- 1Life Science Informatics Group, Department of Mathematics, Natural Sciences and Informatics, Technische Hochschule Mittelhessen (THM) University of Applied Sciences, Giessen, Germany
- 2Department of Anaesthesiology, Kongsberg Hospital, Vestre Viken Hospital Trust, Kongsberg, Norway
- 3Psychological Institute, University of Oslo, Oslo, Norway
Heart rate turbulence (HRT) is a characteristic heart rate pattern triggered by a ventricular premature contraction (VPC). It can be used to assess autonomic function and health risk for various conditions, e.g., coronary artery disease or cardiomyopathy. While comparability is essential for scientific analysis, especially for research focusing on clinical application, the methodology of HRT still varies widely in the literature. Particularly, the ECG measurement and parameter calculation of HRT differs, including the calculation of turbulence slope (TS). In this article, we focus on common variations in the number of intervals after the VPC that are used to calculate TS (#TSRR) posing two questions: 1) Does a change in #TSRR introduce noticeable changes in HRT parameter values and classification? and 2) Do larger values of turbulence timing (TT) enabled by a larger #TSRR still represent distinct HRT? We compiled a free-access data set of 1,080 annotated long-term ECGs provided by Physionet. HRT parameter values and risk classes were determined both with #TSRR 15 and 20. A standard local tachogram was created by averaging the tachograms of only the files with the best heart rate variability values. The shape of this standard VPC sequence was compared to all VPC sequences grouped by their TT value using dynamic time warping (DTW) in order to identify HRT shapes. When calculated with different #TSRR, our results show only a little difference between the number of files with enough valid VPC sequences to calculate HRT (<1%) and files with different risk classes (5 and 6% for HRT0-2 and HRTA-C, respectively). In the DTW analysis, the difference between averaged sequences with a specific TT and the standard sequence increased with increasing TT. Our analysis suggests that HRT occurs in the early intervals after the VPC and TS calculated from late intervals reflects common heart rate variability rather than a distinct response to the VPC. Even though the differences in classification are marginal, this can lead to problems in clinical application and scientific research. Therefore, we recommend uniformly using #TSRR 15 in HRT analysis.
1. Introduction
1.1. Heart Rate Turbulence
With a simple point-of-care investigation of the heart rate, it is possible to estimate the condition and prognosis of patients. A possible method is HRT, which is a naturally occurring phenomenon that arises after a VPC (2): The characteristic pattern comprises an initial drop of interval length (IL) followed by slowly increasing and afterward decreasing length (refer to the Supplementary Figure 1 for a visual representation). This heart rate fluctuation is provoked by the ineffectiveness of the premature beat, which leads to a drop of blood pressure and activates the baroreflex (3, 4).
Because of this dependency on the autonomic nervous system (ANS), HRT can be used as a marker for autonomic health (5). Studies have shown that HRT parameters can be useful risk indicators for all-cause mortality after myocardial infarction or chronic heart failure (5, 6). In combination with other risk indicators, HRT can be used in clinical diagnostics to make therapeutic decisions (7–9). Several methods for the inclusion of HRT in implantable cardioverter defibrillators have already been suggested (10–12). Similarly, GE Healthcare implemented HRT assessment in their Holter analysis software tools MARS and CardioDay, which both have already been used for HRT analysis (13–15).
For HRT, there are three main parameter values that can be calculated (check the Supplementary Figure 1 for a graphical depiction): turbulence onset (TO) describes the first drop of the IL after the VPC compared to the intervals before the VPC. It is, therefore, a marker for the parasympathetic response. TS describes the steepest slope of the tachogram after the compensatory interval (compI). The third parameter TT is the index of the first interval that shows TS (4). Both TS and TT are markers for the sympathetic and parasympathetic activity.
Although the #TSRR was described in the standards as being 15 (16), many studies use 20 instead as suggested in the first description of HRT (17). The first article to give 15 as #TSRR is Barthel et al. (18) but without giving a reason for changing the original method. In reviews about HRT, there is a switch of suggesting #TSRR 20 at first (19–21) and then #TSRR 15 in recent years (2, 5, 22–25). However, many publications of late still use #TSRR 20 (26–30).
1.2. Rationale and Scope
Comparability is one of the key factors of scientific research, especially when developing techniques and workflows used in clinical medicine. Methodological variance diminishes comparable data and can lead to seemingly contradictory results, which make it difficult to assess the usefulness of a technique for a particular use case. For HRT, a standard methodology has been published in the “International Society for Holter and Noninvasive Electrocardiology (ISHNE)” Consensus (16). However, many studies still use different methods to assess HRT (31) causing the aforementioned difficulties.
Until now, no study has analyzed the difference in HRT parameter values when calculated from different #TSRRs. A higher #TSRR increases the risk of artifacts and other arrhythmias to lie in the required calculation range which leads to an exclusion of the VPCSs (VPC snippet, i.e., all RR intervals surrounding the VPC 78 used for HRT calculation). Conversely, with a lower #TSRR, these compromising intervals may lie outside of the needed calculation range for some VPC snippet, i.e. all RR intervals surrounding the VPC used for HRT calculations (VPCSs) which would make them shorter but valid sequences for HRT assessment. In consequence, a change in #TSRR can lead to a selection of different sets of VPCSs and, therefore, affect all HRT parameter values of a person.
Since HRT is triggered by a VPC via the baroreflex, it is plausible that the reaction should arise without any delay. This means that the slope that represents the turbulence should arise first in direct proximity to the compI and second always after a similar time period. Accordingly, TS calculated from either only late intervals or intervals with widely differing indices may only describe random fluctuation rather than a reaction of the ANS. Because TT describes the localization of TS, it can be used to test this assumption.
In this article, we analyze two hypotheses:
• Hypothesis 1: There is a distinct difference in HRT parameters when calculating HRT with #TSRR 15 or 20.We test this on a large free-access data set from Physionet and compare the resulting HRT parameters and classes.
• Hypothesis 2: Persons with a high TT value or a high TT variability do not show HRT, but seemingly random fluctuations, i.e., heart rate variability (HRV).
We, therefore, create an averaged ideal standard VPCS (stVPCS) with distinct HRT by filtering the Physionet data set via HRV parameters. This standard VPCS is then compared with sequences that have been averaged from VPCSs sorted for their respective TT value.
2. Materials and Methods
2.1. Materials
2.1.1. Data
We used databases available on physionet.org (32). The databases had to include annotations of long-term electrocardiograms (ECGs) specifying the beat types. All databases that fit those criteria at the time of analysis (15.01.2021) are summed up in Table 1.
Since our analysis should be independent of the medical background of measurements, we did not exclude databases based on their scope. In sum, our analysis included 1,080 annotation files. If possible, we preferred annotations that were manually corrected, although most of the databases only included automatically generated annotations.
2.1.2. The RHRT Package
For the calculation of the HRT parameter values of each annotation file, we used our R package RHRT (v. 1.1) (38). RHRT provides functions to find VPCSs in time intervals and calculate HRT parameter values with customisable filtering criteria, order of calculation and normalization. The package can be found on The Comprehensive R Archive Network (CRAN) (https://CRAN.R-project.org/package=RHRT) and on github (https://github.com/VBlesius/RHRT). The default methodology of filtering, calculation, and classification is done as suggested in Blesius et al. (31), which mostly follows the ISHNE consensus (16). In contrast to the standards, we use 5 instead of 2 RR intervals in a VPCS before the couplI (preRRs), because the preceding intervals are used to calculate the reference interval (refI) and must, therefore, be included in the filtering process. Furthermore, we use TS normalized after (1) (nTS) which is TS normalized for heart rate and #TSRR. A detailed description can be found in the Supplementary Data Sheet 1 and the documentation of the package.
2.1.3. Other R Packages
Statistical differences between data sets were calculated with the stats package (v 4.1.1). RHRV version 4.2.6 was used to calculate HRV parameter values. For the Poincaré filter and the data preparation of the HRV calculation, we used the packages, geometry (v. 0.4.5), smoother (v. 1.1), and purrr (v. 0.3.4). To compare the stVPCS with the averaged VPCSs dtw (v. 1.22.3) was used. This package provides functions for DTW, which is an algorithm to compare similarities of two sequences: All points of the first sequence are matched to the points of the second one whereas points can be matched to multiple other points. The only limitation is that the first and last points have to be matched to each other, respectively, and mapped indices have to be increasing, meaning that there may not be overlapping matches. Dynamic time warping (DTW) can calculate a matching score, which was used in this analysis.
