- 1School of Medicine, South China University of Technology, Guangzhou, China
- 2Department of Cardiology, Guangdong Provincial People’s Hospital (Guangdong Academy of Medical Sciences), Southern Medical University, Guangzhou, China
- 3Department of Cardiology, Guangdong Provincial People’s Hospital Zhuhai Hospital (Zhuhai Golden Bay Center Hospital), Zhuhai, China
- 4Medical Research Center, Guangdong Provincial Key Laboratory of South China Structural Heart Disease, Guangdong Provincial People’s Hospital (Guangdong Academy of Medical Sciences), Southern Medical University, Guangzhou, China
Background: Blood lipids disorder and atherosclerosis are closely related to coronary artery disease (CAD). This study aims to compare different blood lipid parameters combined with carotid intima-media thickness (cIMT) in predicting CAD.
Methods: This was a retrospective study including patients who underwent coronary angiography for highly suspected CAD. Blood samples were taken for lipid profile analysis and cIMT was evaluated by carotid ultrasound. Logistic analysis was used to establish different models of different lipid parameters in predicting CAD. The area under the receiver operating characteristic curve (AUC) was used to examine the predictive value. The optimal lipid parameter was also used to explore the relationship with multi-vessel CAD.
Results: Patients were classified into two groups based on whether CAD existed. Compared with non-CAD patients, the CAD group had higher lipoprotein (a) [Lp (a)], apolipoprotein B/apolipoprotein A, total cholesterol/high-density lipoprotein cholesterol (HDL-C), triglyceride/HDL-C and LDL-C/HDL-C. According to the AUCs, Lp (a) combined with cIMT (AUC: 0.713, P < 0.001) had the best performance in predicting CAD compared to other lipid parameters. High level of Lp (a) was also associated with multi-vessel CAD (odds ratio: 1.41, 95% confidence interval: 1.02–1.95, P = 0.036).
Conclusion: For patients with highly suspected CAD, Lp (a) better improved the predictive value of CAD rather than most of blood lipid indices, especially in the absence of high levels of LDL-C. Lp (a) also can be used to predict the multi-vessel CAD.
Introduction
Although the treatment of coronary artery disease (CAD) has greatly improved in recent years, the incidence and mortality of CAD remains high (1). Atherosclerosis, as the main pathophysiological mechanism of CAD, closely related to lipid disorders that is poor-controlled (2). Early screening of CAD based on atherosclerosis is of great importance, and there is an urgent need to predict CAD using clinically available information.
The role of lipid parameters in CAD is controversial. Previous studies have shown that blood lipid indices, such as triglyceride/high-density lipoprotein cholesterol (TG/HDL-C) ratio, apolipoprotein B/apolipoprotein A-I (Apo B/Apo A-I) ratio, total cholesterol (TC)/HDL-C ratio or low-density lipoprotein cholesterol (LDL-C)/HDL-C have a better predictive value of CAD than a single parameter (3–8). Recent findings showed that blood lipid indices were significantly correlated with the risk of intracranial atherosclerotic stenosis and metabolic syndrome (9, 10). The combination of lipid parameters represents relative status of blood lipids, which can comprehensively reflect the balance between atherosclerosis and anti-atherosclerosis and accurately assess lipid deposition (11, 12). Lipoprotein (a) [Lp (a)], which has LDL-like moiety comprising Apo B and apolipoprotein (a) [Apo (a)], is independently associated with the diagnosis and prognosis of CAD (13). In an Asian population study, Lp (a) concentration was a risk factor for CAD, and higher levels of Lp (a) increased the risk of CAD (14). As a marker of the residual risk of cardiovascular disease, Lp (a) has a stronger correlation with CAD than LDL-C when LDL-C is in low levels (15, 16). However, it is still unknown whether Lp (a) is superior than the new lipid indices in predicting CAD.
Carotid intima-media thickness (cIMT), a widely used non-invasive marker of subclinical atherosclerosis, can also be used as a preliminary screening tool for CAD (17–19). However, a meta-analysis suggested that the sensitivity and specificity of cIMT for the diagnosis of CAD were quite low, indicating that using cIMT alone as CAD screening tool was still insufficient (20). Since the total load of atherosclerosis is closely related to the concentration of blood lipids, morphological assessment of atherosclerosis based on cIMT has certain limitations in predicting CAD (21–24). Considering subclinical atherosclerosis status and blood lipid profiles together would effectively improve the accuracy of the diagnosis of CAD.
In clinical screening for CAD, blood lipid parameters and cIMT are considered to be available tools. However, it is still unknown which blood lipid parameters combined with cIMT has the optimal accuracy in predicting CAD. Therefore, this study was aimed to compare the predictive value of different blood lipid parameters combined with cIMT on CAD.
Materials and methods
Study design and participants
This retrospective study included 1,598 consecutive patients who underwent coronary angiography for highly suspected CAD, including chest pain with typical change in ECG or severe lesion in coronary CT angiography, from September 2014 to July 2015 in Guangdong Provincial People’s Hospital. A total of 105 patients with a history of stroke, 24 patients without cIMT measurement, and 74 patients with missing information on blood lipid parameters were excluded.
This study was approved by the Ethics Committee of Guangdong Provincial People’s Hospital, and informed verbal consent was obtained from all patients. This study was conducted in accordance with the Declaration of Helsinki.
Data collection and measurements
Demographic data, laboratory test results, and carotid ultrasonography and coronary angiography results from the electronic medical records were collected.
