- Department of Radiology, Affiliated Hospital of Nanjing University of Chinese Medicine, Nanjing, China
Objectives: To investigate the association between the perivascular adipose tissue (PVAT) fat attenuation index (FAI) derived from coronary computed tomography angiography (CCTA) and the prevalence of in-stent restenosis (ISR) in patients with coronary stent implantation.
Methods: A total of 117 patients with previous coronary stenting referred for invasive coronary angiography (ICA) were enrolled in this retrospective observational analysis. All patients underwent CCTA between July 2016 and November 2021. The deep learning-based (DL-based) method was used to analyze and measure the peri-stent FAI value. Additionally, the relationship between hematological and biochemical parameters collected from all the patients was also explored. The least absolute shrinkage and selection operator (LASSO) method was applied to the most useful feature selection, and binary logistic regression was used to test the association between the selected features and ISR. The predictive performance for ISR of the identified subgroups was evaluated by calculating the area under the curve (AUC) of receiver operator curves plotted for each model. The Pearson correlation coefficient was used to assess the correlation of peri-stent FAI values with degrees of ISR.
Results: The peri-stent FAI values in the ISR group were significantly higher than those in the non-ISR group (−78.1 ± 6.2 HU vs. −87.2 ± 7.3 HU, p < 0.001). The predictive ISR features based on the LASSO analysis were peri-stent FAI, high-density lipoprotein cholesterol (HDL-C), apolipoprotein A1 (ApoA1), and high-sensitivity c-reactive protein (hs-CRP), with an AUC of 0.849, 0.632, 0.620, and 0.569, respectively. Binary logistic regression analysis determined that peri-stent FAI was uniquely and independently associated with ISR after adjusting for other risk factors (odds ratio [OR] 1.403; 95% CI: 1.211 to 1.625; p < 0.001). In the subgroup analysis, the AUCs of the left anterior descending coronary artery (LAD), left circumflex coronary artery (LCx), and right coronary artery (RCA) stents groups were 0.80, 0.87, and 0.96, respectively. The Pearson's correlation coefficient indicated a term moderately correlation between ISR severity and peri-stent FAI values (r = 0.579, P < 0.001).
Conclusions: The peri-stent FAI can be used as an independently non-invasive biomarker to predict ISR risk and severity after stent implantation.
Introduction
Percutaneous coronary intervention (PCI) with stent placement is a standard revascularization procedure for coronary artery disease (CAD) with significant flow stenosis (1). However, in-stent restenosis (ISR) reduces the overall efficacy of PCI. Despite the development of drug-eluting stents (DES), ISR can still lead to recurrent angina requiring additional target lesion revascularization (TLR) surgery (2, 3). A study by James et al. demonstrated that about 20% to 35% of patients with bare-metal stents (BMS) and 5% to 10% of patients with DES are diagnosed with ISR (4).
Although the cause of ISR is still challenging to elucidate, inflammation is believed to play an important role in its development and progression (5, 6). After stent placement into the coronary arteries, endothelial damage occurs in the blood vessel resulting in a substantial inflammatory response to wound healing. This stimulates vascular smooth muscle cell proliferation and extracellular matrix deposition, followed by neointimal thickening, granulation formation, and restenosis (6–8). Furthermore, perivascular adipose tissue (PVAT) also plays a pivotal role in regulating vascular homeostasis and disease. PVAT is defined as respective perivascular adipose tissue within a radial distance from the outer vessel wall equal to the diameter of the coronary artery (9). PVAT participates in complicated bidirectional interactions with adjacent vessel walls (9–13) whereby the inflamed coronary arteries release and diffuse the related signals to the PVAT, thereby inhibiting local adipogenesis (9).
The fat attenuation index (FAI) was developed as an imaging biomarker to capture and quantify changes in the attenuation of perivascular fat by inflammation, enabling early detection of coronary inflammation using conventional methods (9). Standard coronary computed tomography angiography (CCTA) allows for a three-dimensional radiomic texture assessment of the PVAT spatial gradients induced by inflammation by mapping the weighted attenuation gradients (9). It has been demonstrated that perivascular FAI based on traditional CCTA measurements can be used as a non-invasive biomarker of coronary artery inflammation, with a higher prognostic value than anatomical coronary artery stenosis or calcification (14). Moreover, further studies have confirmed that the FAI value is associated with the presence of unstable plaques in acute coronary syndromes (15) and can predict the future progression of coronary atherosclerotic plaques (16).
Several artificial intelligence (AI)-based approaches have recently been applied to PVAT quantification (17). The integration of machine learning (ML), deep learning (DL), and radiomic methods has meaningful clinical implications by revealing direct links between tissue imaging phenotyping and tissue biology (18). However, to our knowledge, there has been no investigation of the peri-stent FAI in patients who have undergone intracoronary stenting placement previously.
Therefore, this study aimed to investigate the correlation between peri-stent FAI derived from CCTA using a DL-based method with ISR after PCI. Furthermore, we also examine the feasibility of using peri-stent FAI as an independent predictor of ISR.
Materials and Methods
Study Design and Population
The local Medical Ethics Committee review board approved the study, and the requirement for written informed consent was waived for all patients due to the retrospective nature of the study. This study was conducted in line with the declaration of Helsinki. All patients who have undergone follow-up CCTA examination after a period of time of PCI with stenting placement in our hospital between July 2016 and November 2021 were enrolled in the study (Figure 1). The clinical exclusion criteria for the study were a time interval between the PCI and CCTA examination below 3 months; lack of laboratory data, patients with renal failure, a stent positioned within 10 mm proximal to the vessel or ostium, stents located at a bifurcation, overlapping stents, an anomalous coronary artery origin, and the presence of a coronary artery bypass graft. Patients whose CT images were acquired using CT scanners from different manufacturers and acquisition modes were excluded. Patients whose images could not be used to determine the presence of ISR formation within the stent lumen due to poor image quality or the presence of artifacts were not included in the study. Additionally, patients who had artifacts around the stent that affected the accuracy of the FAI measurement were also excluded. Only one stent per patient was included in the analysis. If a patient had multiple coronary stent stenosis, the most severe one was included in the ISR group. Conversely, if a patient had numerous stents without stenosis, the most visible one was included in the control group. Relevant demographics and medical history were obtained at baseline.
