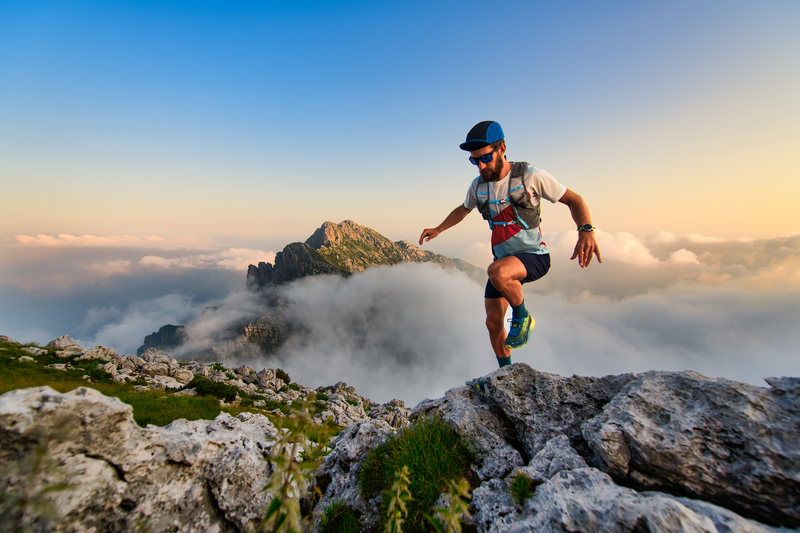
95% of researchers rate our articles as excellent or good
Learn more about the work of our research integrity team to safeguard the quality of each article we publish.
Find out more
ORIGINAL RESEARCH article
Front. Cardiovasc. Med. , 22 April 2022
Sec. Cardiac Rhythmology
Volume 9 - 2022 | https://doi.org/10.3389/fcvm.2022.863988
This article is part of the Research Topic Women in Cardiac Rhythmology View all 8 articles
Background: As people age, circulating levels of insulin-like growth factors (IGFs) and IGF binding protein 3 (IGFBP-3) decline. In rat cardiomyocytes, IGF-I has been shown to regulate sarcolemmal potassium channel activity and late sodium current thus impacting cardiac repolarization and the heart rate-corrected QT (QTc). However, the relationship between IGFs and IGFBP-3 with the QTc interval in humans, is unknown.
Objectives: To examine the association of IGFs and IGFBP-3 with QTc interval in an older age population-based cohort.
Methods: Participants were from the 1946 Medical Research Council (MRC) National Survey of Health and Development (NSHD) British birth cohort. Biomarkers from blood samples at age 53 and 60–64 years (y, exposures) included IGF-I/II, IGFBP-3, IGF-I/IGFBP-3 ratio and the change (Δ) in marker levels between the 60–64 and 53y sampled timepoints. QTc (outcome) was recorded from electrocardiograms at the 60–64y timepoint. Generalized linear multivariable models with adjustments for relevant demographic and clinical factors, were used for complete-cases and repeated after multiple imputation.
Results: One thousand four hundred forty-eight participants were included (48.3% men; QTc mean 414 ms interquartile range 26 ms). Univariate analysis revealed an association between low IGF-I and IGF-I/IGFBP-3 ratio at 60–64y with QTc prolongation [respectively: β −0.30 ms/nmol/L, (95% confidence intervals −0.44, −0.17), p < 0.001; β−28.9 ms/unit (-41.93, −15.50), p < 0.001], but not with IGF-II or IGFBP-3. No association with QTc was found for IGF biomarkers sampled at 53y, however both ΔIGF-I and ΔIGF-I/IGFBP-3 ratio were negatively associated with QTc [β −0.04 ms/nmol/L (−0.08, −0.008), p = 0.019; β −2.44 ms/unit (-4.17, −0.67), p = 0.007] while ΔIGF-II and ΔIGFBP-3 showed no association. In fully adjusted complete case and imputed models (reporting latter) low IGF-I and IGF-I/IGFBP-3 ratio at 60–64y [β −0.21 ms/nmol/L (−0.39, −0.04), p = 0.017; β −20.14 ms/unit (−36.28, −3.99), p = 0.015], steeper decline in ΔIGF-I [β −0.05 ms/nmol/L/10 years (−0.10, −0.002), p = 0.042] and shallower rise in ΔIGF-I/IGFBP-3 ratio over a decade [β −2.16 ms/unit/10 years (−4.23, −0.09), p = 0.041], were all independently associated with QTc prolongation. Independent associations with QTc were also confirmed for other previously known covariates: female sex [β 9.65 ms (6.65, 12.65), p < 0.001], increased left ventricular mass [β 0.04 ms/g (0.02, 0.06), p < 0.001] and blood potassium levels [β −5.70 ms/mmol/L (−10.23, −1.18) p = 0.014].
Conclusion: Over a decade, in an older age population-based cohort, declining levels and bioavailability of IGF-I associate with prolongation of the QTc interval. As QTc prolongation associates with increased risk for sudden death even in apparently healthy people, further research into the antiarrhythmic effects of IGF-I on cardiomyocytes is warranted.
The QT interval on a 12-lead echocardiogram (ECG) represents the time taken by ventricular cardiomyocytes to depolarize and repolarize. A prolonged QT interval, and especially the T-wave onset to T-peak (1), is thought to result from alterations in sympathetic and parasympathetic activity as well as several other risk factors. Since QTc is a measure of ventricular depolarization and repolarization, having a longer than normal QTc interval risks inducing early afterdepolarizations, and possibly also re-entrant excitation and, torsade de pointes (2–4) ultimately leading to ventricular arrhythmias and ventricular fibrillation (5). Prolongation of the heart rate corrected QT (QTc) is a well-established risk factor for increased cardiovascular mortality (5, 6), all-cause mortality and morbidity (7), even in apparently healthy people (8). Although many of the factors associated with QTc prolongation have been identified, including female sex (9, 10), hypokalemia (11), left ventricular hypertrophy (12), hypertension (13), drug side effects (2) and genetics (14, 15), there are still several unknowns. There is now a need to incorporate metabolic biomarkers in our research to understand the pathophysiology of QTc prolongation.
IGF-I regulates somatic growth, reaching its highest levels during teenage years, with levels decreasing with age (16, 17) and it is involved in cell proliferation, protein synthesis, nutrient homeostasis and nervous system, liver, kidney and cardiac development (18, 19). The decline in IGF-I seems to be greater with higher fat mass (16). IGF-I is also influenced by other hormones, age, sex, diet and nutrition. Previous studies in older age cohorts showed that reduced levels of IGF-I increase the risk of ischemic heart disease and cardiovascular mortality (18, 20). Animal work has shown that insulin like growth factor-I (IGF-I) may influence cardiac repolarization, via the phosphatidyl inositol-3 kinase/protein kinase B (PI3-K/Akt) pathway in cardiomyocytes (21). The PI3-K pathway directly regulates most of the heart's ion channels, including the rapid delayed rectifier potassium channel that specifically influences cardiac repolarization (22) and in some animal models, consequently the QTc duration (23). PI3-K was shown to affect many of the channels involved in the action potential duration, late sodium current, calcium current and slow delayed rectifier potassium channels, through its downstream signaling (22). Yet, little is known about the relationship between IGF-I and QTc duration in humans, particularly in older persons, in whom IGF-I levels are known to decline (24). The insulin growth factor-family (IGFs), including IGF-I and IGF-II, has a wide range of physiological functions including the regulation of cellular proliferation, apoptosis, protein synthesis and metabolism (25). IGF-I in the circulation is bound to insulin like growth factor binding protein-3 (IGFBP-3) and therefore the molar ratio between IGF-I and IGFBP-3 indicates IGF-I bioavailability (26).
