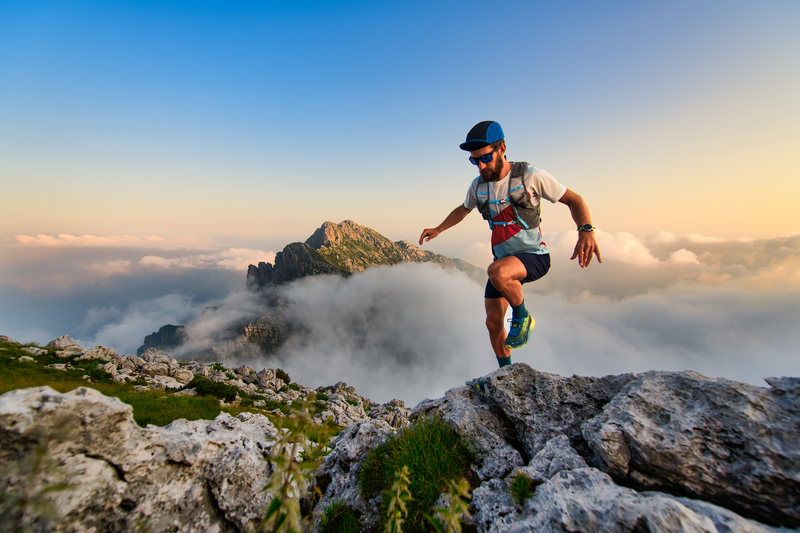
95% of researchers rate our articles as excellent or good
Learn more about the work of our research integrity team to safeguard the quality of each article we publish.
Find out more
ORIGINAL RESEARCH article
Front. Cardiovasc. Med. , 19 April 2022
Sec. Coronary Artery Disease
Volume 9 - 2022 | https://doi.org/10.3389/fcvm.2022.867646
This article is part of the Research Topic Highlights in Coronary Artery Disease: 2021 View all 8 articles
Background: Chronic coronary syndrome (CCS) is a newly proposed concept and is hallmarked by more long-term major adverse cardiovascular events (MACEs), calling for accurate prognostic biomarkers for initial risk stratification.
Methods: Data-independent acquisition liquid chromatography tandem mass spectrometry (DIA LC-MS/MS) quantitative proteomics was performed on 38 patients with CCS; 19 in the CCS events group and 19 in the non-events group as the controls. We also developed a machine-learning-based pipeline to identify proteins as potential biomarkers and validated the target proteins by enzyme-linked immunosorbent assay in an independent prospective cohort.
Results: Fifty-seven differentially expressed proteins were identified by quantitative proteomics and three final biomarkers were preliminarily selected from the machine-learning-based pipeline. Further validation with the prospective cohort showed that endothelial protein C receptor (EPCR) and cholesteryl ester transfer protein (CETP) levels at admission were significantly higher in the CCS events group than they were in the non-events group, whereas the carboxypeptidase B2 (CPB2) level was similar in the two groups. In the Cox survival analysis, EPCR and CETP were independent risk factors for MACEs. We constructed a new prognostic model by combining the Framingham coronary heart disease (CHD) risk model with EPCR and CETP levels. This new model significantly improved the C-statistics for MACE prediction compared with that of the Framingham CHD risk model alone.
Conclusion: Plasma proteomics was used to find biomarkers of predicting MACEs in patients with CCS. EPCR and CETP were identified as promising prognostic biomarkers for CCS.
The Guidelines for chronic coronary syndrome (CCS) were announced at the annual meeting of the European Society of Cardiology (ESC) in August 2019 (1). The ESC updated the “Guidelines for Treatment of Stable Coronary Artery Diseases (SCAD)” released in 2013 (2) and defined the concept of CCS. This changed the previous inherent concept of SCAD and reflected the current deeper understanding of the pathophysiological mechanism of coronary artery disease. Traditionally, the term SCAD was used to describe CCS which often shaped the disease as “stable.” Although CCS is often “relatively stable” compared with acute coronary artery disease, the underlying pathophysiological state can become “unstable” at any time, causing plaque rupture or erosion, leading to acute thrombosis. Therefore, the risk of future cardiovascular events in patients with CCS can be different; hence, predicting major adverse cardiovascular events (MACEs) will have great clinical significance for patients with CCS.
The purpose of genomics is to collectively characterize and quantify all genes, and transcriptomics refers to the study of gene transcription in cells and the regulation of transcription regulation at the overall level. However, in recent years, many researchers have found that the results for proteins are often not highly consistent with those for genomes or transcriptomes (3). This is partly because the products of transcription or translation are usually metabolized or modified, thereby changing the downstream protein abundance (4). Proteins are key regulators of many biological processes, and are directly related to the occurrence of many diseases and their clinical prognosis (5). Proteomics approaches have become increasingly mature, from the discovery of a single biomarker for early disease to the comprehensive characterization of protein abundance profiles of specific diseases. Indeed, some diseases are affected by more than one biological pathway. The advent of high-throughput proteomics has made research on such processes possible, and the study of differentially expressed proteins (DEPs) has provided insights into the molecular mechanisms of many human diseases (6–8). Currently, liquid chromatography tandem mass spectrometry (LC-MS/MS) is the main tool used to analyze whole proteomes, and it has been applied in studies of cardiovascular diseases, including the recently redefined CCS.