Laboratory Analysis
Routine blood tests were obtained from all participants within three days before and after the CCTA examination at our hospital's center. All blood samples were obtained in the morning after an overnight fast of more than 12 h. The hematological and biochemical parameters analyzed included; complete blood cell count; hemoglobin; total bilirubin, direct bilirubin, and indirect bilirubin; creatinine and creatine phosphokinase (CPK), brain natriuretic peptide (BNP), total cholesterol, high-density and low-density lipoprotein cholesterol (HDL-C and LDL-C), triglycerides (TG), apolipoprotein A1, B and E (ApoA1, ApoB, and ApoE); red cell distribution width (RDW), high-sensitivity c-reactive protein (hs-CRP), and hemoglobin A1c (HbA1c).
CCTA Protocol and Image Reconstruction
All CCTA images were acquired on a 256-slice computed tomography scanner (Brilliance iCT, Philips Healthcare, Amsterdam, The Netherlands). β-blocker (25–75 mg) was administered orally 1 h before the examination for patients with a resting heart rate (HR) above 65 beats per minute (bpm) to achieve the target HR of 65 bpm or less. Sublingual nitroglycerin (0.5 mg) was given to all patients for coronary vasodilation while the scan was being performed. A prospective electrocardiogram (ECG) triggering acquisition protocol was adopted. The parameters used were a tube voltage of 120 kV, an effective tube current-time product of 600 mAs with ECG-dependent tube current modulation, a pitch of 0.18, a gantry rotation time of 270 ms, and a detector collimation of 128 × 0.625 mm. A 370 mg iodine/mL bolus of non-ionic iodinated contrast media (iopromide) (Ultravist® Bayer Healthcare, Berlin, Germany) was injected intravenously at a rate of 4.5–6.5 mL/s [according to the body mass index (BMI)], followed by 20–40 mL saline flush. The bolus-tracking technique was used to trigger the scan process. All CCTA data were transferred to the Extended Brilliance Workspace (EBW) and the Philips Healthcare computer workstation was used for data analysis. Multi-planar, curved-planar, and maximum intensity projection (MIP) images were reconstructed from the CCTA data and used for observing the coronary arteries.
Image Analysis
The detected target vessels included the left anterior descending coronary artery (LAD), the left circumflex coronary artery (LCx), and the right coronary artery (RCA). Each vessel was evaluated by two experienced cardiac radiologists (BQ and HZ, with 12 years and 8 years of experience in interpreting cardiac imaging, respectively) who were blinded to the clinical results of the patients. The definition of ISR is recurrent diameter stenosis at the stent segment or its edges (5 mm segments adjacent to the stent) compared to the normal proximal lumen on images orthogonal to the vessel (19). The stent characteristics, including locations, segments, length, and diameter, were recorded by the radiologists and any disagreement was resolved by consensus. The degrees of ISR were obtained by measure the diameter in a cross-sectional view, and was calculated as (normal proximal lumen diameter-minimal stent segment diameter)/ (normal proximal lumen diameter) ×100%. The mean of the stent length, diameter, and degrees of ISR was calculated according to inter-rater agreement analysis.
DL-based FAI measurements were carried out on the CCTA analysis platform of Shukun Technology (Perivascular Fat Analysis Tool, Shukun Technology Co., Beijing, China.), using a pre-trained convolutional neural network (CNN) fully automated DL algorithm as follows (Figure 2). First, the CCTA datasets were converted into three-dimensional (3D) volume data, and the coronary arteries were then detected and inputted to a neural network based on a 3D-resnet architecture for structural positioning. The major arteries and branches were then reconstructed and visualized as a multi-planar reformatted volume. Subsequently, we marked the neural network and named each artery, extracted the centerline, and segmented the lumens. Afterward, the cross-sectional image perpendicular to the centerline of the blood vessel was reconstructed, and the attenuation values of the corresponding voxels around the arteries were estimated for the FAI calculation. Finally, the measured area was positioned cover the stent, and the radial distance was manually adjusted so that the diameter was equal to that of the stent. Peri-stent FAI was automatically calculated based on the PVAT attenuation histogram for Hounsfield units (HU) within the range of −190 to −30 HU as previously studied (9). In order to minimize the effects of the aortic wall on FAI outcomes, as previously mentioned, we excluded cases of stents placed within 10 mm of the most proximal coronary arteries (9, 14). The 3D rendered color gradient was used to reflect the FAI value. The two observers performed the FAI measurements independently for all the participants and the mean of FAI was calculated according to inter-rater agreement analysis.
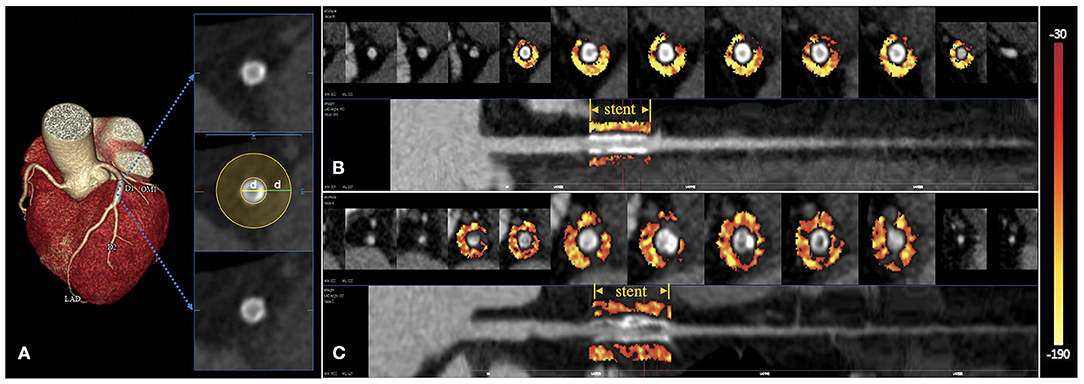
Figure 2. Peri-stent PVAT analysis by DL-based methods. The extent of peri-stent PVAT is visualized in the cross-sectional and straightened views through the adipose tissue Hounsfield unit color table (range −190 HU to −30HU), representing the peri-stent FAI values. (A) The peri-stent PVAT was defined as epicardial adipose tissue within a radial distance equal to the diameter of the stent. (B) A patient with mid-LAD stent placement. CCTA suggested a non-ISR with a peri-stent FAI of −96 HU. (C) Another patient with proximal-LAD stent implantation. ISR can be seen on the CCTA with a peri-stent FAI of −75 HU.
