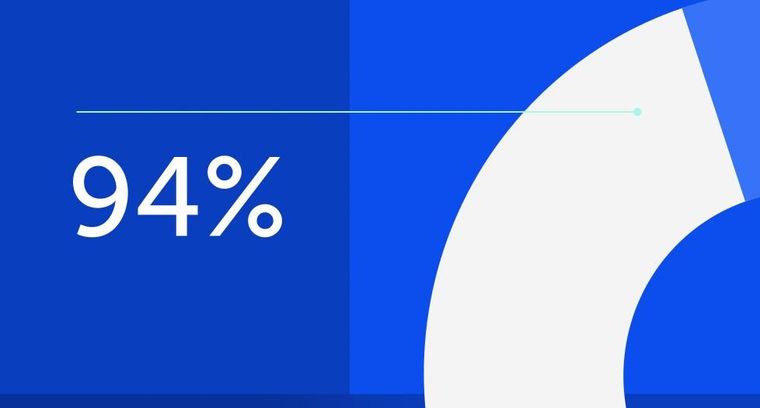
94% of researchers rate our articles as excellent or good
Learn more about the work of our research integrity team to safeguard the quality of each article we publish.
Find out more
ORIGINAL RESEARCH article
Front. Cardiovasc. Med., 06 May 2022
Sec. Heart Failure and Transplantation
Volume 9 - 2022 | https://doi.org/10.3389/fcvm.2022.849688
Objective: The management of cardiogenic shock (CS) in the elderly remains a major clinical challenge. Existing clinical prediction models have not performed well in assessing the prognosis of elderly patients with CS. This study aims to build a predictive model, which could better predict the 30-day mortality of elderly patients with CS.
Methods: We extracted data from the Medical Information Mart for Intensive Care III version 1.4 (MIMIC-III) as the training set and the data of validation sets were collected from the Second Affiliated Hospital and Yuying Children's Hospital of Wenzhou Medical University. Three models, including the cox regression model, the Least Absolute Shrinkage and Selection Operator (LASSO) regression model, and the CoxBoost model, were established using the training set. Through the comparison of area under the receiver operating characteristic (ROC) curve (AUC), C index, net reclassification improvement (NRI), integrated discrimination improvement (IDI), and median improvement in risk score, the best model was selected. Then for external validation, compared the best model with the simplified acute physiology score II (SAPSII) and the CardShock risk score.
Results: A total of 919 patients were included in the study, of which 804 patients were in the training set and 115 patients were in the verification set. Using the training set, we built three models: the cox regression model including 6 predictors, the LASSO regression model including 4 predictors, and the CoxBoost model including 16 predictors. Among them, the CoxBoost model had good discrimination [AUC: 0.730; C index: 0.6958 (0.6657, 0.7259)]. Compared with the CoxBoost model, the NRI, IDI, and median improvement in risk score of other models were all<0. In the validation set, the CoxBoost model was also well-discriminated [AUC: 0.770; C index: 0.7713 (0.6751, 0.8675)]. Compared with the CoxBoost model, the NRI, IDI, and median improvement in risk score of SAPS II and the CardShock risk score were all < 0. And we constructed a dynamic nomogram to visually display the model.
Conclusion: In conclusion, this study showed that in predicting the 30-day mortality of elderly CS patients, the CoxBoost model was superior to the Cox regression model, LASSO regression model, SAPS II, and the CardShock risk score.
Cardiogenic shock (CS) is an extremely serious clinical condition and occurs as a consequence of cardiac pump failure, which has a high mortality (1, 2). In recent decades, the prevalence of CS has increased from 4.1 to 7.7%, and the mortality rate is up to 40% (3–5). Age itself increases the risk of CS associated with myocardial infarction, and advanced age itself is an independent risk factor for CS (6). This suggested that for the elderly, the risk of CS will be higher. The WHO defines the elderly as over 65 years old; as this aging population continues to grow, it is crucial to pay attention to issues related to this group. Therefore, the management of CS in the elderly is a huge clinical challenge (7, 8). Finding an efficient method to early evaluate the prognosis is helpful for clinicians to identify high-risk patients in time, and make better medical decisions in clinical practice.
