation, including physical activity, dietary restriction, and counseling, improves sinus rhythm maintenance (38). In these studies, the study population was perhaps more selected from the general AF population. The components of lifestyle intervention were slightly different from our study, and the primary outcome was AF recurrence, not a hard adverse clinical outcome.
According to our results (Supplementary Table 1), current smoking is the most powerful factor to be associated with a higher risk of MACE [adjusted HR 1.479, 95% confidence interval (CI) 1.393–1.571]. Followed by current smoking, current drinking was associated with a higher risk of MACE by 16–32% [adjusted HR 1.159 (95% CI 1.082–1.241), 1.261 (1.139–1.397), and 1.317 (1.183–1.466) for mild, moderate, and heavy drinker, respectively]. In the case of regular physical activity, although it depends on the degree (or amount) of exercise, lack of exercise was associated with a higher risk of MACE by 10–20%. In this analysis, we did not weight each lifestyle behavior due to primarily exploring the impact of the combination of unhealthy lifestyle behavior on the risk of MACE. Further study is needed to weight each lifestyle behavior by considering the dose-response relationship of each factor and develop a more sophisticated lifestyle score for predicting AF-related adverse.
In subgroup analyses, among relatively low-risk patients with CHA2DS2-VASc ≤ 3, a cluster of healthy lifestyle behaviors was associated with a significantly lower risk of MACE. In addition, the benefit of a healthy lifestyle cluster was consistently observed regardless of whether the patients were anticoagulated or not. According to a recent study, current smoking was a predictor for future ischemic stroke in low-risk patients who were not indicated oral anticoagulation (male with CHA2DS2-VASc 0 or female with CHA2DS2-VASc 1) (39). Hence, proactive lifestyle risk evaluation and promotion of a healthy lifestyle in the early period of AF diagnosis would also be beneficial to prevent stroke in low-risk patients who are not indicated for oral anticoagulation treatment immediately.
The European Hypertension Guidelines regarded AF as an equivalent of cardiovascular disease risk (40). Hence, the comprehensive management of AF patients should include not only oral anticoagulation therapy but also general control or cardiovascular risk factors and comorbidities (7). The time point of immediate after the new diagnosis of AF could be the best chance to promote healthy lifestyle behaviors, including smoking cessation, reducing alcohol consumption, and initiating regular exercise, to improve clinical outcomes and reduce AF-related adverse events in the future. Physician-directed proactive management of modifiable lifestyle risk factors should be more emphasized in a holistic approach to AF care, as recommended in the guidelines (7). Indeed, lifestyle optimization is the C of the ABC (Atrial fibrillation Better Care) pathway whereby ABC pathway compliant care has been shown in numerous studies to be associated with better clinical outcomes (15, 41–43).
This study included a large number of patients with incident AF. It comprehensively analyzed the association between an increased number of healthy lifestyle behaviors and the risk of MACE with sufficient statistical power. However, there are several limitations to this study. First, we classified healthy lifestyle behaviors using self-reported questionnaires. Although several studies have reported the association between lifestyle factors and adverse clinical outcomes using self-reported questionnaires, recall bias could be one of the major potential limitations (19, 22, 23, 31, 33). Second, although we performed multivariable adjustment and balanced the baseline characteristics of the different groups using IPTW, we cannot exclude the possibility of unmeasured confounding factors. For example, the types of AF, such as the paroxysmal and persistent, or the actual burden of AF at baseline could not be measured in this database. Third, changes in baseline variables during follow-up, including lifestyle factors and medication use, were not considered in this analysis. Lastly, in this study, covariates and study outcomes were defined based on the diagnostic codes, which could be affected by the physicians’ clinical practice, thus, might have resulted in an underestimation and overestimation. To overcome this limitation, we used a well-established and widely used operational definition in previous studies, or a validated definition through our own data (21, 44).
Increased number of healthy lifestyle behaviors, including quitting smoking, abstaining from alcohol consumption, and performing regular physical activity, were significantly associated with lower risks of MACE and all-cause death in patients with new-onset AF. These findings support the promotion of a healthy lifestyle and a more holistic or integrated approach to reduce the risk of adverse events in patients with AF.