Statistical Analyses
All statistical analyses were performed on the statistical package for the social sciences (SPSS) statistical software (IBM Co., Armonk, NY, USA) version 26.0, and the R software version 3.6.3 (R Development Core Team). The Prism 9.1.1 software package (GraphPad Prism, San Diego, CA, USA) was used for data presentation. Inter-rater agreement was estimated as Intra-class correlation coefficients (ICC). The least absolute shrinkage and selection operator (LASSO) regression with 10-fold cross-validation was conducted to screen candidate variables with minimum lambda (model 1) and optimal lambda (model 2) to identify the predictors for ISR. The best predictive model was identified by calculating the area under the curve (AUC) of the receiver operative characteristic (ROC) curves plotted for each model. Binary logistic regression analysis determined the association of predictive characteristics with ISR. The Pearson correlation coefficient was calculated to evaluate the relationship between ISR severity and peri-stent FAI values. ROC analysis was also performed to assess the predictive value of selected variables in the diagnosis of ISR and the diagnostic efficacy of peri-stent FAI value for the three major coronary subgroups. A p-value of < 0.05 was deemed statistically significant for all statistical tests.
Results
Clinical Characteristics of the Patients
A total of 117 patients with coronary artery stent implantations were enrolled in our study, 52 patients were included in the ISR group (mean age: 68.0 ± 11.0 years, 39 males), and 65 patients were included in the non-ISR group (mean age: 66.2 ± 9.9 years, 44 males). There was no significant difference in risk factors and clinical characteristics between the two groups. The baseline and clinical characteristics of the study population are presented in Table 1.
Comparison of Laboratory Parameters Between the ISR and Control Groups
The differences in the complete blood cell count, hemoglobin; total bilirubin, direct bilirubin, and indirect bilirubin; creatinine; BNP, total cholesterol, LDL-C, TG, ApoB, ApoE, RDW, hs-CRP, and HbA1c between the two groups were not significant. However, when compared with the control group, the CPK in the ISR group was significantly higher (p = 0.034), while the HDL-C (p = 0.017) and ApoA1 (p = 0.026) in the ISR group were significantly lower. Statistical analyses of hematological and biochemical parameters are described in Table 2.
Stent Characteristics and Peri-Stent FAI Values
The ICCs were 0.978 for stent length (95% CI 0.943–0.992, p < 0.001), 0.986 for stent diameter (95% CI 0.965–0.992, p < 0.001), 0.927 for degrees of ISR (95% CI 0.838–0.975, p < 0.001), correspondingly. There were more cases with LAD stents in both groups, with 33 in the ISR group (63.5%) and 40 in the non-ISR group (61.5%). However, there was no significant difference in the stent locations between the two groups. Among the included cases, the stents located in the distal segment of the coronary artery were less, and most of them were located in the proximal and middle segments. The position, length and diameter of the stents did not differ significantly between the two groups. The DL peri-stent FAI values in the ISR (−78.1 ± 6.2 HU) group were significantly higher when compared with those in the non-ISR group (−87.2 ± 7.3 HU), (p < 0.001). The ICC for the peri-stent FAI was 0.985 (95% CI 0.975–0.991, p < 0.001), The statistical analyses of the stent characteristics and peri-stent FAI values are summarized in Table 3.
Lasso Regression and Binary Logistic Regression Analyses
The Lasso regression analysis included all risk parameters mentioned previously (Tables 1–3) and was performed to reduce the risk of overfitting the models and to identify the predictive factors for further analysis (Figure 3). Model 1 included four candidate variables (peri-stent FAI, HDL-C, ApoA1, and hs-CRP) with a minimum lambda of 0.0624, and an AUC of 0.868. On the other hand, model 2 relied on only one candidate variable, i.e., peri-stent FAI, with an optimal lambda of 0.109, and an AUC of 0.849. Based on these findings, we selected peri-stent FAI, HDL-C, ApoA1, and hs-CRP (Model 1) as predictive factors for ISR. In the binary logistic regression analysis, peri-stent FAI was uniquely and independently associated with ISR after adjusting for age, gender, and risk factors (odds ratio [OR] 1.403; 95% CI: 1.211 to 1.625; p < 0.001) (Figure 4, Table 4).
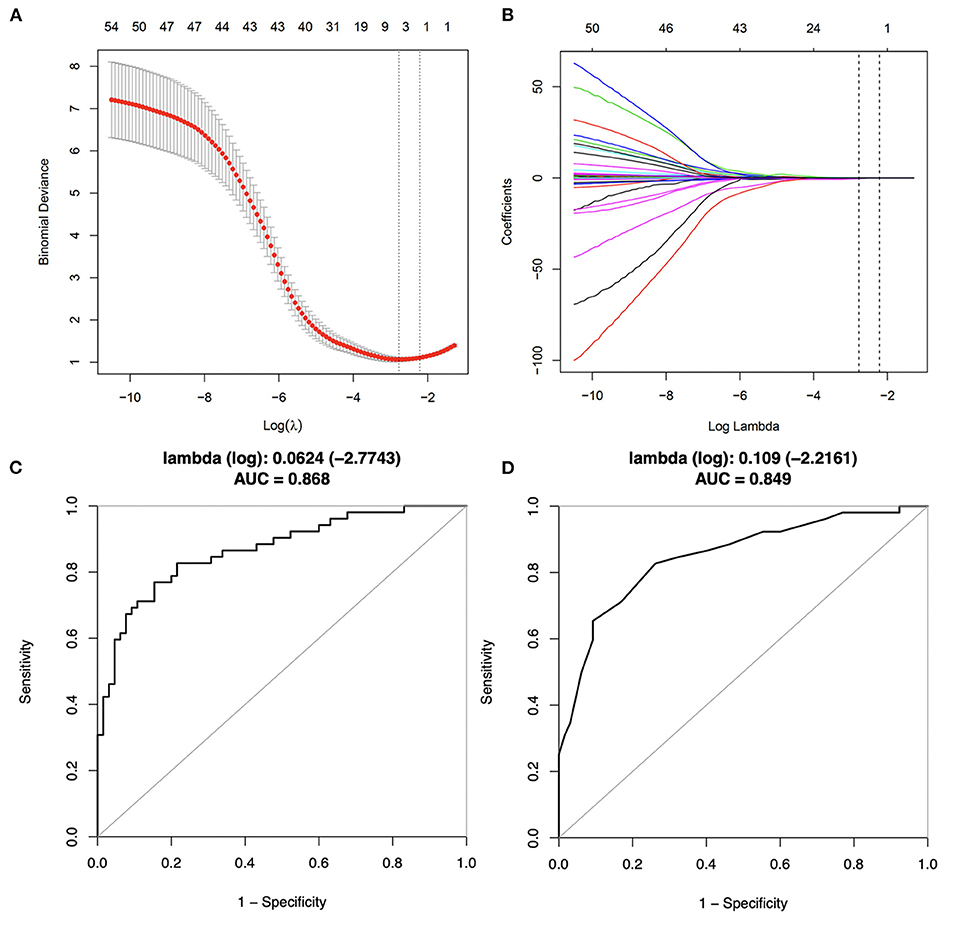
Figure 3. Predictive variables selection using Lasso regression with 10-fold cross-validation. (A) Binomial deviance was plotted vs. log (lambda). A total of 46 candidate variables were included. Dotted lines are depicted at the optimal values by minimum criteria (lambda. min, left vertical dotted line) and 1-SE criteria (lambda.1se, right vertical dotted line). (B) Coefficients plots were produced against the log (lambda) sequence, profiling all the clinical features. (C) Corresponding ROC curve of model 1, with a minimum lambda of 0.0624 and an AUC value of 0.868. (D) Corresponding ROC curve of model 2, with an optimal lambda of 0.109 and an AUC value of 0.849.