2.2. Methods
2.2.1. Comparing Data With #TSRR 15 and 20
We assessed HRT of all files twice with the default parameters of RHRT and the settings numPostRRs = 15 (TSRR15) and numPostRRs = 20 (TSRR20). Additionally, we created a data set from the valid VPCs included in the analysis with #TSRR 20, but calculated HRT results with numPostRRs = 15 (TSRR15∩). This leads to a data set with identical VPCs but shorter VPCSs that allows comparison without considering filtering effects. We then calculated the arithmetic mean and SD of HRT parameter values and classified the data into HRT0-2 and HRTA-C. Depending on the number of files with enough valid VPCSs, either a Welch's unequal variances t-test or a paired Student's t-test (both with t.test of the stats package) were used to detect differences between the sets of parameter values.
2.2.2. Creating a stVPCS
The purpose of the analysis was to compare VPCSs sorted on the basis of TT with an stVPCS. Since HRT is most pronounced in persons without autonomic dysfunction as reviewed in Bauer et al. (16), we needed to select files of supposedly healthy persons. However, the databases used do not include data from persons labeled as healthy. It may be assumed that NSRDB and NSR2DB are comprised of such data, but manual inspection of Poincaré plots revealed abnormal patterns throughout both databases. Therefore, we used HRV to filter the files of all databases that have the most sound values of these autonomic markers. The filtering process included three steps:
2.2.2.1. Length of Files
At first, all files that were shorter than 20 h or longer than 28 h were discarded. Since HRT is correlated with the circadian rhythm of the ANS, it should be calculated from measurements with a length as close as possible to 24 h or its multiple if procurable.
Applying the following filters—especially the Poincaré filter—to full measurements can lead to the discarding of basically valid data due to temporary irregularities in heart rhythm. Furthermore, variability in the length of the measurements can lead to a bias in HRV parameter values (39). Therefore, we cut the measurements into snippets of 30 min and applied the following filters to these chunks. Only data files with at least 75% valid chunks were passed on by the Poincaré filter to the next step. In the HRV filter, the mean of all chunks was calculated for every parameter, respectively, before ranking the measurements.
2.2.2.2. Poincaré Filter
As a next step, we used a filter that quantifies the data distribution within a Poincaré plot: this non-linear method of HRV analysis plots data points of a time series against their respective successors to visualize the beat-to-beat variability of RR intervals. Any pathology that affects the length of RR-intervals causes distinct patterns in the Poincaré plots. These patterns have been systematically analyzed and categorized by Esperer et al. (40) and were called lorenz plot patterns. Plots from persons with sinus rhythm show so-called “comets” or “torpedos,” which are shaped as long cones or ellipses, respectively (Refer to Figure 1A). Other lorenz plot patterns are:
• “island” patterns consisting of four or nine roundly shaped clusters that are connected to atrial tachycardia or atrial flutter, both with the atrioventricular block (Figure 1D).
• “fan” patterns which look like broader spread torpedos or triangles and occur in persons with atrial fibrillation or multifocal atrial tachycardia (Figure 1C).
• “lobe” patterns consisting of one central and several eccentric clusters which occur due to frequent VPCs or atrial premature contractions (Figure 1B).
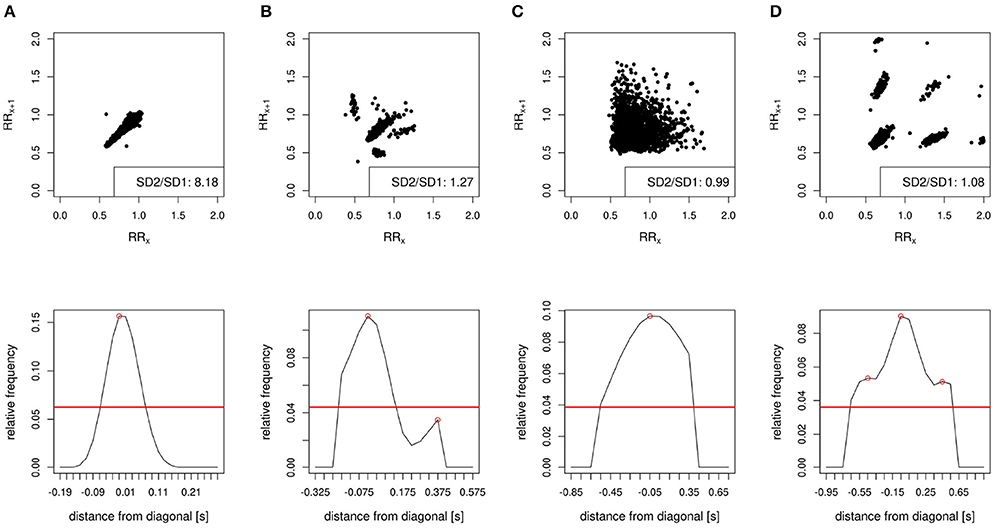
Figure 1. Lorenz plot patterns and their corresponding smoothed histograms of SD1. The plots show 30-min chunks of different measurements that were used in the analysis as an example of different Lorenz plot patterns. The Poincaré plots in the top show a (A) comet, (B) side lobe pattern, (C) fan pattern, and (D) island pattern. The corresponding plots below show the histograms after smoothing. The lobe, fan, and island patterns have an SD2/SD1 ratio of less than 1.5 and would, therefore, be excluded. The histogram of the island patterns includes more than one local maximum, which is another criterion for exclusion. Data: (A) nsr008 from NSR2DB, (B) nsr018 from NSR2DB, (C) 01 from LTAFDB, and (D) f018 from CRISDB.
For our analysis, we focused on filtering out Poincaré plots with island and fan patterns since they are specific for different kinds of atrial arrhythmia and atrioventricular block. This leaves torpedos, comets, and lobe patterns that show sinus rhythm or possible VPCs. Since high-frequent VPCs are an indicator for high risk (41–43), we focused on plots that show mostly comets and torpedos. The chunk-wise analysis of the plots left enough VPCs in the resulting data. In contrast to most other shapes, torpedos and comets consist of just one evenly shaped cluster. We use this fact for two conditions of our filter: First, we projected all data points onto the axis perpendicular to the line of identity and analyzed their distribution. After taking the logarithm and smoothing, histograms with more than one extremum exceeding 40% of the maximum were excluded to rule out strong side lobe and island patterns (refer to Figure 1). Second, we calculated the SD of the projected points which is the HRV parameter SD1. Analogously, we calculated SD2 from the diagonal. The ratio of SD1 with SD2 had to exceed 1.5 since a lower ratio proved to be indicative of broader spread patterns like islands or fans. This cut-off was deduced from Esperer et al. (40), who showed that especially fans have a ratio of the length and the width of the central cluster of less than 1. We used SD1 and SD2 here since they are more commonly known and the exact methodology to create a cluster has not been described in the article.
2.2.2.3. HRV Filter
The last filtering step is based on the HRV time and frequency domain of the data. We calculated the following HRV parameters with the RHRV package: SD of the averages of all normal sinus rhythm intervals (SDNN), SD of the averages of all normal sinus rhythm intervals in any 5 min segments (SDANN), triangular index, i.e., the total number of all normal sinus rhythm intervals divided by the maximum of the interval frequency distribution, square root of the mean of the squared successive differences between adjacent RR intervals (RMSSD), very low frequency power, low frequency power, high frequency power, and the ratio of low and high frequency power. For every parameter, all files were ranked for their HRV values, respectively: Files with a value that exceeded three times the interquartile range, i.e. the difference between the upper and lower quartiles (IQR), were considered to be outliers. If their values were greater or less than the median±3·IQR they were given the penalty score “–1.” For all HRV parameters, high values were assumed to be better, only for triangular index lower values were scored higher. Accordingly, the files were sorted by their HRV value and the best 20% received the score “1,” while the remaining received “0.” After this scoring process for every HRV parameter, the scores were summed up for every file, leading to possible scores from –8 (all parameter values are outliers) to 8 (all parameter values are in the top for their respective parameter). On the basis of the scores, the highest ranking 20% of the files were used to create the stVPCS.
