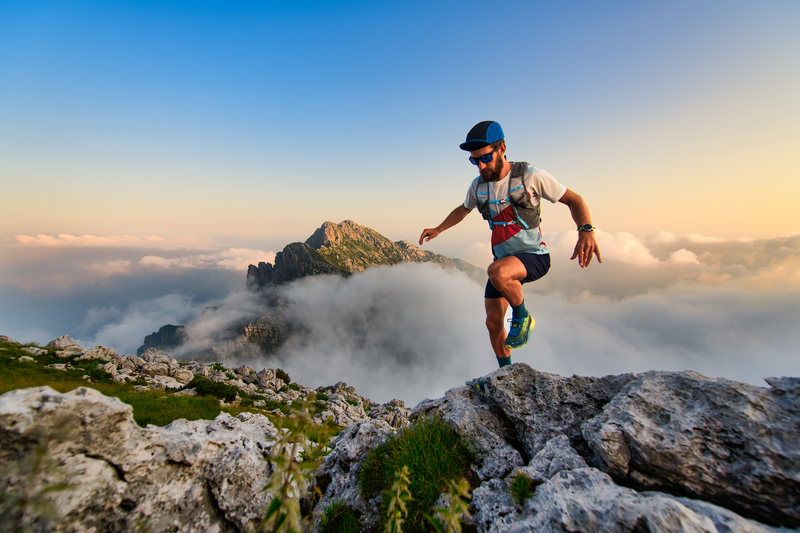
94% of researchers rate our articles as excellent or good
Learn more about the work of our research integrity team to safeguard the quality of each article we publish.
Find out more
ORIGINAL RESEARCH article
Front. Cardiovasc. Med. , 03 March 2022
Sec. Coronary Artery Disease
Volume 9 - 2022 | https://doi.org/10.3389/fcvm.2022.773524
Objective: To investigate the influence of different segmentations on the diagnostic performance of pericoronary adipose tissue (PCAT) CT attenuation and radiomics features for the prediction of ischemic coronary artery stenosis.
Methods: From June 2016 to December 2018, 108 patients with 135 vessels were retrospectively analyzed in the present study. Vessel-based PCAT was segmented along the 40 mm-long proximal segments of three major epicardial coronary arteries, while lesion-based PCAT was defined around coronary lesions. CT attenuation and radiomics features derived from two segmentations were calculated and extracted. The diagnostic performance of PCAT CT attenuation or radiomics models in predicting ischemic coronary stenosis were also compared between vessel-based and lesion-based segmentations.
Results: The mean PCAT CT attenuation was −75.7 ± 9.1 HU and −76.1 ± 8.1 HU (p = 0.395) for lesion-based and vessel-based segmentations, respectively. A strong correlation was found between vessel-based and lesion-based PCAT CT attenuation for all cohort and subgroup analyses (all p < 0.01). A good agreement for all cohort and subgroup analyses was also detected between two segmentations. The diagnostic performance was comparable between vessel-based and lesion based PCAT CT attenuation in predicting ischemic stenosis. The radiomics features of PCAT based on vessel or lesion segmentation can both adequately identify the ischemic stenosis. However, no significant difference was detected between the two segmentations.
Conclusions: The quantitative evaluation of PCAT can be reliably measured both from vessel-based and lesion-based segmentation. Furthermore, the radiomics analysis of PCAT may potentially help predict hemodynamically significant coronary artery stenosis.