We sought to investigate the association between circulating blood levels of IGFs and IGFBP-3 with cardiac repolarization represented by the QTc interval in older age participants of a population-based longitudinal cohort.
Participants were from the Medical Research Council (MRC) National Survey of Health and Development (NSHD), a birth cohort study comprised of 5,362 individuals born in 1 week in 1946 in Britain. The cohort has been in continuous follow up since birth, with 24 data collection cycles (27). Briefly, the cohort has been evaluated multi-dimensionally: anthropometrically, socio-economically (manual and non-manual), and in terms of life-style choices (e.g., smoking) and health function (e.g., mental health, cardiovascular and respiratory function) (27). A consort diagram summarizing the recruitment process for the current study is presented in Figure 1. Previous studies in the NSHD cohort have shown that use of SEP at age 53 as a surrogate for SEP at age 60–64 years is both justifiable and sound, first, because the majority of participants were retired by the age of 60 implying no significant SEP shifts between time-points, and second, because it provided a means to backfill the otherwise high SEP missingness in the cohort at age 60–64 (28, 29).
Figure 1. Consort diagram of the recruitment process. The Medical Research Council National Survey of Health and Development (NSHD) consists of 5,362 individuals recruited in 1 week in March 1946 in Britain. The exposures of interest here were metabolic markers at 60–64 years while the outcome was QTc interval derived from resting 12-lead electrocardiography (ECG) during the same clinic visit. Both pieces of data were available for 1,513 out of the 5,362 participants. The number of participants involved int the study is presented in the figure below. ECG, electrocardiography; IGF-I, insulin-like growth factor 1; IGF-BP3, insulin-like growth factor binding protein 3.
The 2006–2010 NSHD data collection sweep included an in-depth cardiovascular assessment and was granted ethical approval from the Greater Manchester Local Research Ethics Committee and the Scotland Research Ethics Committee (27) and written informed consent was given by all study participants. All procedures performed were in accordance with the ethical standards of our institutional and/or national research ethics committees and conformed to the 1964 Helsinki declaration and its later amendments or comparable ethical standards.
Between 2006 and 2010 when study members were 60–64 years (y), British-based NSHD participants who had not been lost to follow-up or withdrawn, were invited to attend a clinic-based assessment that included a 12-lead resting surface ECG for measurement of QT and R-R intervals using validated computerized algorithms (27). QTc was calculated using Hodges' formula (30):
Those with severe conduction system disease requiring a permanent pacemaker and those with any type of a cardiac implantable electronic device (n=8), atrial fibrillation (n=54) or taking anti-arrhythmic medications (n=14) at this period were excluded.
Blood samples were collected at age 53y (non-fasting), and age 60–64y (fasting), stored at −80°C and assayed together. IGF-I and IGF-II and IGFBP-3 concentrations were obtained by radioimmunoassay using standard protocols in the same laboratory, as previously described (31). The intra- and inter-assay coefficients of variation for IGF-I, IGF-II, and IGFBP-3 have been previously reported (16, 32). IGF-I, IGF-II, and IGFBP-3 values were converted from ng/mL to standard SI units (nmol/L) (33, 34) considering: 1 ng/ml IGF-I= 0 .130 nmol/L IGF-I; 1 ng/ml IGF-II = 0.134 nmol/L IGF-II; and 1 ng/ml IGFBP-3 = 0.036 nmol/L IGFBP-3. IGF-I/IGFBP-3 values were expressed as molar ratios to indicate IGF-I bioavailability. In order to standardize individual biomarkers' deviation (Delta, Δ) over the ~10-year period, i.e., from their respective initial 53y timepoint concentrations, the serum concentration at the 60–64y timepoint was divided by its 53y concentration, as used in previous studies (35), then multiplied by the year difference between sampled timepoints divided by the maximum possible year difference, and finally multiplied by 100 to obtain a Δ marker score (Equation 1).
Covariates were selected a priori, based on previous studies and added into our models successively, after centering on age, to help with the interpretation of coefficients. Model 1 adjusted for sex; Model 2 included additional adjustments for socioeconomic position (SEP); Model 3 added clinical covariates known to be associated with QTc; and Model 4 added cardiac covariates known to be associated with QTc. The same models were used for all the IGF/IGFBP-3 biomarkers, at both time points, including ΔIGFs.
The sex of participants was recorded as male or female. Participants' SEP was evaluated using occupational data from 1989, when they were of active working age, according to the UK Office of Population Censuses and Surveys Registrar General's social class classification and dichotomized into manual or non-manual. Participants' weight and height were used to compute body mass index (BMI). Information about medication usage relevant to the QTc, including antibiotics, antihypertensives, antipsychotics and tricyclic antidepressants (88) was collected through survey instruments and self-reporting along with other relevant clinical information i.e., history of diabetes, heart disease (capturing ischemic heart disease, myocardial infarction, heart failure, heart rhythm abnormality, congenital heart disease, rheumatic heart disease, and other cardiovascular diseases), hypertension, alcohol intake (g per week), physical activity (counting any case of self-reported physical activity carried out over the preceding 4 weeks and 12 months), and smoking into never, ex- or current as previously described (36–38). Additional blood investigations carried out on samples at 60–64y included glucose, glycated hemoglobin A1c, total cholesterol, high- and low-density lipoprotein cholesterol, triglycerides and electrolytes. Assay details have been reported previously (39, 40). At the same clinic visit, two-dimensional transthoracic echocardiography was performed to measure left ventricular ejection fraction and mass (41).
Statistical analysis was performed in R Studio version 4.0.2 (RStudio Team 2020). Distribution of data was assessed visually using Q-Q plots, histograms and the Shapiro-Wilk test. Continuous sample variables are expressed as mean ± 1 standard deviation (SD) or median (interquartile range) as appropriate; categorical sample variables, as counts and percent. Paired biomarker differences across the decade were investigated by the paired Wilcoxon rank sum test.
As a result of the skewed distribution of continuous QT parameters, generalized linear models (glm) with a gamma distribution and log link were used to investigate the association of IGFs with QTc interval. Model assumptions were verified with regression diagnostics. Multi-collinearity between final model variables was excluded by demonstrating variance inflation factors <3. To determine whether the associations of IGFs and IGFBP-3 with QTc differed by sex or by heart disease, interaction terms for sex and heart disease were tested at the 10% significance level for all exposures and no interactions were found to justify stratification by either sex or heart disease. To account for data missingness, we used multiple imputation to generate missing covariates and re-ran the multivariable models. The predictive mean matching multiple imputation model using chained equations (42) included all the exposures, covariates and outcomes from the fully adjusted multivariable models, and generated 5 sets of covariates. Regression coefficient estimates, and their associated variance metrics were calculated for each dataset and combined using the Rubin's rule. Strength of evidence for an association was assessed on the basis of the size of the regression coefficients, their confidence interval (CI) and the p-value after controlling the false discovery rate at 5%. All tests were 2 sided; p < 0.05 was considered statistically significant and no adjustment was made for multiple testing.