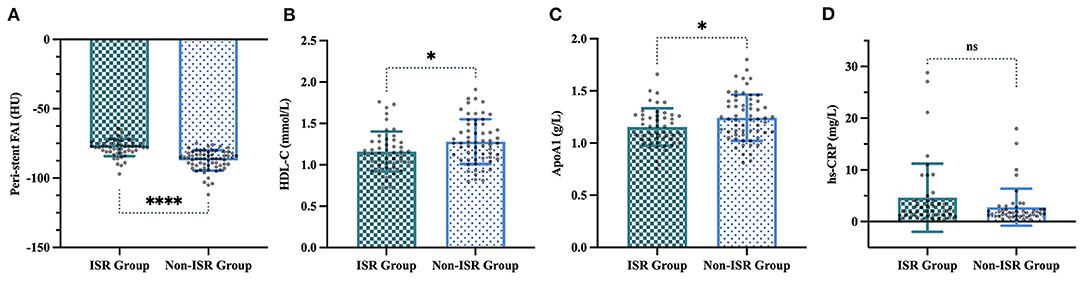
Figure 4. Four variables selected by LASSO regression in the ISR and non-ISR groups. (A) Peri-stent FAI values were −78.1 ± 6.2 HU in the ISR group compared with −87.2 ± 7.3 HU in the non-ISR group (p < 0.001). (B) HDL-C, (1.2 ± 0.2 mmol/L vs. 1.3 ± 0.3 mmol/L, p < 0.05). (C) ApoA1, (1.1 ± 0.2 g/L vs. 1.2 ± 0.2 g/L, p < 0.05). (D) Hs-CRP, (4.6 ± 6.6 mg/L vs. 2.8 ± 3.6 mg/L, p = 0.107). “*”, “****” and “ns” indicate p < 0.05, p < 0.001 and non-significant, respectively.
Predictive Performance of the ISR Testing Parameters
The ROC analysis showed that the peri-stent FAI had the highest prediction performance (AUC: 0.8485, 95% CI, 0.777–0.920) for ISR, followed by HDL-C (AUC: 0.6318 (95% CI, 0.527–0.737), ApoA1, [AUC: 0.6202 (95% CI, 0.514–0.726)] and hs-CRP for predictive performance (AUC: 0.5685, 95% CI, 0.446–0.691) (Figure 5). The cutoff value of peri-stent FAI was 82.5 HU (82.69% sensitivity and 73.85% specificity).
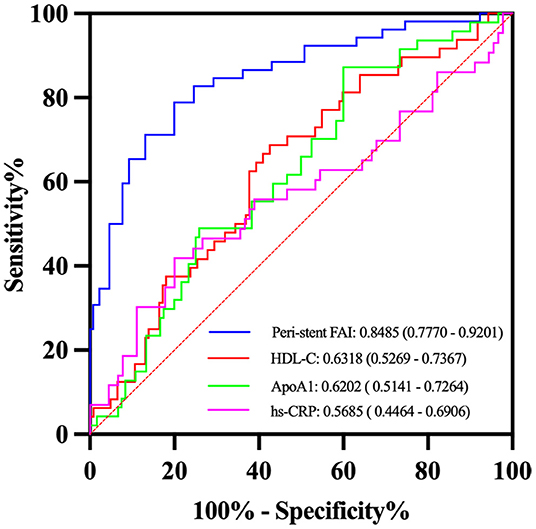
Figure 5. Receiver operator characteristic (ROC) curves analysis of selected variables for detection of ISR. The peri-stent FAI had the best performance with an AUC value of 0.85.
Subgroup Analysis of the Three Major Coronary Arteries
In subgroup analysis, there were 73 patients in the LAD subgroup (40 non-ISR and 33 ISR), 20 patients in the LCx subgroup (9 non-ISR and 11 ISR), and 24 patients in the RCA subgroup (16 non-ISR and 8 ISR). In the LAD subgroup, the peri-stent FAI was significantly lower for the non-ISR group (−86.1 ± 6.0 HU) when compared with the ISR group (−79.5 ± 5.8 HU) (p < 0.001). In the LCx and RCA subgroups, the peri-stent FAI also showed significant differences between the non-ISR and ISR participants (−85.9 ± 6.0 HU vs. −75.7 ± 7.0 HU, p < 0.01 and −90.9 ± 9.9 HU vs. −75.2±5.4 HU, p < 0.001, respectively). The complete subgroup analyses results are presented in Table 5. The AUC values for ISR prediction were 0.80, 0.87, and 0.96, for LAD, LCx, and RCA subgroups respectively (Figure 6).