Vascular inflammation is a driver of coronary atherosclerotic plaque formation and also a typical feature of atherosclerotic plaque rupture (1). Patients with coronary artery disease (CAD) have histological evidence of local inflammation both within culprit lesions and throughout the entire coronary vascular bed (2). Coronary computed tomography angiography (CCTA) is a widely used non-invasive modality for the diagnosis of CAD. Recent research demonstrated that signals released from the inflamed coronary artery diffuse to the perivascular adipose tissue, inhibiting local adipogenesis. Such an inflammatory response changes the composition of perivascular adipose tissue around inflamed arteries, shifting its attenuation on CCTA from the lipid [more negative Hounsfield unit (HU) values (e.g., closer to −190 HU)] to the aqueous phase [less negative HU values (e.g., closer to −30 HU)]. The changes in pericoronary adipose tissue (PCAT) attenuation can be non-invasively measured using routine CCTA, and enable early detection of vascular inflammation in coronary arteries. The relationship between PCAT and coronary atherosclerosis has been studied by several authors, including its link with plaque composition, vulnerability, and hemodynamic significance (3–6). Moreover, higher PCAT CT attenuation was revealed to be associated with an increased risk of cardiac mortality and poor prognosis (7). The importance of PCAT radiomics features in cardiac risk prediction was also revealed by Oikonomou et al. (8). However, the area of PCAT measurement in different studies was inconsistent. Among previous studies, the majority investigated PCAT in the proximal 40-mm segment of all three major epicardial coronary vessels [right coronary artery (RCA), left anterior descending artery (LAD), and left circumflex artery (LCX)] (5, 7, 9–11) or one of the coronary arteries (RCA or LAD) (2–4, 8, 12–16). Some studies suggested that a lesion-specific assessment of PCAT might provide greater insight into atherosclerotic biology than the proximal segments of the major arteries alone (6, 17). To resolve this inconsistency, this study aimed to clarify whether a proximal 40 mm assessment or a lesion-specific assessment was more appropriate in the evaluation of PCAT. Our secondary objective was to compare the diagnostic performance of PCAT CT attenuation and radiomics features between two segmentations for the prediction of hemodynamically significant coronary artery stenosis.
This retrospective study complied with the Helsinki Declaration (2000). From June 2016 to December 2018, 191 consecutive patients with suspect CAD who underwent CCTA, invasive coronary angiography (ICA), and fractional flow reserve (FFR) examination were retrospectively screened from our institution's database. All patients gave written informed consent, and the study protocol was approved by the institutional review board of Xijing Hospital affiliated with the Fourth Military Medical University (KY20194007). Exclusion criteria were previous revascularization (n =47), normal angiograms in CCTA (n = 1), the interval between CCTA and FFR measurement > 1 month (n = 28) and poor CCTA image quality (n =7). Additionally, vessels with total occlusion (n = 8) and normal angiogram (n = 5) were further excluded (Supplementary Figure S1). Finally, 135 lesions in 108 patients (mean age, 59 years ± 10; range, 30 ~ 77 years, 78 males and 20 females) were analyzed. Details of the patients' characteristics were shown in Table 1.
All CCTA scans were performed on a second generation 128-slice dual source CT (Somatom Definition Flash, Siemens Healthineers, Forchheim, Germany) with retrospectively electrocardiogram-triggered spiral acquisition. The detailed scanning parameters are specified in Supplementary Appendix. Plaque characterization was performed using dedicated plaque analysis software (Coronary Plaque Analysis, version 5.0.0, Siemens Healthineers, Germany). Coronary artery calcium score was calculated on gated non-contrast CT using the Agatston method.
Selective ICA was performed by standard catheterization in accordance with the American College of Cardiology recommendations for coronary angiography (18). FFR was measured using a 0.014-inch pressure sensor tipped guidewire (Pressure Wire, St. Jude Medical Systems, St. Paul, Minnesota) as previously described (19). Hyperemia was induced with intravenous continuous infusion (160 μg/kg/min) of adenosine (20). Intracoronary nitroglycerin was administered immediately before measurement of FFR. FFR ≤ 0.80 was indicative of hemodynamically significant stenosis.
To measure PCAT CT attenuation, 3-dimensional layers within radial distance from the outer coronary wall equal in thickness to the average diameter of the vessels were constructed automatically from the CCTA (Figure 1) using semi-automated software (Perivascular Analysis function, Coronary Plaque Analysis, version 5.0.0, Siemens Healthineers, Germany). Within the predefined volume of interest, voxels with tissue attenuation ranging from −190 HU to −30 HU were defined as PCAT (7). Two cardiovascular radiologists (with 8- and 10-years of experience in cardiac imaging) who were blinded to ICA and FFR results, independently performed the PCAT segmentation. On a per vessel level, PCAT segmentation was performed around the 40 mm-long proximal segments of LAD, LCX, and RCA. To avoid the effects of the aortic wall, we excluded the most proximal 10 mm of RCA and analyzed the proximal 10–50 mm of the vessel (7). In LAD and LCX, we did not analyze the left main coronary artery because of its variable length. Given that previous studies (6, 17) have shown PCAT surrounding lesions to be a potential sensor of ischemic stenosis, lesion-based PCAT segmentation was also performed around coronary lesions on a per lesion level. The lesion causing the highest-grade stenosis on each coronary vessel was chosen for PCAT analysis, and the length of the lesion-based PCAT was defined from the proximal to the distal shoulder of the lesion. PCAT CT attenuation was defined as the mean CT attenuation in the adipose tissue.