We ran sensitivity analyses on the imputed models in which we re-analyzed the association between IGFs and IGFBP-3 with QTc after removing participants with known cardiovascular disease, and in which we additionally adjust for heart rate to avoid any remaining confounding.
Of the 5,362 originally enrolled into NSHD, 747 were deceased, 570 had emigrated, 853 had withdrawn and 530 were not contactable, leaving 2,662 that were successfully interviewed between 2006 and 2010. Of these 1,513 had contemporaneous ECG for QTc (outcome) and blood tests for the necessary IGFs/IGFBP-3 biomarkers (exposures). A final sample of 1,448 suitable for analysis remained after excluding participants with a device, atrial fibrillation or on anti-arrhythmics. Of these 1,261, 1,261 and 1,257 participants had repeat measures (at 53y and 60–64y) available for IGF-I, IGF-II and IGFBP-3, respectively. Clinicodemographic characteristics of study participants are presented in Table 1. The population mean QTc was 414 ms (IQR 402–428 ms), with 48.3% being male. Sixty-three male participants (9%) had a QTc prolongation, by definition, (>440 ms) compared to 42 female participants (5.6%; QTc >450 ms) (43), acknowledging the fact that QTc prolongation within the normal range can still be associated with cardiac arrhythmia. Participants with longer QTc intervals had higher BMI, lower resting heart rate, blood potassium, and circulating levels of IGF-I. Missing data for each covariate per exposure-outcome pair are presented in Supplementary Tables 1, 2.
On univariate analysis (Table 2) none of the IGF variables at 53y was significantly associated with a prolonged QTc interval a decade later (60–64y).
On univariate analysis (Table 2) low IGF-I and low IGF-I/IGFBP-3 molar ratio showed an association with QTc prolongation at 60–64y (β −0.30 ms/nmol/L [−0.44, −0.17], p < 0.001 and β −28.9 [−41.93, −15.50], p < 0.001), but IGF-II and IGFBP-3 alone showed no association with QTc. After multivariable adjustment in imputed models, low IGF-I (β −0.21 ms/nmol/L [−0.39, −0.04] p = 0.017; representing a multiplicative increase in QTc duration of ≈0.81 ms (exp[-0.21]) per 1 ms/nmol/L decrease in serum IGF-I levels), and low IGF -I/IGFBP-3 molar ratio at 60–64y (β −20.14 ms/unit [−36.28, −3.99] p = 0.015) were independently associated with QTc (Table 3). Complete case analysis support these inferences (Table 4).
Table 3. Multivariable imputed model exploring the association between IGF-I at 60-64y, IGF-I/IGFBP-3 molar ratio at 60-64y, ΔIGF-I and ΔIGF-I/IGFBP-3 ratio with QTc outcomes (only fully adjusted results for Model 4 are shown here).
Table 4. Multivariable regression (complete case analysis) for IGF-I at 60-64y, IGF-I/BP3 molar ratio at 60-64y, ΔIGF-I, ΔIGF-I/BP3 and exposures of interest with QTc.
Based on these multiple imputation models, independent associations with QTc were also confirmed for other previously known covariates: female sex (β 9.65 [6.65, 12.65] p < 0.001), increased left ventricular mass (β 0.04 ms/g [0.02, 0.06] p < 0.001) and blood potassium levels (β −5.70 ms/mmol/L [−10.23, −1.18] p = 0.014).
IGF-I, IGF-II and IGFBP-3 levels decreased with age (all p < 0.001; Figure 2) while the IGF-I/IGFBP-3 ratio increased with age (p < 0.001; Figure 2).
Figure 2. Boxplots comparing circulating levels of IGF-I, IGF-II, IGFBP-3 and IGF-I/IGFBP-3 molar ratio at 53 and 60–64 years. Whiskers indicate variability outside the third and first quartiles [75th and 25th percentiles] represented as hinges around the median [bold midline]. p-Values derived from Mann-Whitney tests. ECG, electrocardiography; IGF-I, insulin-like growth factor 1; IGF-BP3, insulin-like growth factor binding protein 3.
On univariate analysis (Table 2) a steeper decline in IGF-I, ΔIGF-I, (β −0.04 ms/nmol/L/10 years [−0.08, −0.008], p = 0.019) and a shallower rise in ΔIGF-I/IGFBP-3 (β −2.44 ms/unit/10 years [−4.17, −0.67], p = 0.007) were associated with a longer QTc. These associations persisted in complete case analysis multivariable models (β −0.05 ms/nmol/L/10 years [−0.09, −0.003], p = 0.038 and β −2.14 ms/unit/10 years [−4.13, −0.06], p = 0.042) and in fully adjusted imputed models (β −0.05 ms/nmol/L/10 years [−0.10, −0.002], p = 0.042 and β −2.16 ms/unit/10 years [−4.23, −0.09], p = 0.041). As before, the multiple imputation analyses remained significant for sex, left ventricular mass and blood potassium levels.
In imputed models, observed associations between IGF-I/IGFBP-3 molar ratio at 60–64y and ΔIGF-I/IGFBP-3 ratio with QTc, were only slightly attenuated after removing the 273 participants with known cardiovascular disease (respectively: β −17.60 ms/unit [−34.41, −0.80], p = 0.040 and β −2.35 ms/unit/10 years [−4.44, −0.24], p = 0.029, Supplementary Table 3).
In a UK-based sample of older persons aged 60–64 years, declining serum levels and bioavailability of IGF-I (previously known as Somatomedin C) associated with QTcprolongation, a well-established risk factor for sudden death, independent of sex, SEP, BMI, LV mass, heart disease and hypertension.
In several studies it has been shown that reduced levels of IGF-I were associated with increased risk of cardiovascular disease (44, 45). IGF-I is thought to protect cardiac myocytes from arrhythmogenesis and apoptosis by activating the PI3-K/Akt cell survival intracellular signaling (21), although the exact mechanism remains to be fully elucidated, with a possibility that channel transcription might be affected. The PI3-K pathway activates the serine/threonine protein kinase Akt, and Protein Kinase C, promoting cardiovascular homeostasis, neuroprotection, survival, gene expression, and insulin activity (19, 46). The rapid delayed rectifier potassium channels that influence cardiac repolarization in cardiomyocytes are regulated by PI3-K (21, 22, 47). These channels (IKs, IKr and the atrial specific IKur) conduct outward potassium currents during the plateau phase of the action potential (48). Mutations in the genes encoding delayed rectifiers disrupt normal cardiac repolarization and lead to various cardiac rhythm disorders, including congenital long QT syndrome. Late sodium currents, INaL, were shown to be activated by another a downward pathway of PI3-K, the serum- and glucocorticoid-regulated kinase (SGK), which phosphorylates the sodium channels on the cardiomyocyte surface. GSK was shown to phosphorylates neural precursor cell expressed developmentally down-regulated protein 4 (NEED4), blocking the ubiquitination of the sodium channel (49, 50). These two channels, IKr and INa, were thought to finely interact to maintain a correct ventricular repolarization (51). Reduced IGF-I binding to its receptors on cardiomyocytes, has been shown to decrease the activation of the PI3-K/Akt pathway in animal models (22) thus prolonging the action potential duration and also the QTc. One factor that is thought to mediate the electrophysiological effect of IGF-I is nuclear factor erythroid 2-related factor 2 (Nrf2), which normally induces the transcription of cytoprotective enzymes involved in antioxidative pathways (52, 53) that have the ability to suppress ventricular arrhythmias. Evidence suggests that IGF-I regulates the levels of Nrf2 expression and modulates its transcriptional activity via the PI3K/Akt pathway (54). Based on the results from our current study, it is plausible therefore, that in older persons, declining levels and bioavailability of IGF-I reduce expression of Nrf2, prolonging QTc, and increasing the propensity for malignant arrhythmias.