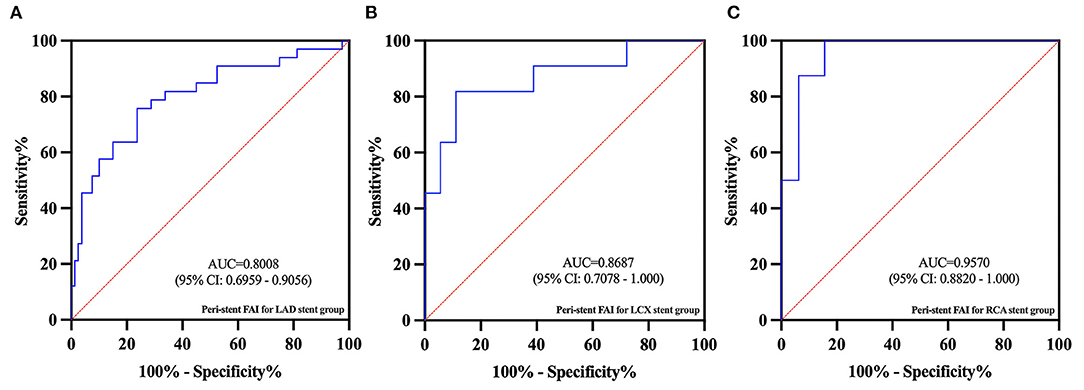
Figure 6. In the subgroup ROC analysis, the AUC values for ISR predicted by peri-stent FAI in the LAD (A), LCx (B), and RCA (C) subgroups were 0.80, 0.87, and 0.96, respectively.
Correlation of Peri-Stent FAI Values With ISR Severity
Peri-stent FAI had the term moderately positive correlation with ISR severity with a Pearson correlation coefficient of r = 0.579, P < 0.001 (95% CI, 0.36–0.74) (Figure 7).
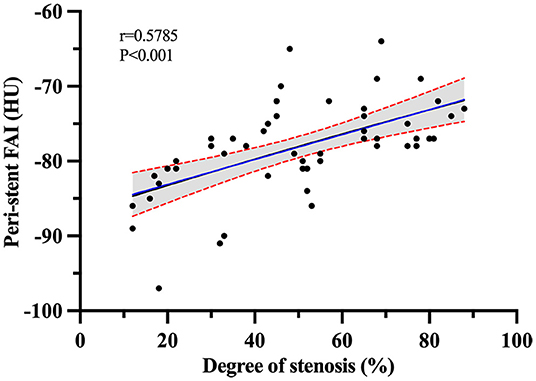
Figure 7. Pearson r correlation analysis between ISR severity and peri-stent FAI values in the ISR group.
Discussion
To the best of our knowledge, this is the first study evaluating the value of peri-stent FAI to detect PVAT inflammation around coronary artery stents and its correlation with ISR. Our findings indicate that as a CCTA-derived biomarker of coronary inflammation based on quantification of PVAT attenuation shifts (20), peri-stent FAI can be used as an independent predictor for ISR after stent implantation. Notably, this significant difference goes beyond hematological and biochemical parameters and has an important clinical value.
Although the development of DESs with antiproliferative drugs reduced the incidence of ISR when compared with BMSs, the incidence of ISR still ranges from 5 to 10% (21). In addition, the incidence of late stent thrombosis in DESs was significantly higher than that in BMSs due to delayed arterial healing (22, 23). Both BMS-ISR and DES-ISR have the same traditional mechanisms for pathogenesis, including biological, mechanical, and technical factors (24). Although DESs minimize the neointimal proliferation and improve the healing process after stent implantation compared to BMSs, high sensitivity to polymers and drugs, local inflammation, and delayed healing may still cause neointimal formation (25).
ISR may be caused by various mechanisms, including inflammation and oxidative stress. Inflammation is considered to play a key role in the ISR process (5, 6). Optical coherence tomography (OCT) studies had revealed that early ISR was characterized by homogeneous neointimal hyperplasia, while late ISR appeared as neoatherosclerosis with thin-cap fibroatheroma and lipid-rich neointima (26). Inflammation has long been implicated in atherogenesis and this has been confirmed by recent clinical trials; therefore, inflammation is also known as an important factor in the restenosis process (27–29). Histological studies from the retrieved ISR tissues revealed the substantial inflammation full of foamy macrophages and T-cell infiltration (30). Pathology studies also showed that although second-generation DESs suggest less inflammation but similar rate of neoatherosclerosis compared to first-generation ones (31). The inflammatory response processes include platelet deposition, leukocyte recruitment, and smooth muscle cell migration and proliferation (32). PVAT exerts paracrine effects on the vascular wall, which promote atherosclerosis through outward-to-inward signaling (33).
Positron emission tomography (PET) imaging is the gold standard in evaluating PVAT inflammation which is assessed by measuring the uptake of 18fluorodeoxyglucose. Still, its use is limited by its high exposure, high cost, and low clinical availability (34). CCTA is a more widely available modality that can detect PVAT changes as a response to inflammation and provide accurate information on ISR. Therefore, it is an ideal non-invasive tool that could be used to follow-up patients following the implantation of coronary artery stents (9, 35). The intravascular inflammatory response alters the composition of PVAT, shifting its FAI value from the lipid (closer to −190 HU) to the aqueous phase (closer to −30 HU), which had been demonstrated by histology (9). Previous clinical studies showed a significant association between CAD and higher perivascular FAI values. FAI changes were identified as a key factor contributing to the development of acute coronary syndromes. The increase of perivascular FAI values was associated with the development of coronary atherosclerotic plaques and acute myocardial infarction (14). Our results suggest that peri-stent FAI can provide an accessible non-invasive imaging method to identify coronary inflammation. The peri-stent PVAT of patients with ISR had a higher inflammatory status level than patients with non-ISR. It should be noted that the peri-stent FAI measurement method used in our study was not adjusted for technical, anatomical, and biological factors, which may limit the clinical value. Weighted FAI measurement (FAI-score) was developed by Oikonomou et al. to correct these factors (36), providing a more standardized metric of coronary inflammation than FAI and would have added further value to the study.
