Corrigendum: Genetic Determinants of Visit-to-Visit Lipid Variability: Genome-Wide Association Study in Statin-Naïve Korean Population
- 1Division of Cardiology, Department of Internal Medicine, Seoul National University Hospital, Seoul, South Korea
- 2DNA Link, Inc., Seoul, South Korea
- 3Division of Cardiology, Department of Internal Medicine, Healthcare System Gangnam Center, Seoul National University Hospital, Seoul, South Korea
- 4Department of Surgery, Healthcare System Gangnam Center, Seoul National University Hospital, Seoul, South Korea
- 5Division of Pulmonology, Department of Internal Medicine, Healthcare System Gangnam Center, Seoul National University Hospital, Seoul, South Korea
Background and Aim: There is a growing evidence that fluctuation in lipid profiles is important in cardiovascular outcomes. We aimed to identify single nucleotide polymorphism (SNP) variants associated with low-density lipoprotein-cholesterol (LDL-C) and high-density lipoprotein-cholesterol (HDL-C) variability in statin-naïve Korean subjects and evaluate their associations with coronary atherosclerosis.
Methods: In statin-naïve subjects from Gene-Environment of Interaction and phenotype cohort, we performed genome-wide association studies of lipid variability; the discovery (first) and replication (second) sets included 4,287 and 1,086 subjects, respectively. Coronary artery calcium (CAC) score and degree of coronary artery stenosis were used as outcome measures. Cholesterol variability was determined by standard deviation and average successive variability, and significant coronary atherosclerosis was defined as CAC score ≥400 or coronary stenosis ≥70%.
Results: Mean HDL-C and LDL-C level were 54 ± 12 and 123 ± 30 mg/dL in the first set and 53 ± 12 and 126 ± 29 mg/dL in the second set. APOA5 rs662799 and APOA5 rs2266788 were associated with LDL-C variability and PXDNL rs80056520, ALDH2 rs671, HECTD4 rs2074356, and CETP rs2303790 were SNPs associated for HDL-C variability. APOA5 rs662799 passed Bonferroni correction with p-value of 1.789 × 10−9. Among the SNPs associated with cholesterol variability, rs80056520 and rs2266788 variants were associated with CACS ≥400 and coronary stenosis ≥70% and rs662799 variant was associated with coronary stenosis ≥70%.
Conclusion: Two SNPs associated with LDL-C variability (APOA5 rs662799 and rs2266788) and one SNP associated with HDL-C variability (PXDNL rs80056520) were significantly associated with advanced coronary artery stenosis. Combining GWAS results with imaging parameters, our study may provide a deeper understanding of underlying pathogenic basis of the link between lipid variability and coronary atherosclerosis.
Introduction
An increased level of serum cholesterol, and more specifically, low-density lipoprotein cholesterol (LDL-C), is a well-established risk factor in the development of atherosclerosis and associated cardiovascular diseases (CVDs) (1). It is also a very common disorder, affecting 17.6% in Korean population (2), and its prevalence is continuously increasing worldwide. Besides high total cholesterol (TC) and LDL-C levels, a low high-density lipoprotein-cholesterol (HDL-C) level is a well-known risk factor of atherosclerotic CVDs (ASCVDs) (3). Several studies have consistently shown that high LDL-C and low HDL-C levels result in a deterioration in cardiovascular outcomes (4–6). Closely monitoring and effectively achieving the target cholesterol levels is thus clinically important in both primary and secondary preventions.
Recent studies show growing evidence that fluctuations in traditional cardiovascular risk factors are significantly associated with clinical outcomes, together with the high average level. Over the past decade, associations of blood pressure (BP) variability with CVD risk and outcomes have become an increasingly common finding in the cardiovascular literature (7, 8). The surge of research interest in BP variability has carried over into other cardiovascular risk factors, and the representative one is cholesterol. A higher intra-individual variability of lipid measures has also been shown to be associated with higher occurrence of adverse cardiovascular events, in patients with or without ASCVD (9–11). A post-hoc analysis from the Treating to New Target trial showed that in patients with stable coronary artery disease (CAD), visit-to-visit LDL-C variability was a powerful and independent predictor of any coronary event, any CVD event, death, myocardial infarction (MI), or stroke, independent of achieved LDL-C levels (10). In patients without history of MI and stroke, high variability of TC levels was associated with an 8% higher risk of MI and a 26% higher risk of all-cause mortality during a median follow up of 8.3 years (11). However, one major limitation addressed in these previous studies was the lack of consideration in the use of lipid-lowering agents, which might be a serious confounding factors influencing both mediator (i.e., lipid variability) and outcome variable (i.e., ASCVD risk). This can also introduce other confounders, such as treatment adherence or change in statin dose, making it difficult to prove causality in trying to analyze the effect of lipid variability.
Beside this problem of potential confounding factor, the causality for the association between lipid variability and ASCVD risk remains unclear, for which critical reasons are the difficulty in conducting a randomized controlled trial to address this question and no obvious pathophysiological and biological rationale yet. Elucidating the genetic variants that contribute to the development of lipid variability may be helpful in understanding pathophysiological mechanisms underlying the link between lipid variability and ASCVD risk. However, the genetic variants that determine visit-to-visit lipid variability has not been established in association with clinical or subclinical ASCVD.
Despite numerous studies correlating lipid variability with clinical outcomes, there is only scarce data on the effect of lipid variability on coronary atherosclerosis. Since coronary atherosclerosis is a crucial pathophysiologic mechanism involved in ASCVD, especially CAD, identifying genetic determinants of coronary atherosclerosis can provide mechanistic insights into their role in the pathogenesis of ASCVD. Imaging surrogate markers of coronary atherosclerosis, such as coronary artery calcium (CAC) and coronary artery lumen stenosis, are reported to be superior to clinical risk factors for the prediction of long-term risk of ASCVD (12). Furthermore, considering that imaging modalities can assess the pathological process of the vascular wall itself, it is not surprising that imaging markers have advantages over classic blood markers which can merely reflect a part of complex pathways leading to coronary atherosclerosis (13). Hence, in daily practice, CAC score (CACS) and degree of coronary artery luminal stenosis remain the dominant measure of calcified atherosclerosis burden and CAD severity, respectively, and both are used to guide patient management.
In this study, we aimed to investigate genetic determinants of visit-to-visit variability in LDL-C and HDL-C in statin-naïve general population using the genome-wide association studies (GWAS) approach. To discern clinical significance of single nucleotide polymorphism (SNP) variants identified by GWAS, we also evaluated SNP variants in association with CACS and coronary artery stenosis.