Heart rate turbulence of all top ranking files was calculated with the RHRT package. All HRT calculations were done with the default settings of the RHRT package except for “numPostRRs” (#TSRR) for which we used 20 intervals because the longer range is the maximum of commonly used #TSRR and provided more intervals for later comparisons. For each file, the averaged VPCS was used as the basis to calculate an overall averaged VPCS (stVPCS).
2.2.3. Comparing VPCSs Based on TT
2.2.3.1. HRT Values
We calculated the HRT parameter values of every file in our databases with the default settings of the RHRT package except “numPostRRs” for which we used 30 intervals to ensure a wide range of possible TT values.
2.2.3.2. DTW With stVPCS
We extracted the RR intervals in a VPCS following the compI (postRRs) of every averaged VPCS, grouped them based on their respective TT, and calculated an averaged postRRs sequence for every TT. For the next step of matching the postRRs of the stVPCS to every averaged postRRs sequence via DTW, we tested two methods: First, we matched the standard sequence dynamically to the averaged sequences with the default step pattern “symmetric2” of the dtw function. Second, we removed the leading intervals of the standard sequence before the TT to receive a sequence that only consists of the intervals that shape the TS and all following intervals. The averaged sequences were cut accordingly and shortened to fit the standard sequence. The standard sequence was matched to all averaged sequences index by index with the dtw step pattern rigid.
2.2.3.3. Intra-Subject Variability of TT
As a measure of the variability of TT within a file, we calculated the SD of TT (TTSD) and the Pearson correlation coefficient for TT and TTSD.
3. Results
3.1. Comparing Data With #TSRR 15 and 20
The number of files that included enough VPCs to calculate HRT was similar with #TSRR 15 (870 files) and #TSRR 20 (862). Similarly, the number of files sorted in different HRT classes were similar with #TSRR 15 and 20 for HRT0-2 (HRT0 402 vs. 404, HRT1 139 vs. 130, and NR 321 vs. 328) as well as HRTA-C (HRTA 396 vs. 385, HRTB 144 vs. 127, and NR 322 vs. 350). Of the 862 files from which HRT parameters could be calculated in both analyzes, 43 and 55 files were differently classified into HRT classes HRT0-2 and HRTA-C, respectively (refer to Tables 2, 3).
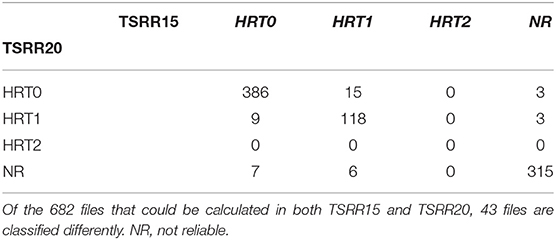
Table 2. HRT0-2 classes of files before and after changing #TSRR from 15 (columns) to 20 (rows) during HRT assessment.
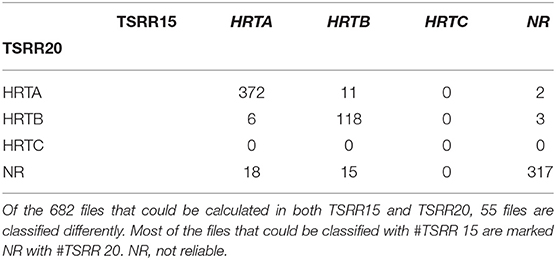
Table 3. HRTA-C classes of files before and after changing #TSRR from 15 (columns) to 20 (rows) during HRT assessment.
When comparing the HRT parameters of TSRR15, TSRR20, and TSRR15∩ (data in TSRR20 recalculated with #TSRR 15), the most influenced parameter is TT with 5.47 ± 2.38 (TSRR15∩) and 5.75 ± 3 (TSRR20) (refer to Table 4). The TO values of TSRR15∩ and TSRR20 were identical, while the mean difference of the TS and TT values were 0.06 (CI 0.03 to 0.09, p = 4.9·10−4) and 0.4 (CI 0.28–0.53, p = 2.9·10−10), respectively. The most differing values of the unpaired t-tests were the TT values of TSRR15 and TSRR20 with CI –0.5 to 0.03 and p = 0.08. The p-values of all other unpaired t-tests ranged from 0.71 to 0.99 with differences of the arithmetic means between 0.001 and 0.043. A noticeable difference is the high number of TT values that were NR with #TSRR 20 (79) compared to both #TSRR 15 analyzes (9 and 6, respectively).
3.2. Creating a stVPCS
Of the 1,080 annotation files included in the analysis, 70 files were shorter than 20 h and 1 file longer than 28 h. Thus, they were excluded.
Of the 1,009 remaining files, 652 were removed through the Poincaré filter, leaving 357 files.
After HRV parameter calculation and averaging 33 of the files contained at least one outlier. The median score of the files was 1 with a minimum of –7 and a maximum of 7.
From the best 20% (71 files), HRT was calculated. In 24 files, no or too few valid VPCSs could be found. While most of the remaining 47 files showed a distinct HRT pattern (refer to Figure 2), some did not (refer to Figure 3). Table 5 shows a detailed overview of the number of filtered files broken down by databases.
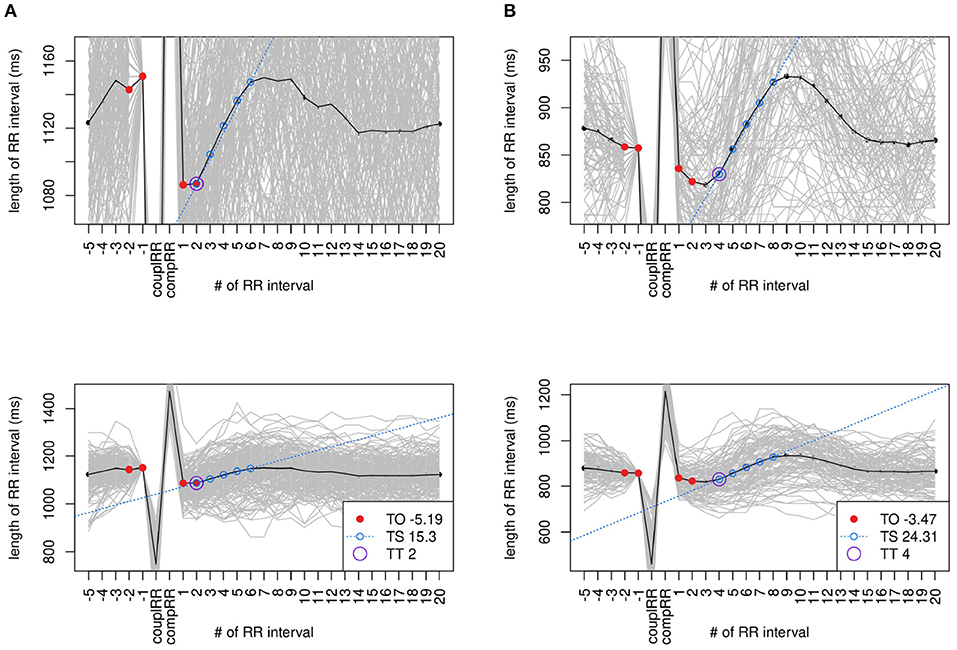
Figure 2. Standard VPCS (stVPCS) files with distinct heart rate turbulence (HRT). Exemplary tachograms of two files used to calculate the stVPCS that show distinct HRT. Both files are in class HRTA. The upper row shows a zoomed in tachogram, the row below the respective tachogram zoomed out. Data: (A) e145a from CRISDB and (B) nsr010 from NSR2DB.