Various easily available inflammatory and oxidative biomarkers have been used to predict ISR. The hs-CRP was identified as a systemic inflammatory marker and has been significantly associated with adverse cardiovascular outcomes (37). Seo et al. determined that increased hs-CRP expressions tended to have higher ISR rates in older patients (29). Kuwano et al. demonstrated that the baseline serum bilirubin could be an independent predictor of ISR (38). Geng et al. found that patients with higher baseline RDW were more likely to have ISR at follow-up (39). Inconsistent with these findings, in our study, only HDL-C and ApoA1 were negatively correlated with ISR risk, and hs-CRP was not correlated with ISR. The differences in hematological biomarkers between the ISR and control groups were far less evident than the differences in the peri-stent FAI. Notably, some cytokines and adipokines [e.g., tumor necrosis factor-alpha (TNF-alpha), adiponectin, leptin, interleukin-6] locally secreted by PVAT may affect vascular physiology and pathology (40). It may be more valuable to investigate these cytokines or adipokines than the liver-derived systemic inflammation markers. Elnabawi et al. found that biologic therapy such as anti-TNF-alpha, anti-interleukin for moderate to severe psoriasis was associated with reduced coronary inflammation assessed by FAI (41).
Although the sample size in this study was small in the subgroup analysis, the RCA subgroup had the highest risk for the development of ISR. This finding can be ascribed to the fact that the RCA is surrounded by more adipose tissue when compared with the other epicardial arteries. Thus, the differences in FAI values in the RCA subgroup were more evident between the ISR and the non-ISR groups (9). It is important to note that despite blooming artifacts and image noise caused by the stents in some cases, it was relatively easy to visualize the stents and measure the FAI in most cases, low variability of intra-observer in the study also proves the feasibility of measuring FAI around the stent.
Our findings also show a term moderately positive correlation between the PVAT inflammation with the severity of ISR, indicating that the peri-stent FAI had a moderate correlation with the severity of ISR. McDonald et al. have shown that a reduction in the inflammatory response may improve long-term clinical outcomes (42). Therefore peri-stent FAI could be used to monitor the progression of ISR and the effectiveness of anti-inflammatory treatments, which would facilitate a more personalized approach to individual care.
This study has some limitations that have to be acknowledged. First, the study was based on a small sample size from a single center, limiting the generalizability of the research findings. Extensive prospective studies are needed to confirm the impact of peri-stent FAI changes on the ISR severity and progression. Furthermore, studies have shown that the clinical presentation, pathogenesis, lesion morphology, and response to intervention between the BMS-ISR and DES-ISR differ significantly (43). In addition, other stent characteristics may have an impact on the predictive value of FAI changes. However, due to the retrospective limited sample size, it was not possible to evaluate the differences between different types of stents and different polymer-coating types of DESs, and no more comprehensive data about cytokines and adipokines were available. Furthermore, the impact of different ISR components on the degree of PVAT inflammation and weighted FAI measurement were not evaluated in this study, highlighting the need for further studies. Finally, the judgment on ISR in this study was based on CCTA. Although CCTA is deemed an appropriate non-invasive angiographic tool for the follow-up of patients with ISR (35), digital subtraction angiography (DSA) is still the gold standard. Future studies should therefore compare the accuracy of our method with DSA.
Conclusion
Our retrospective study has shown that the ISR is associated with the inflammation of peri-stent PVAT. Peri-stent FAI can facilitate ISR identification, and its use is therefore recommended as an independent non-invasive biomarker to predict ISR occurrence after PCI with stenting placement.
Data Availability Statement
The original contributions presented in the study are included in the article/supplementary material, further inquiries can be directed to the corresponding author.
Ethics Statement
The studies involving human participants were reviewed and approved by Medical Ethics Committee of Affiliated Hospital of Nanjing University of Chinese Medicine. Written informed consent for participation was not required for this study in accordance with the national legislation and the institutional requirements. Written informed consent was not obtained from the individual(s) for the publication of any potentially identifiable images or data included in this article.
Author Contributions
BQ and ZW designed the study, wrote the manuscript, and conducted statistical analysis. ZL conducted data inspection and validation. HZ and YL revised the manuscript. HW conducted data collection. All authors contributed to the article and approved the submitted version.
Funding
The present study has received funding from the Postgraduate Research & Practice Innovation Program of Jiangsu Province (grant number: SJCX21_0784).
Conflict of Interest
The authors declare that the research was conducted in the absence of any commercial or financial relationships that could be construed as a potential conflict of interest.
Publisher's Note
All claims expressed in this article are solely those of the authors and do not necessarily represent those of their affiliated organizations, or those of the publisher, the editors and the reviewers. Any product that may be evaluated in this article, or claim that may be made by its manufacturer, is not guaranteed or endorsed by the publisher.
Acknowledgments
We would like to thank TopEdit (www.topeditsci.com) for the English language editing of this manuscript.