However, in the elderly population, information on the prevalence, determinants, and prognostic factors of CS is scarce. Some researchers have constructed risk prediction models to facilitate risk assessment and predict the mortality of CS, such as the CardShock risk score (9, 10), but these models still have some shortcomings, such as the calculation methods are complicated, or the included predictors of some models are not all objective data. For example, in the CardShock risk score, the evaluation of the patients' consciousness depends on the subjective judgment of clinicians. Besides, they are not completely built for the elderly population, the evaluation effect of the prognosis of the elderly needs further assessment.
Compared with traditional forecasting methods, novel machine learning techniques can process high-dimensional data, identify complex relationships between variables and develop precise clinical prediction models, which have received more and more attention and recognition from clinicians (11, 12). There are many studies that apply machine learning to the evaluation of a variety of diseases and with good results (13–15). It has also been used in other medical auxiliary disciplines, such as imaging and anesthesiology (16, 17). Hou et al. (18) used XGboost machine learning to predict 30-days mortality of patients with sepsis. Weyer and Binder (19) developed a weighting approach for judging the effect of patient strata on high-dimensional risk prediction signatures using CoxBoost. Therefore, we hope to use the CoxBoost method to help us better predict the prognosis of elderly patients with CS.
Based on the Medical Information Mart for Intensive Care III (MIMIC-III) database and the Second Affiliated Hospital and Yuying Children's Hospital of Wenzhou Medical University (WMU), the goal of this study is to build a predictive model using CoxBoost machine learning, which can predict the 30-day mortality of elderly patients with CS.
We extracted data from MIMIC-III database version 1.4 as the training set, which is a public and free intensive care unit (ICU) database (20). Since the MIMIC-III database was approved by the Institutional Review Boards (IRB) of Beth Israel Deaconess Medical Center (Boston, MA) and the Massachusetts Institute of Technology (Cambridge, MA), IRB approval from our institution was exempted. Data of validation sets were collected from the patient data of ICU at the Second Affiliated Hospital and Yuying Children's Hospital of WMU. The Medical Ethics Committee of the Second Affiliated Hospital and Yuying Children's Hospital, WMU approved the use of the patient data of the Second Affiliated Hospital and Yuying Children's Hospital (Ethical Review Number: 2021-K-71-01).
The inclusion criteria were as follows: (1) ICU admissions age ≥ 65 years; (2) CS patients in MIMIC-III database and the Second Affiliated Hospital and Yuying Children's Hospital of WMU. Among them, patients from the MIMIC-III database were diagnosed according to the International Classification of Diseases, Ninth Revision (ICD-9) diagnostic code 785.51 or 998.01, and patients from the Second Affiliated Hospital and Yuying Children's Hospital of WMU were selected by unified diagnostic criteria: minimum systolic blood pressure (SBP) <90 mmHg, or need of vasopressors therapy or signs of hypoperfusion. Only records of the first ICU visit were selected for analysis to eliminate duplicate data. The specific process was shown in Figure 1.
We extracted the following information of patients on the first day of admission: (1) demographic information such as age, gender, ethnicity, and pathogeny; (2) vital sign data including heart rate, SBP, mean blood pressure (MBP), diastolic blood pressure (DBP), respiratory rate, temperature, and arterial oxygen saturation (SpO2); (3) laboratory data including white blood cell (WBC) count, red cell distribution width (RDW), hemoglobin, hematocrit, platelet, activated partial thromboplastin time (APTT), international normalized ratio (INR), prothrombin time (PT), anion gap, bicarbonate, glucose, blood lactic acid, serum creatinine, serum urea nitrogen, serum sodium, and serum potassium; (4) comorbidities including congestive heart failure, atrial fibrillation, coronary heart disease, renal failure, liver disease, stroke, tumor, chronic obstructive pulmonary disease (COPD), acute respiratory distress syndrome (ARDS), and pneumonia; (5) traditional severity scores including simplified acute physiology score II (SAPSII); (6) As well as ejection fraction and the use of the vasopressor. If multiple measurements were made in the first 24 h, the vital signs and the laboratory data were based on the results of the first examination. The endpoint was 30-day mortality.