Methods
Study Subjects
This study was conducted as a post-hoc analysis of the previously reported Gene-Environment of Interaction and phenotype (GENIE) cohort, in which blood samples from 17,455 people (9,396 men and 8,059 women) were collected during a routine health check-up program at Seoul National University Hospital Healthcare System Gangnam Center (14). This large population-based cohort provides comprehensive data sets of genetic and phenotypic information, such as SNP, lifestyle, medical history, and biochemical biomarkers related to non-communicable diseases. Briefly, all subjects completed self-administered questionnaire, which included information on lifestyle factors, past medical history, comorbidities, and medications. The details of the cohort have been described previously (14). In the present study, subjects were eligible for study inclusion if they had taken blood tests for more than three times, thus allowing the analysis of lipid variability. According to this inclusion criteria, 6,276 subjects were eligible and screened for medical history and status among 10,349 subjects who agreed to provide genetic information. We excluded subjects at baseline and during follow-up when they were treated with lipid-lowering medications, leaving 5,373 statin-naïve subjects for the GWAS analysis. Among these subjects, GWAS was performed on two sets: first (discovery) and second (validation) set each consisted of 4,287 and 1,086 unrelated subjects, respectively, who passed quality control. Among a total study population of 5,373 subjects, CT scans obtained after lipid measurements were available in 1,801 subjects.
The Institutional Review Board (IRB) of the Seoul National University Hospital approved the storage of bio-specimens with written informed consent (IRB number H-1103-127-357). We retrospectively collected the clinical and genetic parameters, for which the IRB approved this study protocol (IRB number H-1803-081-930) and waived additional informed consent. This study was also performed in accordance with the Declaration of Helsinki.
Measurement of Anthropometric and Laboratory Data
Anthropometric measurements and blood tests were conducted as part of a general screening evaluation. Anthropometric parameters, including body weight, height, and waist circumference, were measured on the day of the examination, and body mass index (BMI) was calculated according to the formula: BMI = weight (kg)/height (m2). Waist circumference was measured at the midpoint between the lower costal margin and the iliac crest by a well-trained nurse. The blood samples were taken after 12 h fasting; complete blood cell counts, TC, triglyceride (TG), HDL-C, fasting blood glucose, glycated hemoglobin, blood urea nitrogen, creatinine, aspartate aminotransferase, alanine aminotransferase, and gamma-glutamyl transpeptidase were measured. In subjects with a TG <400 mg/dL, LDL-C was calculated using the Friedewald equation: LDL-C=TC–(HDL-C+TG/5) (15). In subjects with a TG ≥400 mg/dL, the measured LDL-C was used for analysis.
Visit-to-Visit Lipid Variability
Among the four lipid traits, including TC, TG, HDL-C, and LDL-C, we evaluated HDL-C and LDL-C variability in this study. Visit-to-visit lipid variability was defined as variability in HDL-C and LDL-C as measured at least three times during the health examinations, and four measurements of variability were used (8, 16, 17): (1) standard deviation (SD), (2) average successive variability (ASV), (3) coefficient of variation (CV), and (4) variation independent of mean (VIM). The CV was calculated by SD/mean, and VIM was calculated as 100 × SD/meanβ, where β is the regression coefficient based on natural logarithm of SD on natural logarithm of mean. This uncorrected VIM was corrected by using the formula: [VIM uncorrected×(mean of CV)]/(mean of VIM uncorrected). High and low lipid variability was defined by parameters of LDL-C or HDL-C variability above or below the median value, respectively.
Genotyping and Quality Control
The genomic DNA was extracted from peripheral blood leukocytes of the participants using QuickGene DNA blood kit L with QuickGene-610 L equipment (KURABO, Osaka, Japan) according to standard protocols. Hybridization on Affymetrix Axiom KORV1.0–96 array Axiom2.0 Reagent Kit (Affymetrix, Santa Clara, CA, USA) was used according to the manufacturer's protocol. Approximately 200 ng of genomic DNA was amplified and randomly fragmented into 25–125 base pair (bp) fragments. The initial amplification of Genomic DNA was performed in 40 μl reaction volume, containing 20 μl volume of genomic DNA at a concentration of 10 ng/μl and 20 μl of Denaturation Master Mix. The reaction of initial amplification was performed for 10 min at room temperature. Subsequently, the incubated products were amplified with 130 μl of Axiom 2.0 Neutral Soln, 225 μl of Axiom 2.0 Amp Soln, and 5 μl of Axiom 2.0 Amp Enzyme. The amplification reactions were conducted for 23 ± 1 h at 37°C. The amplification of products was performed under optimized reaction to amplify fragments between 200 and 1,100 bp. A fragmentation step then reduced the amplified products to segments of ~25–125 bp, which were then end-labeled using biotinylated nucleotides. Following hybridization, the bound target was washed under stringent conditions to remove non-specific background to minimize background noise caused by random ligation events. Each polymorphic nucleotide was queried via a multi-color ligation event conducted on the array surface. After ligation, the arrays were stained and imaged on the GeneTitan MC Instrument (Affymetrix, Santa Clara, CA, USA). The image was analyzed using Genotyping Console Software (Affymetrix, Santa Clara, CA, USA). Genotype data were produced using K-CHIP available through the K-CHIP consortium. The K-CHIP was designed by the Center for Genome Science, Korea National Institute of Health, Korea (4845–301, 3000–3031).
Analysis of Coronary Atherosclerosis on Computed Tomography
Coronary calcium scoring computed tomography (CT) and coronary CT angiography (CCTA) were acquired using a 16-slice scanner (Somatom Sensation 16; Siemens Medical Solutions, Forchheim, Germany) or a 256-slice multidetector CT scanner (Brilliance iCT 256; Philips Medical Systems, Cleveland, OH, USA), respectively. With regard to the time sequence between serial lipid measurements and imaging study, the initial lipid measurement preceded CT scans, while the second and further lipid measurements were performed before, at the time of, or after CT evaluation. A standard scanning protocol was used: with a tube voltage of 120 kV, 170 effective mA, and 0.37 ms rotation time for the 16-slice CT and with 128 × 0.625 mm section collimation, 0.27 ms rotation time, 120 kV tube voltage and 800 mA tube current for the 256-slice multidetector CT. All scans were performed with electrocardiogram-gated dose modulation. The CACS was calculated using commercially available CT software (Rapidia 2.8; INFINITT, Seoul, Korea) and the Agatston method (18). Significant coronary atherosclerosis was defined as a CACS ≥400 on coronary calcium scoring CT or presence of a plaque associated with ≥70% stenosis in any of the major epicardial coronary arteries on CCTA.
Statistical Analysis
The SNP determined to have genome-wide significance after Bonferroni correction in the first set was screened using the Affymetrix Axiom KORV1.0–96 array. Statistical analyses were performed using the PLINK version 1.9 (https://www.cog-genomics.org/plink2) and SAS software (SAS Institute, Cary, NC, USA). A Manhattan plot of –log10P was generated using Haploview (http://www.broadinstitute.org/haploview). Regional association plots were created using the LocusZoom (http://locuszoom.org).
The baseline characteristics of the study population were presented as mean ± SD for continuous variables and number with proportion for categorical variables, respectively. Multivariate linear regression adjusted for age and sex was used to determine the effect of SNPs on HDL-and LDL-cholesterol variability indices, which were treated as continuous variables. For the analysis examining the association of the GWAS-identified SNPs with coronary atherosclerosis, we also used multivariate linear regression adjusted for age and sex.