In this study, we performed a proteomics analysis to discover potential biomarkers of CCS, used machine learning methods to screen the identified biomarkers (9, 10), and validated the selected biomarkers in an independent prospective cohort. Two proteins were identified as new biomarkers for predicting the risk of adverse cardiovascular events in patients with CCS.
The overall design of this study is shown in Figure 1. The discovery cohort was a retrospective cohort. We selected 38 patients who had undergone a physical examination conducted at the People’s Liberation Army (PLA) General Hospital from April to July 2015. The inclusion criteria were: (1) patients who were asymptomatic or had stable symptoms within 1 year after onset of acute coronary syndromes; (2) patients who were stable more than 1 year after initial diagnosis or revascularization regardless of symptoms; (3) patients with angina pectoris, suspected vasospasm, or microvascular disease; and (4) Males aged more than 65 years old. The exclusion criteria were: (1) patients with severe heart failure; (2) patients with CHD in the acute phase; and (3) patients with other diseases that made them unsuitable for this study. According to whether MACEs (including cardiovascular related death, non-fatal myocardial infarction, unstable angina, and heart failure) occurred until June 2019, the participants were divided into a CCS events group (Group A, n = 19) as the cases and a non-events group (Group B, n = 19) as the controls for the proteomics analysis. The validation cohort was an independent prospective cohort that included 352 patients who were recruited from those who had undergone a routine physical examination at the PLA General Hospital from April to July 2017. The inclusion and exclusion criteria were the same as those for the discovery cohort above. This cohort was followed up until April 2021. All methods were carried out in accordance with relevant guidelines and regulations. All experimental protocols were approved by Ethics Board of the Chinese PLA General Hospital. All informed consent was obtained from all subjects and/or their legal guardian(s).
Plasma samples were collected after fasting 12 h. The samples were stored at −80°C with ethylenediaminetetraacetate until analysis.
Quantitative proteomics analysis was performed using liquid chromatography tandem mass spectrometry (LC-MS/MS) to identify potential protein biomarker candidates among those proteins differing in abundance between event group and non-event group.
We used an integrated approach involving data-independent acquisition (DIA) strategy, HPLC fractionation to quantify the dynamic changes of the whole proteome.
Firstly, the cellular debris of plasma sample was removed by centrifugation at 12,000 g at 4°C for 10 min. Then, the supernatant was transferred to a new centrifuge tube. The top 12 high abundance proteins were removed by Pierce™ Top 12 Abundant Protein Depletion Spin Columns Kit (Thermo Fisher). Finally, the protein concentration was determined with BCA kit according to the manufacturer’s instructions.
For digestion, the protein solution was reduced with 5 mM dithiothreitol for 30 min at 56°C and alkylated with 11 mM iodoacetamide for 15 min at room temperature in darkness. The protein sample was then diluted by adding 100 mM TEAB to urea concentration less than 2 M. Finally, trypsin was added at 1:50 trypsin-to-protein mass ratio for the first digestion overnight and 1:100 trypsin-to-protein mass ratio for a second 4 h-digestion.
The tryptic peptides were fractionated into fractions by high pH reverse-phase HPLC using Agilent 300Extend C18 column (5 μm particles, 4.6 mm ID, 250 mm length). Briefly, peptides were first separated with a gradient of 8–32% acetonitrile (pH 9.0) over 60 min into 60 fractions. Then, the peptides were combined into 18 fractions and dried by vacuum centrifuging.
The iRT kit was added to all the samples according to manufacturer’s instructions. The tryptic peptides were dissolved in solvent A (0.1% formic acid, 2% acetonitrile), directly loaded onto a home-made reversed-phase analytical column (25-cm length, 100 μm i.d.). Peptides were separated with a gradient from 4 to 32% solvent B (0.1% formic acid in 90% acetonitrile) over 114 min, and climbing to 80% in 3 min then holding at 80% for the last 3 min, all at a constant flowrate of 450 nL/min on an EASY-nLC 1200 UPLC system (Thermo Fisher Scientific). The separated peptides were analyzed in DDA mode by Q Exactiveis™ HF-X (Thermo Fisher Scientific) with a nano-electrospray ion source.