Variables with missing values ≥40% were excluded directly. We used multiple interpolations to deal with missing variables <40%. Patients with individual data missing by more than 10% or outliers [values above the mean of 3 standard deviations (SD)] were excluded. In the training set and the verification set, we carried out the above data processing respectively.
The continuous variables were expressed as mean ± SD of the normal distribution, while the non-normal continuous variables were expressed as median (interquartile range). Wilcoxon W test or Kruskal Wallis test was used to assess the differences among groups. The variance inflation factor (VIF) was calculated to verify whether multicollinearity existed.
In the model development stage, three models were established using the training set: the cox regression model, the Least Absolute Shrinkage and Selection Operator (LASSO) regression model, and the CoxBoost model. In the Cox regression model, we used the monofactor analysis to pick out targeted predictors and screened the predictors twice using multiple regression analysis. After two rounds of screening, the predictive factors were finally incorporated into the Cox regression model. The main idea of LASSO is to construct a first-order penalty function to obtain a refined model and to carry out feature screening by finally determining the coefficients of some variables to be 0. In the LASSO regression model, we determined the cutoff value based on ten-folds cross-validation to select the most useful predictive variables to form the LASSO regression model. CoxBoost (https://github.com/binderh/CoxBoost) is used to fit a Cox proportional hazards model by componentizing likelihood-based boosting, which is particularly suitable for models with a large number of predictors and allows for mandatory covariates with unpenalized parameter estimates. In contrast to gradient boosting, CoxBoost is not based on gradients of loss functions but adapts the offset-based boosting approach for estimating Cox proportional hazards models (21). According to the CoxBoost machine learning analysis of the importance of the predictors, we select targeted variables incorporated into the CoxBoost regression model.
These three models were evaluated together with the classic SAPSII score model. The area under receiver operating characteristic (ROC) curve (AUC), C index, net reclassification improvement (NRI), integrated discrimination improvement (IDI), and median improvement in risk score were used to choose the best model. In the validation set, AUC, C index, NRI, IDI, and median improvement in risk score were also used to evaluate the CoxBoost model, SAPSII score model, and the CardShock risk score model for external validation. We constructed a DynNom-based dynamic nomogram to visually display the final model. R software version 4.0.3 was used for all statistical analyses. P < 0.05 was considered statistically significant.
After excluding the patients who did not meet the inclusion criteria, a total of 919 patients were included in this study. Among them, 804 were from the training set and 115 were from the verification set. We divided these patients into two groups based on their death or survival in 30-day. Table 1 summarizes the basic distribution of all the target patients' baseline characteristics, vital signs, comorbidities, and other indicators. In the training set, the difference in age, SBP, MBP, DBP, respiratory rate, temperature, SpO2, WBC count, RDW, INR, PT, anion gap, bicarbonate, blood lactic acid, serum creatinine, serum urea nitrogen, serum potassium, tumor, SAPSII score, and length of stay in the hospital between the two groups was statistically significant. In the validation set, the difference in temperature, PT, bicarbonate, blood lactic acid, congestive heart failure, pneumonia, SAPSII score, and length of stay in hospital between the two groups was statistically significant.