Publicly available datasets were analyzed in this study. This data can be found here: http://nhiss.nhis.or.kr/bd/ab/bdaba000eng.do.
The studies involving human participants were reviewed and approved by Seoul National University Hospital Institutional Review Board. Written informed consent for participation was not required for this study in accordance with the national legislation and the institutional requirements.
S-RL, E-KC, and GL: conceptualization. S-WL and K-DH: methodology and data curation. K-DH: software and resources. S-RL, S-WL, S-HP, and E-KC: validation. S-WL: formal analysis. S-RL: investigation, writing—original draft preparation, and visualization. S-RL, S-HP, E-KC, and GL: writing—review and editing. SO: supervision. E-KC: project administration and funding acquisition. All authors have read and agreed to the published version of the manuscript.
This work was supported by the Korea Medical Device Development Fund grant funded by the Korea Government (the Ministry of Science and ICT, the Ministry of Trade, Industry and Energy, the Ministry of Health and Welfare, Republic of Korea, the Ministry of Food and Drug Safety) (Project Number: 202013B14), and by the Korea National Research Foundation funded by the Ministry of Education, Science and Technology (grant: 2020R1F1A106740).
E-KC: research grants or speaking fees from Bayer, BMS/Pfizer, Biosense Webster, Chong Kun Dang, Daiichi-Sankyo, Dreamtech Co., Ltd., Medtronic, Samjinpharm, Sanofi-Aventis, Seers Technology, Skylabs, and Yuhan. GL: consultant and speaker for BMS/Pfizer, Boehringer Ingelheim and Daiichi-Sankyo, but no fees are received personally; no other relationships or activities that could appear to have influenced the submitted work.
The remaining authors declare that the research was conducted in the absence of any commercial or financial relationships that could be construed as a potential conflict of interest.
All claims expressed in this article are solely those of the authors and do not necessarily represent those of their affiliated organizations, or those of the publisher, the editors and the reviewers. Any product that may be evaluated in this article, or claim that may be made by its manufacturer, is not guaranteed or endorsed by the publisher.
The Supplementary Material for this article can be found online at: https://www.frontiersin.org/articles/10.3389/fcvm.2022.885016/full#supplementary-material
AF, atrial fibrillation; ASD, absolute standardized difference; CI, confidence interval; HLS, healthy lifestyle behavior score; HR, hazard ratio; IPTW, inverse probability of treatment weighting; MACE, major adverse cardiovascular event; NHIS, National Health Insurance Service.
1. Kornej J, Borschel CS, Benjamin EJ, Schnabel RB. Epidemiology of atrial fibrillation in the 21st century: novel methods and new insights. Circ Res. (2020) 127:4–20. doi: 10.1161/CIRCRESAHA.120.316340
2. Lee SR, Choi EK, Han KD, Cha MJ, Oh S. Trends in the incidence and prevalence of atrial fibrillation and estimated thromboembolic risk using the CHA2DS2-VASc score in the entire Korean population. Int J Cardiol. (2017) 236:226–31. doi: 10.1016/j.ijcard.2017.02.039
3. Chao TF, Liu CJ, Tuan TC, Chen TJ, Hsieh MH, Lip GYH, et al. Lifetime risks, projected numbers, and adverse outcomes in asian patients with atrial fibrillation: a report from the Taiwan nationwide af cohort study. Chest. (2018) 153:453–66. doi: 10.1016/j.chest.2017.10.001
4. Wolf PA, Abbott RD, Kannel WB. Atrial fibrillation as an independent risk factor for stroke: the Framingham study. Stroke. (1991) 22:983–8.
5. Benjamin EJ, Wolf PA, D’Agostino RB, Silbershatz H, Kannel WB, Levy D. Impact of atrial fibrillation on the risk of death: the Framingham heart study. Circulation. (1998) 98:946–52.