The separated peptides were analyzed in Q Exactive™ HF-X (Thermo Fisher Scientific) with a nano-electrospray ion source. The full MS scan resolution was set to 120,000 for a scan range of 385–1200 m/z. The data acquisition was performed in DIA mode. Each cycle contains one full scan followed by 70 DIA MS/MS scans with predefined precursor m/z range. The higher-energy collisional dissociation (HCD) fragmentation was performed at a normalized collision energy (NCE) of 27%. The fragments were detected in the Orbitrap at a resolution of 15,000. Fixed first mass was set as 200 m/z. Automatic gain control (AGC) target was set at 5E5.
Spectral library generation: the resulting DDA data were processed using MaxQuant search engine (v.1.6.6.0). Tandem mass spectra were searched against the human SwissProt database (20,387 entries) concatenated with reverse decoy database. Trypsin/P was specified as cleavage enzyme allowing up to 2 missing cleavages. The mass tolerance for precursor ions was set as 20 ppm in First search and 4.5 ppm in Main search, and the mass tolerance for fragment ions was set as 0.02 Da. Carbamidomethyl on Cys was specified as fixed modification. Acetylation on protein N-terminal and oxidation on Met were specified as variable modifications. FDR was adjusted to <1%. The false discovery rates of the PSMs and proteins were set to less than 1%.
Data-independent acquisition data analysis: all DIA data were analyzed in Skyline (v 4.1.0). The DDA search results were imported to Skyline to generate the spectral library, and the retention times were aligned to iRT reference values. Transition settings: precursor charges were set as 2, 3, 4, 5, ion charges were set as 1, 2. The ion match tolerance was set as 0.02 Da. Six most intense fragment ions from the spectral library were selected for each precursor. Decoy generation was based on shuffled sequences, and the FDR was estimated with the mProphet approach and set to 1%. Relative quantification of proteins was performed using MSstats package.
For the Gene Ontology (GO) and Kyoto Encyclopedia of Genes and Genomes (KEGG) analyses, we used the two-tailed Fisher’s exact test to determine the significance of the functional enrichment of the DEPs against all the identified proteins. A corrected p-value < 0.05 was considered significant.
We used three machine learning classification algorithms, Logistic Regression, Support Vector Machine, and Random Forest, as the base classifiers (Supplementary Figure 1). On the basis of these classifiers, we built a voting classifier. When a new sample had to be assigned to a category, each base classifier was used to predict the probability that the new sample belonged to a particular category. The final classification result was determined by the weighted value of the predicted probability of each category by all three base classifiers. This is an integrated method that used the Voting Classifier model in Python.
Each sample was represented by a feature vector composed of numerous expression data. To quantify the ability of these expression features to distinguish different samples, we performed univariate feature analysis using a variance test to calculate the correlation between each feature and the sample category one by one. In this way, the ability of each feature to distinguish the sample category was obtained and the score and the corresponding p-value was calculated. The expression features were sorted according to the calculated p-value, and used in the subsequent analysis.
To compare the difference between the category predicted by the model and the actual sample category, we calculated an accuracy index using the Matthews coefficient value as an indicator of the accuracy of the predictive power of the model as
where, TP, TN, FP, and FN are true positive, true negative, false positive, and false negative, respectively.
We plotted the calculated accuracy index results against the number of features in the expression feature subset as the incremental feature selection curve. When the Matthews coefficient reached the maximum value, we considered the expression feature subset corresponding to the model as the optimal expression feature subset.
The expression feature subset and sample category were used as input of the Voting Classifier model, and 10-fold cross-validation was used to calculate the prediction accuracy of the local optimal expression feature subset for a sample. The results were expressed by the receiver operating characteristic curve (ROC). This is a dynamic validation that reduced the impact of data partitioning.
Data are presented as numbers and frequencies for categorical variables and as means ± SD for continuous variables. Baseline characteristics were compared using the Chi-square test for categorical variables and analysis of variance test for continuous variables. The effect of the candidate biomarkers was evaluated using a Cox proportional hazards model, and p-values were calculated using the log-rank test. The ROC was compared using a z-test (DeLong’s method) between the classic Framingham CHD risk model alone and the Framingham CHD model combined with the candidate biomarkers EPCR and CETP. The Framingham CHD model included age, sex, total cholesterol, high-density lipoprotein cholesterol (HDL-C), systolic blood pressure, current smoking, and diabetes status as confounding factors. p-Value of <0.05 was considered to indicate statistically significant difference for all the analyses. The statistical analyses were performed using SPSS (version 23.0), STATA (version 12.0), and MedCalc Software.
The datasets generated and/or analyzed during the current study are available in the PRIDE repository.1 Project ID: PXD029473.