Based on the training set, we developed three models. In the traditional cox regression model, we first used the monofactor analysis to pick out 8 predictors (P < 0.05), the results are presented in Supplementary Table 1. Then, we screened the predictors twice using multiple regression analysis (Supplementary Table 2). The final Cox regression model was shown in Table 2, a total of 6 predictors including age, heart rate, temperature, WBC count, anion gap, and blood lactic acid. In the LASSO regression model, we determined the cutoff value based on the Loess smoothing function and the Youden index. In this way, 36 features were reduced to 4 potential predictors (Supplementary Table 3), including age, SBP, anion gap, and blood lactic acid (Table 3). According to the CoxBoost machine learning analysis of the importance of the predictors (Supplementary Table 4), we selected 17 predictors (P < 0.05) to construct the CoxBoost model, considering the existence of multicollinearity, we removed MBP. The final model was shown in Table 4, including age, heart rate, SBP, DBP, respiratory rate, temperature, SpO2, WBC count, RDW, INR, PT, anion gap, bicarbonate, blood lactic acid, serum urea nitrogen, and tumor. VIF proved there was no significant multicollinearity in all three models (VIF ≤ 3).
These three models were evaluated together with the classic SAPSII score model to choose the best one. The CoxBoost model had good discrimination (AUC: 0.730) in the training set, which was better than others (Figure 2A). Besides, using the CoxBoost model as a reference, the Cox regression model, LASSO regression model, and SAPSII score model did worse in the C index (cox regression model: 0.6835; LASSO regression model: 0.6786; SAPSII: 0.6490), NRI (cox regression model: −0.0590; LASSO regression model: −0.1480; SAPSII: −0.1630), IDI (cox regression model: −0.0040; LASSO regression model: −0.0300; SAPSII: −0.0530), and median improvement in risk score (cox regression model: −0.0040; LASSO regression model:−0.0220; SAPSII: −0.0570) (Table 5). Therefore, we choose the CoxBoost model as the target model.
Figure 2. (A) Receiver operating characteristic (ROC) comparison of training set models. (B) ROC comparison of validation set models.
In the validation set, we further evaluated and validated the CoxBoost model. The CoxBoost model was well-discriminated in the external validation set (AUC: 0.770), which was greater than the SAPSII score model (AUC: 0.724) and the CardShock risk score (Supplementary Table 5) (AUC: 0.677) (Figure 2B). In the C index, the CoxBoost model has the best performance (C index: CoxBoost model 0.7713, SAPSII score model 0.7341, CardShock risk score 0.6628, Table 5). Using the CoxBoost model as a reference, the NRI of the SAPSII score model and the CardShock risk score were −0.3620 and −0.3480, the IDI of the two models were −0.1740 and −0.2490, and the median improvement in risk scores were −0.2380 and −0.1910 respectively (Table 5), all of which suggested that the CoxBoost model was better.
We constructed a DynNom-based dynamic nomogram to visually display the final model (Table 4) (https://CoxBoost-model.shinyapps.io/DynNomapp/), which could directly obtain the patient's in-hospital mortality by inputting the values of the relevant predictors. The final model formula and its regression coefficients was as follows: survival possibility in 30-day = 0.0339 * age + 0.0104 * heart rate – 0.0070 * SBP – 0.0127 * DBP + 0.0126 * respiratory rate – 0.1959 * temperature – 0.0112 * SpO2 + 0.0250 * WBC count + 0.0422 * RDW + 0.0176 * INR + 0.0021 * PT + 0.0355 * anion gap – 0.0055 * bicarbonate + 0.0463 * blood lactic acid + 0.0026 * serum urea nitrogen + 0.2125 * (tumor = 1).
Based on the data of the training set, we developed three models altogether: the Cox regression model, the LASSO regression model, and the CoxBoost model. After extensive evaluation, the CoxBoost model was chosen as the best model. The model includes 16 predictors, which can simply and effectively predict the 30-day mortality of CS patients. In external validation, the discrimination of the CoxBoost model was better than the SAPSII and the CardShock risk score. And we developed a dynamic nomogram.