Results
The baseline characteristics of the study population are shown in Table 1. Briefly, the mean age of the overall study population was 52 ± 9 years and 3,096 (57.6%) were male. Among 5,373 study subjects, 639 (11.9%) subjects had hypertension, 124 (2.3%) subjects had diabetes, and 2,355 (43.8%) subjects had smoking history. Mean LDL-C and HDL-C levels in the entire study population were 124 ± 30 and 54 ± 12 mg/dL, respectively. Variability parameters of LDL-C and HDL-C are shown in Table 1.
The clinical characteristics and laboratory measurements of two sets are given in Table 1. The mean age and comorbidities including hypertension, diabetes, and smoking history did not significantly differ between the two sets (p = 0.672 for age, p = 0.834 for hypertension, p = 1.000 for diabetes, and p = 0.575 for smoking). Four parameters of HDL-C variability did not significantly differ either (p = 0.851 for SD, p = 0.749 for ASV, p = 0.248 for CV, and p = 0.179 for VIM), while all indices of LDL-C variability were significantly lower in the first set (p<0.001 for SD, p = 0.002 for ASV, p = 0.002 for CV, and p = 0.001 for VIM of LDL-C variability). Compared to the second set, BMI and mean LDL-C were significantly lower in the first set (p = 0.002 for BMI and p = 0.003 for mean LDL-C).
SNPs Associated With Visit-to-Visit Lipid Variability
Table 2 summarizes the six identified SNPs that significantly affected the variability of HDL-C and LDL-C in the first set. Specifically, APOA5 (apolipoprotein A5) rs662799 and APOA5 rs2266788 were significantly related with LDL-C variability as measured by SD and ASV. PXDNL (peroxidasin-like protein) rs80056520, ALDH2 (Aldehyde Dehydrogenase 2) rs671, HECTD4 (HECT Domain E3 Ubiquitin Protein Ligase 4) rs2074356, and CETP (cholesteryl ester transfer protein) rs2303790 were significantly associated with HDL-C variability defined as SD and ASV.
Of the six SNPs, the APOA5 variant rs662799 was significantly associated for LDL-C variability in the first set, which also passed Bonferroni correction (p = 1.789 × 10−9, p = 1.061 × 10−8, p = 2.772 × 10−7, and p = 4.632 × 10−9 for LDL-C variability by SD, CV, ASV, and VIM, respectively, Figures 1, 2). The APOA5 variant rs2266788 was significantly related with LDL-C variability (p = 5.342 × 10−5, p = 2.289 × 10−5, p = 3.546 × 10−3, p = 2.106 × 10−5 for LDL-C variability by SD, CV, ASV, and VIM, respectively). The results were similar in the second set; the APOA5 variant rs662799 and the APOA5 variant rs2266788 were SNPs significantly related with LDL-C variability (p = 1.211 × 10−3, p = 1.707 × 10−3, p = 8.268 × 10−3 and 1.388 × 10−3 for LDL-C variability by SD, CV, ASV, and VIM, respectively).
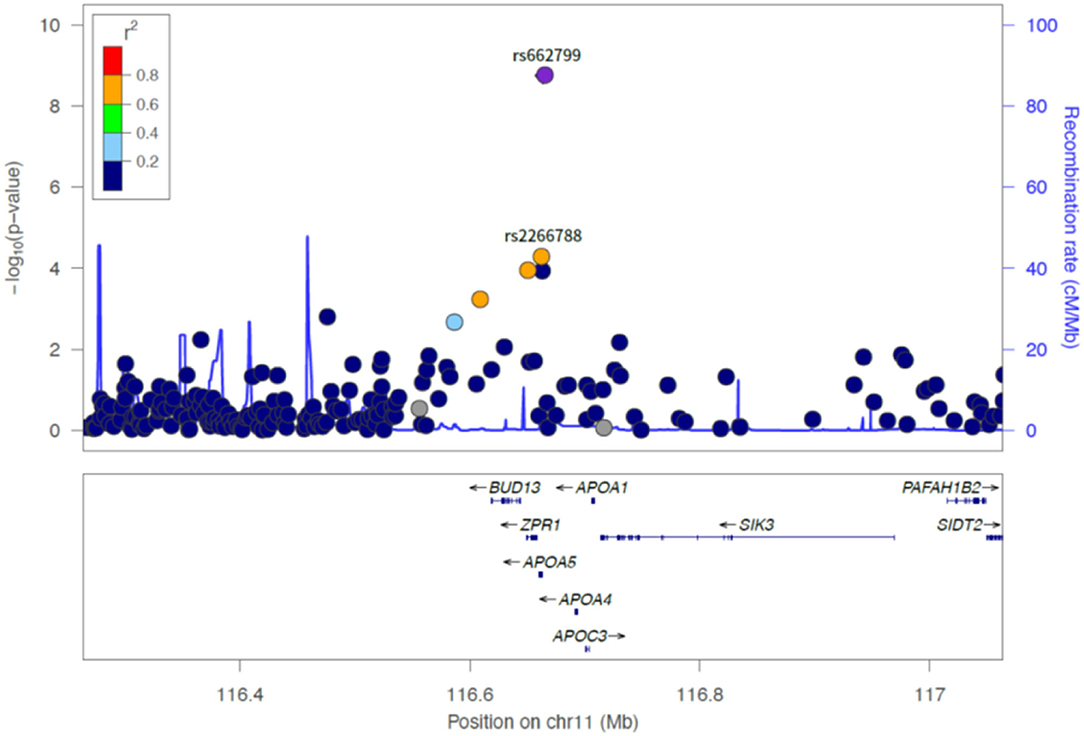
Figure 2. LocusZoom plot showing the region associated with LDL cholesterol variability near rs662799 (APOA5 gene).
The ALDH2 variant rs671 was significantly associated with HDL-C variability, with p-value passing Bonferroni correction (p = 2.466 × 10−8 in the discovery set for HDL-C variability by SD). PXDNL variant rs80056520, HECTD4 variant rs2074356, and CETP variant rs2303790 were significant determinants of HDL-C variability (p = 3.618 × 10−2, 1.03 × 10−2, p = 2.599 × 10−2, and p = 9.327 × 10−3 for PXDNL variant rs80056520 by SD, CV, ASV, and VIM, p = 6.619 × 10−7 and p = 8.695 × 10−4 for HECTD4 variant rs2074356 by SD and ASV, p = 3.918 × 10−7 and p = 1.399 × 10−4 for CETP variant rs2303790 by SD and ASV, respectively). From the second set, ALDH2 rs671, PXDNL rs80056520, HECTD4 rs2074356, and CETP rs2303790 variants were significant variants associated with HDL-C variability (p = 2.102 × 10−3 and p = 1.135 × 10−3 for ALDH2 variant rs671 by SD and ASV, p = 4.637 × 10−7, p = 6.262 × 10−7, p = 9.074 × 10−7, and p = 1.191 × 10−6 for PXDNL variant rs80056520 by SD, CV, ASV, and VIM, p = 6.243 × 10−3 and p = 2.742 × 10−3 for HECTD4 variant rs2074356 by SD and ASV, p = 5.496 × 10−4 and p = 1.299 × 10−2 for CETP variant rs2303790 by SD and ASV, respectively).