The baseline clinical data of the discovery and validation cohorts are shown in Table 1. The discovery cohort comprised 19 cases (including 2 cardiovascular death, 4 non-fatal myocardial infarction, 12 unstable angina, and 1 heart failure) and 19 controls, with an average follow-up time of 47.6 months. In the validation cohort, after an average of 41.6 months of follow-up, 86 patients (24.3%) had MACEs (including 9 cardiovascular death, 12 non-fatal myocardial infarction, 57 unstable angina, and 8 heart failure). Age, fibrinogen, D-dimer, glycosylated hemoglobin, and NT-proBNP (N-terminal-pro-brain natriuretic peptide) were significantly higher in the events group than they were in the non-events group. Current smoking, diastolic blood pressure, and HDL-C were significantly higher in the non-events group than they were in the events group.
Quality control results for the proteomics data are shown in Supplementary Figure 2. The length distribution showed that 70% of the identified peptides were 7–20 amino acids long, which is consistent with the general rules of trypsin enzymatic hydrolysis and HCD. Peptides shorter than 5 amino acids cannot be effectively identified, and peptides longer than 20 amino acids are not suitable for HCD because of their high mass and charge. The distribution of peptides per protein showed that there were more than two peptides for most proteins. In general, proteins that have multiple corresponding specific peptides increase the precision and accuracy of the quantification results. The mass distribution of the proteins showed that the mass was relatively well-distributed, indicating that there was no significant molecular weight bias for proteins during sample preparation. These results confirm that the overall proteomics data meet the quality control requirements.
A total of 5,480 peptides were identified in the mass spectrum. By comparing the peptides back to proteins, we identified a total of 1,120 proteins, of which 783 were quantified.
We used the proteomic data to identify signatures of MACEs by analyzing the plasma proteins that underwent significant fold changes (FCs) between Group A and Group B (FC > 1.2 or FC < 0.8; unpaired two-sided Welch’s t-test; p < 0.05). As shown in the volcano map (Supplementary Figure 3), a total of 57 DEPs were identified under this condition.
The DEPs were annotated with GO terms and assigned KEGG pathways for the functional enrichment analyses (Figure 2). The highly enriched processes included cellular lipid metabolic process, acylglycerol transport, inflammatory response, response to bacterium, glycerolipid metabolism, hypertrophic cardiomyopathy, complement and coagulation cascades, and PI3K-Akt signaling pathway. These analyses pinpointed specific pathways (lipid metabolic, coagulation, and inflammation) that may operate in the events group compared with the non-events group, and highlighted CCS-related pathways for further functional investigation.
We used the plasma proteomic data of the discovery cohort and developed a series of algorithms to identify potential biomarker combinations to classify CCS cases. The feature analysis was used to rank the expression features based on scores and p-values, as shown in Supplementary Figure 4. To visualize the ranking results of the protein expression characteristics, we plotted a ranking histogram of the top 30 features with the highest scores (Supplementary Figure 5).
The incremental feature selection curve (Supplementary Figure 6) shows that when the top eight ranked features were selected, the Matthews coefficient value of the model reached the maximum for the first time. These eight features were analyzed further. Next, we selected the protein combinations with the highest area under the curve (AUC) value from the 10-fold cross-validation. The ROC curve (Figure 3) showed that the AUC of the first six proteins in the final trained model were >0.8. These six proteins were identified as candidate proteins and the detailed information is shown in Supplementary Table 1. The distribution of these six proteins between the events and non-events groups was significantly different as shown in the box plots in Supplementary Figure 7.
By combining the results of the DEP selection, GO and KEGG pathway analyses, machine learning, previous knowledge and clinical relevance, we selected three proteins EPCR (Q9UNN8), CPB2 (Q96IY4), and CETP (P11597) as the target proteins for validation. The expression levels of the three proteins are shown in Supplementary Figure 8. EPCR and CETP levels at admission were significantly higher in the CCS events group than in the control non-events group, whereas the CPB2 levels were similar. The Cox survival analysis showed that EPCR and CETP were risk factors for MACEs (Table 2). After correcting for the confounding factors in the Framingham CHD risk model, EPCR and CETP were still found to be independent risk factors for MACEs. Additionally, a new prognostic model was constructed by combining the Framingham CHD risk model with the candidate biomarkers EPCR and CETP. This new model significantly improved the C-statistics for MACE prediction compared with that of the Framingham CHD risk model alone (AUC 0.732 vs. 0.684, p < 0.05) (Figure 4). These results showed that EPCR and CETP were independent risk factors for MACEs in patients with CCS, and combined with the classic Framingham model, EPCR and CETP provided better prediction metrics than the Framingham model alone.
We performed a series of studies on the plasma proteins of patients with CCS, followed by global proteomic mass spectrometry identification, machine learning-based selection of biomarkers, and enzyme-linked immunosorbent assay (ELISA) for prospective validation of expanded samples. First, we used discovery mass spectrometry to quantify thousands of different proteins without the need for previous knowledge, and thus identify proteins not previously associated with CCS. After protein relative quantification between the CCS events and non-events groups, the classification power was evaluated by machine learning-based selection. On the basis of the results and clinical relevance, we identified three proteins, endothelial protein C receptor (EPCR), carboxypeptidase B2 (CPB2), and cholesteryl ester transfer protein (CETP) as candidate biomarkers. Finally, in the independent validation cohort, we found that EPCR and CETP were better than CPB2 for predicting MACEs in patients with CCS by ELISA and, when combined with the Framingham coronary heart disease (CHD) risk model, they improved the risk prediction beyond the Framingham CHD risk model alone.