The measurement of cIMT by carotid ultrasonography was detailed in our previous study (25). Carotid artery ultrasound was performed by experienced sonographers using the GE Vivid E95 (GE Healthcare, Milwaukee, WI, USA) interfaced with a 7.5–12 MHz phased array probe. The ultrasound probe scanned the entire length of the carotid artery longitudinally (from the bottom of the neck to the angle of the mandible). The region of interest for cIMT measurement is located at the far wall of the bilateral carotid arteries proximal to the bifurcation, along with ≥ 10 mm of plaque-free lesions on each side. Mean cIMT was calculated as the mean of two sides of cIMT. Two sonographers performed the cIMT measurements and determined the final results together. If there was any discrepancy, the results were determined together with a third sonographer. All patients underwent coronary angiography, and the degree of coronary stenosis was judged by two experienced cardiologists. Hypertension was diagnosed according to the European Society of Cardiology guidelines (26). Diabetes mellitus (DM) was defined based on the presence of diabetes or was diagnosed during hospitalization following the criteria of the European Society of Cardiology guidelines (27). Chronic kidney disease (CKD) was defined as previous medical history. Smoking was defined as previous or current smoking. Alcohol consumption was defined as previous drinking habit. Lp (a) was measured by AU5800 spectrophotometer (Beckman Coulter, USA) via immunoturbidimetry, with trihydroxy aminomethane buffer and anti Lp (a) antibody sensitized granules. HDL-C, LDL-C, TC, TG, Apo A-I, and Apo B were also detected using AU5800 spectrophotometer (Beckman Coulter, USA) via colorimetry or immunoturbidimetry. Based on previous studies (3–10), we selected six lipid ratios, Apo B/Apo A-I, LDL-C/Apo B, TC/HDL-C, TG/HDL-C, LDL-C/HDL-C, and HDL-C/Apo A-I, that may be associated with CAD.
Definitions
As suggested by the American College of Cardiology in 2016, a ≥ 70% luminal diameter narrowing of an epicardial stenosis or ≥ 50% luminal diameter narrowing of the left main artery observed by visual assessment was considered as severe lesion that used as the diagnostic criteria for CAD (28). Two or more coronary arteries with severe stenosis were defined as multi-vessel CAD.
Statistical analysis
The total procedure of statistical analysis was divided into four steps. First, Student’s t-test was used for normally distributed data, the Mann–Whitney U test was used for non-normally distributed data, and the Chi-square test or Fisher’s exact test was used for categorical variables to identify significant differences between two groups. Second, except for blood lipid parameters, the basic prediction model considered potential confounding factors that were both clinically and statistically significant in a backward stepwise logistic regression model (with 0.1 significance level for removal), and odds ratios (ORs) with 95% confidence intervals (CIs) were calculated. Third, a receiver operating characteristic (ROC) curve was constructed to evaluate the sensitivity, specificity, and area under the ROC curve (AUC) of different lipid parameters in predicting CAD based on the basic prediction model. Furthermore, net reclassification improvement (NRI) and integrated discrimination improvement (IDI) were calculated to evaluate the improvement of the new model when compared with the basic prediction model at low (< 50%)/intermediate (50–80%)/high risk (> 80%) of CAD. The 95% CI of NRI was obtained after bootstrapping 10,000 times. Fourth, a fully adjusted logistic model was used to explore the relationship between the optimal blood lipid parameter and multi-vessel CAD in the subgroup of diagnosed CAD. Comparisons with P < 0.05 (two-sided) were considered to be statistically significant. All of the analyses were performed with Stata 15.0 (StataCorp LLC, College Station, TX, USA), R version 3.4.3 (The R Project for Statistical Computing, Vienna, Austria), and EmpowerStats (X&Y Solutions, Inc., Boston, MA, USA).
Results
Study population
A total of 1,395 patients were eventually enrolled in this study (Figure 1). Baseline information of patients with and without CAD is presented in Table 1. There was no significant difference in LDL-C between the two groups. Hypertension, DM, and CKD were more prevalent in the CAD group. As for blood lipid indices, TC/HDL-C, LDL-C/HDL-C, Apo B/Apo A-I, and TG/HDL-C ratios were higher, whereas the LDL-C/Apo B ratio was lower in the CAD group. Lp (a) and mean-cIMT were also significantly higher in patients with CAD than in those without CAD. In addition, for medication history, statins was more commonly used in the CAD group.
Univariate and multivariate analysis
Univariate and stepwise multivariate logistic models were performed to select both clinically and statistically significant risk factors (Table 2). Age, sex, hypertension, smoking, alcohol consumption, DM, and mean-cIMT were associated with CAD and included in the basic prediction model. Among these, mean-cIMT was the strongly associated with the presence of CAD (OR 2.73, 95% CI: 1.64–4.52, P < 0.001).
Predictive value of different blood lipid parameters on CAD
The ROC curves of the different blood lipid parameters on the basic prediction model for predicting CAD are shown in Figure 2. The addition of Lp (a) as well as Apo B/Apo A-I ratio improved the prediction effect of the basic prediction model [AUC: 0.7129 for Lp (a), P < 0.001; AUC: 0.6848 for Apo B/Apo A-I ratio, P < 0.05]. However, when LDL-C/Apo B, TC/HDL-C, TG/HDL-C, and LDL-C/HDL-C ratios were added into the basic prediction model, the AUC of the new model was not significantly improved.