IGF-I and IGF-II are mainly produced by the liver under the influence of growth hormone and nutrition (19, 55, 56). They have a similar structure to insulin with a similar direct effect on the body's glucose metabolism (57, 58), but are found in much higher concentrations in the blood where they are bound to binding proteins (mainly to isoform IGFBP-3), also produced by the liver. Binding to IGFBP-3 increases its half-life and modulates receptor binding. IGF-I fulfills several important functions in the human body by reaching several targets, where both insulin and IGF-1 receptors are found. An imbalance in serum IGF-I levels has been associated with a variety of negative effects, in several body systems, including obesity, diabetes and atherosclerosis. Reduced levels of IGF-I increase risk of hypertension, inflammation and endothelial dysfunction as normal IGF-I levels were shown to be protective, stimulating the release of nitric oxide, a vasodilator, and promoting cell proliferation and differentiation (46, 59). Quantitatively, IGF-II is the predominant circulating IGF, present in adults at a concentration up to three times that of IGF-I (as noted in our cohort). In mammals, IGF-I mediates the growth promoting effects of growth hormone during postnatal life and throughout adulthood. It influences cardiomyocytes and the cardiac action potential as described above, but IGF-II is more involved in placental and fetal growth (including cardiac development) in utero (60), is less growth hormone dependent than IGF-I (61), and is not known to influence the cardiac action potential in mature cardiomyocytes. Indeed, we found no statistically significant association between circulating levels of IGF-II and QTc interval at either time point. As IGF-I fulfills important somatic growth function it reaches its highest levels during teenage years, with levels subsequently decreasing with age (16, 17) in a highly variable and individual process that is related to fat mass (16), sex, diet and hormonal status. Among its various functions, IGF-I protects against inflammation, hypertension, endothelial and β-cell dysfunction (46, 59, 62), inhibits growth hormone hypersecretion and suppresses insulin secretion whilst enhancing insulin's action (63). An imbalance in serum IGF-I levels has been associated with obesity, diabetes and atherosclerosis (46, 59).
The six IGF-binding proteins (IGFBP-1-6) have both IGF-dependent and independent functions (64, 65). IGFBP-3 is the most abundant of the six IGFBPs in the circulation. Low IGFBP-3 levels have been associated with adverse cardiovascular effects, increased vascular disease and higher risk of coronary events (66). IGFBPs serve not only to transport IGFs in the circulation but also to prolong their half-lives, modulate their tissue specificity, and to either potentiate or neutralize their biological actions at tissue level (65). Measuring free (unbound) IGF-I remains a challenge (52) and total measured serum IGF-I is not tantamount to bioavailable IGF-I (33): almost all circulating IGF-I is bound to IGFBPs leaving <1% of IGF-I in a free form bioavailable for receptor-binding (47). The molar ratio of total IGF-I to IGFBP-3 is widely used as the proxy for bioavailable IGF-I (67).
Our study examined whether the differential decline in IGFs, IGFBP-3 or IGF-I/IGFBP-3 ratio over a decade (53 to 60–64 years) put some older persons at higher risk of QTc prolongation. Results showed that adults who experienced a steeper decline in IGF-I over a decade of later life were at higher risk of QTc prolongation. With age, levels of IGFBP-3 decline more steeply than IGF-I which is why the IGF-I/IGFBP-3 ratio (and therefore IGF-I bioavailability) appears to increase (32) (Figure 2). We show that older persons whose molar ratios increased least over a decade—implying less bioavailable IGF-I overall—had prolonged QTc compared to those who had higher IGF-I bioavailability. This aligns with other recently published data showing how low levels of IGFBP-3 increased the risk of cardiovascular disease and mortality (18) and how low IGF-I/IGFBP-3 ratios increased the risk of metabolic syndrome and insulin resistance (68). The fact that after removing persons with cardiovascular disease in the sensitivity analysis, only the 60–64y IGF-I/IGFBP-3 molar ratio and ΔIGF-I/IGFBP-3 ratio retained significant association with QTc, adds credence to the notion that it is the free, bioavailable IGF-I which most strongly determines the electrophysiological effect observed. Therefore, the IGF-I/IGFBP-3 ratio has to maintained at safe levels to avoid adverse metabolic, cardiovascular and neoplastic effects (69).
Our finding, that low levels of IGF-I relate to QTc prolongation and therefore higher sudden cardiac death risk, fits with several other known adverse cardiovascular effects of IGF-I deficiency, that include accelerated cardiovascular aging, reduced cardiac contractility, hypertrophy, hypertension, coronary disease and even atrial fibrillation (19, 53, 70). Conversely, normal IGF-I levels appear to be cardioprotective, by stimulating the release of vasodilatory nitric oxide, and by promoting cell proliferation and differentiation (46, 59). In mice IGF-I receptor deficiency has been associated with cardiomyopathy and heart failure (53).
Previous works that failed to account for confounders, have observed an association between diabetes or insulin resistance with QTc prolongation (71–75), but we found no association between fasting blood glucose levels or HbA1c with QTc at age 60-64. The mechanism of QTc prolongation in insulin resistance is still unclear but it is known that insulin may increase the transmembrane potential of cardiomyocytes by activating the electrogenic Na+/K+-ATPase leading to hyperpolarization and therefore QT prolongation (76).
We found an association between low blood potassium levels and QTc prolongation, as anticipated. Important cardiomyocyte-specific mechanisms are the main determinants of hypokalemia-induced QT prolongation (77) in addition to altering autonomic nervous system activity (78, 79). Potassium deficiency, as shown experimentally in a legacy study on rats (80), also reduces IGF-I, so it potentially confounds the relationship between IGF-I and QTc. Several previous studies (81–83) explored an association between increased LV mass and prolonged QTc, supporting our evidence, that LV mass is also an important determinant of QTc.
As with most epidemiological studies, the main limitation is unmeasured or residual confounding as this precludes causal inferences. The extent of missing data in our study (Supplementary Tables 1, 2) was small and we go on to show that key associations between biomarkers and QTc persisted after multiple imputation. Multiple imputation, however, cannot account for sample selection. The inclusion of British people born during the same week in 1946, leads to issues with external validity as the findings may not be applicable to non-British populations.
Earlier measurements of IGFs and IGFBP-3 (pre-53y), as well as measurements at shorter time intervals could have helped detect temporal trends better in this cohort. Levels of other IGFBPs that may have more direct effects on cardiac function were not measured (20, 84). The broader metabolic effects of IGF-I linked to other metabolic markers such as leptin, insulin or glucose, could not be explored over the decade as measurements of these additional blood markers were not available at the age of 53y. Several methods for QT correction exist and although the Bazett's formula remains one of the most widely used methods, it is known to overcorrect the QT interval (85, 86). Other correction methods (86, 87), including Hodges' formula used here, have been shown to be better (86). Comparisons between the various QTc correction methods was beyond the scope of this study, yet we go on to show in the sensitivity analysis (Supplementary Table 4) that key biomarker associations with QTc persisted after additional adjustment for heart rate in the multivariable models.