Over the years, various scoring systems have been widely used in the ICU (22, 23). However, in order to be suitable for various types of critical patients, the sensitivity and specificity of SAPSII are low. And the accuracy of the results of the assessment system depends on the experience of practitioners. There are also some small studies that used SASPAII to predict the prognosis of patients with CS (24, 25). But the integrated ICU severity score cannot accurately and reliably predict the mortality of elderly patients with CS. The CoxBoost model constructed in this study was targeted at elderly patients with CS, with higher sensitivity and specificity. In the results of our study, it can be found that the performance of the CoxBoost model was relatively better than SAPSII in the both training set and validation set.
In recent years, models have been developed specifically to assess the prognosis of patients with CS, but also have some drawbacks (26, 27). For example, the IABP-SHOCK II study established a scoring system for predicting 30-day mortality in patients with CS (9), but it is more suitable for patients with CS after emergency percutaneous coronary intervention (PCI). The CardShock risk score (10) need complicated calculation and scoring when it is used and partly depend on the subjective judgment of clinicians. Hongisto et al. evaluated the prognostic ability of two models in elderly patients with CS and the results showed that the predictive power was not very good (28). The CoxBoost model was applicable to all types of elderly patients, and external verification showed that its prediction ability was better than the CardShock risk score. Last but not least, the CoxBoost model could evaluate the short-term prognosis of elderly CS patients by simply collecting patients' vital signs, simple laboratory data, and relevant medical history at admission, all the selected predictors are objective data. The dynamic nomogram further simplified the use of the model through an easy-to-use web page.
The 16 factors that made up the CoxBoost model included all the predictive factors in the cox model and LASSO model, which showed that the CoxBoost approach has better performance and accuracy. In the factors that made up the CoxBoost model, blood lactic acid, WBC count, and anion gap were the three most important laboratory indicators. Among them, blood lactate was recognized as an important independent prognostic factor for CS (29–31). WBC count could assess the prognosis of patients, which may be related to the systemic inflammatory response caused by hypoperfusion during CS (32, 33). But the mechanism for the anion gap's prediction was unclear. Zhang et al. found that the anion gap was identified as a significant predictor of poor prognosis in patients with CS (34). It could be linked to lactic acid levels and keto, because elevated serum anion gap is usually associated with excess production of organic acid anions and reduced anion excretion (35). More experiments are needed to confirm this.
This research was a multi-center retrospective study, the main advantage was that the CoxBoost model was used for the first time to predict the 30-day mortality of elderly patients with CS. Multiple validation methods were used to compare the CoxBoost model with traditional regression analysis, LASSO regression analysis, and clinical scoring systems. The dynamic nomogram was developed to facilitate users. However, there are some limitations in this study: first, due to the relatively low incidence of CS, the sample size of patients selected in our study was relatively small. Second, there are potential differences between the training set and the validation set in terms of patient demography and research years. Third, the objective limitations of the database may produce bias. Such as the use of diagnostic codes to select patients may occur selective bias to a certain extent. Or cause a lack of potential predictors. Fourth, there was no further exploration of the database, which may make the model imperfect. We will expand the sample size and refine the sample data to conduct more in-depth research to validate our model. Overall, we believe that the model developed in this study may be useful in evaluating the prognosis of elderly patients with CS.
In conclusion, this study showed that in predicting the 30-day mortality of elderly patients with CS, the CoxBoost model was superior to the Cox regression model, LASSO regression model, SAPSII, and the CardShock risk score. It is a simple and objective score that can be applied in clinical practice.
The raw data supporting the conclusions of this article will be made available by the authors, without undue reservation.
The studies involving human participants were reviewed and approved by the Medical Ethics Committee of the Second Affiliated Hospital and Yuying Children's Hospital of Wenzhou Medical University. Written informed consent for participation was not required for this study in accordance with the national legislation and the institutional requirements.
KJ took responsibility for the content of the manuscript, including the data and analysis. FR had full access to all of the data in the study and take responsibility for the integrity of the data and the accuracy of the data analysis. All authors contributed substantially to the study design, data analysis and interpretation, and the writing of the manuscript. All authors contributed to the article and approved the submitted version.