6. Patel NJ, Deshmukh A, Pant S, Singh V, Patel N, Arora S, et al. Contemporary trends of hospitalization for atrial fibrillation in the United States, 2000 through 2010: implications for healthcare planning. Circulation. (2014) 129:2371–9. doi: 10.1161/CIRCULATIONAHA.114.008201
7. Hindricks G, Potpara T, Dagres N, Arbelo E, Bax JJ, Blomstrom-Lundqvist C, et al. 2020 ESC Guidelines for the diagnosis and management of atrial fibrillation developed in collaboration with the European association for cardio-thoracic surgery (EACTS): the task force for the diagnosis and management of atrial fibrillation of the European Society of Cardiology (ESC) developed with the special contribution of the European heart rhythm association (EHRA) of the ESC. Eur Heart J. (2021) 42:373–498.
8. Ruff CT, Giugliano RP, Braunwald E, Hoffman EB, Deenadayalu N, Ezekowitz MD, et al. Comparison of the efficacy and safety of new oral anticoagulants with warfarin in patients with atrial fibrillation: a meta-analysis of randomised trials. Lancet. (2014) 383:955–62.
9. Chao TF, Chiang CE, Lin YJ, Chang SL, Lo LW, Hu YF, et al. Evolving changes of the use of oral anticoagulants and outcomes in patients with newly diagnosed atrial fibrillation in Taiwan. Circulation. (2018) 138:1485–7. doi: 10.1161/CIRCULATIONAHA.118.036046
10. Kirchhof P, Camm AJ, Goette A, Brandes A, Eckardt L, Elvan A, et al. Early rhythm-control therapy in patients with atrial fibrillation. N Engl J Med. (2020) 383:1305–16.
11. Lip GY, Frison L, Halperin JL, Lane DA. Identifying patients at high risk for stroke despite anticoagulation: a comparison of contemporary stroke risk stratification schemes in an anticoagulated atrial fibrillation cohort. Stroke. (2010) 41:2731–8. doi: 10.1161/STROKEAHA.110.590257
12. Albertsen IE, Rasmussen LH, Lane DA, Overvad TF, Skjoth F, Overvad K, et al. The impact of smoking on thromboembolism and mortality in patients with incident atrial fibrillation: insights from the danish diet, Cancer, and health study. Chest. (2014) 145:559–66. doi: 10.1378/chest.13-1740
13. Voskoboinik A, Kalman JM, De Silva A, Nicholls T, Costello B, Nanayakkara S, et al. Alcohol Abstinence in Drinkers with Atrial Fibrillation. N Engl J Med. (2020) 382:20–8.
14. Lim C, Kim TH, Yu HT, Lee SR, Cha MJ, Lee JM, et al. Effect of alcohol consumption on the risk of adverse events in atrial fibrillation: from the COmparison study of Drugs for symptom control and complication prEvention of Atrial Fibrillation (CODE-AF) registry. Europace. (2021) 23:548–56. doi: 10.1093/europace/euaa340
15. Proietti M, Boriani G, Laroche C, Diemberger I, Popescu MI, Rasmussen LH, et al. Self-reported physical activity and major adverse events in patients with atrial fibrillation: a report from the EURObservational research programme pilot survey on atrial fibrillation (EORP-AF) general registry. Europace. (2017) 19:535–43. doi: 10.1093/europace/euw150
16. Schuit AJ, van Loon AJ, Tijhuis M, Ocke M. Clustering of lifestyle risk factors in a general adult population. Prev Med. (2002) 35:219–24. doi: 10.1006/pmed.2002.1064
17. Poortinga W. The prevalence and clustering of four major lifestyle risk factors in an English adult population. Prev Med. (2007) 44:124–8. doi: 10.1016/j.ypmed.2006.10.006
18. Li C, Ford ES, Mokdad AH, Jiles R, Giles WH. Clustering of multiple healthy lifestyle habits and health-related quality of life among U.S. adults with diabetes. Diabetes Care. (2007) 30:1770–6. doi: 10.2337/dc06-2571
19. Lee SR, Choi EK, Ahn HJ, Han KD, Oh S, Lip GYH. Association between clustering of unhealthy lifestyle factors and risk of new-onset atrial fibrillation: a nationwide population-based study. Sci Rep. (2020) 10:19224. doi: 10.1038/s41598-020-75822-y
20. Cheol Seong S, Kim YY, Khang YH, Heon Park J, Kang HJ, Lee H, et al. Data resource profile: the national health information database of the national health insurance service in South Korea. Int J Epidemiol. (2017) 46:799–800.