Figure 1. Representative CCTA image of PCAT around LAD. A hemodynamically significant stenosis (FFR = 0.73) in the middle segment of LAD. PCAT CT attenuation was defined as the mean CT attenuated value within a radial distance equal to the diameter of the vessel. The length of the lesion-based PCAT was defined from the proximal to the distal shoulder of the lesion (A), the vessel-based PCAT was focused on the proximal 40-mm segments of all three major epicardial coronary vessels (10 to 50 mm from right coronary artery ostium) (B). LAD, left anterior descending artery; PCAT, pericoronary adipose tissue.
Lesion- and vessel-based PCAT segmentations were loaded into a stand-alone software prototype (Radiomics, version 1.2.2, Siemens Healthineers, Germany), which extracted radiomics features via PyRadiomics library. A total of 1,691 features were calculated for each segmentation, including 17 shape, 18 first-order and 75 texture features in the original images. High-dimensional radiomics features were calculated through pre-processing, which multiplied first-order and texture features through Laplacian of Gaussian (LoG) filtering, wavelet filtering, and non-linear intensity transforms (Supplementary Table S1).
Eligible lesions were randomly split into a training (60%) and a testing set (40%), in order to identify optimal radiomics features extracted from different PCAT segmentations and to further validate their association with hemodynamic status of coronary artery stenosis. Univariate logistic regression was conducted in the training dataset to initially screen out radiomics features irrelevant to hemodynamic outcome by creating Manhattan plots. Based on a pre-defined statistical significance of p < 0.05, features above the threshold of 1.301 (–log10 based) remained for more rigorous selection. The least absolute shrinkage and selection operator (LASSO) with logistic regression was used to separately select the ideal combination of features from lesion-based and vessel-based PCAT. Finally, 10-fold cross validation was applied to determine the optimal lambda value, which was fed into the prediction model in order to discern dichotomized hemodynamic significance.
Continuous variables are presented as mean ± standard deviation and categorical variables as frequencies (percentages). The interobserver agreement for PCAT CT attenuation was determined with the intraclass correlation coefficient (ICC). Pearson correlation coefficient was calculated for assessment of the relationship of vessel-based and lesion-based PCAT CT attenuation in the entire cohort as well as sub-group analysis. Bland-Altman plots were created comparing vessel-based and lesion-based PACT CT attenuation. Confidence limits were created assuming a parametric distribution, and 1-sample t-tests were run to detect any significant fixed bias. Diagnostic performance of PCAT radiomics features was assessed with receiver operating characteristic (ROC) curve analysis, and the DeLong test was used to compare the area under the curves (AUCs). Statistical analysis was performed using MedCalc version 11.4.2 (MedCalc Software, Mariakerke, Belgium) and R, version 3.6.3 (R Foundation for Statistical Computing, Vienna, Austria). A 2-tailed value of p < 0.05 was considered statistically significant.
Lesions were most often present in LAD (71.8%), followed by RCA (15.6%) and LCX (12.6%). Among the 135 lesions, 76 (56.3%) lesions were located in the proximal of coronary artery, 33 (24.4%) lesions in the middle segment and 26 (19.3%) lesions in the distal segment. Moreover, 103 (76.3%) lesions with stenosis ≥50% were detected, and 63 (46.7%) lesions were considered ischemic stenosis according to FFR (FFR ≤ 0.80) (Table 2). The mean lesion length was 26.2 ± 12.3 mm.
PCAT CT attenuation on lesion-based and vessel-based segmentation had excellent interobserver reproducibility with ICC of 0.921 and 0.984 in all vessels, 0.918 and 0.982 in vessels with FFR >0.80, and 0.932 and 0.991 in vessels with FFR ≤ 0.80. The mean PCAT CT attenuation was −75.7 ± 9.1 HU for lesion-based measurement, and −76.1 ± 8.1 HU for vessel-based measurement (p = 0.395) (Table 2). A strong significant correlation was observed between vessel-based and lesion-based PCAT CT attenuation (r = 0.7686, p < 0.0001) in the entire cohort (Figure 2A), as well as sub-group analysis [r = 0.7540, p < 0.0001 for vessels with FFR > 0.80 (Figure 2B) and r = 0.8166, p < 0.0001 for vessels with FFR ≤ 0.80 (Figure 2C)]. A Bland-Altman analysis comparing lesion-based and vessel-based PCAT CT attenuation showed the following mean differences: 0.4356 (95% limits of agreement: −11.18 to 12.05) in all vessels (Figure 2D), 1.467 (−11.16 to 14.09) for vessels with FFR >0.80 (Figure 2E) and −0.743 (−10.70 to 9.212) for vessels with FFR ≤ 0.80 (Figure 2F), respectively.