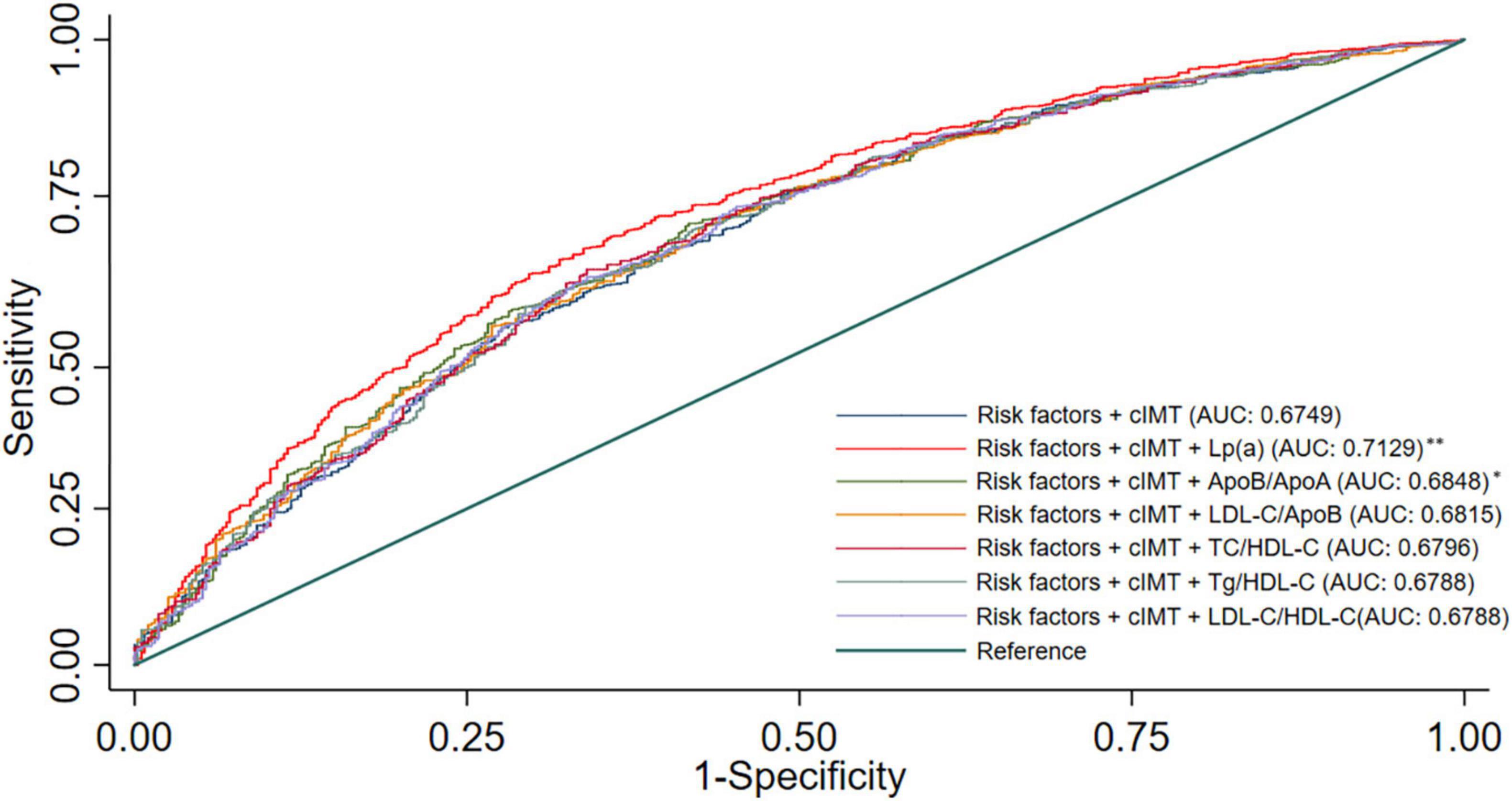
Figure 2. Receiver operating characteristic curves for models with different lipid parameters. Illustration: Basic prediction model adjusted for age, sex, hypertension, smoking, alcohol consumption, DM, and mean-cIMT; *P < 0.05 compared with basic prediction model; **P < 0.001 compared with basic prediction model.
The new model that included Lp (a) achieved an NRI of 12.8%, as compared with the basic prediction model for predicting CAD (Table 3), which means that 12.8% of patients were correctly reclassified. The IDI is also listed in Table 3 and also showed a significant improvement in accuracy generated with the new model. These results indicated that the new model including Lp (a) had better predictive capability for CAD than the other blood lipid indices.
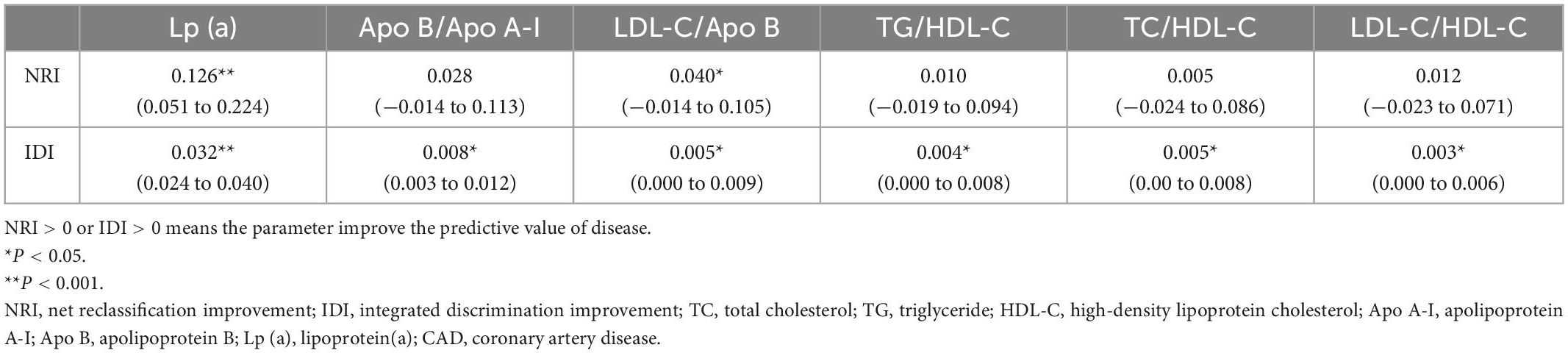
Table 3. Comparison of different lipid parameters for predicting CAD based on basic prediction model using the NRI and IDI with cut-off point at low (< 50%)/intermediate (50–80%)/high risk (> 80%) of CAD.
