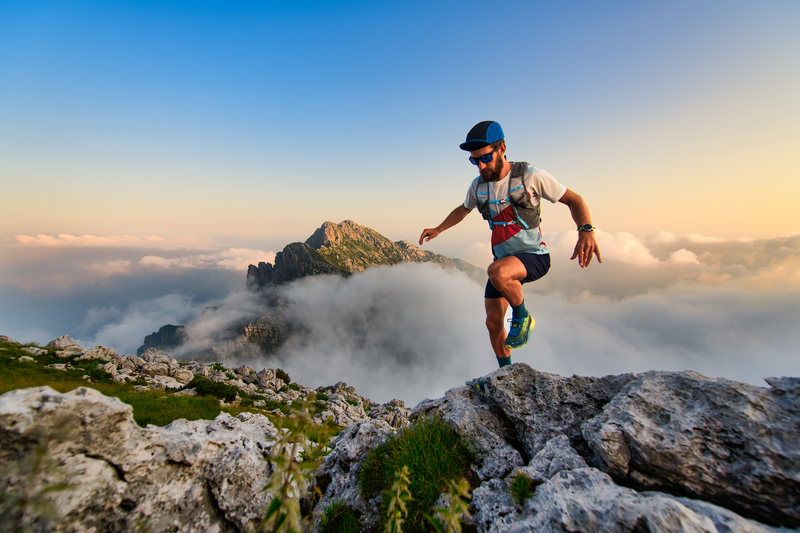
95% of researchers rate our articles as excellent or good
Learn more about the work of our research integrity team to safeguard the quality of each article we publish.
Find out more
ORIGINAL RESEARCH article
Front. Cardiovasc. Med. , 14 December 2022
Sec. Cardiovascular Imaging
Volume 9 - 2022 | https://doi.org/10.3389/fcvm.2022.1079046
Introduction: In-stent neoatherosclerosis has emerged as a crucial factor in post-stent complications including late in-stent restenosis and very late stent thrombosis. In this study, we investigated the ability of quantitative plaque characteristics from intravascular optical coherence tomography (IVOCT) images taken just prior to stent implantation to predict neoatherosclerosis after implantation.
Methods: This was a sub-study of the TRiple Assessment of Neointima Stent FOrmation to Reabsorbable polyMer with Optical Coherence Tomography (TRANSFORM-OCT) trial. Images were obtained before and 18 months after stent implantation. Final analysis included images of 180 lesions from 90 patients; each patient had images of two lesions in different coronary arteries. A total of 17 IVOCT plaque features, including lesion length, lumen (e.g., area and diameter); calcium (e.g., angle and thickness); and fibrous cap (FC) features (e.g., thickness, surface area, and burden), were automatically extracted from the baseline IVOCT images before stenting using dedicated software developed by our group (OCTOPUS). The predictive value of baseline IVOCT plaque features for neoatherosclerosis development after stent implantation was assessed using univariate/multivariate logistic regression and receiver operating characteristic (ROC) analyses.
Results: Follow-up IVOCT identified stents with (n = 19) and without (n = 161) neoatherosclerosis. Greater lesion length and maximum calcium angle and features related to FC were associated with a higher prevalence of neoatherosclerosis after stent implantation (p < 0.05). Hierarchical clustering identified six clusters with the best prediction p-values. In univariate logistic regression analysis, maximum calcium angle, minimum calcium thickness, maximum FC angle, maximum FC area, FC surface area, and FC burden were significant predictors of neoatherosclerosis. Lesion length and features related to the lumen were not significantly different between the two groups. In multivariate logistic regression analysis, only larger FC surface area was strongly associated with neoatherosclerosis (odds ratio 1.38, 95% confidence interval [CI] 1.05–1.80, p < 0.05). The area under the ROC curve was 0.901 (95% CI 0.859–0.946, p < 0.05) for FC surface area.
Conclusion: Post-stent neoatherosclerosis can be predicted by quantitative IVOCT imaging of plaque characteristics prior to stent implantation. Our findings highlight the additional clinical benefits of utilizing IVOCT imaging in the catheterization laboratory to inform treatment decision-making and improve outcomes.
Percutaneous coronary intervention (PCI) is the most common revascularization procedure for treating coronary artery disease, with an average of 660,000 procedures performed per year (1). Despite the advent of newer-generation drug-eluting stents that markedly reduced late thrombotic events (2, 3), PCI continues to be associated with late stent failure and stent thrombosis or restenosis (4–7). De novo development of atherosclerosis within the stent neointimal region, i.e., in-stent neoatherosclerosis (8–10), has emerged as a crucial contributing factor to late vascular complications including late in-stent restenosis and very late stent thrombosis (4–7, 10–16).