Figure 2. Correlation and Bland-Altman plots of vessel-based and lesion-based PCAT CT attenuation as clarified by invasive FFR. Pearson correlation analyses show the correlation vessel-based and lesion-based PCAT CT attenuation in all vessels (A), vessels with FFR > 0.80 (B) and vessels with FFR ≤0.80 (C), respectively. Bland-Altman plots show PCAT CT attenuation between vessel-based and lesion-based in all vessels (D), vessels with FFR >0.80 (E) and vessels with FFR ≤0.80 (F). The red dotted lines represent the mean difference (bias), and the blue dotted lines represent the 95% limits of agreement. FFR, fractional flow reserve; PCAT, pericoronary adipose tissue.
Pearson correlation coefficients of vessel-based and lesion-based PCAT CT attenuation were as follows: RCA: r = 0.7649 (p = 0.0001) (Figure 3A), LAD: r = 0.7921 (p < 0.0001) (Figure 3B), and LCX: r = 0.7656 (p = 0.0003) (Figure 3C). The difference between vessel-based and lesion-based PCAT CT attenuation in RCA was 4.821 (95% limits of agreement: −9.928 to 19.57) (Figure 3D), −0.2577 (−10.56 to 10.05) in LAD (Figure 3E), and −1.026 (−11.25 to 9.195) in LCX (Figure 3F). Furthermore, a good correlation and agreement of vessel-based and lesion-based PCAT CT attenuation was found regardless of lesion locations (Supplementary Figure S2).
Figure 3. Correlation and Bland-Altman plots of vessel-based and lesion-based PCAT CT attenuation in different coronary arteries. Pearson correlation analyses show the correlation vessel-based and lesion-based PCAT CT attenuation in RCA (A), LAD (B) and LCX (C), respectively. Bland-Altman plots show PCAT CT attenuation between vessel-based and lesion-based in RCA (D), LAD (E) and LCX (F). The red dotted lines represent the mean difference (bias), and the blue dotted lines represent the 95% limits of agreement. LAD, left anterior descending artery; LCX, left circumflex artery; PCAT, pericoronary adipose tissue; RCA, right coronary artery.
There was no significant difference in PCAT CT attenuation between vessels with FFR >0.80 and FFR ≤ 0.80 regardless of the segmentation method (vessel-based p = 0.453; lesion-based p = 0.463). According to ROC curve analysis, the AUC of PCAT CT attenuation in vessel-based segmentation was 0.524 (95% CI: 0.436–0.611), which had similar diagnostic performance compared to lesion-based PCAT CT attenuation (0.547, 95% CI: 0.459–0.633, p = 0.814) (Figure 4).
Figure 4. ROC curve analysis of PCAT CT attenuation measured from lesion- and vessel-based segmentations for identifying functionally significant coronary stenosis. Two segmentations had similar AUC (0.547 vs. 0.524, p = 0.814). AUC, area under the curve; ROC, receiver operating characteristic curve.
The dataset was randomly split into a training set (n = 82) and a testing set (n = 53). There was no difference in stenosis diameter, lesion distribution, and PCAT CT attenuation between the training and the testing set (all p > 0.05) (Table 3). Univariable logistic regression selected 24 and 15 radiomics features from lesion-based and vessel-based PCAT segmentations, respectively. Ten-fold cross validation was performed to further refine the remaining features. Seven features from lesion-based PCAT segmentation (Figure 5A), and six from vessel-based PACT segmentation (Figure 5B) were ultimately used to construct the radiomics models using LASSO.
Figure 5. Manhattan plots demonstrated the strength of association [–log10 (P-value)] of univariate logistic regression] comparing PCAT radiomics features derived from lesion-based (A) and vessel-based (B) PCAT segmentation against the hemodynamic significance of coronary stenosis. Solid points above the horizontal cutoff line denote features qualified for univariate logistic regression and LASSO-logistic regression, and their corresponding feature names are displayed. LASSO, least absolute shrinkage and selection operator; PCAT, pericoronary adipose tissue.