The authors declare that the research was conducted in the absence of any commercial or financial relationships that could be construed as a potential conflict of interest.
All claims expressed in this article are solely those of the authors and do not necessarily represent those of their affiliated organizations, or those of the publisher, the editors and the reviewers. Any product that may be evaluated in this article, or claim that may be made by its manufacturer, is not guaranteed or endorsed by the publisher.
We thank for the Second Affiliated Hospital and Yuying Children's Hospital of WMU for supporting our work.
The Supplementary Material for this article can be found online at: https://www.frontiersin.org/articles/10.3389/fcvm.2022.849688/full#supplementary-material
1. Cecconi M, De Backer D, Antonelli M, Beale R, Bakker J, Hofer C, et al. Consensus on circulatory shock and hemodynamic monitoring. Task force of the European Society of Intensive Care Medicine. Intensive Care Med. (2014) 40:1795–815. doi: 10.1007/s00134-014-3525-z
2. Harjola V-P, Lassus J, Sionis A, Køber L, Tarvasmäki T, Spinar J, et al. Corrigendum to “Clinical picture and risk prediction of short-term mortality in cardiogenic shock” Eur J Heart Fail. 2015 17:501–9. Eur J Heart Fail. (2015) 17:984. doi: 10.1002/ejhf.349
3. Puymirat E, Fagon JY, Aegerter P, Diehl JL, Monnier A, Hauw-Berlemont C, et al. Cardiogenic shock in intensive care units: evolution of prevalence, patient profile, management and outcomes, 1997–2012. Eur J Heart Fail. (2017) 19:192–200. doi: 10.1002/ejhf.646
4. Azoulay E, Adrie C, De Lassence A, Pochard F, Moreau D, Thiery G, et al. Determinants of postintensive care unit mortality: a prospective multicenter study. Crit Care Med. (2003) 31:428–32. doi: 10.1097/01.CCM.0000048622.01013.88
5. Knaus WA, Wagner DP, Zimmerman JE, Draper EA. Variations in mortality and length of stay in intensive care units. Ann Intern Med. (1993) 118:753–61. doi: 10.7326/0003-4819-118-10-199305150-00001
6. Aissaoui N, Puymirat E, Juilliere Y, Jourdain P, Blanchard D, Schiele F, et al. Fifteen-year trends in the management of cardiogenic shock and associated 1-year mortality in elderly patients with acute myocardial infarction: the FAST-MI programme. Eur J Heart Fail. (2016) 18:1144–52. doi: 10.1002/ejhf.585
7. van Diepen S, Katz JN, Albert NM, Henry TD, Jacobs AK, Kapur NK, et al. Contemporary management of cardiogenic shock: a scientific statement from the American Heart Association. Circulation. (2017) 136:e232–68. doi: 10.1161/CIR.0000000000000525
8. Shah P, Cowger JA. Cardiogenic shock. Crit Care Clin. (2014) 30:391–412. doi: 10.1016/j.ccc.2014.03.001
9. Pöss J, Köster J, Fuernau G, Eitel I, de Waha S, Ouarrak T, et al. Risk stratification for patients in cardiogenic shock after acute myocardial infarction. J Am Coll Cardiol. (2017) 69:1913–20. doi: 10.1016/j.jacc.2017.02.027
10. Harjola VP, Lassus J, Sionis A, Køber L, Tarvasmäki T, Spinar J, et al. Clinical picture and risk prediction of short-term mortality in cardiogenic shock. Eur J Heart Fail. (2015) 17:501–9. doi: 10.1002/ejhf.260
11. Deo RC. Machine learning in medicine. Circulation. (2015) 132:1920–30. doi: 10.1161/CIRCULATIONAHA.115.001593
12. Goecks J, Jalili V, Heiser LM, Gray JW. How machine learning will transform biomedicine. Cell. (2020) 181:92–101. doi: 10.1016/j.cell.2020.03.022