Functional Significance of GWAS-Identified SNPs for Lipid Variability in Coronary Atherosclerosis
We evaluated the association of GWAS-derived SNPs responsible for LDL-C and HDL-C variability with coronary atherosclerosis assessed by CACS and coronary artery stenosis. Three SNPs (APOA5 rs662799, APOA5 rs2266788, and PXDNL rs80056520) were identified as being associated with significant coronary atherosclerosis after adjusting for age and sex, as shown in Table 3.
Among the two genetic variants for LDL-C variability (rs2266788 and rs662799), the SNP rs2266788 in APOA5 was significantly associated with increased risks of having CACS ≥400 (adjusted odds ratio [OR] 2.196, 95% confidence interval [CI] 1.098–4.394, p = 0.0262) and coronary stenosis ≥70% (adjusted OR 3.083, 95% CI 1.18–8.639, p = 0.0323). The SNP rs662799 of APOA5 significantly increased the risk of having coronary stenosis ≥70% (adjusted OR 3.263 95% CI 1.406–7.569, p = 0.0059), but not that of having CACS ≥400.
The SNP rs80056520 in PXDNL, an identified genetic variant for HDL-C variability, was significantly associated with an increased risk of CACS ≥400 (adjusted OR 4.057, 95% CI 1.009–16.309, p = 0.0485). The SNP rs80056520 was also associated with coronary stenosis ≥70% (adjusted OR 9.101, 95% CI 1.566–52.904, p = 0.0139).
Discussion
In this study, we used a GWAS to identify SNP variants associated with LDL-C and HDL-C variability in statin-naïve Korean subjects and studied their functional significance in relation with coronary atherosclerosis. We found two novel loci (APOA5 rs662799 and APOA5 rs2266788) associated with LDL-C variability and four novel loci (PXDNL rs80056520, ALDH2 rs671, HECTD4 rs2074356, and CETP rs2303790) significantly associated with HDL-C variability. Among these variant SNPs, APOA5 rs662799 passed Bonferroni correction and was also replicated in the replication set. Moreover, we showed that three loci among the six SNP variants found were associated with significant coronary atherosclerosis, increasing the translational value of our findings, particularly in relation to clinical implications.
Clinical Significance of Lipid Variability
Together with achieving target levels for ASCVD risk factors, maintaining consistently optimal control of risk factors with fewer fluctuations is an important aspect of ASCVD prevention. Much interest has been recently engendered about the prognostic value of intra-individual variability, including BP, glucose, and lipid profiles, which are representative risk factors for ASCVD. Mounting evidence indicates that the fluctuation of these risk factors is linked with increased risks of cardiovascular and cerebrovascular outcomes (9, 10, 19–22). When focusing on lipid variability, its role as a potential predictor of future adverse events was firstly noted in patients with known CAD (9, 10, 17). To extend these results to a general population setting, Kim et al. performed a nationwide population-based study including 3,656,648 Korean subjects (11), and showed that high lipid variability was significantly associated with adverse cardiovascular events and all-cause mortality, even after adjusting multiple traditional cardiovascular risk factors (11). The exact mechanism of poor outcomes driven by lipid variability remains unclear, but one reasonable explanation is that fluctuating lipid levels are a reflection of interruption or irregular use of statins, whose association with adverse ASCVD events is well-established. Thus, in our previous study, we excluded subjects taking statins at baseline and censored those during follow-up, to minimize the confounding effects related to statin use, such as dose change and compliance, which can directly influence lipid variability (23). In this study only including from a statin-naïve young population, high lipid variability, as opposed to abnormal lipid levels, was not associated with increased risk of MI and stroke, implying the previously reported link between lipid variability and adverse ASCVD events might be confounded by statin therapy, and ultimately raising doubt about a causal role of lipid variability in the development and prognosis of ASCVD. Another possible explanation is that lipid variability hinders lipid efflux from atheroma and consequently induce plaque progression and increase its vulnerability (24, 25). However, the biological mechanisms underlying lipid variability and the association with the fate of atherosclerosis still requires further investigation. In the present study, we have selected only statin-naïve subjects to eliminate the effect of statin exposure and showed that those with SNP variants associated with LDL-C and HDL-C variability had increased risk of CACS ≥400 and coronary stenosis ≥70%, suggesting a genetic contribution to lipid variability and its link with ASCVD.
Genetic Variants Associated With LDL-C Variability
The two SNPs rs2266788 and rs662799 are previously described genetic variants associated with APOA5 (26–28), which are markers for classic hyperlipoproteinemia phenotypes and metabolic syndrome (29–31). Specifically, APOA5 induces lipolysis through the increase in lipoprotein lipase activity and facilitation in removal of lipoprotein particles (12), and thus genetic variants leading to dysfunctional APOA5 protein can result in dysregulation of lipolysis and lipid metabolism (32). In a meta-analysis of 91 studies including 51,868 subjects of Asian, European, and other ethnic populations, the APOA5 rs662799 SNP showed significant effects on TG, LDL-C, and HDL-C levels (33). The APOA5 rs2266788 minor allele carriage was also strongly associated with high TG and low HDL-C levels in another study from Korean population (26). The APOA5 rs662799 SNP showed a significant association with the risk for metabolic syndrome in various ethnic groups including Korean, Chinese, and Hungarian (30, 33, 34). Considering that all these findings suggested the role of two APOA5 SNPs rs2266788 and rs662799 in abnormal lipid levels, there seems to be relatively good biological plausibility for the association between these genetic variants of APOA5 and lipid variability observed in our study as compared with other genes and loci whose biological function is completely unknown. However, the role of APOA5 genetic variants in lipid variability cannot be directly extrapolated from the results supporting that in abnormal lipid levels. There is emerging evidence that the APOA5 genetic variants may contribute to ASCVD beyond their effects on lipid levels. For instance, a previous study demonstrated a strong association between rs662799 and the risk of early MI after adjusting for triglyceride levels, raising the possibility that this APOA5 SNP can affect the risk for early-onset MI beyond its known effects on lipid levels (12, 35). Our findings allow careful speculation that the APOA5 genetic variants may exert additional atherogenic effects that are mediated by lipid variability. Further studies are needed to investigate this speculation and therefore to gain better understanding of the genetic variants associated with lipid variability, beyond the genetic variants that are already known to cause abnormal lipid levels.
Genetic Variants Associated With HDL-C Variability
Conversely, not much is known about the effect of PXDNL rs80056520 SNP on lipid metabolism nor coronary atherosclerosis. Peterfi et al. first discovered PXDNL as a novel peroxidase homolog and found that its expression was increased in the failing myocardium (36). Although the role of PXDNL in vascular health has not been investigated clearly peroxidase, a homolog of PXDNL, is known to promote vascular disease through oxidizing lipoproteins and uncoupling endothelial nitric oxide synthase (37). Furthermore, vascular peroxidase 1 has been suggested as having a role in the regulation of lipid homeostasis and the development of atherosclerosis, by mediating ApoE oxidation and impairing plasma lipid clearance (38). Given that PXDNL is a peroxidase homolog, it can be carefully speculated that PXDNL has a similar role in lipid metabolism and atherosclerosis. Although the exact mechanism is to be elucidated, our study demonstrated for the first time that individuals with PXDNL SNP rs80056520 had higher HDL-C variability and moreover, higher risk of having significant coronary atherosclerosis defined by CACS ≥400 and coronary stenosis ≥70%, compared with their counterparts. These findings support the possibility of a genetic predisposition both in HDL-C variability and the risk of ASCVD. Other well-known genetic variants for HDL-C levels are the mutations associated with apolipoprotein A-I, adenosine triphosphate binding cassette protein A1, lecithin cholesterol acyltransferase, lipoprotein lipase, and CETP (39). From our Korean cohort, the CETP rs2303790 SNP was significantly associated with HDL-C variability, but not with the risk of CACS ≥400 nor coronary stenosis ≥70%.