The discriminatory power of radiomics models was shown in Table 4. The AUC, specificity, and sensitivity of the radiomics model of lesion-based PCAT segmentation in the training set were 0.799, 86.8%, and 61.4%, 0.741, 80.0%, and 53.6% in the testing set, respectively (Figure 6). The vessel-based radiomics model produced comparable performance: 0.792, 78.9, and 70.5% in the training set, and 0.763, 64.0, and 67.9% in the testing set. No significant difference was detected between vessel-based and lesion-based PCAT segmentations for the prediction of ischemic coronary artery stenosis in both the training set (AUC: 0.792 vs. 0.799, p = 0.900) and the testing set (AUC: 0.764 vs. 0.741, p = 0.810).
Figure 6. ROC curves indicated comparable diagnostic performance between vessel-based and lesion-based PCAT radiomics models for identifying functionally significant coronary stenosis. PCAT, pericoronary adipose tissue; ROC, receiver operating characteristic.
The current study compared PCAT CT attenuation and radiomics features derived from different PCAT segmentations. Our study's main finding is that vessel-based segmentation shows good agreement with lesion-based segmentation, along with a small bias for PCAT CT attenuation. In addition, vessel-based and lesion-based PCAT radiomics models show comparable diagnostic performance in predicting ischemic stenosis.
Most studies (3–5, 7, 10, 13, 21) have validated that higher vessel-based PCAT CT attenuation was associated with an increased risk of cardiac mortality, poor prognosis, higher plaque burden and impaired myocardial perfusion. However, the association between vessel-based PCAT CT attenuation and the hemodynamic stenosis was not reported. A recent published study (6) demonstrated that the increase of lesion-based PCAT CT attenuation was associated with the hemodynamic significant of stenosis. Our study evaluated both lesion-based and vessel-based PCAT CT attenuation, as well as their respective diagnostic performance for the hemodynamic stenosis. The present results showed PCAT CT attenuation derived from vessel-based and lesion-based segmentations had a good agreement for all vessels analysis as well as sub-group analysis. However, the good correlation and agreement of PCAT CT attenuation between lesion-based and vessel-based segmentation in our study may be attributed to the location and length of the lesion. In this study, 80.7% (109/135) of lesions were located in the proximal to middle segments of coronary artery, with the mean lesion length of 26.2 ± 12.3 mm. In view of good correlation between PCAT CT attenuation measurements from vessel segmentation and lesion segmentation, therefore, quantitative evaluation of PCAT can be reliable from both segmentations. As with real-world studies, the PACT segmentation can be at the discretion of the radiologists.
However, it was unexpected that PCAT CT attenuation was not significantly different between hemodynamically significant stenoses and non-significant ones, regardless of the PCAT segmentation method. This finding is inconsistent with two previous studies (6, 16). The mean PCAT CT attenuation in coronary arteries with FFR >0.80 was similar to the above-mentioned studies (6, 16). The discrepancy in PCAT CT attenuation between this study and the previous studies was predominantly in coronary arteries with FFR ≤ 0.80. The probable explanation may be attributed to the different diameter stenosis. Similar to previous studies (5, 16), patients with high PCAT CT attenuation usually had high-grade stenosis according to previous studies. In our clinical practice, most patients referred for FFR often had intermediate stenosis (30–70%). The mean stenosis diameter (64.0 ± 17.3%) in coronary arteries with FFR ≤ 0.80 was obviously less severe in our study than in the Yu et al. study (76.32 ± 8.52%) (6); thus, leading to a low mean PCAT CT attenuation. Statistical differences in degree of stenosis between coronary arteries with FFR >0.80 and those with FFR ≤ 0.80 did differ between Yu et al. (6) and our study, however, the diagnostic performance of PCAT CT attenuation in predicting ischemic stenosis in Yu et al. was slightly superior than in our study (AUC: 0.630 vs. 0.547 and 0.524). In the Hoshino et al. study, several patients with severe stenosis (FFR < 0.5) with elevated PCAT CT attenuation were enrolled, thus leading to a higher average PCAT CT attenuation. However, patients enrolled in the present study were hemodynamically less severe, which is reflected in the minimal FFR value of 0.59. Thus, the mean PCAT CT attenuation in coronary arteries with FFR ≤ 0.80 was lower, and not significantly different from those with FFR >0.80.