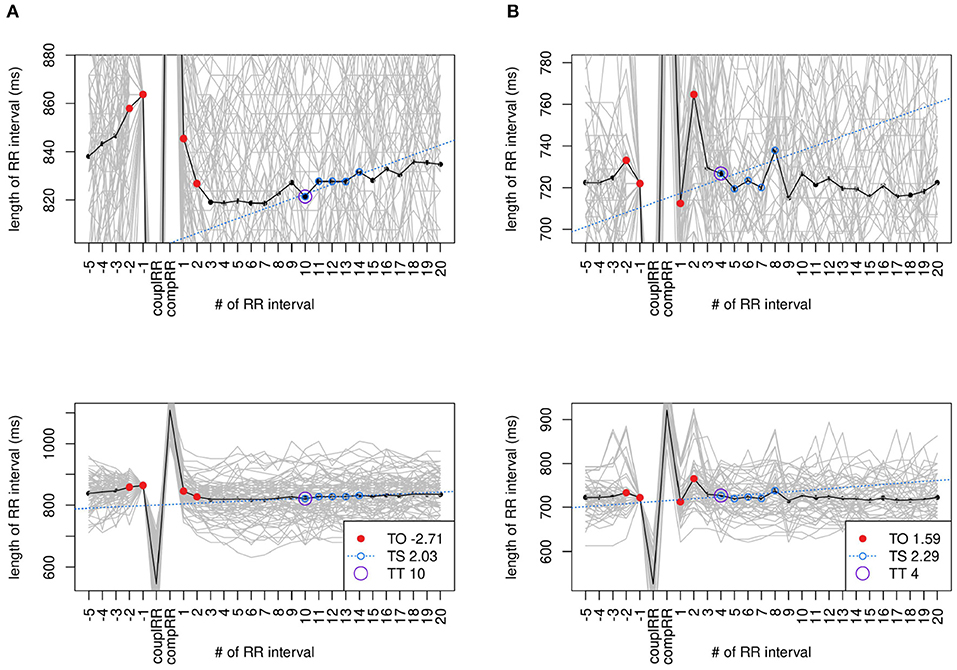
Figure 3. stVPCS files without distinct HRT. Exemplary tachograms of two files used to calculate the stVPCS that do not show distinct HRT. Both files are in class HRTB. The upper row shows a zoomed in tachogram, the row below the respective tachogram zoomed out. Data: (A) s20491 from LTSTDB and (B) chf202 from CHF2DB.
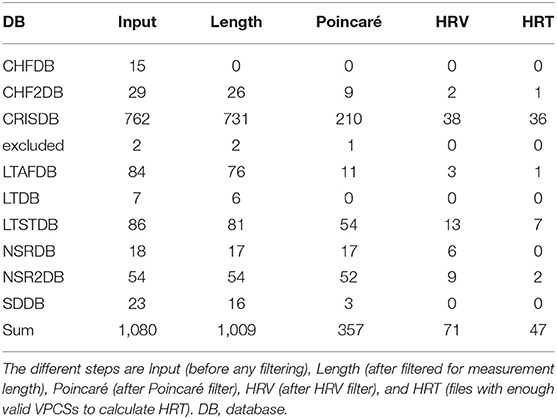
Table 5. Overview of the number of files remaining after every analysis step sorted by their databases.
After classification, 41 of the files used for the stVPCS had HRT class HRT0, 5 files had HRT1, and 1 file HRT2. Of these files, 7 (2 HRT0, 4 HRT1, 1 HRT2) are marked as not reliable by the RHRT package. When adding TT to the classification, 40 files had HRT class HRTA and 7 files HRTB, whereas the classification from 10 files (4 of HRTA, 6 of HRTB) are marked as unreliable.
Because stVPCS should be used for comparison as the ideal HRT shape, it is important that it shows a pronounced reaction to the VPC and low risk HRT parameters. The sequence averaged from all 47 VPCSs showed a distinct HRT pattern (refer to Figure 4 with TO = 3.12%, TS = 7.85 ms/RR, and TT = 3. Therefore, it falls in the lowest risk categories HRT0 and HRTA. The parameter nTS could not be calculated for stVPCS because RMSSD needs to be calculated from a respective long-term measurement, which is not applicable for the averaged VPCS.
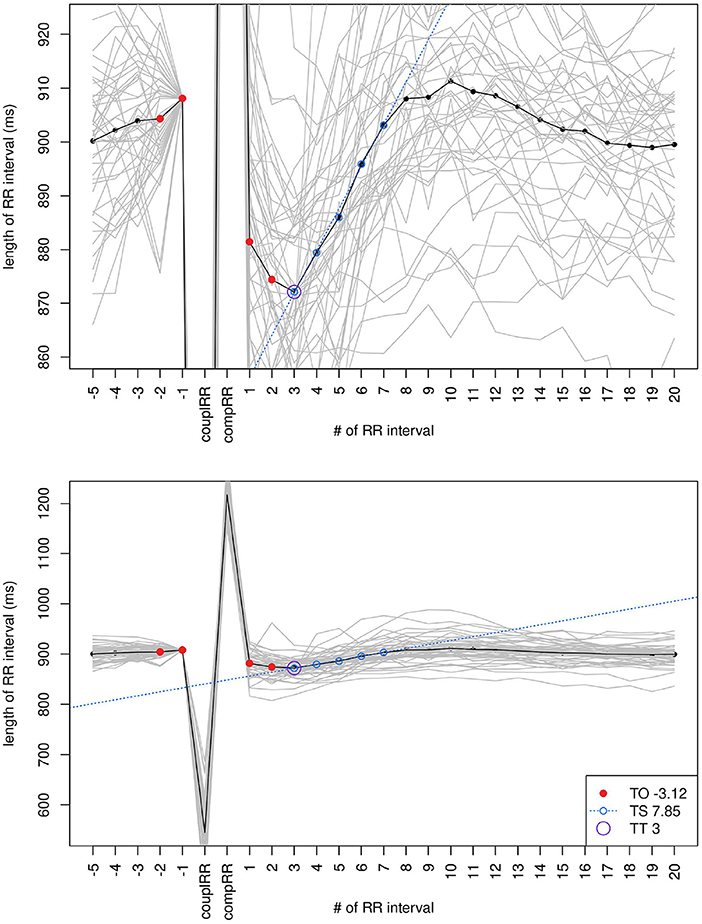
Figure 4. The stVPCS calculated from 47 files that matched all filter criteria and had the best HRV parameters.
3.3. Comparing VPCSs Based on TT
3.3.1. HRT Values
The HRT parameter values of all files sorted by TT can be seen in Figure 5 and in the Supplementary Table 1 in more detail. Half of the files have a TT between 4 and 8. The median TS is above the threshold of 2.5 ms/RR for TT values 6 and lower and under the threshold for most higher TT values. For high TT values, the median of TS varies, whereas the number of files in these groups is considerably lower. Unequal distribution is noticeable since the groups with more than 20 files (TT of 2 to 10) include 82% of all files. Analogously to TS, the median of TO is below the threshold for low TT values (1 to 11) and varies with increasing TT.
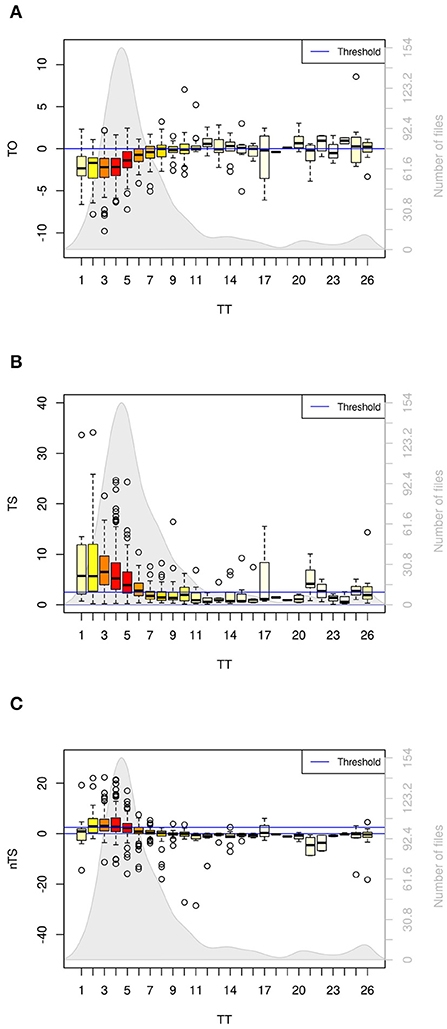
Figure 5. Averaged HRT parameter values of all files grouped by the respective turbulence timing (TT) value. The color of the boxes and the gray background graph illustrate the number of files in the different groups. The common threshold of TO (0%) (A) and TS (2.5 ms/RR) (B) is represented as a blue line. The common cut-off of TS is used for nTS, because no threshold for nTS has been established yet (C). The median of the groups with low TT (TT ≤ 10 for TO and TT ≤ 6 for TS) lie on the low-risk side of the threshold. For nTS, only the medians of the groups with a TT of 2–4 lie above the threshold.