Clinical Implications of Genetic Variants for Lipid Variability
In this study, we evaluated the possible link between potential lipid variability-related genes and coronary atherosclerosis in statin-naïve subjects, after excluding the confounding effect of lipid lowering agents. The novelty of our study relies in not only discovering genes related with lipid variability, but also showing their possible functional significance using CT findings.
With a tremendous improvement in diagnostic methods, CT-based screening for CAD has gained a significant role in detecting subclinical atherosclerosis, before clinically evident disease develops (40, 41). CACS is now regarded as an indicator of subclinical CAD, showing a strong correlation with the extent of atherosclerosis and an incremental predictive value over traditional cardiovascular risk factors. CACS reflects both the plaque burden and the severity of atherosclerotic changes in coronary arteries and is associated with adverse cardiovascular outcomes (42–44). CACS also has a clinically useful role in risk stratification, especially in intermediate risk subjects, which is recommended in current guidelines (12, 45). Intriguingly, a recent study showed that the appropriate age for initiating CAC testing was approximately 42 years for men and 58 years for women without ASCVD risk factors, but this age was shortened to 37 years for men and 50 years for women when they have diabetes (46), suggesting that the optimal timing of CACS screening differs according to the individual risk-factor profiles for premature development of atherosclerosis. However, no established framework is available to guide CAC testing to detect the earliest manifestations of CAC at young ages. Considering that genetic risk factors can be measured early in life and remain constant throughout the individual's lifetime, understanding and identifying genetic determinants of premature CAC may allow for a more individualized diagnostic approach. Our study supports this possibility by showing that APOA5 rs2266788 related with LDL-C variability and PXDNL rs80056520 related with HDL-C variability may be the candidate genetic variants contributing to increased risk of CAC, independent of age and gender.
Despite the ability of CACS in clinical outcome prediction, CACS of zero still cannot guarantee the absence of significant CAD and vulnerable plaques (47), since CACS only reflects calcified plaque burden. The major advantage of CCTA is its ability to assess degree of stenosis and plaque characteristics, including not only calcified plaque, but also lipid-rich plaque, non-invasively. It is therefore not surprising that CCTA provides additional information beyond CACS, and widely used in real-world practice Also, from a previous study comparing the prognostic power of clinical, biochemical, and imaging parameters in asymptomatic Korean population, degree of coronary artery stenosis measured by CCTA was an independent predictor for adverse outcomes and was a better prognostic marker than CACS (48). However, contrast agent-related adverse effects and the relatively high cost of CCTA hampers its routine use, particularly in asymptomatic population. Again, identification of genetic risk factors for significant coronary artery stenosis may enable selection of a tailored diagnostic pathway at the individual level. Although further validation is warranted, we found that APOA5 rs662799 was a significant genetic determinant of coronary artery stenosis ≥70%, but not of advanced CAC, raising the possibility that this genetic variant carries significant information about the risk of non-calcified plaque development and progression.
Applying Different Metrics for Lipid Variability Measurement
Among the four different metrics of lipid variability described in the Methods, SD, and ASV were used for the main analysis in our study. Multiple metrics have been introduced to assess visit-to-visit variability, mostly regarding blood pressure variability, which include SD, SD independent of mean (SDIM), CV, ASV, range, and others. Several studies investigated the usefulness of applying different metrics and studies showed excellent interrelationships of variability metrics with one another (49, 50). Levitan et al. (50) compared visit-to-visit variability metrics for BP variability and found that most of the parameters showed a higher agreement than would be expected by chance (p < 0.001 for all comparisons). Notably, different variability metrics seem to convey the same information. For instance, it has been suggested that SD, SDIM and CV show nearly the same information about the variability around the mean values across visits, whereas ASV reflects the variability from one visit to the next visit. We therefore chose SD and ASV from the four different metrics of lipid variability in this study, as SD is a metric of overall variability and ASV is a metric of variability between consecutive visits. When we additionally analyzed using VIM and CV, results were similar to those using SD and ARV. APOA5 rs662799 and APOA5 rs2266788 were significantly related with LDL-C variability as measured by VIM and CV. PXDNL rs80056520 was significantly related with HDL-C variability as measured by VIM and CV.
Limitations
First, since the number of the study population is not big enough to represent most of Korean population, our result should not be extrapolated to the general population. However, our result contains medical history and blood test results together with genetic analysis, allowing a more comprehensive evaluation of the clinical significance of genetic mutation. Second, our study was designed as a retrospective cohort study with the inherent problems, such as selection bias and unmeasured confounders, limiting the strength of our results. For example, the change in diet and physical activity can affect lipid variability, which could not be thoroughly measured in our study. Furthermore, knowing the result of CT scan per se may result in the change in diet and physical activity, which can in turn lead to increased lipid variability. Considering that HDL-C is known to have a strong inherited basis, although environmental factors also have a role, the confounding effects due to unmeasured variables may be relatively small when interpreting results on HDL-C variability, compared with those on LDL-C. Third, instead of taking major adverse cardiovascular events as an outcome measure, we defined study outcomes using CT findings, including coronary artery luminal stenosis and CACS, since our study population consisted of asymptomatic subjects at low risk of developing adverse clinical events. However, these imaging parameters derived from CT are increasingly being used as study endpoints for several reasons: (1) there is robust evidence that coronary artery luminal stenosis and CACS can help more accurately predict future risk of clinical ASCVD in a wide spectrum of populations, including even asymptomatic subjects without known CAD (51–55); (2) these imaging metrics are already widely used in the real-world clinical practice and their use will expand with increased availability and reliability and reduced costs, enhancing the translational applicability of research results; and (3) imaging can provide anatomic and functional information with shorter study durations and smaller sample sizes than possible with typical research assessing cardiovascular morbidity and mortality, enabling more efficient study conduct. Fourth, our study cannot determine whether high lipid variability is a disease mediator or effect moderator between SNP variants and advanced coronary atherosclerosis, for which further studies are warranted. Lastly, our data has not been validated externally in a different cohort, and should be extrapolated carefully.
Conclusion
We found six SNP variants associated with LDL-C and HDL-C variability in statin-naïve Korean population. The APOA5 rs662799 SNP was a significant determinant of LDL-C variability and also associated with significant coronary artery stenosis. Among other SNPs, APOA5 rs2266788 and PXDNL s80056520 showed significant associations with CAC ≥400 and coronary artery stenosis ≥70%. Considering that genetic variants responsible for ASCVD, especially in association with lipid variability, have not been evaluated, our findings provide the grounds for a better understanding of potential mechanism of increased ASCVD in individuals with high lipid variability, which could lead to future mechanistic research. Further studies are also warranted to determine whether therapeutic strategies targeting lipid variability can lead to the reduced risk of advanced coronary atherosclerosis and major adverse clinical events.