In a large older-age population-based cohort, declining levels and bioavailability of IGF-I associate with prolonged QTc interval. As QTc prolongation is known to be associated with increased risk for sudden death even in apparently healthy people, further work is needed to understand and preserve the potentially anti-arrhythmic effects of IGF-I in older age.
NSHD data is available through the Medical Research Council Skylark website (https://skylark.ucl.ac.uk/Skylark) and full details on the archived data is available at: https://www.nshd.mrc.ac.uk/data.
The studies involving human participants were reviewed and approved by Greater Manchester Local Research Ethics Committee and the Scotland Research Ethics Committee. The patients participants provided their written informed consent to participate in this study.
CC and GC conceived of the study. CC wrote the manuscript and analyzed the data. JM, JH, NC, and AH provided expert review of the manuscript. All authors contributed to the article and approved the submitted version.
GC and JM are supported by the Barts Charity HeartOME grant (MGU0427). GC was supported by British Heart Foundation (MyoFit46 Special Programme Grant SP/20/2/34841) and by the NIHR UCL Hospitals Biomedical Research Center. The NSHD cohort was funded by the UK MRC (program codes MC_UU_12019/1; MC_UU_12019/4; MC_UU_12019/5). JM is directly and indirectly supported by the UCL Hospitals NIHR BRC and Biomedical Research Unit at Barts Hospital, respectively. AH receives support from the British Heart Foundation, the Economic and Social Research Council (ESRC), the Horizon 2020 Framework Programme of the European Union, the National Institute on Aging, the National Institute for Health Research University College London Hospitals Biomedical Research Center and the UK MRC.
The authors declare that the research was conducted in the absence of any commercial or financial relationships that could be construed as a potential conflict of interest.
All claims expressed in this article are solely those of the authors and do not necessarily represent those of their affiliated organizations, or those of the publisher, the editors and the reviewers. Any product that may be evaluated in this article, or claim that may be made by its manufacturer, is not guaranteed or endorsed by the publisher.
The authors would like to thank NSHD members for their participation and continuous engagement with follow-up and all past and present NSHD scientific and data collection teams. The authors are also grateful to Imran Shah and Andrew Wong at the MRC Unit for Lifelong Health and Aging at UCL for his assistance with NSHD data access.
The Supplementary Material for this article can be found online at: https://www.frontiersin.org/articles/10.3389/fcvm.2022.863988/full#supplementary-material
1. O'Neal Wesley T, Singleton Matthew J, Roberts Jason D, Tereshchenko Larisa G, Sotoodehnia N, Chen Lin Y, et al. Association Between QT-Interval Components and Sudden Cardiac Death. Circ Arrhythm Electrophysiol. (2017) 10:e005485. doi: 10.1161/CIRCEP.117.005485
2. Nachimuthu S, Assar MD, Schussler JM. Drug-induced QT interval prolongation: mechanisms and clinical management. Ther Adv Drug Saf. (2012) 3:241–53. doi: 10.1177/2042098612454283
3. Noord C van, Sturkenboom MCJM, Straus SMJM, Hofman A, Kors JA, Witteman JCM, et al. Serum glucose and insulin are associated with QTc and RR intervals in nondiabetic elderly. Eur J Endocrinol. (2010) 162:241–8. doi: 10.1530/EJE-09-0878
4. Winter J, Tipton MJ, Shattock MJ. Autonomic conflict exacerbates long QT associated ventricular arrhythmias. J Mol Cell Cardiol. (2018) 116:145–54. doi: 10.1016/j.yjmcc.2018.02.001
5. Schwartz PJ, Wolf S. QT interval prolongation as predictor of sudden death in patients with myocardial infarction. Circulation. (1978) 57:1074–7. doi: 10.1161/01.CIR.57.6.1074
6. Strohmer B, Pichler M, Iglseder B, Paulweber B. Relationship of QT interval duration with carotid intima media thickness in a clinically healthy population undergoing cardiovascular risk screening. J Intern Med. (2005) 257:238–46. doi: 10.1111/j.1365-2796.2004.01436.x
7. Gibbs C, Thalamus J, Kristoffersen DT, Svendsen MV, Holla ØL, Heldal K, et al. prolongation predicts short-term mortality independent of comorbidity. EP Europace. (2019) 21:1254–60. doi: 10.1093/europace/euz058
8. Schouten EG, Dekker JM, Meppelink P, Kok FJ, Vandenbroucke JP, Pool J, et al. interval prolongation predicts cardiovascular mortality in an apparently healthy population. Circulation. (1991) 84:1516–23. doi: 10.1161/01.CIR.84.4.1516
9. Sedlak T, Shufelt C, Iribarren C, Merz CNB. Sex hormones and the QT interval: a review. J Womens Health. (2012) 21:933–41. doi: 10.1089/jwh.2011.3444
10. Veglio M, Borra M, Stevens LK, Fuller JH, Perin PC. The relation between QTc interval prolongation and diabetic complications. The EURODIAB IDDM Complication Study Group. Diabetologia. (1999) 42:68–75. doi: 10.1007/s001250051115
11. Osadchii OE. Mechanisms of hypokalemia-induced ventricular arrhythmogenicity. Fundam Clin Pharmacol. (2010) 24:547–59. doi: 10.1111/j.1472-8206.2010.00835.x
12. Festa A, D'Agostino R, Rautaharju P, Mykkänen L, Haffner SM. Relation of systemic blood pressure, left ventricular mass, insulin sensitivity, and coronary artery disease to QT interval duration in nondiabetic and type 2 diabetic subjects. Am J Cardiol. (2000) 86:1117–22. doi: 10.1016/S0002-9149(00)01170-X
13. Sun G-Z, Zhou Y, Ye N, Wu S-J, Sun Y-X. Independent Influence of Blood Pressure on QTc Interval: Results from a General Chinese Population. Biomed Res Int. (2019) 2019:1656123. doi: 10.1155/2019/1656123
14. Maragna R, Mazzanti A, Priori SG. Genetics of Long QT Short QT Syndromes. In: eLS = John Wiley & Sons, Ltd (2017). Available online at: https://onlinelibrary.wiley.com/doi/abs/10.1002/9780470015902.a0024386 (accessed March 17).