Association between Lp (a) and multi-vessel CAD
As shown in Figure 3, after adjusting for other risk factors in the CAD group (n = 1004), high levels of Lp (a) was associated with multi-vessel CAD than those with low levels of Lp (a) (OR 1.41, 95% CI 1.02–1.95, P = 0.036).
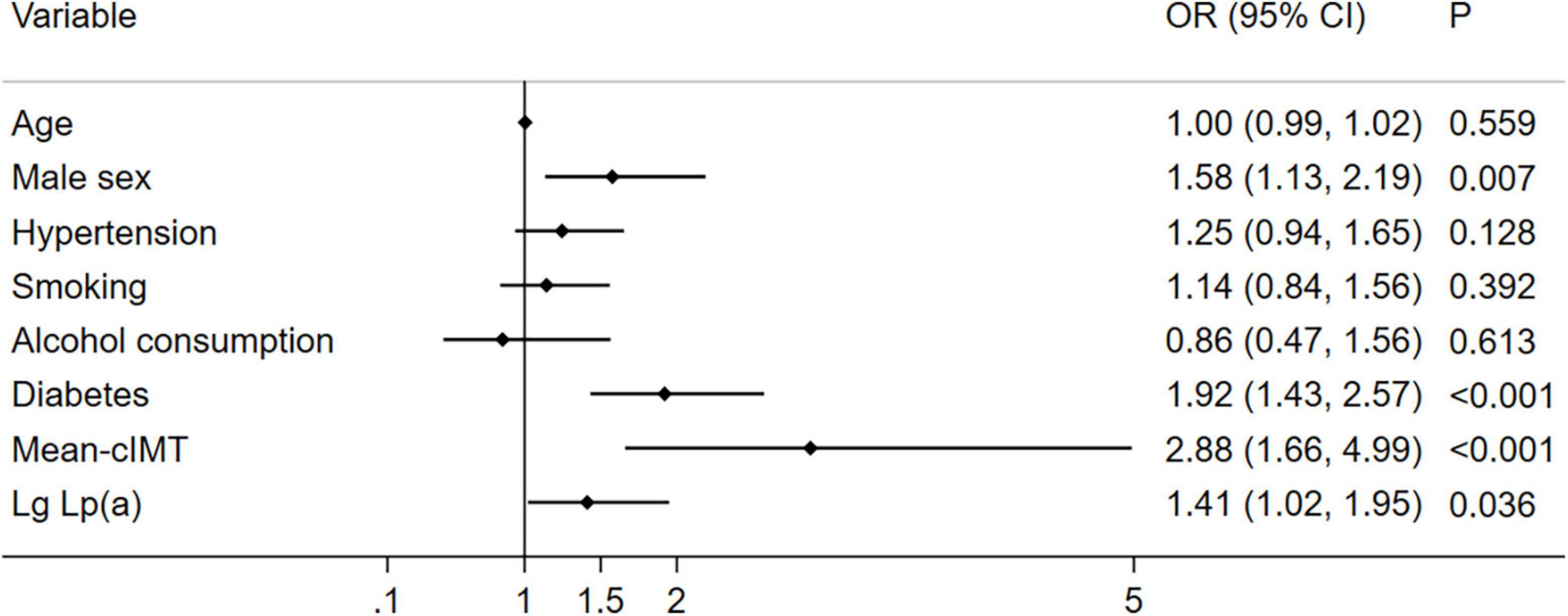
Figure 3. Relationship between Lp (a) combined with cIMT and multi-vessel CAD (n = 1004). Illustration: Lp (a) was transferred to Lg Lp (a).
Discussion
By comparing the predictive value of different blood lipid parameters combined with cIMT on CAD, the study had three major findings: (1) Lp (a) better improved the prediction accuracy of CAD in comparison with other blood lipid parameters; (2) in the absence of high levels of LDL-C, the predictive value of Lp (a) on CAD was highlighted; (3) Lp (a) can also be used to predict multi-vessel CAD.
Atherosclerosis is a systemic vascular disease caused by a lipid metabolism disorder and most likely involves the carotid and coronary arteries (29). Although coronary angiography is the gold standard for the diagnosis of CAD, it is an invasive examination that is unsuitable for general screening of CAD. In addition to identifying traditional risk factors such as demographic information and healthy behavior to predict CAD, screening for subclinical atherosclerosis is also important. Carotid ultrasound is a non-invasive examination (18). cIMT is widely recognized as a marker of early stage atherosclerosis and the severity of CAD, which can be used to indirectly assess coronary artery conditions (19, 30). Many studies have shown that cIMT is associated with cardiovascular risk factors and cardiovascular disease (CVD) (31, 32). Furthermore, several cohort studies have found that increased cIMT is associated with future cardiovascular events (33, 34). However, the results of a meta-analysis indicated that the sensitivity and specificity of cIMT for the diagnosis of CAD were 0.68 (95% CI: 0.57–0.77) and 0.70 (95% CI: 0.64–0.75), respectively, which indicated that only cIMT has limited effectiveness as a diagnostic tool for CAD screening (19). In addition, since lipid metabolism disorders cannot be reflected directly, it is of limited value in the use of single cIMT in actual clinical application (21, 35). Combining cIMT and lipid parameters could further improve the diagnostic efficiency of CAD. In recent years, research progress regarding lipid abnormalities has focused on the blood lipid indices, which can reflect lipid metabolism disorders better than a single one (36–38). Wu et al found that multivariate logistic regression analysis showed that Apo B/Apo A-I ratio, a composite index, had a larger OR value than a single index (LDL-C or Apo B) and was better than a single lipid index in the prediction of CAD (36). Similarly, in a cohort study by Rabizadeh et al. the analysis showed that LDL-C/Apo B ratio ≤ 1.2 can independently predict CAD (OR = 1.841, P = 0.002) (37). In a cohort study conducted by Kappelle et al. Apo B/Apo A-I ratio and TC/HDL-C ratio were able to predict CAD and the first major adverse event during follow-up (38). Consistent with previous studies, this study found that Lp (a), TC/HDL-C, LDL-C/HDL-C, Apo B/Apo AI, TG/HDL-C, and LDL-C/Apo B ratios were significantly associated with CAD. In terms of effectively predicting CAD, the addition of lipid parameters on traditional risk factors model improved the accuracy of the estimates, and Lp (a) was the best.