Data Availability Statement
The data analyzed in this study is subject to the following licenses/restrictions: Complete summary statistics of the GENIE cohort are not publicly available due to restrictions (institutional policy to protect the privacy of research participants), but are available from the corresponding author on reasonable request. However, all other data are contained in the article or are available upon reasonable request. Requests to access these datasets should be directed to aHlvZXVubWQxJiN4MDAwNDA7Z21haWwuY29t or NjU1MjkmI3gwMDA0MDtzbnVoLm9yZw==.
Ethics Statement
The Institutional Review Board (IRB) of the Seoul National University Hospital approved the storage of bio-specimens with written informed consent (IRB number H-1103-127-357). We retrospectively collected the clinical and genetic parameters, for which the IRB approved this study protocol (IRB number H-1803-081-930) and waived additional informed consent. This study was also performed in accordance with the Declaration of Helsinki.
Author Contributions
J-BP, HP, and ES: conceptualization, writing—original draft, and writing—review and editing. J-BP, HP, ES, SL, S-YC, and HL: data curation. J-BP, HP, ES, J-EL, and SL: formal analysis. J-BP, HP, S-YC, HL, ES, and J-EL: investigation. J-BP, HP, ES, S-YC, HL, and SC: methodology. J-BP, HP, ES, S-YC, EC, and SC: project administration. HP, HL, S-YC, EC, and SC: resources. J-BP, HP, ES, SL, and J-EL: software. J-BP, HP, ES, EC, and SC: supervision. ES, J-EL, SL, EC, and SC: validation. ES, J-EL, and SL: visualization. All authors contributed to the article and approved the submitted version.
Funding
This study was supported by grant no. 27-2017-0050 from the SNUH Research Fund.
Conflict of Interest
ES, SL, and J-EL are employed by DNA Link, Inc. DNA Link, Inc. provided support in the form of salaries to these authors. There are no patents, products in development or marketed products to declare.
The remaining authors declare that the research was conducted in the absence of any commercial or financial relationships that could be construed as a potential conflict of interest.
Publisher's Note
All claims expressed in this article are solely those of the authors and do not necessarily represent those of their affiliated organizations, or those of the publisher, the editors and the reviewers. Any product that may be evaluated in this article, or claim that may be made by its manufacturer, is not guaranteed or endorsed by the publisher.
References
1. Prospective Studies Collaboration, Lewington S, Whitlock G, Clarke R, Sherliker P, Emberson J, Halsey J, et al. Blood cholesterol and vascular mortality by age, sex, and blood pressure: a meta-analysis of individual data from 61 prospective studies with 55,000 vascular deaths. Lancet. (2007) 370:1829–39. doi: 10.1016/S0140-6736(07)61778-4
2. Rhee EJ. Prevalence and current management of cardiovascular risk factors in korean adults based on fact sheets. Endocrinol Metab. (2020) 35:85–94. doi: 10.3803/EnM.2020.35.1.85
3. Ference BA, Ginsberg HN, Graham I, Ray KK, Packard CJ, Bruckert E, et al. Low-density lipoproteins cause atherosclerotic cardiovascular disease. 1. evidence from genetic, epidemiologic, and clinical studies. a consensus statement from the European Atherosclerosis Society Consensus Panel. Eur Heart J. (2017) 38:2459–72. doi: 10.1093/eurheartj/ehx144
4. Briel M, Ferreira-Gonzalez I, You JJ, Karanicolas PJ, Akl EA, Wu P, et al. Association between change in high density lipoprotein cholesterol and cardiovascular disease morbidity and mortality: systematic review and meta-regression analysis. BMJ. (2009) 338:b92. doi: 10.1136/bmj.b92
5. Gordon T, Castelli WP, Hjortland MC, Kannel WB Dawber TR. High density lipoprotein as a protective factor against coronary heart disease. the Framingham study. Am J Med. (1977) 62:707–14. doi: 10.1016/0002-9343(77)90874-9
6. van der Steeg WA, Holme I, Boekholdt SM, Larsen ML, Lindahl C, Stroes ES, et al. High-density lipoprotein cholesterol, high-density lipoprotein particle size, and apolipoprotein A-I: significance for cardiovascular risk: the IDEAL and EPIC-Norfolk studies. J Am Coll Cardiol. (2008) 51:634–42. doi: 10.1016/j.jacc.2007.09.060
7. Tai C, Sun Y, Dai N, Xu D, Chen W, Wang J, et al. Prognostic significance of visit-to-visit systolic blood pressure variability: a meta-analysis of 77,299 patients. J Clin Hypertens. (2015) 17:107–15. doi: 10.1111/jch.12484
8. Rothwell PM, Howard SC, Dolan E, O'Brien E, Dobson JE, Dahlof B, et al. Prognostic significance of visit-to-visit variability, maximum systolic blood pressure, and episodic hypertension. Lancet. (2010) 375:895–905. doi: 10.1016/S0140-6736(10)60308-X
9. Boey E, Gay GM, Poh KK, Yeo TC, Tan HC Lee CH. Visit-to-visit variability in LDL- and HDL-cholesterol is associated with adverse events after ST-segment elevation myocardial infarction: a 5-year follow-up study. Atherosclerosis. (2016) 244:86–92. doi: 10.1016/j.atherosclerosis.2015.10.110
10. Bangalore S, Breazna A, DeMicco DA, Wun CC, Messerli FH, Committee TNTS and Investigators. Visit-to-visit low-density lipoprotein cholesterol variability and risk of cardiovascular outcomes: insights from the TNT trial. J Am Coll Cardiol. (2015) 65:1539–48. doi: 10.1016/j.jacc.2015.02.017
11. Kim MK, Han K, Kim HS, Park YM, Kwon HS, Yoon KH, et al. Cholesterol variability and the risk of mortality, myocardial infarction, and stroke: a nationwide population-based study. Eur Heart J. (2017) 38:3560–6. doi: 10.1093/eurheartj/ehx585
12. Villines TC, Rodriguez Lozano P. Transitioning from stenosis to plaque burden in the cardiac CT era: the changing risk paradigm. J Am Coll Cardiol. (2020) 76:2814–6. doi: 10.1016/j.jacc.2020.10.030
13. Duivenvoorden R, de Groot E, Stroes ES, Kastelein JJ. Surrogate markers in clinical trials–challenges and opportunities. Atherosclerosis. (2009) 206:8–16. doi: 10.1016/j.atherosclerosis.2008.12.009