Cardiovascular disease is one of the leading causes of death worldwide (11). One of the main risks of developing cardiovascular disease is vascular endothelial dysfunction (12). In a healthy state, endothelial cells maintain a balanced hemostatic state by producing procoagulants and anticoagulants, as well as proinflammatory and anti-inflammatory cytokines. In the disease state, endothelial cells are activated and exert procoagulant and proinflammatory effects. Endothelial cell dysfunction leads to thrombosis and coagulation imbalance (13). The new international guidelines consider that CHD is a dynamic process of atherosclerotic plaque accumulation and changes in coronary circulatory function. Plaques can show the following trends: gradually increased instability or even rupture, stability maintained for a long time, and gradual shrinking. The composition of plaques also continues to change. However, about one-third of patients with a cardiovascular disease have angina pectoris, but have no obstructive coronary artery disease (14). Therefore, patients with CCS can have a relatively stable period, but the relatively stable vascular environment and circulatory function may become unstable because of inflammatory reactions, vulnerable plaques, and abnormal lipid metabolism (15). Therefore, “stability” is only temporary and relative, not absolute. The clinical evaluation and management of such seemingly “stable” patients with CCS is of great significance to improve the prognosis. In this study, we found EPCR and CETP were closely related to vascular endothelial homeostasis.
There are two variants of EPCR: mEPCR (membrane EPCR), which is present on endothelial cell membranes, and soluble EPCR (soluble EPCR), which circulates in the blood (16). Protein C is a vitamin K-dependent serine protease that is synthesized mainly in the liver and circulates in the plasma. Protein C binds to mEPCR with high affinity, and is converted to activated protein C (APC) by the thrombin–thrombomodulin complex on the surface of endothelial cells through a limited proteolytic process (17). The mEPCR variant can bind to APC and plays important roles in anticoagulation, anti-inflammatory, cell protection (anti-apoptosis), protecting endothelial barrier function, and promoting neovascularization (18–22). When activated by the thrombin–thrombomodulin complex, APC dissociates from the membrane-bound receptor mEPCR, and functions as an anticoagulant by inactivating coagulation factors Va and VIIIa (23). When APC is combined with mEPCR, it shows strong anti-inflammatory and cytoprotective activities. The cytoprotective signal activity of APC is mediated by protease activated receptor 1 (PAR1) on endothelial cells bound by mEPCR (24). The APC–mEPCR complex relies on the anti-inflammatory activity of PAR1 to mediate the inhibition of inflammatory gene expression, including c-Fos and FosB, which belong to the activator protein 1 (AP-1) family. Protective signals can also inhibit the release of inflammatory cytokines (such as IL-1β, IL-6, and tumor necrosis factor-α) and the nuclear translocation of NF-κB, and downregulate the expression of genes that encode endothelial cell adhesion proteins (such as ICAM1, VCAM1, and E-selectin), thereby restricting the penetration of white blood cells through the vascular system, protecting the endothelial barrier function, and inhibiting inflammation (25). Conversely, the sEPCR variant detaches from the cell membrane surface through shedding and enters the circulation. Possible reasons for shedding include a systemic inflammatory response and vascular endothelial damage (26). The sEPCR plays a negative competitive role in blood circulation. It binds protein C and APC with similar affinity and inhibits protein C activation on the endothelium. It also inhibits the anticoagulant activity of APC by blocking the binding of APC to phospholipids. It is the sEPCR variant in blood circulation that was tested in this study. Elevated levels of sEPCR can disturb vascular homeostasis, promote coagulation, aggravate inflammation, and accelerate endothelial cell apoptosis, which is associated with increased risk of thrombosis (27).
Cholesteryl ester transfer protein is a plasma protein secreted by the liver. It is one of the most effective endogenous regulators of plasma HDL-C, which protects the cardiovascular system in many ways (28). It can promote the transfer of cholesterol ester from HDL-C to apolipoprotein B (ApoB). In addition to removing excess cholesterol from the arterial wall, HDL-C can also inhibit lipid oxidation, restore endothelial function, and exert anti-inflammatory and anti-apoptotic effects (29, 30). CETP leads to a net reduction of HDL-C in plasma, which increases the risk of atherosclerosis development. Some large randomized controlled trials have studied the effects of CETP inhibitors on lipid metabolism and MACEs, but the results are inconsistent (31–33). Possible reasons include incomplete function of the CETP inhibitors and side effects of drugs. A study explored whether CETP was related to atherosclerosis through its role in HDL-C and low-density lipoprotein metabolism. In a case–control study of 50 patients with coronary atherosclerosis and 50 controls, no significant difference was detected in the lipid profiles between the two groups, even though the serum CETP level of the case group was significantly higher than that of control group (34). This finding indicated that CETP may have atherogenic effects. However, no further studies have reported the long-term adverse prognosis risk of CHD. In our study, we included both case–control and prospective cohorts, which more fully illustrated the relationship between CETP and the poor prognosis of patients with CCS.