Many studies have reported that elevated plasma Lp (a) is an independent risk factor for CAD, which mainly participates in the pathophysiological process of CAD via prothrombotic/anti-fibrinolytic effects and promoting the deposition of cholesterol in the vascular intima (39–41). On an equimolar basis, Lp (a) is more likely to cause atherosclerosis than LDL because it not only contains all proatherogenic components of LDL-C but also those of Apo (a) (13). Because the structure of Lp (a) has one more Apo (a) than that of LDL-C, its ability to enhance atherosclerotic thrombosis through other mechanisms including inflammation is stronger than that of LDL-C (42). In a chart-controlled study of 143,087 subjects, Lp (a) concentration was associated with the risk of CAD in a dose-dependent manner, with the risk of CAD increasing as the percentile level of Lp (a) increased (43). With the development of genetic technology, the relationship between Lp (a) and CVD has been well elucidated at the genetic level (44). Many large observational and genetic epidemiological studies have shown that high Lp (a) levels and corresponding LPA genotypes increase the risk of CVD (45, 46). In addition, elevated level of LDL-C is strongly associated with the development of CAD (47). The 2019 ESC guidelines also recommend using LDL-C as the primary indicator of lipid-lowering therapy (48). In the baseline information of the study patients, the LDL-C of the CAD group was similar to that of the non-CAD group, possibly because the CAD group took more statins. In such condition, the levels of LDL-C were comparable in both groups, and the additional effect of Lp (a) could be investigated. Previous studies have shown that patients still have a certain risk of CAD after the treatment for reducing LDL-C, and this residual risk was related to elevated Lp (a) (49–53). In our study, the mean LDL-C level in CAD group was 2.6 mmol/L, meeting the LDL-C management target at a low level. Among patients whose target blood lipids are normal or below the target, Lp (a) will play an important role in the prediction of CAD. This may explain the results that the AUC value of Lp (a) was greater than that of LDL-C-associated blood lipid indices in the ROC curve.
In addition, elevated Lp (a) levels were correlated with multi-vessel CAD. This result is consistent with a recent finding demonstrating that high Lp (a) was significantly associated with increased CAD severity, evaluated using the SYNTAX score (44). The findings of the present study highlight the importance of Lp (a) combined with cIMT in assessing the risk of CAD, especially for those with normal level of LDL-C, which may be useful for guiding primary prevention decisions.
This study has some strengths. This study compared the effectiveness of different lipid parameters combined with cIMT in predicting CAD in the absence of high LDL-C for the first time, and recognized that Lp (a) was superior to other lipid parameters in CAD discrimination, through NRI and IDI. This study has certain limitations. First, this was a retrospective study and no causal conclusion can be drawn. Second, Some people with CAD have a history of statin use, which may have an effect on Lp (a) levels. Third, this study lacked height and weight data to calculate individual body mass index (BMI). As the aim of our study was to compare the predictive value of different lipid parameters rather than to build a predictive model for CAD, the effect of BMI on the primary outcome of this study was not significant. Finally, the severity of CAD was based on the number of coronary artery lesions, and the corresponding degree of stenosis in each branch was not well quantified.
Conclusion
For patients with highly suspected CAD, Lp (a) combined with cIMT better improved the predictive value of CAD compared with other blood lipid parameters, especially in the absence of high levels of LDL-C. High concentration of Lp (a) was also associated with the multi-vessel CAD. In the future, Lp (a) may need more attention and management in the prevention of CAD.
Data availability statement
The raw data supporting the conclusions of this article will be made available by the authors, without undue reservation.
Ethics statement
The studies involving human participants were reviewed and approved by the Ethics Committee of Guangdong Provincial People’s Hospital. The patients/participants provided their written informed consent to participate in this study.
Author contributions
BY and YW: manuscript preparation and writing—original draft. WL, LZ, and YL: data collection and collation. BY, YW, and WW: data analysis. GL and YZ: writing—critical revisions. XH and XL: conceptualization and approval of the final version of the manuscript for submission. All authors read and approved the final manuscript.
Funding
This research was supported by the Guangzhou Municipal Science and Technology Grant (202002030112) and the National Key Research and Development Program of China (No. 2016YFC1301202).
Acknowledgments
The researchers are grateful to Accdon (www.accdon.com) for its linguistic assistance during the preparation of this manuscript.
Conflict of interest
The authors declare that the research was conducted in the absence of any commercial or financial relationships that could be construed as a potential conflict of interest.
Publisher’s note
All claims expressed in this article are solely those of the authors and do not necessarily represent those of their affiliated organizations, or those of the publisher, the editors and the reviewers. Any product that may be evaluated in this article, or claim that may be made by its manufacturer, is not guaranteed or endorsed by the publisher.