13. Zhang K, Zhang S, Cui W, Hong Y, Zhang G, Zhang Z. Development and validation of a sepsis mortality risk score for sepsis-3 patients in intensive care unit. Front Med. (2020) 7:609769. doi: 10.3389/fmed.2020.609769
14. Awan SE, Sohel F, Sanfilippo FM, Bennamoun M, Dwivedi G. Machine learning in heart failure: ready for prime time. Curr Opin Cardiol. (2018) 33:190–5. doi: 10.1097/HCO.0000000000000491
15. DeGregory KW, Kuiper P, DeSilvio T, Pleuss JD, Miller R, Roginski JW, et al. A review of machine learning in obesity. Obes Rev. (2018) 19:668–85. doi: 10.1111/obr.12667
16. Connor CW. Artificial intelligence and machine learning in anesthesiology. Anesthesiology. (2019) 131:1346–59. doi: 10.1097/ALN.0000000000002694
17. Currie G, Hawk KE, Rohren E, Vial A, Klein R. Machine learning and deep learning in medical imaging: intelligent imaging. J Med Imaging Radiat Sci. (2019) 50:477–87. doi: 10.1016/j.jmir.2019.09.005
18. Hou N, Li M, He L, Xie B, Wang L, Zhang R, et al. Predicting 30-days mortality for MIMIC-III patients with sepsis-3: a machine learning approach using XGboost. J Transl Med. (2020) 18:462. doi: 10.1186/s12967-020-02620-5
19. Weyer V, Binder H. A weighting approach for judging the effect of patient strata on high-dimensional risk prediction signatures. BMC Bioinformatics. (2015) 16:294. doi: 10.1186/s12859-015-0716-8
20. Johnson AE, Pollard TJ, Shen L, Lehman LW, Feng M, Ghassemi M, et al. MIMIC-III, a freely accessible critical care database. Sci Data. (2016) 3:160035. doi: 10.1038/sdata.2016.35
21. Zhang ZH, Chen L, Xu P, Hong YC. Predictive analytics with ensemble modeling in laparoscopic surgery: a technical note. Laparosc Endosc Robot Surg. (2022) 5:25–34. doi: 10.1016/j.lers.2021.12.003
22. Le Gall JR, Lemeshow S, Saulnier F. A new Simplified Acute Physiology Score (SAPS II) based on a European/North American multicenter study. JAMA. (1993) 270:2957–63. doi: 10.1001/jama.1993.03510240069035
23. Godinjak A, Iglica A, Rama A, Tančica I, Jusufović S, Ajanović A, et al. Predictive value of SAPS II and APACHE II scoring systems for patient outcome in a medical intensive care unit. Acta Med Acad. (2016) 45:97–103. doi: 10.5644/ama2006-124.165
24. Kellner P, Prondzinsky R, Pallmann L, Siegmann S, Unverzagt S, Lemm H, et al. Predictive value of outcome scores in patients suffering from cardiogenic shock complicating AMI: APACHE II, APACHE III, Elebute-Stoner, SOFA, and SAPS II. Med Klin Intensivmed Notfmed. (2013) 108:666–74. doi: 10.1007/s00063-013-0234-2
25. Popovic B, Fay R, Cravoisy-Popovic A, Levy B. Cardiac power index, mean arterial pressure, and Simplified Acute Physiology Score II are strong predictors of survival and response to revascularization in cardiogenic shock. Shock. (2014) 42:22–6. doi: 10.1097/SHK.0000000000000170
26. Rivas-Lasarte M, Sans-Roselló J, Collado-Lledó E, González-Fernández V, Noriega FJ, Hernández-Pérez FJ, et al. External validation and comparison of the CardShock and IABP-SHOCK II risk scores in real-world cardiogenic shock patients. Eur Heart J Acute Cardiovasc Care. (2020) 2020:2048872619895230. doi: 10.1177/2048872619895230
27. Miller RJH, Southern D, Wilton SB, James MT, Har B, Schnell G, et al. Comparative prognostic accuracy of risk prediction models for cardiogenic shock. J Intensive Care Med. (2020) 35:1513–9. doi: 10.1177/0885066619878125