14. Chung GE, Lee Y, Yim JY, Choe EK, Kwak MS, Yang JI, et al. Genetic polymorphisms of PNPLA3 and SAMM50 are associated with nonalcoholic fatty liver disease in a korean population. Gut Liver. (2018) 12:316–23. doi: 10.5009/gnl17306
15. Friedewald WT, Levy RI, Fredrickson DS. Estimation of the concentration of low-density lipoprotein cholesterol in plasma, without use of the preparative ultracentrifuge. Clin Chem. (1972) 18:499–502. doi: 10.1093/clinchem/18.6.499
16. Dolan E, O'Brien E. Blood pressure variability: clarity for clinical practice. Hypertension. (2010) 56:179–81. doi: 10.1161/HYPERTENSIONAHA.110.154708
17. Smit RAJ, Jukema JW, Postmus I, Ford I, Slagboom PE, Heijmans BT, et al. Visit-to-visit lipid variability: clinical significance, effects of lipid-lowering treatment, and (pharmaco) genetics. J Clin Lipidol. (2018) 12:266–76 e3. doi: 10.1016/j.jacl.2018.01.001
18. Agatston AS, Janowitz WR, Hildner FJ, Zusmer NR, Viamonte M Jr, Detrano R. Quantification of coronary artery calcium using ultrafast computed tomography. J Am Coll Cardiol. (1990) 15:827–32. doi: 10.1016/0735-1097(90)90282-T
19. Gosmanova EO, Mikkelsen MK, Molnar MZ, Lu JL, Yessayan LT, Kalantar-Zadeh K, et al. Association of systolic blood pressure variability with mortality, coronary heart disease, stroke, and renal disease. J Am Coll Cardiol. (2016) 68:1375–86. doi: 10.1016/j.jacc.2016.06.054
20. Stevens SL, Wood S, Koshiaris C, Law K, Glasziou P, Stevens RJ, et al. Blood pressure variability and cardiovascular disease: systematic review and meta-analysis. BMJ. (2016) 354:i4098. doi: 10.1136/bmj.i4098
21. Echouffo-Tcheugui JB, Zhao S, Brock G, Matsouaka RA, Kline D, Joseph JJ. Visit-to-visit glycemic variability and risks of cardiovascular events and all-cause mortality: the ALLHAT study. Diabetes Care. (2019) 42:486–93. doi: 10.2337/dc18-1430
22. Kim DH, Lipsitz LA, Ferrucci L, Varadhan R, Guralnik JM, Carlson MC, et al. Association between reduced heart rate variability and cognitive impairment in older disabled women in the community: Women's Health and Aging Study I. J Am Geriatr Soc. (2006) 54:1751–7. doi: 10.1111/j.1532-5415.2006.00940.x
23. Park JB, Kim DH, Lee H, Hwang IC, Yoon YE, Park HE, et al. Mildly abnormal lipid levels, but not high lipid variability, are associated with increased risk of myocardial infarction and stroke in “Statin-Naive” young population a nationwide cohort study. Circ Res. (2020) 126:824–35. doi: 10.1161/CIRCRESAHA.119.315705
24. Clark D 3rd, Nicholls SJ, St John J, Elshazly MB, Kapadia SR, Tuzcu EM, et al. Visit-to-visit cholesterol variability correlates with coronary atheroma progression and clinical outcomes. Eur Heart J. (2018) 39:2551–8. doi: 10.1093/eurheartj/ehy209
25. Rohatgi A, Khera A, Berry JD, Givens EG, Ayers CR, Wedin KE, et al. HDL cholesterol efflux capacity and incident cardiovascular events. N Engl J Med. (2014) 371:2383–93. doi: 10.1056/NEJMoa1409065
26. Park S, Kang S. Alcohol, carbohydrate, and calcium intakes and smoking interactions with APOA5 rs662799 and rs2266788 were associated with elevated plasma triglyceride concentrations in a cross-sectional study of korean adults. J Acad Nutr Diet. (2020) 120:1318–29 e1. doi: 10.1016/j.jand.2020.01.009
27. Jasim AA, Al-Bustan SA, Al-Kandari W, Al-Serri A, AlAskar H. Sequence analysis of APOA5 among the kuwaiti population identifies association of rs2072560, rs2266788, and rs662799 with TG and VLDL levels. Front Genet. (2018) 9:112. doi: 10.3389/fgene.2018.00112
28. Lin E, Kuo PH, Liu YL, Yang AC, Tsai SJ. Detection of susceptibility loci on APOA5 and COLEC12 associated with metabolic syndrome using a genome-wide association study in a Taiwanese population. Oncotarget. (2017) 8:93349–59. doi: 10.18632/oncotarget.20967
29. Kim OY, Moon J, Jo G, Kwak SY, Kim JY, Shin MJ. Apolipoprotein A5 3'-UTR variants and cardiometabolic traits in Koreans: results from the Korean genome and epidemiology study and the Korea National Health and Nutrition Examination Survey. Nutr Res Pract. (2018) 12:61–8. doi: 10.4162/nrp.2018.12.1.61
30. Kim YR, Hong SH. Association of apolipoprotein A5 gene polymorphisms with metabolic syndrome in the Korean population. Genet Test Mol Biomarkers. (2016) 20:130–6. doi: 10.1089/gtmb.2015.0250
31. Wang J, Ban MR, Kennedy BA, Anand S, Yusuf S, Huff MW, et al. APOA5 genetic variants are markers for classic hyperlipoproteinemia phenotypes and hypertriglyceridemia. Nat Clin Pract Cardiovasc Med. (2008) 5:730–7. doi: 10.1038/ncpcardio1326
32. Lai CQ, Arnett DK, Corella D, Straka RJ, Tsai MY, Peacock JM, et al. Fenofibrate effect on triglyceride and postprandial response of apolipoprotein A5 variants: the GOLDN study. Arterioscler Thromb Vasc Biol. (2007) 27:1417–25. doi: 10.1161/ATVBAHA.107.140103
33. Xu C, Bai R, Zhang D, Li Z, Zhu H, Lai M, et al. Effects of APOA5−1131T>C (rs662799) on fasting plasma lipids and risk of metabolic syndrome: evidence from a case-control study in China and a meta-analysis. PLoS One. (2013) 8:e56216. doi: 10.1371/journal.pone.0056216
34. Maasz A, Kisfali P, Horvatovich K, Mohas M, Marko L, Csongei V, et al. Apolipoprotein A5 T-1131C variant confers risk for metabolic syndrome. Pathol Oncol Res. (2007) 13:243–7. doi: 10.1007/BF02893505
35. De Caterina R, Talmud PJ, Merlini PA, Foco L, Pastorino R, Altshuler D, et al. Strong association of the APOA5-1131T>C gene variant and early-onset acute myocardial infarction. Atherosclerosis. (2011) 214:397–403. doi: 10.1016/j.atherosclerosis.2010.11.011
36. Peterfi Z, Toth ZE, Kovacs HA, Lazar E, Sum A, Donko A, et al. Peroxidasin-like protein: a novel peroxidase homologue in the human heart. Cardiovasc Res. (2014) 101:393–9. doi: 10.1093/cvr/cvt256
37. Papageorgiou AP, Heymans S. Peroxidasin-like protein: expanding the horizons of matrix biology. Cardiovasc Res. (2014) 101:342–3. doi: 10.1093/cvr/cvu017
38. Yang Y, Cao Z, Tian L, Garvey WT, Cheng G. VPO1 mediates ApoE oxidation and impairs the clearance of plasma lipids. PLoS One. (2013) 8:e57571. doi: 10.1371/journal.pone.0057571