References
1. Virani S, Alonso A, Benjamin E, Bittencourt M, Callaway C, Carson A, et al. Heart disease and stroke statistics-2020 update: a report from the American Heart Association. Circulation. (2020) 141:e139–596. doi: 10.1161/CIR.0000000000000757
2. Spannella F, Giulietti F, Di Pentima C, Sarzani R. Prevalence and control of dyslipidemia in patients referred for high blood pressure: the disregarded “double-trouble” lipid profile in overweight/obese. Adv Ther. (2019) 36:1426–37. doi: 10.1007/s12325-019-00941-6
3. Gardener H, Della Morte D, Elkind M, Sacco R, Rundek T. Lipids and carotid plaque in the Northern Manhattan study (NOMAS). BMC Cardiovasc Disord. (2009) 9:55. doi: 10.1186/1471-2261-9-55
4. Sone H, Tanaka S, Tanaka S, Iimuro S, Ishibashi S, Oikawa S, et al. Comparison of various lipid variables as predictors of coronary heart disease in Japanese men and women with type 2 diabetes: subanalysis of the Japan diabetes complications study. Diabetes Care. (2012) 35:1150–7. doi: 10.2337/dc11-1412
5. Enomoto M, Adachi H, Hirai Y, Fukami A, Satoh A, Otsuka M, et al. LDL-C/HDL-C ratio predicts carotid intima-media thickness progression better than HDL-C or LDL-C alone. J Lipids. (2011) 2011:549137. doi: 10.1155/2011/549137
6. Takata K, Kataoka Y, Andrews J, Puri R, Hammadah M, Duggal B, et al. Triglyceride-to-high-density lipoprotein cholesterol ratio and vulnerable plaque features with statin therapy in diabetic patients with coronary artery disease: frequency-domain optical coherence tomography analysis. JACC Cardiovasc Imaging. (2018) 11:1721–3. doi: 10.1016/j.jcmg.2018.02.017
7. Ljungberg J, Holmgren A, Bergdahl I, Hultdin J, Norberg M, Näslund U, et al. Lipoprotein(a) and the apolipoprotein B/A1 ratio independently associate with surgery for aortic stenosis only in patients with concomitant coronary artery disease. J Am Heart Assoc. (2017) 6:e007160. doi: 10.1161/JAHA.117.007160
8. Byun A, Lee S, Lee H, Shim K. What is the most appropriate lipid profile ratio predictor for insulin resistance in each sex? A cross-sectional study in Korean populations (The fifth Korea national health and nutrition examination survey). Diabetol Metab Syndr. (2015) 7:59. doi: 10.1186/s13098-015-0051-2
9. Yang W, Li R, Shen Y, Wang X, Liu Q, Wang H, et al. Importance of lipid ratios for predicting intracranial atherosclerotic stenosis. Lipids Health Dis. (2020) 19:160.
10. Abbasian M, Delvarianzadeh M, Ebrahimi H, Khosravi F. Lipid ratio as a suitable tool to identify individuals with MetS risk: a case- control study. Diabetes Metab Syndr. (2017) 11 Suppl 1:S15–9. doi: 10.1016/j.dsx.2016.08.011
11. Marcovina S, Packard C. Measurement and meaning of apolipoprotein AI and apolipoprotein B plasma levels. J Intern Med. (2006) 259:437–46.
12. Yaseen R, El-Leboudy M, El-Deeb H. The relation between ApoB/ApoA-1 ratio and the severity of coronary artery disease in patients with acute coronary syndrome. Egypt Heart J. (2021) 73:24. doi: 10.1186/s43044-021-00150-z
13. Tsimikas SA. Test in context: lipoprotein(a): diagnosis, prognosis, controversies, and emerging therapies. J Am Coll Cardiol. (2017) 69:692–711. doi: 10.1016/j.jacc.2016.11.042
14. Loh W, Chang X, Aw T, Phua S, Low A, Chan M, et al. Lipoprotein(a) as predictor of coronary artery disease and myocardial infarction in a multi-ethnic Asian population. Atherosclerosis. (2022) 349:160–5. doi: 10.1016/j.atherosclerosis.2021.11.018
15. Shah N, Pajidipati N, McGarrah R, Navar A, Vemulapalli S, Blazing M, et al. Lipoprotein (a): an update on a marker of residual risk and associated clinical manifestations. Am J Cardiol. (2020) 126:94–102. doi: 10.1016/j.amjcard.2020.03.043
16. Li C, Chen Q, Zhang M, Liu Y, Chu Y, Meng F, et al. The correlation between lipoprotein(a) and coronary atherosclerotic lesion is stronger than LDL-C, when LDL-C is less than 104 mg/dL. BMC Cardiovasc Disord. (2021) 21:41. doi: 10.1186/s12872-021-01861-6
17. Kablak-Ziembicka A, Tracz W, Przewlocki T, Pieniazek P, Sokolowski A, Konieczynska M, et al. Association of increased carotid intima-media thickness with the extent of coronary artery disease. Heart. (2004) 90:1286–90.
18. Zhang Y, Guallar E, Qiao Y, Wasserman B. Is carotid intima-media thickness as predictive as other noninvasive techniques for the detection of coronary artery disease? Arterioscler Thromb Vasc Biol. (2014) 34:1341–5.
19. Nambi V, Chambless L, Folsom A, He M, Hu Y, Mosley T, et al. Carotid intima-media thickness and presence or absence of plaque improves prediction of coronary heart disease risk: the ARIC (Atherosclerosis Risk In Communities) study. J Am Coll Cardiol. (2010) 55:1600–7.
20. Liu D, Du C, Shao W, Ma G. Diagnostic role of carotid intima-media thickness for coronary artery disease: a meta-analysis. Biomed Res Int. (2020) 2020:9879463.