PCAT analysis was also performed around either all three major epicardial coronary vessels (5, 7, 9–11) or one of the coronary arteries (RCA or LAD) (2–4, 8, 12–16). The CRISP CT showed the statistical collinearity between PCAT CT attenuation measurements around RCA and LAD. Our study extended earlier findings and indicated that the high correlation between vessel-based and lesion-based PCAT CT attenuation was also detected in all three major epicardial coronary vessels.
Radiomics analysis suggested that no significant differences were found between lesion-based and vessel-based radiomics models with comparable AUCs (0.799 vs. 0.792 in training set, and 0.741 vs. 0.764 in testing set). Radiomics features were mainly limited to intensity and texture, although the detailed features were not identical in the two segmentation methods. Among the significant radiomics features, texture homogeneity and wavelet-transformed intensity distribution within PCAT were particularly important in predicting the hemodynamic significance of coronary stenosis, regardless of different segmentation methods. A possible reason is that coronary stenoses are usually followed by an inflammatory response, which stimulates neovascularization and fibrosis while breaking down PCAT (8). As a result of lipolysis, local inflammation leads to sporadic transformation of adipose tissue to an aqueous component, hence increasing CT attenuation and tissue heterogeneity. Therefore, intensity and texture radiomics features could be indications of such processes, which suggest the potential development of coronary stenoses.
Geometric characteristics such as flatness and elongation were also highlighted in our study, although PCAT was segmented with strictly defined rules. These metrics are defined as the square root of the ratio between the lesser and the largest principal components in a three-dimensional volume. In the vessel-based segmentations with constant length, elongation and flatness depend mainly on the stiffness of vessels. Vessels with flow-limiting (FFR ≤ 0.80) lesions were associated with impaired vasodilator capacity, and were likely stiffer than vessels with non-flow-limiting lesions (6). The visually indiscernible slight change of geometric features of PCAT adjacent to vessel wall could be captured by radiomics. Therefore, the two geometric radiomics features of elongation and flatness may be associated with FFR.
This study had some limitations. Firstly, this was a single-center, retrospective study with a relatively small sample size. An inclusion bias existed because the study population did not include patients with minimal or severe stenosis. Furthermore, due to its retrospective nature, the impact of the related clinical factors on PCAT CT attenuation, such as serum highly sensitive C-reactive protein level, left ventricular ejection fractions, left ventricular hypertrophy, etc., could not be investigated in our study. Secondly, all patients underwent CCTA using the same CT scanner and protocol. Hence, the generalizability of our findings to other populations may be limited, as image acquisition and reconstruction settings can affect the reproducibility of PCAT CT attenuation and radiomics features. Thirdly, the software for PCAT quantification is limited to a single vendor and is not yet commercially available. Finally, we did not exclude the patients treated by statins in this study, and potentially introduced unnecessary bias to our results as statins may influence PCAT CT attenuation and PCAT radiomics.
This study demonstrated that quantitative evaluation of PCAT can be reliably measured both from lesion-based and vessel-based segmentation. We also found that PCAT radiomics features may potentially help predict hemodynamically significant coronary stenoses.
The original contributions presented in the study are included in the article/Supplementary Material, further inquiries can be directed to the corresponding author.
The studies involving human participants were reviewed and approved by Xijing Hospital of Fourth Military Medical University. The patients/participants provided their written informed consent to participate in this study.
MZ: guarantor of integrity of the entire study. DW and MZ: study concepts and design. RA: literature research. WY and RA: clinical studies. SL and YJ: experimental studies and data analysis. DW and SL: statistical analysis. DW and RA: manuscript preparation. RA and MZ: manuscript editing. All authors contributed to the article and approved the submitted version.
This study has received funding from the National Natural Science Foundation of China (Grant No. 82071917 to MZ), the Key Research and Development Plan of Shaanxi Province (Grant No. 2020ZDLSF01-01 to MZ), the National Science Foundation of Shaanxi Province (Grant No. 2020JQ-461 to DW), and the Discipline Promotion Projects of Xijing Hospital (Grant No. XJZT19Z13 to MZ).
SL was employed by the company Siemens Healthineers Ltd.
The remaining authors declare that the research was conducted in the absence of any commercial or financial relationships that could be construed as a potential conflict of interest.
All claims expressed in this article are solely those of the authors and do not necessarily represent those of their affiliated organizations, or those of the publisher, the editors and the reviewers. Any product that may be evaluated in this article, or claim that may be made by its manufacturer, is not guaranteed or endorsed by the publisher.