15. Hedley PL, Jørgensen P, Schlamowitz S, Wangari R, Moolman-Smook J, Brink PA, et al. The genetic basis of long QT and short QT syndromes: a mutation update. Hum Mutat. (2009) 30:1486–511. doi: 10.1002/humu.21106
16. Bann D, Holly JMP, Lashen H, Hardy R, Adams J, Kuh D, et al. Changes in insulin-like growth factor-I and -II associated with fat but not lean mass in early old age. Obesity. (2015) 23:692–8. doi: 10.1002/oby.21002
17. Higashi Y, Gautam S, Delafontaine P, Sukhanov S. IGF-1 and cardiovascular disease. Growth Horm IGF Res. (2019) 45:6–16. doi: 10.1016/j.ghir.2019.01.002
18. Friedrich N, Haring R, Nauck M, Lüdemann J, Rosskopf D, Spilcke-Liss E, et al. Mortality and Serum Insulin-Like Growth Factor (IGF)-I and IGF Binding Protein 3 Concentrations. J Clin Endocrinol Metab. (2009) 94:1732–9. doi: 10.1210/jc.2008-2138
19. Puche JE, Castilla-Cortázar I. Human conditions of insulin-like growth factor-I (IGF-I) deficiency. J Transl Med. (2012) 10:224. doi: 10.1186/1479-5876-10-224
20. Laughlin GA, Barrett-Connor E, Criqui MH, Kritz-Silverstein D. The prospective association of serum insulin-like growth factor I (IGF-I) and IGF-binding protein-1 levels with all cause and cardiovascular disease mortality in older adults: the Rancho Bernardo Study. J Clin Endocrinol Metab. (2004) 89:114–20. doi: 10.1210/jc.2003-030967
21. Millis RM, Alvin ZV, Zhao A, Haddad GE. Effects of IGF-1 on and Channels via PI3K/Akt Signaling in Neonatal Cardiac Myocytes. Int J Cell Biol. (2012) 2012:712153. doi: 10.1155/2012/712153
22. Ballou Lisa M, Lin Richard Z, Cohen Ira S. Control of Cardiac Repolarization by Phosphoinositide 3-Kinase Signaling to Ion Channels. Circ Res. (2015) 116:127–37. doi: 10.1161/CIRCRESAHA.116.303975
23. Lu Z, Jiang Y-P, Wu C-YC, Ballou LM, Liu S, Carpenter ES, et al. Increased Persistent Sodium Current Due to Decreased PI3K Signaling Contributes to QT Prolongation in the Diabetic Heart. Diabetes. (2013) 62:4257–65. doi: 10.2337/db13-0420
24. Junnila RK, List EO, Berryman DE, Murrey JW, Kopchick JJ. The GH/IGF-1 axis in ageing and longevity. Nat Rev Endocrinol. (2013) 9:366–76. doi: 10.1038/nrendo.2013.67
25. Jones JI, Clemmons DR. Insulin-like growth factors and their binding proteins: biological actions*. Endocr Rev. (1995) 16:3–34. doi: 10.1210/edrv-16-1-3
26. Juul A, Main K, Blum WF, Lindholm J, Ranke MB, Skakkebaek NE. The ratio between serum levels of insulin-like growth factor (IGF)-I and the IGF binding proteins (IGFBP-1, 2 and 3) decreases with age in healthy adults and is increased in acromegalic patients. Clin Endocrinol. (1994) 41:85–93. doi: 10.1111/j.1365-2265.1994.tb03788.x
27. Kuh D, Pierce M, Adams J, Deanfield J, Ekelund U, Friberg P, et al. Cohort profile: updating the cohort profile for the MRC national survey of health and development: a new clinic-based data collection for ageing research. Int J Epidemiol. (2011) 40:e1–9. doi: 10.1093/ije/dyq231
28. Bann D, Cooper R, Wills AK, Adams J, Kuh D. Socioeconomic position across life and body composition in early old age: findings from a British birth cohort study. J Epidemiol Community Health. (2014) 68:516–23. doi: 10.1136/jech-2013-203373
29. Jay MA, Bendayan R, Cooper R, Muthuri SG. Lifetime socioeconomic circumstances and chronic pain in later adulthood: findings from a British birth cohort study. BMJ Open. (2019) 9:e024250. doi: 10.1136/bmjopen-2018-024250
30. Luo S, Michler K, Johnston P, Macfarlane PW. A comparison of commonly used QT correction formulae: the effect of heart rate on the QTc of normal ECGs. J Electrocardiol. (2004) 37:81–90. doi: 10.1016/j.jelectrocard.2004.08.030
31. Lemmey A, Maddison P, Breslin A, Cassar P, Hasso N, McCann R, et al. Association between insulin-like growth factor status and physical activity levels in rheumatoid arthritis. J Rheumatol. (2001) 28:29–34.
32. Salzmann A, James S-N, Williams DM, Richards M, Cadar D, Schott JM, et al. Investigating the Relationship Between IGF-I, IGF-II, and IGFBP-3 Concentrations and Later-Life Cognition and Brain Volume. J Clin Endocrinol Metab. (2021) 106:1617–29. doi: 10.1210/clinem/dgab121
33. Kucera R, Topolcan O, Pecen L, Kinkorova J, Svobodova S, Windrichova J, et al. Reference values of IGF1, IGFBP3 and IGF1/IGFBP3 ratio in adult population in the Czech Republic. Clin Chim Acta. (2015) 444:271–7. doi: 10.1016/j.cca.2015.02.036
34. Clemmons DR. Consensus statement on the standardization and evaluation of growth hormone and insulin-like growth factor assays. Clin Chem. (2011) 57:555–9. doi: 10.1373/clinchem.2010.150631
35. Friedrich N, Wolthers OD, Arafat AM, Emeny RT, Spranger J, Roswall J, et al. Age- and sex-specific reference intervals across life span for insulin-like growth factor binding protein 3 (IGFBP-3) and the IGF-I to IGFBP-3 ratio measured by new automated chemiluminescence assays. J Clin Endocrinol Metab. (2014) 99:1675–86. doi: 10.1210/jc.2013-3060
36. Murray ET, Jones R, Thomas C, Ghosh AK, Sattar N, Deanfield J, et al. Life course socioeconomic position: associations with cardiac structure and function at age 60-64 years in the 1946 british birth cohort. PLoS ONE. (2016) 11:e0152691. doi: 10.1371/journal.pone.0152691
37. Jones R, Hardy R, Sattar N, Deanfield JE, Hughes A, Kuh D, et al. Novel coronary heart disease risk factors at 60–64 years and life course socioeconomic position: the 1946 British birth cohort. Atherosclerosis. (2015) 238:70–6. doi: 10.1016/j.atherosclerosis.2014.11.011
38. Strand BH, Mishra G, Kuh D, Guralnik JM, Patel KV. Smoking history and physical performance in midlife: results from the british 1946 birth cohort. J Gerontol A Biol Sci Med Sci. (2011) 66A:142–9. doi: 10.1093/gerona/glq199
39. Bann D, Hardy R, Cooper R, Lashen H, Keevil B, Wu FCW, et al. Socioeconomic conditions across life related to multiple measures of the endocrine system in older adults: Longitudinal findings from a British birth cohort study. Soc Sci Med. (2015) 147:190–9. doi: 10.1016/j.socscimed.2015.11.001
40. James S-N, Wong A, Tillin T, Hardy R, Chaturvedi N, Richards M. The effect of mid-life insulin resistance and type 2 diabetes on older-age cognitive state: the explanatory role of early-life advantage. Diabetologia. (2019) 62:1891–900. doi: 10.1007/s00125-019-4949-3