21. Choi EK. Cardiovascular research using the korean national health information database. Korean Circ J. (2020) 50:754–72.
22. Kim MK, Han K, Park YM, Kwon HS, Kang G, Yoon KH, et al. Associations of variability in blood pressure, glucose and cholesterol concentrations, and body mass index with mortality and cardiovascular outcomes in the general population. Circulation. (2018) 138:2627–37.
23. Kim K, Choi S, Hwang SE, Son JS, Lee JK, Oh J, et al. Changes in exercise frequency and cardiovascular outcomes in older adults. Eur Heart J. (2020) 41:1490–9.
24. Lee SR, Choi EK, Kwon S, Han KD, Jung JH, Cha MJ, et al. Effectiveness and safety of contemporary oral anticoagulants among asians with nonvalvular atrial fibrillation. Stroke. (2019) 50:2245–9. doi: 10.1161/STROKEAHA.119.025536
25. Kim D, Yang PS, Kim TH, Jang E, Shin H, Kim HY, et al. Ideal blood pressure in patients with atrial fibrillation. J Am Coll Cardiol. (2018) 72:1233–45.
26. Austin PC, Stuart EA. Moving towards best practice when using inverse probability of treatment weighting (IPTW) using the propensity score to estimate causal treatment effects in observational studies. Stat Med. (2015) 34:3661–79. doi: 10.1002/sim.6607
27. Austin PC. Balance diagnostics for comparing the distribution of baseline covariates between treatment groups in propensity-score matched samples. Stat Med. (2009) 28:3083–107. doi: 10.1002/sim.3697
28. Larsen TB, Skjoth F, Nielsen PB, Kjaeldgaard JN, Lip GY. Comparative effectiveness and safety of non-vitamin K antagonist oral anticoagulants and warfarin in patients with atrial fibrillation: propensity weighted nationwide cohort study. BMJ. (2016) 353:i3189. doi: 10.1136/bmj.i3189
29. Chung MK, Eckhardt LL, Chen LY, Ahmed HM, Gopinathannair R, Joglar JA, et al. Lifestyle and risk factor modification for reduction of atrial fibrillation: a scientific statement from the american heart association. Circulation. (2020) 141:e750–72.
30. Gorenek B, Pelliccia A, Benjamin EJ, Boriani G, Crijns HJ, Fogel RI, et al. European Heart Rhythm Association (EHRA)/European Association of Cardiovascular Prevention and Rehabilitation (EACPR) position paper on how to prevent atrial fibrillation endorsed by the Heart Rhythm Society (HRS) and Asia Pacific Heart Rhythm Society (APHRS). Europace. (2017) 19:190–225.
31. Garnvik LE, Malmo V, Janszky I, Ellekjaer H, Wisloff U, Loennechen JP, et al. Physical activity, cardiorespiratory fitness, and cardiovascular outcomes in individuals with atrial fibrillation: the HUNT study. Eur Heart J. (2020) 41:1467–75.
32. Choi S, Chang J, Kim K, Kim SM, Koo HY, Cho MH, et al. Association of smoking cessation after atrial fibrillation diagnosis on the risk of cardiovascular disease: a cohort study of South Korean men. BMC Public Health. (2020) 20:168. doi: 10.1186/s12889-020-8275-y
33. Pathak RK, Elliott A, Middeldorp ME, Meredith M, Mehta AB, Mahajan R, et al. Impact of CARDIOrespiratory fitness on arrhythmia recurrence in obese individuals with atrial fibrillation: the CARDIO-FIT study. J Am Coll Cardiol. (2015) 66:985–96. doi: 10.1016/j.jacc.2015.06.488
34. Kim YG, Han KD, Choi JI, Boo KY, Kim DY, Lee KN, et al. Frequent drinking is a more important risk factor for new-onset atrial fibrillation than binge drinking: a nationwide population-based study. Europace. (2020) 22:216–24. doi: 10.1093/europace/euz256
35. Samieri C, Perier MC, Gaye B, Proust-Lima C, Helmer C, Dartigues JF, et al. Association of cardiovascular health level in older age with cognitive decline and incident dementia. JAMA. (2018) 320:657–64.