The Supplementary Material for this article can be found online at: https://www.frontiersin.org/articles/10.3389/fcvm.2022.773524/full#supplementary-material
1. Libby P, Loscalzo J, Ridker PM, Farkouh ME, Hsue PY, Fuster V, et al. Inflammation, immunity, and infection in atherothrombosis: JACC review topic of the week. J Am Coll Cardiol. (2018) 72:2071–81. doi: 10.1016/j.jacc.2018.08.1043
2. Lin A, Kolossváry M, Yuvaraj J, Cadet S, McElhinney PA, Jiang C, et al. Myocardial infarction associates with a distinct pericoronary adipose tissue radiomic phenotype: a prospective case-control study. JACC Cardiovasc Imaging. (2020) 13:2371–83. doi: 10.1016/j.jcmg.2020.06.033
3. Goeller M, Tamarappoo BK, Kwan AC, Cadet S, Commandeur F, Razipour A, et al. Relationship between changes in pericoronary adipose tissue attenuation and coronary plaque burden quantified from coronary computed tomography angiography. Eur Heart J Cardiovasc Imaging. (2019) 20:636–43. doi: 10.1093/ehjci/jez013
4. Goeller M, Rahman Ihdayhid A, Cadet S, Lin A, Adams D, Thakur U, et al. Pericoronary adipose tissue and quantitative global non-calcified plaque characteristics from CT angiography do not differ in matched south asian, east asian and european-origin caucasian patients with stable chest pain. Eur J Radiol. (2020) 125:108874. doi: 10.1016/j.ejrad.2020.108874
5. Goeller M, Achenbach S, Cadet S, Kwan AC, Commandeur F, Slomka PJ, et al. Pericoronary adipose tissue computed tomography attenuation and high-risk plaque characteristics in acute coronary syndrome compared with stable coronary artery disease. JAMA Cardiol. (2018) 3:858–63. doi: 10.1001/jamacardio.2018.1997
6. Yu M, Dai X, Deng J, Lu Z, Shen C, Zhang J. Diagnostic performance of perivascular fat attenuation index to predict hemodynamic significance of coronary stenosis: a preliminary coronary computed tomography angiography study. Eur Radiol. (2020) 30:673–81. doi: 10.1007/s00330-019-06400-8
7. Oikonomou EK, Marwan M, Desai MY, Mancio J, Alashi A, Hutt Centeno E, et al. Non-invasive detection of coronary inflammation using computed tomography and prediction of residual cardiovascular risk (the CRISP CT study): a post-hoc analysis of prospective outcome data. Lancet. (2018) 392:929–39. doi: 10.1016/S0140-6736(18)31114-0
8. Oikonomou EK, Williams MC, Kotanidis CP, Desai MY, Marwan M, Antonopoulos AS, et al. A novel machine learning-derived radiotranscriptomic signature of perivascular fat improves cardiac risk prediction using coronary CT angiography. Eur Heart J. (2019) 40:3529–43. doi: 10.1093/eurheartj/ehz592
9. Balcer B, Dykun I, Schlosser T, Forsting M, Rassaf T, Mahabadi AA. Pericoronary fat volume but not attenuation differentiates culprit lesions in patients with myocardial infarction. Atherosclerosis. (2018) 276:182–8. doi: 10.1016/j.atherosclerosis.2018.05.035
10. Nomura CH, Assuncao AN Jr, Guimarães PO, Liberato G, Morais TC, Fahel MG, et al. Association between perivascular inflammation and downstream myocardial perfusion in patients with suspected coronary artery disease. Eur Heart J Cardiovasc Imaging. (2020) 21:599–605. doi: 10.1093/ehjci/jeaa023
11. Tzolos E, McElhinney P, Williams MC, Cadet S, Dweck MR, Berman DS, et al. Repeatability of quantitative pericoronary adipose tissue attenuation and coronary plaque burden from coronary ct angiography. J Cardiovasc Comput Tomogr. (2021) 15:81–4. doi: 10.1016/j.jcct.2020.03.007
12. Almeida S, Pelter M, Shaikh K, Cherukuri L, Birudaraju D, Kim K, et al. Feasibility of measuring pericoronary fat from precontrast scans: effect of iodinated contrast on pericoronary fat attenuation. J Cardiovasc Comput Tomogr. (2020) 14:490–4. doi: 10.1016/j.jcct.2020.04.004
13. Kwiecinski J, Dey D, Cadet S, Lee SE, Otaki Y, Huynh PT, et al. Peri-coronary adipose tissue density is associated with 18F-sodium fluoride coronary uptake in stable patients with high-risk plaques. JACC Cardiovasc Imaging. (2019) 12:2000–10. doi: 10.1016/j.jcmg.2018.11.032