Neoatherosclerosis is characterized histologically by the accumulation of lipid-laden foamy macrophages with or without necrotic core formation and/or calcification within the neointimal tissue (17). Multiple studies have analyzed the characteristics and formation of neoatherosclerosis using intravascular imaging modalities, such as intravascular ultrasound (IVUS), near-infrared spectroscopy, and intravascular optical coherence tomography (IVOCT). IVUS and IVOCT can improve procedural success, and these techniques are recommended by current guidelines to evaluate the mechanisms of stent failure (18, 19). However, findings from IVUS should be interpreted with caution due to insufficient resolution (150–200 μm) to enable reliable tissue characterization. IVOCT, which has higher resolution (10–20 μm), has become one of the best methods to assess the neointimal tissue (20–22). IVOCT allows for comprehensive assessment of morphological characteristics of neoatherosclerotic plaque composition, such as macrophage infiltration, in-stent calcification, and neointimal rupture (21). On IVOCT images, neoatherosclerosis is mainly limited to lipidic and calcific neointima (23, 24): lipidic neointima appears as a signal-poor region with diffused borders and a fast IVOCT signal drop-off, whereas calcific neointima appears as a signal-poor region with sharply delineated borders (25, 26).
Several studies have identified key factors associated with neoatherosclerosis risk after PCI, including stent type (15, 17, 23, 27–32), clinical factors (24, 27, 33), plaque characteristics (11, 34–36), and stent characteristics (37); however, the mechanism underlying accelerated development of neoatherosclerosis after stent implantation remains unknown. Given that IVOCT provides better resolution of the neointima and neoatherosclerotic plaque characteristics than any other imaging modality, we investigated the value of quantitative IVOCT measurements before stent implantation for predicting post-stent neoatherosclerosis. Building on our previous work in the analysis of atherosclerotic plaque (38–51), we used a number of computational tools (i.e., machine learning, deep learning, and statistical modeling) to evaluate serial IVOCT images collected as part of a large clinical study. Using a dedicated software (OCTOPUS) (38), we performed plaque characterization in the baseline IVOCT images, computed IVOCT plaque features, and determined their association with the development of neoatherosclerosis after stent implantation.
This was a sub-study of the TRiple Assessment of Neointima Stent FOrmation to Reabsorbable polyMer with Optical Coherence Tomography (TRANSFORM-OCT) trial (52), a prospective, randomized, open-label, assessor-blinded, controlled study of patients with multivessel disease undergoing staged PCI with stent implantation at two hospitals in Italy. Patients with stable angina and documented ischemia or acute coronary syndrome who had undergone IVOCT examination were eligible for the study. Major exclusion criteria were the presence of unprotected left main disease, chronic total occlusion, baseline serum creatinine >2.0 mg/dL, life expectancy <18 months, and unsuitable for OCT imaging (at the investigator’s discretion). The full list of inclusion and exclusion criteria is provided in elsewhere (52). Final analysis included 180 lesions among 90 patients. Each patient had a staged PCI with the culprit lesion treated at the index timepoint and a second lesion treated 3 months later. This study was conducted in accordance with the Declaration of Helsinki and with approval from the Institutional Review Board of University Hospitals Cleveland Medical Center (Cleveland, OH, USA). Written informed consent was obtained from all patients.
Intravascular optical coherence tomography images were acquired with a frequency-domain OCT system (ILUMIEN OPTIS, Abbott Vascular, Santa Clara, CA, USA), which has a tunable laser light source sweeping from 1,250 to 1,360 nm at a frame rate of 180 fps. Baseline and follow-up IVOCT pullbacks were obtained for two independent lesions per patient. The first lesion was treated immediately after randomization, and the entire lesion was stented with one or more allocated stents. Similarly, the second lesion in a different coronary artery was covered by implanting the same allocated stent 3 months from randomization (Figure 1) (52). IVOCT imaging of the first lesion was obtained before and immediately after initial stent implantation (T0) and at 18 months after initial stent implantation (T2). The second lesion was imaged 3 months after randomization, before and after the second stent implantation (T1) and at 15 months after the second stent implantation (T2). Details of IVOCT image acquisition are provided elsewhere (52). Figure 2 shows a representative example of pre- and post-stent IVOCT images in a single study participant with evidence of neoatherosclerosis.
Figure 1. Diagram of intravascular optical coherence tomography (IVOCT) imaging timing, performed before and after first stent implantation (T0), 3 months later before and after second stent implantation (T1), and 18 months after the first implantation (T2).