The parameter values of nTS worsen clearly and the pattern changes compared to the TS values: Only the medians of nTS from a TT of 2–4 still lie above the threshold. The nTS medians of all other TT values including 1 lie below the threshold. For many TT values, no file has an nTS value that exceeds the threshold.
3.3.2. DTW With stVPCS
The results of the DTW analysis are shown in Figures 6, 7. The plots for all TT values can be found in the Supplementary Figures 2 and 3. The averaged VPCS that matched the stVPCS the best was TT 3. Apart from TT 1, with rising TT, the difference between VPCS and stVPCS increased. The averaged VPCS with TT 1 lacked the characteristic delayed IL decrease but showed an immediate IL rise followed only by a shallow IL decline.
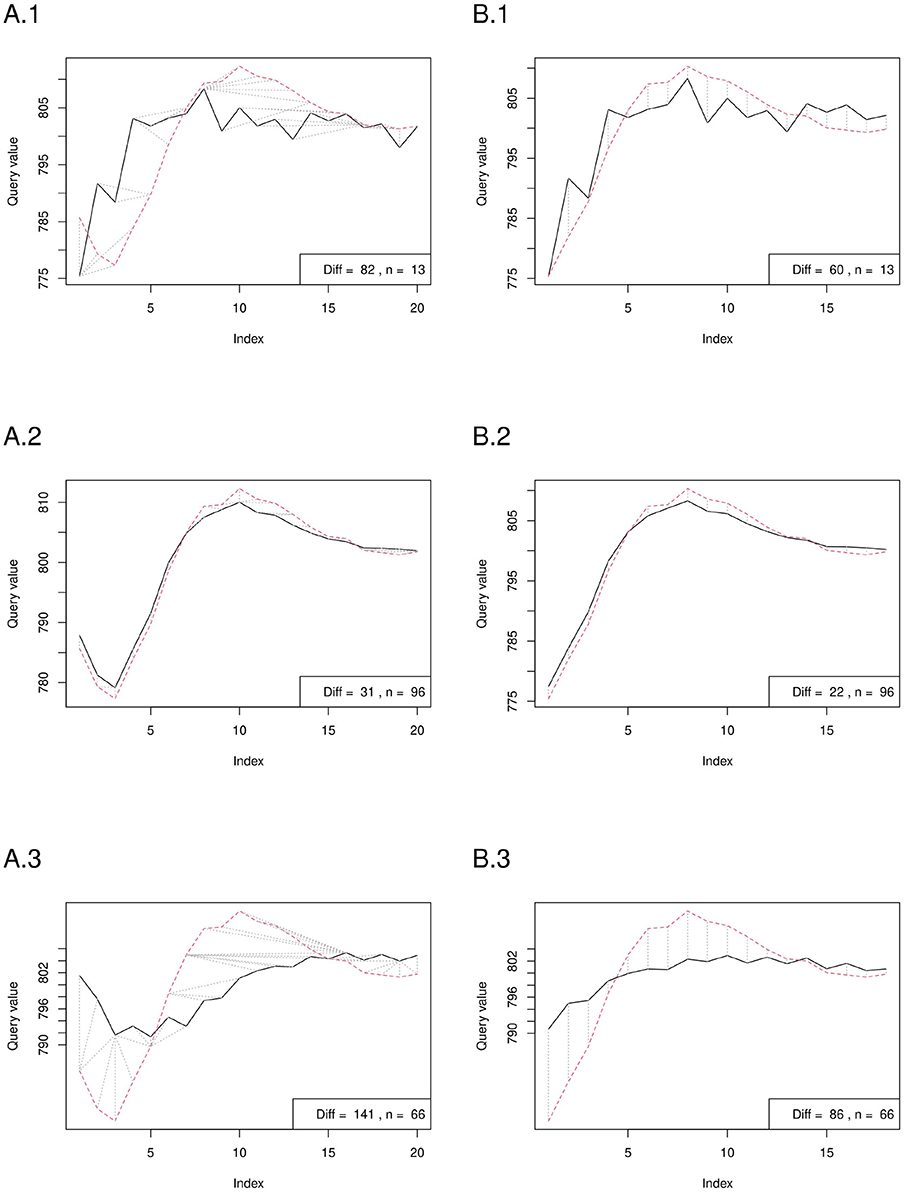
Figure 6. Dynamic time warping (DTW) analysis of exemplary postRRs grouped by their respective TT. The left side (A) shows the analysis of the stVPCS with the full postRRs sequences, the right side (B) with the cut sequences. From up to down the plots show the comparisons for TT 1 (1), 3 (2), and 7 (3). The full averaged sequence of TT 1 (A.1) lacks the initial bend and shows an immediate IL incline. The sequence of TT = 3 fits the stVPCS the best, both in the full (A.2) and in the cut version (B.2).
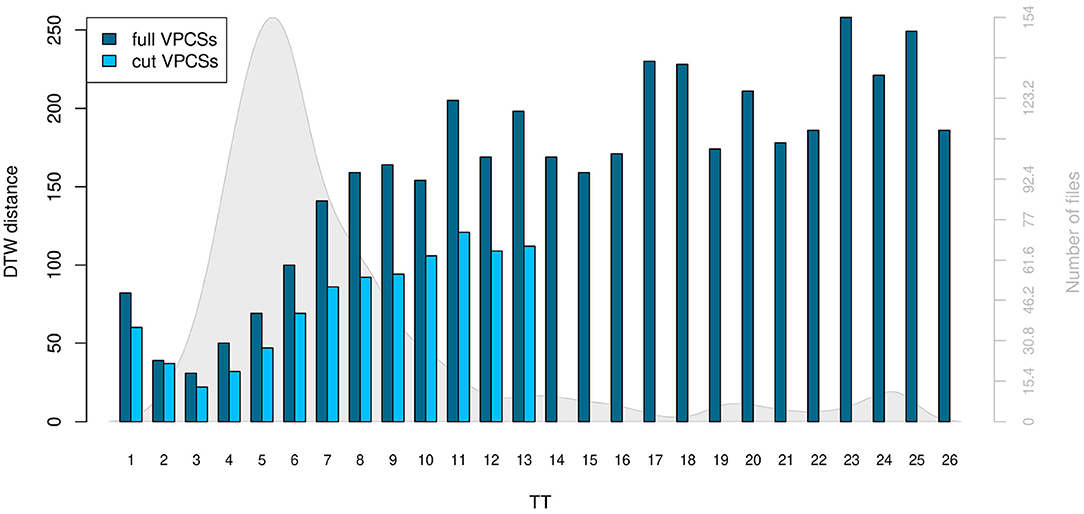
Figure 7. Distances calculated by DTW. The differences between stVPCS and the averaged VPCSs of all files grouped by their respective TT are shown in dark blue (full sequences) and light blue (cut sequences). The distances of the cut sequences are lower than the distances of the full sequences. In both TT 3 has the lowest distance to the stVPCS. The gray background graph and the corresponding axis on the right illustrate the number of files in the different groups.
Analogously to the comparison with the full sequences, the averaged VPCS with TT 3 matched the best with the stVPCS after cutting. The difference of the VPCS of TT 1 to the best sequence is similar to the analysis without cutting (full VPCSs: DiffTT1 82, DiffTT3 31; with cutting: DiffTT1 60, DiffTT3 22). The sequences with TT 2 to 4 considerably line with the stVPCS, while the sequences flatten out continuously with rising TT.
3.3.3. Intra-Subject Variability of TT
The TT and TTSD within a file were significantly correlated (ρ = 0.26, p < 0.005, refer to Figure 8).
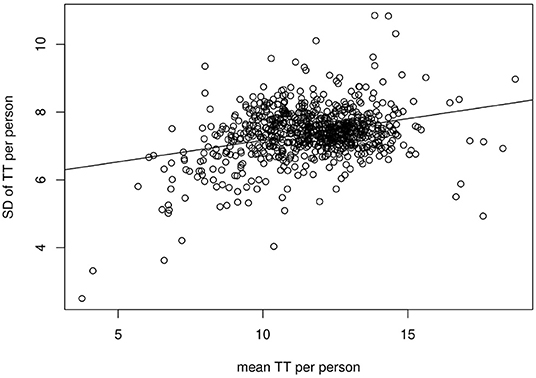
Figure 8. Linear regression analysis of TT and its respective TTSD of all files included in the analysis.