On the other hand, several studies are designed to provide the genomic or metabolomic background of CHD (35–37). All these studies, once combined together, might provide essential evidence for the efficient risk-stratification of patients with CHD.
To our knowledge, this is the first study to evaluate the relationship between plasma proteomics and the prognosis of patients with CCS. This study was layered involving three different methods, and the results are accurate and reliable. In addition, we analyzed more cases with longer follow-up times than most of the other studies. Our study has some limitations. First, the discovery and validation cohorts were from a single center and had relatively fewer older adult female patients. Therefore, to reduce bias, only male patients were included in this study. Second, we identified 57 DEPs that could predict the occurrence of MACEs, which may provide a rich biomarker pool for CCS, but further refinement of the diagnostic biomarkers is needed. Third, whether EPCR and CETP will act as prognostic predictors of CCS caused by other etiologies needs further study.
We performed plasma proteomics analysis to find biomarkers for predicting MACEs in patients with CCS. We identified EPCR and CETP as independent risk factors for MACEs. The Framingham CHD risk model combined with EPCR and CETP was found to be a high-performance prognostic model for CCS. EPCR and CETP may be associated with vascular homeostasis, involving lipid metabolism, and inflammatory, coagulation, and cell protection processes. Further investigations are needed to understand the specific mechanisms involved.
The datasets presented in this study can be found in online repositories. The names of the repository/repositories and accession number(s) can be found in the article/Supplementary Material.
The studies involving human participants were reviewed and approved by the Ethics Board of the Chinese PLA General Hospital. The patients/participants provided their written informed consent to participate in this study.
Y-LC and H-BL designed the protocol. Y-LC drafted the manuscript. H-BL supervised the patient recruitment and study procedures. Y-LC, B-CH, J-QC, and Y-RL conducted the study procedures. All authors carried out study procedures, critically revised the manuscript for important intellectual content, and approved the final manuscript.
This work was supported by the National Key Research Program of China (2020YFC2008304).
The authors declare that the research was conducted in the absence of any commercial or financial relationships that could be construed as a potential conflict of interest.
All claims expressed in this article are solely those of the authors and do not necessarily represent those of their affiliated organizations, or those of the publisher, the editors and the reviewers. Any product that may be evaluated in this article, or claim that may be made by its manufacturer, is not guaranteed or endorsed by the publisher.
The Supplementary Material for this article can be found online at: https://www.frontiersin.org/articles/10.3389/fcvm.2022.867646/full#supplementary-material
1. Knuuti J, Wijns W, Saraste A, Capodanno D, Barbato E, Funck-Brentano C, et al. 2019 ESC Guidelines for the diagnosis and management of chronic coronary syndromes. Eur Heart J. (2020) 41:407–77.
2. Task Force M, Montalescot G, Sechtem U, Achenbach S, Andreotti F, Arden C, et al. 2013 ESC guidelines on the management of stable coronary artery disease: the task force on the management of stable coronary artery disease of the European Society of Cardiology. Eur Heart J. (2013) 34:2949–3003. doi: 10.1093/eurheartj/eht296
3. Zhang B, Wang J, Wang X, Zhu J, Liu Q, Shi Z, et al. Proteogenomic characterization of human colon and rectal cancer. Nature. (2014) 513:382–7.
4. Zhang H, Liu T, Zhang Z, Payne SH, Zhang B, McDermott JE, et al. Integrated proteogenomic characterization of human high-grade serous ovarian cancer. Cell. (2016) 166:755–65. doi: 10.1016/j.cell.2016.05.069
5. Papaioannou MD, Djuric U, Kao J, Karimi S, Zadeh G, Aldape K, et al. Proteomic analysis of meningiomas reveals clinically distinct molecular patterns. Neuro Oncol. (2019) 21:1028–38. doi: 10.1093/neuonc/noz084
6. Djomehri SI, Gonzalez ME, da Veiga Leprevost F, Tekula SR, Chang HY, White MJ, et al. Quantitative proteomic landscape of metaplastic breast carcinoma pathological subtypes and their relationship to triple-negative tumors. Nat Commun. (2020) 11:1723. doi: 10.1038/s41467-020-15283-z
7. Shu T, Ning W, Wu D, Xu J, Han Q, Huang M, et al. Plasma proteomics identify biomarkers and pathogenesis of COVID-19. Immunity. (2020) 53:1108–1122.e5. doi: 10.1016/j.immuni.2020.10.008
8. Wu D, Zhang S, Xie Z, Chen E, Rao Q, Liu X, et al. Plasminogen as a prognostic biomarker for HBV-related acute-on-chronic liver failure. J Clin Invest. (2020) 130:2069–80. doi: 10.1172/JCI130197
9. Chua W, Purmah Y, Cardoso VR, Gkoutos GV, Tull SP, Neculau G, et al. Data-driven discovery and validation of circulating blood-based biomarkers associated with prevalent atrial fibrillation. Eur Heart J. (2019) 40:1268–76.