References
1. Smith Jr SC, Dove JT, Jacobs AK, Kennedy JW, Kereiakes D, Kern MJ, et al. ACC/AHA guidelines for percutaneous coronary intervention (revision of the 1993 PTCA guidelines)-executive summary: a report of the American College of Cardiology/American Heart Association task force on practice guidelines (committee to revise the 1993 guidelines for percutaneous transluminal coronary angioplasty) endorsed by the society for cardiac angiography and interventions. Circulation. (2001) 103:3019–41. doi: 10.1161/01.CIR.103.24.3019
2. Abbate A, Biondi-Zoccai GG, Agostoni P, Lipinski MJ, Vetrovec GW. Recurrent angina after coronary revascularization: a clinical challenge. Eur Heart J. (2007) 28:1057–65. doi: 10.1093/eurheartj/ehl562
3. Byrne RA, Joner M, Kastrati A. Stent thrombosis and restenosis: what have we learned and where are we going? the andreas gruntzig lecture ESC 2014. Eur Heart J. (2015) 36:3320–31. doi: 10.1093/eurheartj/ehv511
4. James SK, Stenestrand U, Lindbäck J, Carlsson J, Scherstén F, Nilsson T, et al. Long-term safety and efficacy of drug-eluting versus baremetal stents in Sweden. N Engl J Med. (2009) 360:1933–45. doi: 10.1056/NEJMoa0809902
5. Kornowski R, Hong MK, Tio FO, Bramwell O, Wu H, Leon MB. In-stent restenosis: contributions of inflammatory responses and arterial injury to neointimal hyperplasia. J Am Coll Cardiol. (1998) 31:224–30. doi: 10.1016/S0735-1097(97)00450-6
6. Welt FG, Rogers C. Inflammation and restenosis in the stent era. Arterioscler Thromb Vasc Biol. (2002) 22:1769–76. doi: 10.1161/01.ATV.0000037100.44766.5B
7. Khouzam RN, Shaheen M, Aziz RK, Ibebuogu UN. The important role of inflammatory biomarkers pre and post bare-metal and drug-eluting stent implantation. Can J Cardiol. (2012) 28:700–5. doi: 10.1016/j.cjca.2012.05.012
8. Hoffmann R, Mintz GS, Dussaillant GR, Popma JJ, Pichard AD, Satler LF, et al. Patterns and mechanisms of in-stent restenosis. a serial intravascular ultrasound study. Circulation. (1996) 94:1247–54. doi: 10.1161/01.CIR.94.6.1247
9. Antonopoulos AS, Sanna F, Sabharwal N, Thomas S, Oikonomou EK, Herdman L, et al. Detecting human coronary inflammation by imaging perivascular fat. Sci Transl Med. (2017) 9: eaal2658. doi: 10.1126/scitranslmed.aal2658
10. Antonopoulos AS, Margaritis M, Coutinho P, Shirodaria C, Psarros C, Herdman L, et al. Adiponectin as a link between type 2 diabetes and vascular NADPH oxidase activity in the human arterial wall: the regulatory role of perivascular adipose tissue. Diabetes. (2015) 64:2207–19. doi: 10.2337/db14-1011
11. Margaritis M, Antonopoulos AS, Digby J, Lee R, Reilly S, Coutinho P, et al. Interactions between vascular wall and perivascular adipose tissue reveal novel roles for adiponectin in the regulation of endothelial nitric oxide synthase function in human vessels. Circulation. (2013) 127:2209–21. doi: 10.1161/CIRCULATIONAHA.112.001133
12. Antonopoulos AS, Margaritis M, Coutinho P, Digby J, Patel R, Psarros C, et al. Reciprocal effects of systemic inflammation and brain natriuretic peptide on adiponectin biosynthesis in adipose tissue of patients with ischemic heart disease. Arterioscler Thromb Vasc Biol. (2014) 34:2151–9. doi: 10.1161/ATVBAHA.114.303828
13. Oikonomou EK, Antoniades C. The role of adipose tissue in cardiovascular health and disease. Nat Rev Cardiol. (2019) 16:83–99. doi: 10.1038/s41569-018-0097-6
14. Oikonomou EK, Marwan M, Desai MY, Mancio J, Alashi A, Centeno EH, et al. Non-invasive detection of coronary inflammation using computed tomography and prediction of residual cardiovascular risk (the CRISP CT study): a post-hoc analysis of prospective outcome data. Lancet. (2018) 392:929–39. doi: 10.1016/S0140-6736(18)31114-0
15. Goeller M, Achenbach S, Cadet S, Kwan AC, Commandeur F, Slomka PJ, et al. Pericoronary adipose tissue computed tomography attenuation and high-risk plaque characteristics in acute coronary syndrome compared with stable coronary artery disease. JAMA Cardiol. (2018) 3:858–63. doi: 10.1001/jamacardio.2018.1997
16. Goeller M, Tamarappoo BK, Kwan AC, Cadet S, Commandeur F, Razipour A, et al. Relationship between changes in pericoronary adipose tissue attenuation and coronary plaque burden quantified from coronary computed tomography angiography. Eur Heart J Cardiovasc Imaging. (2019) 20:636–43. doi: 10.1093/ehjci/jez013
17. Lin A, Kolossváry M, Motwani M, Išgum I, Maurovich-Horvat P, Slomka PJ, et al. Artificial intelligence in cardiovascular imaging for risk stratification in coronary artery disease. Radiol Cardiothorac Imaging. (2021) 3:e200512. doi: 10.1148/ryct.2021200512
18. Oikonomou EK, Siddique M, Antoniades C. Artificial intelligence in medical imaging: A radiomic guide to precision phenotyping of cardiovascular disease. Cardiovasc Res. (2020) 116:2040–54. doi: 10.1093/cvr/cvaa021
19. Tang CX, Guo BJ, Schoepf JU, Bayer RR, Liu CY, Qiao HY, et al. Feasibility and prognostic role of machine learning-based FFRCT in patients with stent implantation. Eur Radiol. (2021) 31:6592–604. doi: 10.1007/s00330-021-07922-w
20. Oikonomou EK, Williams MC, Kotanidis CP, Desai MY, Marwan M, Antonopoulos AS, et al. A novel machine learning-derived radiotranscriptomic signature of perivascular fat improves cardiac risk prediction using coronary CT angiography. Eur Heart J. (2019) 40:3529–43. doi: 10.1093/eurheartj/ehz592
21. Bønaa KH, Mannsverk J, Wiseth R, Aaberge L, Myreng Y, Nygård O, et al. Drug-eluting or bare-metal stents or coronary artery disease. N Engl J Med. (2016) 375:1242–52. doi: 10.1056/NEJMoa1607991
22. Bavry AA, Bhatt DL. Appropriate use of drug- eluting stents: balancing the reduction in reste- nosis with the concern of late thrombosis. Lancet. (2008) 371:2134–43. doi: 10.1016/S0140-6736(08)60922-8
23. Joner M, Finn AV, Farb A, Mont EK, Kolodgie FD, Ladich E, et al. Pathology of drug-eluting stents in humans: delayed healing and late thrombotic risk. J Am Coll Cardiol. (2006) 48:193–202. doi: 10.1016/j.jacc.2006.03.042