4. Discussion
4.1. Differences in Classification
In our analysis of 1,080 files, only 8 could additionally be classified when using a lower #TSRR. Furthermore, there were 43 and 55 files that changed classification due to #TSRR in the classification systems HRT0-2 and HRTA-C, respectively. The switches were both within HRT classes as well as between an HRT class and NR. Interestingly, a high number of these files switched from an HRT class when calculated with #TSRR 15 to NR with #TSRR 20, meaning that a higher #TSRR leads to more variability in the data.
The same can be seen for TT values, where a higher amount of values was NR with #TSRR 20. This can be explained by the majority of the files with not reliable TT values showing a very shallow tachogram in visual analysis. With no distinct IL increase, random fluctuations have a stronger influence on the location of the steepest slope, thus increasing the variability of TT. Furthermore, longer VPCSs lead to a higher number of possible TT values and therefore higher variability. This high variability combined with a lower number of VPCSs results in non-significant results in the reliability check and, thus, a higher number of files with NR TT.
The only HRT parameter with distinctly differing values is TT which is to be expected with higher #TSRR. This leads to the differences in classification being marginal with less than 1% more classifiable files and 5–6% files changing the resulting classes. However, in clinical settings, even small numbers of patients that cannot be classified or are differently classified based on methodological variances are unfavorable-especially if this could be avoided by uniformly adjusting one parameter.
4.2. stVPCS
The stVPCS received through our pipeline shows a distinct HRT pattern with the HRT classes of HRT0 and HRTA, which imply the least possible risk. The tachogram of our stVPCS is similar to tachograms showing characteristic HRT patterns in reviews (16, 19, 22). Although the databases used to consist of files from subjects with severe diseases, with the filtering pipeline, we were able to find a set of files without pathological abnormalities based on their sound HRT parameter values. The resulting stVPCS seemed to be a feasible approximation of a healthy HRT reaction that could be used as a template for the following analysis.
4.3. Random Fluctuation With High TTs
Apart from TT 1, the tachograms with low TTs showed a similar pattern to the stVPCS (check the Supplementary Data Sheet 2 for a discussion of VPCS with TT 1). With increasing TT the tachograms get more shallow meaning the reaction to the VPC becomes less distinct with increasing distance to the VPC. Especially with high TT values, the tachograms show no distinct pattern but apparently random fluctuation. This can also be seen in the mean HRT values grouped by TT. As expected, the VPCSs with a low TT show the best TS values. The same can be seen for TO. With high TT values, however, the medians for both TS and TO vary, which implies common HRV rather than HRT. Still, the number of VPCSs used to calculate the medians decreases with increasing TT, which may bias this observation.
Nevertheless, TTSD is lower with lower TT values meaning that in persons with low TT the fastest slope occurs in a narrower range. Again, a narrower range implies a steady underlying mechanism that causes turbulence within a distinct time interval while a high fluctuation of TT values within a person suggests randomness. Therefore, TTSD may possibly be used as a measurement for the reliability of TT as well as TS and nTS.
Our data suggest, that only measurements with TT 2 to approximately 6 show distinct HRT. This can be seen in the DTW plots in combination with the median values of the HRT parameters grouped by TT. We recommend visually inspecting all measurements with TT 1 or higher than 7 to ensure the validity of the HRT parameters. Admittedly, manual visual inspection introduces unpreventable human error and, thus, variability to the analyzes, which should be avoided wherever possible. Therefore, DTW may be a method to ensure a reliable reaction to the VPC by comparing the progress of the tachogram of a person to a standard tachogram established from a healthy peer group. Additionally, stVPCS could be generated for different pathological conditions, which would enable using HRT not only for risk assessment but also as part of diagnostics. Possibly, DTW could replace the original HRT parameters, because it analyzes the tachogram as a whole instead of reducing it to selective parameters that can be biased as seen in this study with TS. Using DTW for HRT analysis needs establishing the mentioned stVPCSs through a sufficiently large data set with fitting health conditions and with respect to factors influencing HRT like age or circadian rhythm (31). A similar approach has already been done by Martínez et al. (44) based on a Neyman-Pearson detector (44) that compares a VPCS to the first three functions of a Karhunen-Loève transform expansion (45, 46). Under certain circumstances, this assessment is more robust regarding noise than TO and TS and needs fewer VPCSs to reach a high probability of detecting distinct HRT (46), which shows that comparison of shape patterns as a whole instead of reducing them to restricted aspects of the curve progression offers promising risk assessment parameters.
4.4. Hypotheses
Our first hypothesis suggested a distinct difference in HRT values when calculated with different #TSRRs. Although we could show a difference in the number of assessable HRT values and HRT classes, the differences are not as distinct as we expected with < 1% and 5–6% affected files, respectively. However, no variable risk assessment is obstructive in clinical diagnostics, especially if the results obtained from the same person vary solely based on a difference in methodology. Consequentially, the question remains which of the commonly used #TSRR are optimal for the analysis.
Therefore, our second hypothesis tackled the question of whether high TT or TTSD values do not show actual HRT but random fluctuation. The tachograms of the files with different parameter values based on #TSRR show, that these differences are mainly based on variability due to different sets of VPCSs used for calculation instead of actual HRT at the end of the VPCSs. Furthermore, the comparison of the stVPCS with averaged VPCSs grouped by TT verifies that with increasing TT the response to the VPCs decreases considerably. The same result is implied by HRT values passing their respective thresholds to an increasing degree with rising TT.
4.5. Limitations
While some of the files included in the stVPCS derive from NSR2DB that is defined as subjects with “normal sinus rhythm,” the vast majority of files belong to the CRISDB, LTSTDB, LTAFDB, and CHF2DB that include ECGs of persons after myocardial infarction, with ST-segment anomalies, atrial fibrillation, and congestive heart failure, respectively. Therefore, it is probable that files were included from persons with diagnosed pathologies that are not visible in the used autonomic markers and may bias our results. It would be interesting to repeat the analysis with data from healthy subjects to examine a possible difference in the stVPCSs.
Due to the lack of meta-data in the user databases, we did not analyze any influence of medication on #TSRR. To our knowledge, a temporal change in the HRT response has not been studied so far. The focus of HRT research rather lies on the strength of the response than its delay. The same goes for any response of the baroreflex: Baroreflex sensitivity has been shown to change with antihypertensive medication (47, 48), but its temporal aspect has not been studied. Since the baroreflex response latency can be influenced through short directed intervention such as tilt or atropine administration (49), it is possible that drugs influencing the sympathovagal balance like beta-blockers can change the response delay as well. However, the temporal scale of the difference does not exceed 1 s which amounts to approximately two intervals (49) and is likely to be less with long-term medication and adapted baroreceptor sensitivity. Therefore, we expect that any medication influencing HRT does not influence our results, but this also should be investigated with appropriate data.
It is important to mention that our results allow conclusions about the behavior of the autonomic marker but not its predictive power. Since HRT is a risk marker for major adverse cardiac events, analysis without meta-data about the outcome of the studied patients can only be the first step and must be verified with appropriate clinical data.
5. Conclusion
We recommend using #TSRR 15 for HRT analysis. The lower number of valid intervals results in a higher amount of VPCSs that can be used in the analysis as well as discarding of intervals that show random fluctuation instead of HRT. Therefore, it leads to more reliable data.
Data Availability Statement
Publicly available datasets were analyzed in this study. This data can be found at: https://physionet.org/content/chfdb/1.0.0/; https://physionet.org/content/chf2db/1.0.0/; https://physionet.org/content/crisdb/1.0.0/; https://physionet.org/content/excluded/1.0.0/; https://physionet.org/content/ltafdb/1.0.0/; https://physionet.org/content/ltdb/1.0.0/; https://physionet.org/content/ltstdb/1.0.0/; https://physionet.org/content/nsrdb/1.0.0/; https://physionet.org/content/nsr2db/1.0.0/; https://physionet.org/content/sddb/1.0.0.