10. Hoshino A, Kim HS, Bojmar L, Gyan KE, Cioffi M, Hernandez J, et al. Extracellular Vesicle and Particle Biomarkers Define Multiple Human Cancers. Cell. (2020) 182:1044–1061.e18. doi: 10.1016/j.cell.2020.07.009
11. Balakumar P, Maung UK, Jagadeesh G. Prevalence and prevention of cardiovascular disease and diabetes mellitus. Pharmacol Res. (2016) 113:600–9.
12. Zhang P, Liu Y, Su J, Bai J, Zhao S, Zhao S. Resistin impairs activation of protein C by suppressing EPCR and increasing SP1 expression. Biomed Pharmacother. (2019) 109:930–7. doi: 10.1016/j.biopha.2018.09.160
13. Nagareddy P, Smyth SS. Inflammation and thrombosis in cardiovascular disease. Curr Opin Hematol. (2013) 20:457–63. doi: 10.1097/moh.0b013e328364219d
14. Ford TJ, Corcoran D, Berry C. Stable coronary syndromes: pathophysiology, diagnostic advances and therapeutic need. Heart. (2018) 104:284–92.
15. van Diepen JA, Berbee JF, Havekes LM, Rensen PC. Interactions between inflammation and lipid metabolism: relevance for efficacy of anti-inflammatory drugs in the treatment of atherosclerosis. Atherosclerosis. (2013) 228:306–15. doi: 10.1016/j.atherosclerosis.2013.02.028
16. Ku SK, Han MS, Lee MY, Lee YM, Bae JS. Inhibitory effects of oroxylin A on endothelial protein C receptor shedding in vitro and in vivo. BMB Rep. (2014) 47:336–41. doi: 10.5483/bmbrep.2014.47.6.198
17. Sriwastva MK, Kunjunni R, Andrabi M, Prasad K, Saxena R, Subbiah V. Neuroprotective effects of activated protein C involve the PARP/AIF pathway against oxygen-glucose deprivation in SH-SY5Y cells. Brain Sci. (2020) 10:959.
18. Minhas N, Xue M, Jackson CJ. Activated protein C binds directly to Tie2: possible beneficial effects on endothelial barrier function. Cell Mol Life Sci. (2017) 74:1895–906. doi: 10.1007/s00018-016-2440-6
19. Xue M, Dervish S, Chan B, Jackson CJ. The endothelial protein C receptor is a potential stem cell marker for epidermal keratinocytes. Stem Cells. (2017) 35:1786–98. doi: 10.1002/stem.2630
20. Catieau B, Devos V, Chtourou S, Borgel D, Plantier JL. Endothelial cell surface limits coagulation without modulating the antithrombin potency. Thromb Res. (2018) 167:88–95. doi: 10.1016/j.thromres.2018.05.019
21. Shavit Stein E, Ben Shimon M, Artan Furman A, Golderman V, Chapman J, Maggio N. Thrombin inhibition reduces the expression of brain inflammation markers upon systemic LPS treatment. Neural Plast. (2018) 2018:7692182.
22. Wang H, Wang P, Liang X, Li W, Yang M, Ma J, et al. Down-regulation of endothelial protein C receptor promotes preeclampsia by affecting actin polymerization. J Cell Mol Med. (2020) 24:3370–83. doi: 10.1111/jcmm.15011
23. Lopez-Ramirez MA, Pham A, Girard R, Wyseure T, Hale P, Yamashita A, et al. Cerebral cavernous malformations form an anticoagulant vascular domain in humans and mice. Blood. (2019) 133:193–204.