41. Topriceanu C-C, Moon JC, Hardy R, Chaturvedi N, Hughes AD, Captur G. Longitudinal birth cohort study finds that life-course frailty associates with later-life heart size and function. Sci Rep. (2021) 11:6272. doi: 10.1038/s41598-021-85435-8
42. Horton NJ, Lipsitz SR. Multiple imputation in practice: comparison of software packages for regression models with missing variables. Am Stat. (2001) 55:244–54. doi: 10.1198/000313001317098266
43. Rautaharju PM, Mason JW, Akiyama T. New age- and sex-specific criteria for QT prolongation based on rate correction formulas that minimize bias at the upper normal limits. Int J Cardiol. (2014) 174:535–40. doi: 10.1016/j.ijcard.2014.04.133
44. Juul A, Scheike T, Davidsen M, Gyllenborg J, Jørgensen T. Low serum insulin-like growth factor I is associated with increased risk of ischemic heart disease: a population-based case-control study. Circulation. (2002) 106:939–44. doi: 10.1161/01.CIR.0000027563.44593.CC
45. Saber H, Himali JJ, Beiser AS, Shoamanesh A, Pikula A, Roubenoff R, et al. Serum insulin-like growth factor 1 and the risk of ischemic stroke: the Framingham study. Stroke. (2017) 48:1760–5. doi: 10.1161/STROKEAHA.116.016563
46. Orrù S, Nigro E, Mandola A, Alfieri A, Buono P, Daniele A, Mancini A, Imperlini E. A Functional Interplay between IGF-1 and Adiponectin. Int J Mol Sci. (2017) 18:2145. doi: 10.3390/ijms18102145
47. Teos LY, Zhao A, Alvin Z, Laurence GG, Li C, Haddad GE. Basal and IGF-I-dependent regulation of potassium channels by MAP kinases and PI3-kinase during eccentric cardiac hypertrophy. Am J Physiol Heart Circ Physiol. (2008) 295:H1834–1845. doi: 10.1152/ajpheart.321.2008
48. Chen L, Sampson KJ, Kass RS. Cardiac delayed rectifier potassium channels in health and disease. Card Electrophysiol Clin. (2016) 8:307–22. doi: 10.1016/j.ccep.2016.01.004
49. Debonneville C, Flores SY, Kamynina E, Plant PJ, Tauxe C, Thomas MA, et al. Phosphorylation of Nedd4-2 by Sgk1 regulates epithelial Na+ channel cell surface expression. EMBO J. (2001) 20:7052–9. doi: 10.1093/emboj/20.24.7052
50. Das S, Aiba T, Rosenberg M, Hessler K, Xiao C, Quintero PA, et al. Pathological role of serum- and glucocorticoid-regulated kinase 1 in adverse ventricular remodeling. Circulation. (2012) 126:2208–19. doi: 10.1161/CIRCULATIONAHA.112.115592
51. Hegyi B, Chen-Izu Y, Izu LT, Rajamani S, Belardinelli L, Bers DM, et al. Balance between rapid delayed rectifier K+ current and late Na+ current on ventricular repolarization: an effective antiarrhythmic target? Circ Arrhythm Electrophysiol. (2020) 13:e008130. doi: 10.1161/CIRCEP.119.008130
52. Yang J, Yin H-S, Cao Y-J, Jiang Z-A, Li Y-J, Song M-C, et al. Arctigenin attenuates ischemia/reperfusion induced ventricular arrhythmias by decreasing oxidative stress in rats. Cell Physiol Biochem. (2018) 49:728–42. doi: 10.1159/000493038
53. Ungvari Z, Csiszar A. The emerging role of IGF-1 deficiency in cardiovascular aging: recent advances. J Gerontol A Biol Sci Med Sci. (2012) 67A:599–610. doi: 10.1093/gerona/gls072
54. Bailey-Downs LC, Mitschelen M, Sosnowska D, Toth P, Pinto JT, Ballabh P, et al. Liver-specific knockdown of IGF-1 decreases vascular oxidative stress resistance by impairing the Nrf2-dependent antioxidant response: a novel model of vascular aging. J Gerontol A Biol Sci Med Sci. (2012) 67A:313–29. doi: 10.1093/gerona/glr164
55. Conti E, Musumeci MB, De Giusti M, Dito E, Mastromarino V, Autore C, et al. IGF-1 and atherothrombosis: relevance to pathophysiology and therapy. Clin Sci. (2011) 120:377–402. doi: 10.1042/CS20100400
56. Friedrich N, Thuesen B, Jørgensen T, Juul A, Spielhagen C, Wallaschofksi H, et al. The association between IGF-I and insulin resistance. Diabetes Care. (2012) 35:768–73. doi: 10.2337/dc11-1833
57. Laviades C, Gil MJ, Monreal I, González A, Díez J. Tissue availability of insulin-like growth factor I is inversely related to insulin resistance in essential hypertension: effects of angiotensin converting enzyme inhibition. J Hypertens. (1998) 16:863–70. doi: 10.1097/00004872-199816060-00018
58. Clemmons DR. The relative roles of growth hormone and IGF-1 in controlling insulin sensitivity. J Clin Invest. (2004) 113:25–7. doi: 10.1172/JCI20660
59. Higashi Y, Quevedo HC, Tiwari S, Sukhanov S, Shai S-Y, Anwar A, et al. The interaction between IGF-1, atherosclerosis and vascular aging. Front Horm Res. (2014) 43:107–24. doi: 10.1159/000360571
60. Wang K, Shen H, Gan P, Cavallero S, Kumar SR, Lien C-L, et al. Differential roles of insulin like growth factor 1 receptor and insulin receptor during embryonic heart development. BMC Dev Biol. (2019) 19:5. doi: 10.1186/s12861-019-0186-8
61. Adamek A, Kasprzak A. Insulin-like growth factor (IGF) system in liver diseases. Int J Mol Sci. (2018) 19:1308. doi: 10.3390/ijms19051308
62. Schneider HJ, Friedrich N, Klotsche J, Schipf S, Nauck M, Völzke H, et al. Prediction of incident diabetes mellitus by baseline IGF1 levels. Eur J Endocrinol. (2011) 164:223–9. doi: 10.1530/EJE-10-0963
63. Yakar S, Liu J-L, Fernandez AM, Wu Y, Schally AV, Frystyk J, et al. Liver-specific igf-1 gene deletion leads to muscle insulin insensitivity. Diabetes. (2001) 50:1110–8. doi: 10.2337/diabetes.50.5.1110
64. Varma Shrivastav S, Bhardwaj A, Pathak KA, Shrivastav A. Insulin-like growth factor binding protein-3 (IGFBP-3): unraveling the role in mediating IGF-independent effects within the cell. Front Cell Dev Biol. (2020) 8:286. doi: 10.3389/fcell.2020.00286
65. Baxter RC. Insulin-like growth factor binding protein-3 (IGFBP-3): novel ligands mediate unexpected functions. J Cell Commun Signal. (2013) 7:179–89. doi: 10.1007/s12079-013-0203-9
66. Kaplan RC, McGinn AP, Pollak MN, Kuller LH, Strickler HD, Rohan TE, et al. Association of total insulin-like growth factor-I, insulin-like growth factor binding protein-1 (IGFBP-1), and IGFBP-3 levels with incident coronary events and ischemic stroke. J Clin Endocrinol Metab. (2007) 92:1319–25. doi: 10.1210/jc.2006-1631
67. Zhang WB, Aleksic S, Gao T, Weiss EF, Demetriou E, Verghese J, et al. Insulin-like growth factor-1 and IGF binding proteins predict all-cause mortality and morbidity in older adults. Cells. (2020) 9:1368. doi: 10.3390/cells9061368