28. Hongisto M, Lassus J, Tarvasmäki T, Sionis A, Sans-Rosello J, Tolppanen H, et al. Mortality risk prediction in elderly patients with cardiogenic shock: results from the CardShock study. ESC Heart Fail. (2021) 8:1398–407. doi: 10.1002/ehf2.13224
29. Valente S, Lazzeri C, Vecchio S, Giglioli C, Margheri M, Bernardo P, et al. Predictors of in-hospital mortality after percutaneous coronary intervention for cardiogenic shock. Int J Cardiol. (2007) 114:176–82. doi: 10.1016/j.ijcard.2006.01.024
30. Attaná P, Lazzeri C, Chiostri M, Picariello C, Gensini GF, Valente S. Lactate clearance in cardiogenic shock following ST elevation myocardial infarction: a pilot study. Acute Card Care. (2012) 14:20–6. doi: 10.3109/17482941.2011.655293
31. Li CL, Wang H, Jia M, Ma N, Meng X, Hou XT. The early dynamic behavior of lactate is linked to mortality in postcardiotomy patients with extracorporeal membrane oxygenation support: a retrospective observational study. J Thorac Cardiovasc Surg. (2015) 149:1445–50. doi: 10.1016/j.jtcvs.2014.11.052
32. Kohsaka S, Menon V, Lowe AM, Lange M, Dzavik V, Sleeper LA, et al. Systemic inflammatory response syndrome after acute myocardial infarction complicated by cardiogenic shock. Arch Intern Med. (2005) 165:1643–50. doi: 10.1001/archinte.165.14.1643
33. Stegman BM, Newby LK, Hochman JS, Ohman EM. Post-myocardial infarction cardiogenic shock is a systemic illness in need of systemic treatment: is therapeutic hypothermia one possibility? J Am Coll Cardiol. (2012) 59:644–7. doi: 10.1016/j.jacc.2011.11.010
34. Zhang T, Wang J, Li X. Association between anion gap and mortality in critically ill patients with cardiogenic shock. Int J Gen Med. (2021) 14:4765–73. doi: 10.2147/IJGM.S329150
Keywords: cardiogenic shock, intensive care unit, machine learning, CoxBoost, predictive model
Citation: Rong F, Xiang H, Qian L, Xue Y, Ji K and Yin R (2022) Machine Learning for Prediction of Outcomes in Cardiogenic Shock. Front. Cardiovasc. Med. 9:849688. doi: 10.3389/fcvm.2022.849688
Received: 06 January 2022; Accepted: 07 March 2022;
Published: 06 May 2022.
Edited by:
Francois Roubille, Université de Montpellier, FranceReviewed by:
Ole Kristian Lerche Helgestad, Odense University Hospital, DenmarkCopyright © 2022 Rong, Xiang, Qian, Xue, Ji and Yin. This is an open-access article distributed under the terms of the Creative Commons Attribution License (CC BY). The use, distribution or reproduction in other forums is permitted, provided the original author(s) and the copyright owner(s) are credited and that the original publication in this journal is cited, in accordance with accepted academic practice. No use, distribution or reproduction is permitted which does not comply with these terms.
*Correspondence: Kangting Ji, amlrdEB3bXUuZWR1LmNu; Ripen Yin, MTU5NDg2NTdAcXEuY29t
†These authors have contributed equally to this work
Disclaimer: All claims expressed in this article are solely those of the authors and do not necessarily represent those of their affiliated organizations, or those of the publisher, the editors and the reviewers. Any product that may be evaluated in this article or claim that may be made by its manufacturer is not guaranteed or endorsed by the publisher.
Research integrity at Frontiers
Learn more about the work of our research integrity team to safeguard the quality of each article we publish.