39. Qasim A, Rader DJ. Human genetics of variation in high-density lipoprotein cholesterol. Curr Atheroscler Rep. (2006) 8:198–205. doi: 10.1007/s11883-006-0074-0
40. Berry JD, Liu K, Folsom AR, Lewis CE, Carr JJ, Polak JF, et al. Prevalence and progression of subclinical atherosclerosis in younger adults with low short-term but high lifetime estimated risk for cardiovascular disease: the coronary artery risk development in young adults study and multi-ethnic study of atherosclerosis. Circulation. (2009) 119:382–9. doi: 10.1161/CIRCULATIONAHA.108.800235
41. Toth PP. Subclinical atherosclerosis: what it is, what it means and what we can do about it. Int J Clin Pract. (2008) 62:1246–54. doi: 10.1111/j.1742-1241.2008.01804.x
42. Otton JM, Yu CY, McCrohon J, Sammel N, Feneley M. Accuracy and clinical outcomes of computed tomography coronary angiography in the presence of a high coronary calcium score. Heart Lung Circ. (2013) 22:980–6. doi: 10.1016/j.hlc.2013.05.647
43. Hou ZH, Lu B, Gao Y, Jiang SL, Wang Y, Li W, et al. Prognostic value of coronary CT angiography and calcium score for major adverse cardiac events in outpatients. JACC Cardiovasc Imaging. (2012) 5:990–9. doi: 10.1016/j.jcmg.2012.06.006
44. Kramer CK, Zinman B, Gross JL, Canani LH, Rodrigues TC, Azevedo MJ, et al. Coronary artery calcium score prediction of all cause mortality and cardiovascular events in people with type 2 diabetes: systematic review and meta-analysis. BMJ. (2013) 346:f1654. doi: 10.1136/bmj.f1654
45. Greenland P, Alpert JS, Beller GA, Benjamin EJ, Budoff MJ, Fayad ZA, et al. 2010 ACCF/AHA guideline for assessment of cardiovascular risk in asymptomatic adults: a report of the American College of Cardiology Foundation/American Heart Association task force on practice guidelines. J Am Coll Cardiol. (2010) 56:e50–103. doi: 10.1016/j.jacc.2010.09.001
46. Dzaye O, Razavi AC, Dardari ZA, Shaw LJ, Berman DS, Budoff MJ, et al. Modeling the recommended age for initiating coronary artery calcium testing among at-risk young adults. J Am Coll Cardiol. (2021) 78:1573–83. doi: 10.1016/j.jacc.2021.08.019
47. Yoon YE, Chang SA, Choi SI, Chun EJ, Cho YS, Youn TJ, et al. The absence of coronary artery calcification does not rule out the presence of significant coronary artery disease in Asian patients with acute chest pain. Int J Cardiovasc Imaging. (2012) 28:389–98. doi: 10.1007/s10554-011-9819-0
48. Park HE, Chun EJ, Choi SI, Lee SP, Yoon CH, Kim HK, et al. Clinical and imaging parameters to predict cardiovascular outcome in asymptomatic subjects. Int J Cardiovasc Imaging. (2013) 29:1595–602. doi: 10.1007/s10554-013-0235-5
49. Shimbo D, Newman JD, Aragaki AK, LaMonte MJ, Bavry AA, Allison M, et al. Association between annual visit-to-visit blood pressure variability and stroke in postmenopausal women: data from the Women's Health Initiative. Hypertension. (2012) 60:625–30. doi: 10.1161/HYPERTENSIONAHA.112.193094
50. Levitan EB, Kaciroti N, Oparil S, Julius S, Muntner P. Relationships between metrics of visit-to-visit variability of blood pressure. J Hum Hypertens. (2013) 27:589–93. doi: 10.1038/jhh.2013.19
51. Hecht H, Blaha MJ, Berman DS, Nasir K, Budoff M, Leipsic J, et al. Clinical indications for coronary artery calcium scoring in asymptomatic patients: expert consensus statement from the Society of Cardiovascular Computed Tomography. J Cardiovasc Comput Tomogr. (2017) 11:157–68. doi: 10.1016/j.jcct.2017.02.010
52. Nasir K, Rubin J, Blaha MJ, Shaw LJ, Blankstein R, Rivera JJ, et al. Interplay of coronary artery calcification and traditional risk factors for the prediction of all-cause mortality in asymptomatic individuals. Circ Cardiovasc Imaging. (2012) 5:467–73. doi: 10.1161/CIRCIMAGING.111.964528
53. Yeboah J, Young R, McClelland RL, Delaney JC, Polonsky TS, Dawood FZ, et al. Utility of nontraditional risk markers in atherosclerotic cardiovascular disease risk assessment. J Am Coll Cardiol. (2016) 67:139–47. doi: 10.1016/j.jacc.2015.10.058
54. Kelkar AA, Schultz WM, Khosa F, Schulman-Marcus J, O'Hartaigh BW, Gransar H, et al. Long-term prognosis after coronary artery calcium scoring among low-intermediate risk women and men. Circ Cardiovasc Imaging. (2016) 9:e003742. doi: 10.1161/CIRCIMAGING.115.003742
55. Cho I, Chang HJ, B OH, Shin S, Sung JM, Lin FY, et al. Incremental prognostic utility of coronary CT angiography for asymptomatic patients based upon extent and severity of coronary artery calcium: results from the COronary CT Angiography EvaluatioN For Clinical Outcomes InteRnational Multicenter (CONFIRM) study. Eur Heart J. (2015) 36:501–8. doi: 10.1093/eurheartj/ehu358
Keywords: cholesterol variability, coronary artery calcium, coronary artery stenosis, genome wide association study, Apo A5
Citation: Park J-B, Shin E, Lee J-E, Lee SJ, Lee H, Choi S-Y, Choe EK, Choi SH and Park HE (2022) Genetic Determinants of Visit-to-Visit Lipid Variability: Genome-Wide Association Study in Statin-Naïve Korean Population. Front. Cardiovasc. Med. 9:811657. doi: 10.3389/fcvm.2022.811657
Received: 09 November 2021; Accepted: 03 January 2022;
Published: 31 January 2022.
Edited by:
Marat V. Ezhov, Ministry of Health of the Russian Federation, RussiaReviewed by:
Chien-Yi Hsu, Taipei Medical University Hospital, TaiwanScott M. Gordon, University of Kentucky, United States
Copyright © 2022 Park, Shin, Lee, Lee, Lee, Choi, Choe, Choi and Park. This is an open-access article distributed under the terms of the Creative Commons Attribution License (CC BY). The use, distribution or reproduction in other forums is permitted, provided the original author(s) and the copyright owner(s) are credited and that the original publication in this journal is cited, in accordance with accepted academic practice. No use, distribution or reproduction is permitted which does not comply with these terms.
*Correspondence: Hyo Eun Park, aHlvZXVubWQxJiN4MDAwNDA7Z21haWwuY29t; aHlvZXVubWQmI3gwMDA0MDtnbWFpbC5jb20=
†These authors have contributed equally to this work and share first authorship