68. Sierra-Johnson J, Romero-Corral A, Somers VK, Lopez-Jimenez F, Mälarstig A, Brismar K, et al. IGF-I/IGFBP-3 ratio: a mechanistic insight into the metabolic syndrome. Clin Sci. (2009) 116:507–12. doi: 10.1042/CS20080382
69. Gaddas M, Périn L, Le Bouc Y. Evaluation of IGF1/IGFBP3 molar ratio as an effective tool for assessing the safety of growth hormone therapy in small-for-gestational-age, growth hormone-deficient and prader-willi children. J Clin Res Pediatr Endocrinol. (2019) 11:253–61. doi: 10.4274/jcrpe.galenos.2019.2018.0277
70. Sirbu A, Nicolae H, Martin S, Barbu C, Copaescu C, Florea S, et al. IGF-1 and Insulin resistance are major determinants of common carotid artery thickness in morbidly obese young patients. Angiology. (2016) 67:259–65. doi: 10.1177/0003319715586499
71. Yang X, Su J, Zhang X, Zhao L, Xu F, Wang X, et al. The relationship between insulin sensitivity and heart rate-corrected QT interval in patients with type 2 diabetes. Diabetol Metab Syndr. (2017) 9:69. doi: 10.1186/s13098-017-0268-3
72. Li X, Ren H, Xu Z, Liu Y, Yang X, Liu J. Prevalence and risk factors of prolonged QTc interval among Chinese patients with type 2 diabetes. Exp Diabetes Res. (2012) 2012:234084. doi: 10.1155/2012/234084
73. Timar R, Popescu S, Simu M, Diaconu L, Timar B. QTc interval and insulin resistance in type 2 diabetes mellitus. Eur Sci J. (2013) 9:12. doi: 10.19044/esj.2013.v9n12p%p
74. Soydinc S, Davutoglu V, Akcay M. Uncomplicated metabolic syndrome is associated with prolonged electrocardiographic QTc interval and QTc dispersion. Ann Noninvasive Electrocardiol. (2006) 11:313–7. doi: 10.1111/j.1542-474X.2006.00123.x
75. Milovancev A, Stokic ES. Popovic D, Tomic-Naglic D, Rankov O, Ilincic B. Body weight reduction and QTc interval in obesity. Adv Weigh Loss Manag Med Dev. (2016) 1:1. doi: 10.35248/2593-9793.16.1.102
76. Gastaldelli A, Emdin M, Conforti F, Camastra S, Ferrannini E. Insulin prolongs the QTc interval in humans. Am J Physiol Regul Integr Comp Physiol. (2000) 279:R2022–5. doi: 10.1152/ajpregu.2000.279.6.R2022
77. Weiss JN, Qu Z, Shivkumar K. The electrophysiology of hypo- and hyperkalemia. Circ Arrhythm Electrophysiol. (2017) 10:e004667. doi: 10.1161/CIRCEP.116.004667
78. Chen Y, Guo X, Sun G, Li Z, Zheng L, Sun Y. Effect of serum electrolytes within normal ranges on QTc prolongation: a cross-sectional study in a Chinese rural general population. BMC Cardiovasc Disord. (2018) 18:175. doi: 10.1186/s12872-018-0906-1
79. Numaguchi H, Johnson JP, Petersen CI, Balser JR. A sensitive mechanism for cation modulation of potassium current. Nat Neurosci. (2000) 3:429–30. doi: 10.1038/74793
80. Flyvbjerg A, Dørup I, Everts ME, Ørskov H. Evidence that potassium deficiency induces growth retardation through reduced circulating levels of growth hormone and insulin-like growth factor I. Metabolism. (1991) 40:769–75. doi: 10.1016/0026-0495(91)90001-D
81. Mukerji R, Terry BE, Fresen JL, Petruc M, Govindarajan G, Alpert MA. Relation of left ventricular mass to QTc in normotensive severely obese patients. Obesity. (2012) 20:1950–4. doi: 10.1038/oby.2011.255
82. Salles GF, Cardoso CRL, Deccache W. Multivariate associates of QT interval parameters in diabetic patients with arterial hypertension: importance of left ventricular mass and geometric patterns. J Hum Hypertens. (2003) 17:561–7. doi: 10.1038/sj.jhh.1001590
83. Haugaa KH, Bos JM, Borkenhagen EJ, Tarrell RF, Morlan BW, Caraballo PJ, et al. Impact of left ventricular hypertrophy on QT prolongation and associated mortality. Heart Rhythm. (2014) 11:1957–65. doi: 10.1016/j.hrthm.2014.06.025
84. Allard JB, Duan C. IGF-Binding Proteins: Why do they exist and why are there so many? Front Endocrinol. (2018) 9:117. doi: 10.3389/fendo.2018.00117
85. Ether ND, Jantre SR, Sharma DB, Leishman DJ, Bailie MB, Lauver DA. Improving corrected QT; why individual correction is not enough. J Pharmacol Toxicol Methods. (2022) 113:107126. doi: 10.1016/j.vascn.2021.107126
86. Vandenberk B, Vandael E, Robyns T, Vandenberghe J, Garweg C, Foulon V, et al. Which QT correction formulae to use for QT monitoring? J Am Heart Assoc. (2016) 5:e003264. doi: 10.1161/JAHA.116.003264
87. Vandenberk B, Vandael E, Robyns T, Vandenberghe J, Garweg C, Foulon V, et al. correction across the heart rate spectrum, in atrial fibrillation and ventricular conduction defects. Pacing Clin Electrophysiol. (2018) 41:1101–8. doi: 10.1111/pace.13423
Keywords: QTc interval prolongation, cardiac repolarization, IGF-I (insulin-like growth factor-I), IGFBP-3, IGF-I/IGFBP-3 molar ratio, IGF-II
Citation: Charalambous C, Moon JC, Holly JMP, Chaturvedi N, Hughes AD and Captur G (2022) Declining Levels and Bioavailability of IGF-I in Cardiovascular Aging Associate With QT Prolongation–Results From the 1946 British Birth Cohort. Front. Cardiovasc. Med. 9:863988. doi: 10.3389/fcvm.2022.863988
Received: 27 January 2022; Accepted: 21 March 2022;
Published: 22 April 2022.
Edited by:
Flavia Prodam, University of Eastern Piedmont, ItalyReviewed by:
Bert Vandenberk, University of Calgary, CanadaCopyright © 2022 Charalambous, Moon, Holly, Chaturvedi, Hughes and Captur. This is an open-access article distributed under the terms of the Creative Commons Attribution License (CC BY). The use, distribution or reproduction in other forums is permitted, provided the original author(s) and the copyright owner(s) are credited and that the original publication in this journal is cited, in accordance with accepted academic practice. No use, distribution or reproduction is permitted which does not comply with these terms.
*Correspondence: Gabriella Captur, Z2FicmllbGxhLmNhcHR1ckB1Y2wuYWMudWs=
Disclaimer: All claims expressed in this article are solely those of the authors and do not necessarily represent those of their affiliated organizations, or those of the publisher, the editors and the reviewers. Any product that may be evaluated in this article or claim that may be made by its manufacturer is not guaranteed or endorsed by the publisher.
Research integrity at Frontiers
Learn more about the work of our research integrity team to safeguard the quality of each article we publish.