21. Vlachopoulos C, Xaplanteris P, Aboyans V, Brodmann M, Cífková R, Cosentino F, et al. The role of vascular biomarkers for primary and secondary prevention. A position paper from the European society of cardiology working group on peripheral circulation: endorsed by the association for research into arterial structure and physiology (ARTERY) society. Atherosclerosis. (2015) 241:507–32. doi: 10.1016/j.atherosclerosis.2015.05.007
22. Tabas I, Williams K, Borén J. Subendothelial lipoprotein retention as the initiating process in atherosclerosis: update and therapeutic implications. Circulation. (2007) 116:1832–44. doi: 10.1161/CIRCULATIONAHA.106.676890
23. Borén J, Williams K. The central role of arterial retention of cholesterol-rich apolipoprotein-B-containing lipoproteins in the pathogenesis of atherosclerosis: a triumph of simplicity. Curr Opin Lipidol. (2016) 27:473–83. doi: 10.1097/MOL.0000000000000330
24. Jellinger P, Handelsman Y, Rosenblit P, Bloomgarden Z, Fonseca V, Garber A, et al. American association of clinical endocrinologists and American college of endocrinology guidelines for management of dyslipidemia and prevention of cardiovascular disease. Endocr Pract. (2017) 23(Suppl. 2):1–87. doi: 10.4158/EP171764.GL
25. Hu X, Li W, Wang C, Zhang H, Lu H, Li G, et al. Association between the plasma-glycosylated hemoglobin A1c/high-density lipoprotein cholesterol ratio and carotid atherosclerosis: a retrospective study. J Diabetes Res. (2021) 2021:9238566. doi: 10.1155/2021/9238566
26. Williams B, Mancia G, Spiering W, Agabiti Rosei E, Azizi M, Burnier M, et al. 2018 ESC/ESH guidelines for the management of arterial hypertension. Eur Heart J. (2018) 39:3021–104. doi: 10.1097/HJH.0000000000001940
27. Cosentino F, Grant P, Aboyans V, Bailey C, Ceriello A, Delgado V, et al. 2019 ESC guidelines on diabetes, pre-diabetes, and cardiovascular diseases developed in collaboration with the EASD. Eur Heart J. (2020) 41:255–323.
28. Patel M, Calhoon J, Dehmer G, Grantham J, Maddox T, Maron D, et al. ACC/AATS/AHA/ASE/ASNC/SCAI/SCCT/STS 2016 appropriate use criteria for coronary revascularization in patients with acute coronary syndromes : a report of the American college of cardiology appropriate use criteria task force, American association for thoracic surgery, American heart association, American society of echocardiography, American society of nuclear cardiology, society for cardiovascular angiography and interventions, society of cardiovascular computed tomography, and the society of thoracic surgeons. J Nucl Cardiol. (2017) 24:439–63. doi: 10.1007/s12350-017-0780-8
29. Tuzcu E, Schoenhagen P. Acute coronary syndromes, plaque vulnerability, and carotid artery disease: the changing role of atherosclerosis imaging. J Am Coll Cardiol. (2003) 42:1033–6. doi: 10.1016/s0735-1097(03)00904-5
30. Lorenz M, Markus H, Bots M, Rosvall M, Sitzer M. Prediction of clinical cardiovascular events with carotid intima-media thickness: a systematic review and meta-analysis. Circulation. (2007) 115:459–67. doi: 10.1161/CIRCULATIONAHA.106.628875
31. Hensley B, Huang C, Cruz Martinez C, Shokoohi H, Liteplo A. Ultrasound measurement of carotid intima-media thickness and plaques in predicting coronary artery disease. Ultrasound Med Biol. (2020) 46:1608–13. doi: 10.1016/j.ultrasmedbio.2020.03.004
32. Mannami T, Konishi M, Baba S, Nishi N, Terao A. Prevalence of asymptomatic carotid atherosclerotic lesions detected by high-resolution ultrasonography and its relation to cardiovascular risk factors in the general population of a Japanese city: the Suita study. Stroke. (1997) 28:518–25. doi: 10.1161/01.str.28.3.518
33. Folsom A, Kronmal R, Detrano R, O’Leary D, Bild D, Bluemke D, et al. Coronary artery calcification compared with carotid intima-media thickness in the prediction of cardiovascular disease incidence: the multi-ethnic study of atherosclerosis (MESA). Arch Intern Med. (2008) 168:1333–9.
34. O’Leary D, Polak J, Kronmal R, Manolio T, Burke G, Wolfson S Jr., et al. Carotid-artery intima and media thickness as a risk factor for myocardial infarction and stroke in older adults. Cardiovascular health study collaborative research group. N Engl J Med. (1999) 340:14–22. doi: 10.1056/NEJM199901073400103
35. Cappelletti A, Astore D, Godino C, Bellini B, Magni V, Mazzavillani M, et al. Relationship between syntax score and prognostic localization of coronary artery lesions with conventional risk factors, plasma profile markers, and carotid atherosclerosis (CAPP Study 2). Int J Cardiol. (2018) 257:306–11. doi: 10.1016/j.ijcard.2017.12.012
36. Wu T, Zheng Y, Yang Y, Li X, Ma Y, Xie X, et al. Age, sex, and cardiovascular risk attributable to lipoprotein cholesterol among chinese individuals with coronary artery disease: a case-control study. Metab Syndr Relat Disord. (2019) 17:223–31. doi: 10.1089/met.2018.0067
37. Rabizadeh S, Rajab A, Mechanick J, Moosaie F, Rahimi Y, Nakhjavani M, et al. LDL/apo B ratio predict coronary heart disease in Type 2 diabetes independent of ASCVD risk score: a case-cohort study. Nutr Metab Cardiovasc Dis. (2021) 31:1477–85. doi: 10.1016/j.numecd.2021.01.013
38. Kappelle P, Gansevoort R, Hillege J, Wolffenbuttel B, Dullaart R, Prevend Study Group, et al. Apolipoprotein B/A-I and total cholesterol/high-density lipoprotein cholesterol ratios both predict cardiovascular events in the general population independently of nonlipid risk factors, albuminuria and C-reactive protein. J Intern Med. (2011) 269:232–42. doi: 10.1111/j.1365-2796.2010.02323.x
39. Mehta A, Virani S, Ayers C, Sun W, Hoogeveen R, Rohatgi A, et al. Lipoprotein(a) and family history predict cardiovascular disease risk. J Am Coll Cardiol. (2020) 76:781–93.