24. Piccolo R, Stefanini GG, Franzone A, Spitzer E, Blöchlinger S, Heg D, et al. Safety and efficacy of resolute zotarolimus-eluting stents compared with everolimus-eluting stents: a meta-analysis. Circ Cardiovasc Interv. (2015) 8:e002223. doi: 10.1161/CIRCINTERVENTIONS.114.002223
25. Lee SY, Hong MK, Jang Y. Formation and transformation of neointima after drug-eluting stent implantation: insights from optical coherence tomographic studies. Korean Circ J. (2017) 47:823–32. doi: 10.4070/kcj.2017.0157
26. Jinnouchi H, Kuramitsu S, Shinozaki T, Tomoi Y, Hiromasa T, Kobayashi Y, et al. Difference of tissue characteristics between early and late restenosis after second-generation drug-eluting stents implantation-an optical coherence tomography study. Circ J. (2017) 81:450–57. doi: 10.1253/circj.CJ-16-1069
27. Ross R. Atherosclerosis-an inflammatory disease. N Engl J Med. (1999) 340:115–26. doi: 10.1056/NEJM199901143400207
28. Tuñón J, Badimón L, Bochaton-Piallat ML, Cariou B, Daemen MJ, Egido J, et al. Identifying the anti-inflammatory response to lipid lowering therapy: a position paper from the working group on atherosclerosis and vascular biology of the european society of cardiology. Cardiovasc Res. (2019) 115:10–9. doi: 10.1093/cvr/cvy293
29. Seo DJ, Kim YK, Seo YH, Song IG, Kim KH, Kwon TG, et al. In-stent restenosis-prone coronary plaque composition: a retrospective virtual histology-intravascular ultrasound study. Cardiol J. (2018) 25:7–13. a2017.0124 doi: 10.5603/CJ.a2017.0124
30. Tsuji Y, Koide M, Katsura K, Fujita H, Ishibashi-Ueda H, Otsuka F. In-stent restenosis with “inflammatory” neointima following everolimus-eluting stent implantation. Int Heart J. (2018) 59:1142–5. doi: 10.1536/ihj.17-602
31. Otsuka F, Vorpahl M, Nakano M, Foerst J, Newell JB, Sakakura K., et al. Pathology of second-generation everolimus-eluting stents versus first-generation sirolimus- and paclitaxel-eluting stents in humans. Circulation. (2014) 129:211–23. doi: 10.1161/CIRCULATIONAHA.113.001790
32. Dangas GD, Claessen BE, Caixeta A, Sanidas EA, Mintz GS, Mehran R. In-stent restenosis in the drug-eluting stent era. J Am Coll Cardiol. (2010) 56:1897–907. doi: 10.1016/j.jacc.2010.07.028
33. Akoumianakis I, Antoniades C. The interplay between adipose tissue and the cardiovascular system: is fat always bad? Cardiovasc Res. (2017) 113:999–1008. doi: 10.1093/cvr/cvx111
34. Joshi NV, Vesey AT, Williams MC, Shah AS, Calvert PA, Craighead FH, et al. 18F-fluoride positron emission tomography for identification of ruptured and high-risk coronary atherosclerotic plaques: a prospective clinical trial. Lancet. (2014) 383:705–13. doi: 10.1016/S0140-6736(13)61754-7
35. Dai T, Wang JR, Hu PF. Diagnostic performance of computed tomography angiography in the detection of coronary artery in-stent restenosis: evidence from an updated meta-analysis. Eur Radiol. (2018) 28:1373–82. doi: 10.1007/s00330-017-5097-0
36. Oikonomou EK, Antonopoulos AS, Schottlander D, Marwan M, Mathers C, Tomlins P, et al. Standardized measurement of coronary inflammation using cardiovascular computed tomography: integration in clinical care as a prognostic medical device. Cardiovasc Res. (2021) 117:2677–90. doi: 10.1093/cvr/cvab286
37. Mincu RI, Jánosi RA, Vinereanu D, Rassaf T, Totzeck M. Preprocedural C-reactive protein predicts outcomes after primary percutaneous coronary intervention in patients with STelevation myocardial infarction a systematic meta-analysis. Sci Rep. (2017) 7:41530. doi: 10.1038/srep41530
38. Kuwano T, Miura SI, Shirai K, Ike A, Mori K, Shimizu T, et al. Serum levels ofbilirubin as an independent predictor of coronary in-stent restenosis: a newlook at an old molecule. J Atheroscler Thromb. (2011) 18:574–83. doi: 10.5551/jat.6643
39. Geng N, Su GS, Wang SJ, Zou DL, Pang WY, Sun YX. High red blood cell distribution width is closely associated with in-stent restenosis in patients with unstable angina pectoris. BMC Cadiovasc Disord. (2019) 19:175. doi: 10.1186/s12872-019-1159-3
40. Rafeh R, Viveiros A, Oudit GY, El-Yazbi AF. Targeting perivascular and epicardial adipose tissue inflammation: therapeutic opportunities for cardiovascular disease. Clin Sci (Lond). (2020) 134:827–51. doi: 10.1042/CS20190227
41. Elnabawi YA, Oikonomou EK, Dey AK, Mancio J, Rodante JA, Aksentijevich M, et al. Association of biologic therapy with coronary inflammation in patients with psoriasis as assessed by perivascular fat attenuation index. JAMA Cardiol. (2019) 4:885–91. doi: 10.1001/jamacardio.2019.2589
42. McDonald RA, Halliday CA, Miller AM, Diver LA, Dakin RS, Montgomery J, et al. Reducing in-stent restenosis: therapeutic manipulation of miRNA in vascular remodeling and inflammation. J Am Coll Cardiol. (2015) 65:2314–27. doi: 10.1016/j.jacc.2015.03.549
Keywords: computed tomography angiography (CTA), perivascular adipose tissue (PVAT), fat attenuation index (FAI), in-stent restenosis (ISR), stents
Citation: Qin B, Li Z, Zhou H, Liu Y, Wu H and Wang Z (2022) The Predictive Value of the Perivascular Adipose Tissue CT Fat Attenuation Index for Coronary In-stent Restenosis. Front. Cardiovasc. Med. 9:822308. doi: 10.3389/fcvm.2022.822308
Received: 25 November 2021; Accepted: 04 April 2022;
Published: 26 April 2022.
Edited by:
Cheerag Shirodaria, Oxford University Hospitals NHS Trust, United KingdomReviewed by:
Rita Pavasini, University Hospital of Ferrara, ItalyHa Won Kim, Augusta University, United States
Copyright © 2022 Qin, Li, Zhou, Liu, Wu and Wang. This is an open-access article distributed under the terms of the Creative Commons Attribution License (CC BY). The use, distribution or reproduction in other forums is permitted, provided the original author(s) and the copyright owner(s) are credited and that the original publication in this journal is cited, in accordance with accepted academic practice. No use, distribution or reproduction is permitted which does not comply with these terms.
*Correspondence: Zhongqiu Wang, emhxMjAwMXVzJiN4MDAwNDA7MTI2LmNvbQ==