24. Kondreddy V, Wang J, Keshava S, Esmon CT, Rao LVM, Pendurthi UR. Factor VIIa induces anti-inflammatory signaling via EPCR and PAR1. Blood. (2018) 131:2379–92. doi: 10.1182/blood-2017-10-813527
25. Healy LD, Fernandez JA, Mosnier LO, Griffin JH. Activated protein C and PAR1-derived and PAR3-derived peptides are anti-inflammatory by suppressing macrophage NLRP3 inflammasomes. J Thromb Haemost. (2021) 19:269–80. doi: 10.1111/jth.15133
26. Lecuyer H, Virion Z, Barnier JP, Matczak S, Bourdoulous S, Bianchini E, et al. An ADAM-10 dependent EPCR shedding links meningococcal interaction with endothelial cells to purpura fulminans. PLoS Pathog. (2018) 14:e1006981. doi: 10.1371/journal.ppat.1006981
27. Tanalp AC, Oduncu V, Erkol A, Gozubuyuk G, Ozveren O, Dundar C, et al. Soluble endothelial protein C receptor levels and protein C activity in patients with acute ST-segment elevation myocardial infarction. Coron Artery Dis. (2013) 24:209–16. doi: 10.1097/MCA.0b013e32835e5c45
28. Kontush A. HDL-mediated mechanisms of protection in cardiovascular disease. Cardiovasc Res. (2014) 103:341–9. doi: 10.1093/cvr/cvu147
29. Chapman MJ, Le Goff W, Guerin M, Kontush A. Cholesteryl ester transfer protein: at the heart of the action of lipid-modulating therapy with statins, fibrates, niacin, and cholesteryl ester transfer protein inhibitors. Eur Heart J. (2010) 31:149–64. doi: 10.1093/eurheartj/ehp399
30. Kettunen J, Holmes MV, Allara E, Anufrieva O, Ohukainen P, Oliver-Williams C, et al. Lipoprotein signatures of cholesteryl ester transfer protein and HMG-CoA reductase inhibition. PLoS Biol. (2019) 17:e3000572.
31. Lincoff AM, Nicholls SJ, Riesmeyer JS, Barter PJ, Brewer HB, Fox KAA, et al. Evacetrapib and cardiovascular outcomes in high-risk vascular disease. N Engl J Med. (2017) 376:1933–42.
32. Tall AR, Rader DJ. Trials and tribulations of CETP inhibitors. Circ Res. (2018) 122:106–12. doi: 10.1161/CIRCRESAHA.117.311978
33. Armitage J, Holmes MV, Preiss D. Cholesteryl ester transfer protein inhibition for preventing cardiovascular events: JACC review topic of the week. J Am Coll Cardiol. (2019) 73:477–87. doi: 10.1016/j.jacc.2018.10.072
34. Devi A, Singh R, Dawar R, Tyagi S. Association of cholesteryl ester transfer protein (CETP) gene -629C/A polymorphism with angiographically proven atherosclerosis. Indian J Clin Biochem. (2017) 32:235–8. doi: 10.1007/s12291-016-0585-6
35. Vizirianakis IS, Chatzopoulou F, Papazoglou AS, Karagiannidis E, Sofidis G, Stalikas N, et al. The GEnetic Syntax Score: a genetic risk assessment implementation tool grading the complexity of coronary artery disease-rationale and design of the GESS study. BMC Cardiovasc Disord. (2021) 21:284. doi: 10.1186/s12872-021-02092-5
36. Guo N, Wang P, Yang J, Yang X, van der Voet M, Wildwater M, et al. Serum metabolomic analysis of coronary heart disease patients with stable angina pectoris subtyped by traditional Chinese medicine diagnostics reveals biomarkers relevant to personalized treatments. Front Pharmacol. (2021) 12:664320. doi: 10.3389/fphar.2021.664320
Keywords: proteomics, chronic coronary syndrome (CCS), prognosis, aging, geriatrics
Citation: Cai Y-L, Hao B-C, Chen J-Q, Li Y-R and Liu H-B (2022) Correlation Between Plasma Proteomics and Adverse Outcomes Among Older Men With Chronic Coronary Syndrome. Front. Cardiovasc. Med. 9:867646. doi: 10.3389/fcvm.2022.867646
Received: 01 February 2022; Accepted: 23 March 2022;
Published: 19 April 2022.
Edited by:
Serban Puricel, Fribourg – Cantonal Hospital, SwitzerlandReviewed by:
Rita Pavasini, University Hospital of Ferrara, ItalyCopyright © 2022 Cai, Hao, Chen, Li and Liu. This is an open-access article distributed under the terms of the Creative Commons Attribution License (CC BY). The use, distribution or reproduction in other forums is permitted, provided the original author(s) and the copyright owner(s) are credited and that the original publication in this journal is cited, in accordance with accepted academic practice. No use, distribution or reproduction is permitted which does not comply with these terms.
*Correspondence: Hong-Bin Liu, bGl1aGJpbjMwMUAxNjMuY29t
†These authors share first authorship
Disclaimer: All claims expressed in this article are solely those of the authors and do not necessarily represent those of their affiliated organizations, or those of the publisher, the editors and the reviewers. Any product that may be evaluated in this article or claim that may be made by its manufacturer is not guaranteed or endorsed by the publisher.
Research integrity at Frontiers
Learn more about the work of our research integrity team to safeguard the quality of each article we publish.