Ethics Statement
Ethical review and approval was not required for the study on human participants in accordance with the local legislation and institutional requirements. Written informed consent for participation was not required for this study in accordance with the national legislation and the institutional requirements.
Author Contributions
VB conceived the project, implemented the analysis pipeline and performed all analyzes and literature research, created all figures and tables, and wrote the initial draft of the manuscript and revised it. CS provided technical advice in the implementation of the analysis pipeline. CS, GE, and AD reviewed the manuscript. AD supervised the project. All authors contributed to the article and approved the submitted version.
Conflict of Interest
The authors declare that the research was conducted in the absence of any commercial or financial relationships that could be construed as a potential conflict of interest.
Publisher's Note
All claims expressed in this article are solely those of the authors and do not necessarily represent those of their affiliated organizations, or those of the publisher, the editors and the reviewers. Any product that may be evaluated in this article, or claim that may be made by its manufacturer, is not guaranteed or endorsed by the publisher.
Supplementary Material
The Supplementary Material for this article can be found online at: https://www.frontiersin.org/articles/10.3389/fcvm.2022.793535/full#supplementary-material
Abbreviations
ANS, autonomic nervous system; compI, compensatory interval; couplI, coupling interval; CRAN, The Comprehensive R Archive Network; DTW, dynamic time warping; ECG, electrocardiogram; HRT, heart rate turbulence; HRV, heart rate variability; IL, interval length; IQR, interquartile range, i.e., the difference between the upper and lower quartiles; ISHNE, International Society for Holter and Noninvasive Electrocardiology; NR, not reliable; nRMSSD, RMSSD normalized for heart rate; nTS, TS normalized after (1); numTSRR[#TSRR], number of RR intervals in which TS is calculated; postRRs, RR intervals in a VPCS following the compI; preRRs, RR intervals in a VPCS before the couplI; refI, reference interval; RMSSD, square root of the mean of the squared successive differences between adjacent RR intervals; SD, standard deviation; SD1, SD of data points in poincare plot projected to the axis perpendicular to the line of identity; SD2, SD of data points in poincare plot projected to the line of identity; SDANN, standard deviation of the averages of all normal sinus rhythm intervals in any 5 min segments; SDNN, standard deviation of the averages of all normal sinus rhythm intervals; stVPCS, standard VPCS; TO, turbulence onset; TS, turbulence slope; TT, turbulence timing; TTSD, SD of TT; VPCS, VPC snippet, i.e., all RR intervals surrounding the VPC used for HRT calculation; VPC, ventricular premature contraction.
References
1. Hallstrom AP, Stein PK, Schneider R, Hodges M, Schmidt G, Ulm K. Structural relationships between measures based on heart beat intervals: potential for improved risk assessment. IEEE Trans Biomed Eng. (2004) 51:1414–20. doi: 10.1109/TBME.2004.828049
2. Lombardi F, Stein PK. Origin of heart rate variability and turbulence: an appraisal of autonomic modulation of cardiovascular function. Front Physiol. (2011) 2:95. doi: 10.3389/fphys.2011.00095
3. Wichterle D, Melenovsky V, Malik M. Mechanisms involved in heart rate turbulence. Card Electrophysiol Rev. (2002) 6:262–6. doi: 10.1023/A:1016385126668
4. Watanabe MA, Marine JE, Sheldon R, Josephson ME. Effects of ventricular premature stimulus coupling interval on blood pressure and heart rate turbulence. Circulation. (2002) 106:325–30. doi: 10.1161/01.CIR.0000022163.24831.B5
5. Cygankiewicz I. Heart rate turbulence. Prog Cardiovasc Dis. (2013) 56:160–71. doi: 10.1016/j.pcad.2013.08.002
6. Disertori M, Masè M, Rigoni M, Nollo G, Ravelli F. Heart rate turbulence is a powerful predictor of cardiac death and ventricular arrhythmias in postmyocardial infarction and heart failure patients: a systematic review and meta-analysis. Circ Arrhythm Electrophysiol. (2016) 9:e004610. doi: 10.1161/CIRCEP.116.004610
7. Golukhova EZ, Gromova O, Grigoryan M, Merzlyakov V, Shumkov K, Bockeria L, et al. Noninvasive predictors of malignant arrhythmias. Cardiology. (2016) 135:36–42. doi: 10.1159/000445881
8. Lin K, Wei L, Huang Z, Zeng Q. Combination of Ewing test, heart rate variability, and heart rate turbulence analysis for early diagnosis of diabetic cardiac autonomic neuropathy. Medicine. (2017) 96:e8296. doi: 10.1097/MD.0000000000008296
9. Bugan B, Cekirdekci EI. Prognostic significance of heart rate turbulence parameters in patients with noncompaction cardiomyopathy. Cardiology. (2019) 142:56–62. doi: 10.1159/000499408
10. Gimeno-Blanes FJ, Blanco-Velasco M, Barquero-Pérez Ó, García-Alberola A, Rojo-Álvarez JL. Sudden cardiac risk stratification with electrocardiographic indices - a review on computational processing, technology transfer, and scientific evidence. Front Physiol. (2016) 7:82. doi: 10.3389/fphys.2016.00082
11. Frolov AV, Vaikhanskaya TG, Melnikova OP, Vorobiev AP, Guel LM. Risk stratification personalised model for prediction of life-threatening ventricular tachyarrhythmias in patients with chronic heart failure. Kardiol Pol. (2017) 75:682–8. doi: 10.5603/KP.a2017.0060
12. Soguero-Ruiz C, Mora-Jiménez I, Ramos-López J, Quintanilla Fernández T, García A, Díez-Mazuela D, et al. An interoperable system toward cardiac risk stratification from ECG monitoring. Int J Environ Res Public Health. (2018) 15:428. doi: 10.3390/ijerph15030428
13. Fazio G, Sarullo FM, D'Angelo L, Lunetta M, Visconti C, Di Gesaro G, et al. Heart rate turbulence for guiding electric therapy in patients with cardiac failure. J Clin Monit Comput. (2010) 24:125–9. doi: 10.1007/s10877-009-9218-4
14. Kaplan RM, Herzog CA, Larive B, Subacius H, Nearing BD, Verrier R, et al. T-Wave alternans, heart rate turbulence, and ventricular ectopy in standard versus daily hemodialysis: results from the FHN daily trial. Ann Noninvasive Electrocardiol. (2016) 21:566–71. doi: 10.1111/anec.12354
15. Uznańska-Loch B, Wikło K, Trzos E, Wierzbowska-Drabik K, Chrzanowski Ł, Kasprzak JD, et al. Advanced and traditional electrocardiographic risk factors in pulmonary arterial hypertension: the significance of ventricular late potentials. Kardiol Pol. (2018) 76:586–93. doi: 10.5603/KP.a2017.0257
16. Bauer A, Malik M, Schmidt G, Barthel P, Bonnemeier H, Cygankiewicz I, et al. Heart rate turbulence: standards of measurement, physiological interpretation, and clinical use: international society for Holter and Noninvasive electrophysiology consensus. J Am Coll Cardiol. (2008) 52:1353–65. doi: 10.1016/j.jacc.2008.07.041
17. Schmidt G, Malik M, Barthel P, Schneider R, Ulm K, Rolnitzky L, et al. Heart-rate turbulence after ventricular premature beats as a predictor of mortality after acute myocardial infarction. Lancet. (1999) 353:1390–6. doi: 10.1016/S0140-6736(98)08428-1
18. Barthel P, Schneider R, Bauer A, Ulm K, Schmitt C, Schömig A, et al. Risk stratification after acute myocardial infarction by heart rate turbulence. Circulation. (2003) 108:1221–6. doi: 10.1161/01.CIR.0000088783.34082.89
19. Guzik P, Schmidt G. A phenomenon of heart-rate turbulence, its evaluation, and prognostic value. Card Electrophysiol Rev. (2002) 6:256–61. doi: 10.1023/A:1016333109829
20. Bauer A, Schmidt G. Heart rate turbulence. J Electrocardiol. (2003) 36(Suppl.):89–93. doi: 10.1016/j.jelectrocard.2003.09.020
22. Watanabe MA, Schmidt G. Heart rate turbulence: a 5-year review. Heart Rhythm. (2004) 1:732–8. doi: 10.1016/j.hrthm.2004.09.003
23. Francis J, Watanabe MA, Schmidt G. Heart rate turbulence: a new predictor for risk of sudden cardiac death. Ann Noninvasive Electrocardiol. (2005) 10:102–9. doi: 10.1111/j.1542-474X.2005.10102.x
24. Bauer A, Zürn CS, Schmidt G. Heart rate turbulence to guide treatment for prevention of sudden death. J Cardiovasc Pharmacol. (2010) 55:531–8. doi: 10.1097/FJC.0b013e3181d4c973
25. Zuern CS, Barthel P, Bauer A. Heart rate turbulence as risk-predictor after myocardial infarction. Front Physiol. (2011) 2:99. doi: 10.3389/fphys.2011.00099
26. Yamada S, Yoshihisa A, Hijioka N, Kamioka M, Kaneshiro T, Yokokawa T, et al. Autonomic dysfunction in cardiac amyloidosis assessed by heart rate variability and heart rate turbulence. Ann Noninvasive Electrocardiol. (2020) 25:e12749. doi: 10.1111/anec.12749
27. Oyelade T, Canciani G, Bottaro M, Zaccaria M, Formentin C, Moore K, et al. Heart rate turbulence predicts survival independently from severity of liver dysfunction in patients with cirrhosis. Front Physiol. (2020) 11:602456. doi: 10.3389/fphys.2020.602456
28. Yamada S, Yoshihisa A, Sato Y, Sato T, Kamioka M, Kaneshiro T, et al. Utility of heart rate turbulence and T-wave alternans to assess risk for readmission and cardiac death in hospitalized heart failure patients. J Cardiovasc Electrophysiol. (2018) 29:1257–64. doi: 10.1111/jce.13639
29. Yildiz C, Yildiz A, Tekiner F. Heart rate turbulence analysis in subclinical hypothroidism heart rate turbulence in hypothyroidism. Acta Cardiologica Sinica. (2015) 31:444–8. doi: 10.6515/acs20150428a
30. Soylu MO, Altun I, Basaran O, Uzun Y, Dogan V, Ergun G, et al. Impact of QRS morphology on heart rate turbulence and heart rate variability after cardiac resynchronization therapy in patients with heart failure. Eur Rev Med Pharmacol Sci. (2016) 20:317–22.