40. Nordestgaard B, Chapman M, Ray K, Borén J, Andreotti F, Watts G, et al. Lipoprotein(a) as a cardiovascular risk factor: current status. Eur Heart J. (2010) 31:2844–53. doi: 10.1093/eurheartj/ehq386
41. Jang A, Han S, Sohn I, Oh P, Koh K. Lipoprotein(a) and cardiovascular diseases - revisited. Circ J. (2020) 84:867-74. doi: 10.1253/circj.CJ-20-0051
42. Klein-Szanto A, Bassi D. Keep recycling going: new approaches to reduce LDL-C. Biochem Pharmacol. (2019) 164:336–41. doi: 10.1016/j.bcp.2019.04.003
43. Gudbjartsson D, Thorgeirsson G, Sulem P, Helgadottir A, Gylfason A, Saemundsdottir J, et al. Lipoprotein(a) concentration and risks of cardiovascular disease and diabetes. J Am Coll Cardiol. (2019) 74:2982–94.
44. Arsenault B, Kamstrup P. Lipoprotein(a) and cardiovascular and valvular diseases: a genetic epidemiological perspective. Atherosclerosis. (2022) 349:7–16. doi: 10.1016/j.atherosclerosis.2022.04.015
45. Kamstrup P. Lipoprotein(a) and cardiovascular disease. Clin Chem. (2021) 67:154–66. doi: 10.1093/clinchem/hvaa247
46. Reyes-Soffer G, Ginsberg H, Berglund L, Duell P, Heffron S, Kamstrup P, et al. Lipoprotein(a): a genetically determined, causal, and prevalent risk factor for atherosclerotic cardiovascular disease: a scientific statement from the American heart association. Arterioscler Thromb Vasc Biol. (2022) 42:e48–60. doi: 10.1161/ATV.0000000000000147
47. Mach F, Baigent C, Catapano A, Koskinas K, Casula M, Badimon L, et al. 2019 ESC/EAS guidelines for the management of dyslipidaemias: lipid modification to reduce cardiovascular risk. Eur Heart J. (2020) 41:111–88. doi: 10.15829/1560-4071-2020-3826
48. Piepoli M, Hoes A, Agewall S, Albus C, Brotons C, Catapano A, et al. 2016 European guidelines on cardiovascular disease prevention in clinical practice: the sixth joint task force of the European society of cardiology and other societies on cardiovascular disease prevention in clinical practice (constituted by representatives of 10 societies and by invited experts) developed with the special contribution of the European association for cardiovascular prevention & rehabilitation (EACPR). Eur Heart J. (2016) 37:2315–81.
49. Cai A, Li L, Zhang Y, Mo Y, Mai W, Zhou Y, et al. Lipoprotein(a): a promising marker for residual cardiovascular risk assessment. Dis Markers. (2013) 35:551–9.
50. Wei W, Li X, Feng Q, Kubo M, Kullo I, Peissig P, et al. LPA variants are associated with residual cardiovascular risk in patients receiving statins. Circulation. (2018) 138:1839–49. doi: 10.1161/CIRCULATIONAHA.117.031356
51. Hoogeveen R, Ballantyne C. Residual cardiovascular risk at low LDL: remnants, lipoprotein(a), and inflammation. Clin Chem. (2021) 67:143–53. doi: 10.1093/clinchem/hvaa252
52. Kelly E, Hemphill L. Lipoprotein(a): a lipoprotein whose time has come. Curr Treat Options Cardiovasc Med. (2017) 19:48. doi: 10.1007/s11936-017-0549-z
Keywords: lipoprotein (a), blood lipid indices, carotid intima-media thickness, carotid ultrasound, coronary artery disease
Citation: Yu B, Wu Y, Li W, Zhou L, Lin Y, Wang W, Li G, Zhou Y, Hu X and Li X (2023) Predictive effect of different blood lipid parameters combined with carotid intima-media thickness on coronary artery disease. Front. Cardiovasc. Med. 9:1105413. doi: 10.3389/fcvm.2022.1105413
Received: 22 November 2022; Accepted: 30 December 2022;
Published: 12 January 2023.
Edited by:
Yunzhao Hu, Shunde Hospital of Southern Medical University Affiliated Chencun Hospital, ChinaReviewed by:
Wei Huang, University of Cincinnati, United StatesYa Meng, Zhuhai People’s Hospital, China
Copyright © 2023 Yu, Wu, Li, Zhou, Lin, Wang, Li, Zhou, Hu and Li. This is an open-access article distributed under the terms of the Creative Commons Attribution License (CC BY). The use, distribution or reproduction in other forums is permitted, provided the original author(s) and the copyright owner(s) are credited and that the original publication in this journal is cited, in accordance with accepted academic practice. No use, distribution or reproduction is permitted which does not comply with these terms.
*Correspondence: Xiaohong Li, bGl4aWFvaG9uZ0BnZHBoLm9yZy5jbg==; Xiangming Hu,
aHhtZ2RjekAxNjMuY29t
†These authors have contributed equally to this work