14. Elnabawi YA, Oikonomou EK, Dey AK, Mancio J, Rodante JA, Aksentijevich M, et al. Association of biologic therapy with coronary inflammation in patients with psoriasis as assessed by perivascular fat attenuation index. JAMA Cardiol. (2019) 4:885–91. doi: 10.1001/jamacardio.2019.2589
15. Eisenberg E, McElhinney PA, Commandeur F, Chen X, Cadet S, Goeller M, et al. Deep learning-based quantification of epicardial adipose tissue volume and attenuation predicts major adverse cardiovascular events in asymptomatic subjects. Circ Cardiovasc Imaging. (2020) 13:e009829. doi: 10.1161/CIRCIMAGING.119.009829
16. Hoshino M, Yang S, Sugiyama T, Zhang J, Kanaji Y, Yamaguchi M, et al. Peri-coronary inflammation is associated with findings on coronary computed tomography angiography and fractional flow reserve. J Cardiovasc Comput Tomogr. (2020) 14:483–9. doi: 10.1016/j.jcct.2020.02.002
17. Dai X, Deng J, Yu M, Lu Z, Shen C, Zhang J. Perivascular fat attenuation index and high-risk plaque features evaluated by coronary CT angiography: relationship with serum inflammatory marker level. Int J Cardiovasc Imaging. (2020) 36:723–30. doi: 10.1007/s10554-019-01758-8
18. Scanlon PJ, Faxon DP, Audet AM, Carabello B, Dehmer GJ, Eagle KA, et al. ACC/AHA guidelines for coronary angiography. A report of the American College of Cardiology/American Heart Association task force on practice guidelines (committee on coronary angiography). Developed in collaboration with the society for cardiac angiography and interventions. J Am Coll Cardiol. (1999) 33:1756–824. doi: 10.1016/s0735-1097(99)00126-6
19. Cami E, Tagami T, Raff G, Gallagher MJ, Fan A, Hafeez A, et al. Importance of measurement site on assessment of lesion-specific ischemia and diagnostic performance by coronary computed tomography angiography-derived fractional flow reserve. J Cardiovasc Comput Tomogr. (2021) 15:114–20. doi: 10.1016/j.jcct.2020.08.005
20. Yu M, Shen C, Dai X, Lu Z, Wang Y, Lu B, et al. Clinical outcomes of dynamic computed tomography myocardial perfusion imaging combined with coronary computed tomography angiography versus coronary computed tomography angiography-guided strategy. Circ Cardiovasc Imaging. (2020) 13:e009775. doi: 10.1161/CIRCIMAGING.119.009775
Keywords: pericoronary adipose tissue, pericoronary adipose tissue CT attenuation, radiomics analysis, ischemic coronary artery stenosis, fractional flow reserve
Citation: Wen D, An R, Lin S, Yang W, Jia Y and Zheng M (2022) Influence of Different Segmentations on the Diagnostic Performance of Pericoronary Adipose Tissue. Front. Cardiovasc. Med. 9:773524. doi: 10.3389/fcvm.2022.773524
Received: 10 September 2021; Accepted: 10 February 2022;
Published: 03 March 2022.
Edited by:
Giuseppe Signoriello, University of Campania Luigi Vanvitelli, ItalyReviewed by:
Jiayin Zhang, Shanghai General Hospital, ChinaCopyright © 2022 Wen, An, Lin, Yang, Jia and Zheng. This is an open-access article distributed under the terms of the Creative Commons Attribution License (CC BY). The use, distribution or reproduction in other forums is permitted, provided the original author(s) and the copyright owner(s) are credited and that the original publication in this journal is cited, in accordance with accepted academic practice. No use, distribution or reproduction is permitted which does not comply with these terms.
*Correspondence: Minwen Zheng, emhlbmdtdzIwMDdmbW11QDE2My5jb20=
†These authors have contributed equally to this work and share first authorship
Disclaimer: All claims expressed in this article are solely those of the authors and do not necessarily represent those of their affiliated organizations, or those of the publisher, the editors and the reviewers. Any product that may be evaluated in this article or claim that may be made by its manufacturer is not guaranteed or endorsed by the publisher.
Research integrity at Frontiers
Learn more about the work of our research integrity team to safeguard the quality of each article we publish.