31. Blesius V, Schölzel C, Ernst G, Dominik A. HRT assessment reviewed: a systematic review of heart rate turbulence methodology. Physiol Meas. (2020) 41:08TR01. doi: 10.1088/1361-6579/ab98b3
32. Goldberger AL, Amaral LA, Glass L, Hausdorff JM, Ivanov PC, Mark RG, et al. PhysioBank, physiotoolkit, and physionet: components of a new research resource for complex physiologic signals. Circulation. (2000) 101:E215–20. doi: 10.1161/01.CIR.101.23.e215
33. Baim DS, Colucci WS, Monrad ES, Smith HS, Wright RF, Lanoue A, et al. Survival of patients with severe congestive heart failure treated with oral milrinone. J Am Coll Cardiol. (1986) 7:661–70. doi: 10.1016/S0735-1097(86)80478-8
34. Stein PK, Domitrovich PP, Kleiger RE, Schechtman KB, Rottman JN. Clinical and demographic determinants of heart rate variability in patients post myocardial infarction: insights from the cardiac arrhythmia suppression trial (CAST). Clin Cardiol. (2000) 23:187–94. doi: 10.1002/clc.4960230311
35. Petrutiu S, Sahakian AV, Swiryn S. Abrupt changes in fibrillatory wave characteristics at the termination of paroxysmal atrial fibrillation in humans. EP Europace. (2007) 9:466–70. doi: 10.1093/europace/eum096
36. Jager F, Taddei A, Moody GB, Emdin M, Antoliċ G, Dorn R, et al. Long-term ST database: a reference for the development and evaluation of automated ischaemia detectors and for the study of the dynamics of myocardial ischaemia. Med Biol Eng Comput. (2003) 41:172–82. doi: 10.1007/BF02344885
37. Greenwald SD. The Development and Analysis of a Ventricular Fibrillation Detector (Master's thesis) (1986). Available online at: https://dspace.mit.edu/handle/1721.1/92988
38. Blesius V, Dominik A. RHRT: an R package to assess heart rate turbulence. J Open Source Softw. (2021) 6:3540. doi: 10.21105/joss.03540
39. Malik M, Bigger JT, Camm AJ, Kleiger RE, Malliani A, Moss AJ, et al. Heart rate variability-Standards of measurement, physiological interpretation, and clinical use. Eur Heart J. (1996) 17:354–81. doi: 10.1093/oxfordjournals.eurheartj.a014868
40. Esperer HD, Esperer C, Cohen RJ. Cardiac arrhythmias imprint specific signatures on lorenz plots. Ann Noninvasive Electrocardiol. (2008) 13:44–60. doi: 10.1111/j.1542-474X.2007.00200.x
41. Lown B, Wolf M. Approaches to sudden death from coronary heart disease. Circulation. (1971) 44:130–42. doi: 10.1161/01.CIR.44.1.130
42. Kostis JB, McCrone K, Moreyra AE, Gotzoyannis S, Aglitz NM, Natarajan N, et al. Premature ventricular complexes in the absence of identifiable heart disease. Circulation. (1981) 63:1351–6. doi: 10.1161/01.CIR.63.6.1351
43. Latchamsetty R, Bogun F. Premature ventricular complexes and premature ventricular complex induced cardiomyopathy. Curr Probl Cardiol. (2015) 40:379–422. doi: 10.1016/j.cpcardiol.2015.03.002
44. Martínez JP, Laguna P, Solem K, Sörnmo L. Evaluation of a Neyman-Pearson heart-rate turbulence detector. Annu Int Conf IEEE Eng Med Biol Soc. (2008). 2008:4407–10. doi: 10.1109/IEMBS.2008.4650188
45. Smith D, Solem K, Laguna P, Martínez JP, Sörnmo L. Model-based detection of heart rate turbulence using mean shape information. IEEE Trans Biomed Eng. (2010) 57:334–42. doi: 10.1109/TBME.2009.2030669
46. Martínez JP, Cygankiewicz I, Smith D, Bayés de Luna A, Laguna P, Sörnmo L. Detection performance and risk stratification using a model-based shape index characterizing heart rate turbulence. Ann Biomed Eng. (2010) 38:3173–84. doi: 10.1007/s10439-010-0081-8
47. Berdeaux A, Giudicelli Jf. Antihypertensive drugs and baroreceptor reflex control of heart rate and blood pressure. Fundamental Clin Pharmacol. (1987). 1:257–82. doi: 10.1111/j.1472-8206.1987.tb00565.x
48. Grassi G, Trevano FQ, Seravalle G, Scopelliti F, Mancia G. Baroreflex function in hypertension: consequences for antihypertensive therapy. Prog Cardiovasc Dis. (2006) 48:407–15. doi: 10.1016/j.pcad.2006.03.002
Keywords: heart rate turbulence (HRT), noninvasive risk stratification, heart rate variability (HRV), heart failure, myocardial infarction, methodology, standardization
Citation: Blesius V, Schölzel C, Ernst G and Dominik A (2022) Comparability of Heart Rate Turbulence Methodology: 15 Intervals Suffice to Calculate Turbulence Slope – A Methodological Analysis Using PhysioNet Data of 1074 Patients. Front. Cardiovasc. Med. 9:793535. doi: 10.3389/fcvm.2022.793535
Received: 12 October 2021; Accepted: 07 February 2022;
Published: 06 April 2022.
Edited by:
Maurizio Acampa, Siena University Hospital, ItalyReviewed by:
Akiomi Yoshihisa, Fukushima Medical University, JapanAxel Bauer, Medical University of Innsbruck, Austria
Copyright © 2022 Blesius, Schölzel, Ernst and Dominik. This is an open-access article distributed under the terms of the Creative Commons Attribution License (CC BY). The use, distribution or reproduction in other forums is permitted, provided the original author(s) and the copyright owner(s) are credited and that the original publication in this journal is cited, in accordance with accepted academic practice. No use, distribution or reproduction is permitted which does not comply with these terms.
*Correspondence: Valeria Blesius, aHJ0QGJsZXNpdXMuZXU=