36. Bonaccio M, Di Castelnuovo A, Costanzo S, De Curtis A, Persichillo M, Cerletti C, et al. Impact of combined healthy lifestyle factors on survival in an adult general population and in high-risk groups: prospective results from the Moli-sani study. J Intern Med. (2019) 286:207–20. doi: 10.1111/joim.12907
37. Pathak RK, Middeldorp ME, Lau DH, Mehta AB, Mahajan R, Twomey D, et al. Aggressive risk factor reduction study for atrial fibrillation and implications for the outcome of ablation: the ARREST-AF cohort study. J Am Coll Cardiol. (2014) 64:2222–31. doi: 10.1016/j.jacc.2014.09.028
38. Rienstra M, Hobbelt AH, Alings M, Tijssen JGP, Smit MD, Brugemann J, et al. Targeted therapy of underlying conditions improves sinus rhythm maintenance in patients with persistent atrial fibrillation: results of the RACE 3 trial. Eur Heart J. (2018) 39:2987–96.
39. Kwon S, Kim TJ, Choi EK, Ahn HJ, Lee E, Lee SR, et al. Predictors of ischemic stroke for low-risk patients with atrial fibrillation: a matched case-control study. Heart Rhythm. (2021) 18:702–8.
40. Williams B, Mancia G, Spiering W, Agabiti Rosei E, Azizi M, Burnier M, et al. 2018 ESC/ESH Guidelines for the management of arterial hypertension. Eur Heart J. (2018) 39:3021–104.
41. Yoon M, Yang PS, Jang E, Yu HT, Kim TH, Uhm JS, et al. improved population-based clinical outcomes of patients with atrial fibrillation by compliance with the simple ABC (Atrial Fibrillation Better Care) pathway for integrated care management: a nationwide cohort study. Thromb Haemost. (2019) 119:1695–703. doi: 10.1055/s-0039-1693516
42. Proietti M, Romiti GF, Olshansky B, Lane DA, Lip GYH. Comprehensive management with the ABC (Atrial Fibrillation Better Care) pathway in clinically complex patients with atrial fibrillation: a post hoc ancillary analysis from the AFFIRM trial. J Am Heart Assoc. (2020) 9:e014932. doi: 10.1161/JAHA.119.014932
43. Guo Y, Lane DA, Wang L, Zhang H, Wang H, Zhang W, et al. Mobile health technology to improve care for patients with atrial fibrillation. J Am Coll Cardiol. (2020) 75:1523–34.
Keywords: atrial fibrillation, lifestyle, stroke, myocardial infarction, heart failure
Citation: Lee S-R, Choi E-K, Park S-H, Lee S-W, Han K-D, Oh S and Lip GYH (2022) Clustering of Unhealthy Lifestyle and the Risk of Adverse Events in Patients With Atrial Fibrillation. Front. Cardiovasc. Med. 9:885016. doi: 10.3389/fcvm.2022.885016
Received: 27 February 2022; Accepted: 16 June 2022;
Published: 04 July 2022.
Edited by:
Shaojie Chen, Cardioangiological Center Bethanien (CCB), GermanyReviewed by:
Bert Vandenberk, University of Calgary, CanadaCopyright © 2022 Lee, Choi, Park, Lee, Han, Oh and Lip. This is an open-access article distributed under the terms of the Creative Commons Attribution License (CC BY). The use, distribution or reproduction in other forums is permitted, provided the original author(s) and the copyright owner(s) are credited and that the original publication in this journal is cited, in accordance with accepted academic practice. No use, distribution or reproduction is permitted which does not comply with these terms.
*Correspondence: Eue-Keun Choi, Y2hvaWVrMTdAc251LmFjLmty
Disclaimer: All claims expressed in this article are solely those of the authors and do not necessarily represent those of their affiliated organizations, or those of the publisher, the editors and the reviewers. Any product that may be evaluated in this article or claim that may be made by its manufacturer is not guaranteed or endorsed by the publisher.
Research integrity at Frontiers
Learn more about the work of our research integrity team to safeguard the quality of each article we publish.