Figure 2. Representative intravascular optical coherence tomography (IVOCT) images documenting the formation of in-stent neoatherosclerosis in one study participant. Images taken at (A) baseline, (B) 3-month follow-up, and (C) 18-month follow-up. Follow-up pullbacks (B,C) were manually registered to the baseline pullback (A). In panel (C), the green and red markers indicate the development of lipidic neoatherosclerosis and the formation of neointima, respectively.
Atherosclerotic plaque characteristics in pre-stent images were analyzed using a dedicated software (Optical Coherence TOmography PlaqUe and Stent, OCTOPUS), developed by our group (38, 41, 43–46). A total of 17 IVOCT features, including lesion length and several lumen, calcium, and fibrous cap (FC) features, were extracted from the baseline IVOCT images (before stent implantation, T0 and T1). All features were automatically computed using OCTOPUS. Lesion length was defined as the length of vessel segment where the stent was implanted. Lumen features included minimum/mean lumen area and lumen diameter; calcium features consisted of maximum/minimum calcium angle, calcium thickness, and calcium depth; and FC features comprised maximum/minimum FC angle, minimum FC thickness, maximum FC area, FC surface area, and FC burden. FC angle, FC thickness, and FC area were calculated from each IVOCT frame, whereas FC surface area and FC burden were calculated from the entire lesion. FC thickness was automatically calculated by identifying the luminal and abluminal boundaries based on the method developed by our group (42, 48). Briefly, the lumen boundary was obtained using the deep learning segmentation method (41). We used dynamic programming (42) to segment the abluminal boundary since it has a gradual transition of pixel intensity from bright to dark. FC surface area was defined as the amount of FC area covering the surface of the lumen. FC burden was computed as FC area divided by the surface area of the lumen. Table 1 shows the 17 IVOCT features analyzed as predictors of post-stent neoatherosclerosis.
Table 1. Intravascular optical coherence tomography (IVOCT) plaque features measured as potential predictors of post-stent neoatherosclerosis.
In this study, neoatherosclerosis was defined as the presence of accumulation of lipid-laden foamy macrophages with or without necrotic core in IVOCT images (17). Two experienced interventional cardiologists manually identified neoatherosclerosis. In case of disagreement between the two readers, they reevaluated the frames and reached a consensus decision.
We evaluated the value of atherosclerosis characteristics in pre-stent IVOCT images as predictors of post-stent neoatherosclerosis using various data science approaches. Statistical analyses were performed using R Studio (version 1.4.1717) software. All variables are presented as mean ± standard deviation. A student t-test was used for baseline comparisons of clinical and IVOCT plaque characteristics between neoatherosclerosis and no-neoatherosclerosis groups. To assess inter-correlations of IVOCT features, we performed a heatmap analysis using the non-parametric Spearman’s rank correlation coefficient and hierarchical clustering of the individual IVOCT plaque features using the squared Euclidean distance method. A heatmap analysis was very important to eliminate the collinearity of features for further regression. To examine the incremental value of IVOCT plaque features for predicting neoatherosclerosis, univariate and multivariate logistic regression analyses were performed with 95% confidence intervals (CI). Variables that were significant in the univariate analysis (p < 0.05) were included in further multivariate analyses. We created receiver operating characteristic (ROC) curves to analyze the ability of pre-stent image features to predict post-stent neoatherosclerosis. Results were evaluated with area under the curve (AUC). The optimal cutoff values for various parameters were determined using the maximum sum of sensitivity and specificity. A p-value <0.05 was considered statistically significant.
This study included 90 patients with multi-vessel disease who had undergone staged PCI with stent implantation. No patients were excluded on the basis of clinical characteristics or image processing results. The median age was 64.0±10.0 years, and 80.0% were men. Among the 90 patients, 55 (61.1%) had hypertension, 57 (63.3%) were current smokers, and 15 (16.7%) had diabetes mellitus. Mean total cholesterol and low-density lipoprotein cholesterol (LDL-C) decreased significantly from baseline to 3 months (p < 0.01), and the level of LDL-C was sustained at 18 months. High-density lipoprotein cholesterol did not change over time (p>0.05). Details of patient characteristics are provided elsewhere (52).
Intravascular optical coherence tomography plaque characteristics at baseline were compared for stents that developed neoatherosclerosis (n = 19) or did not develop neoatherosclerosis (n = 161) at follow-up (Table 2). Longer lesion length was associated with higher prevalence of neoatherosclerosis (35.2 mm vs. 28.1 mm, p < 0.05). In the stented segment, the neoatherosclerosis group had smaller mean lumen diameter (2.39 mm vs. 2.68 mm, p < 0.05) and larger maximum calcium angle (193.8° vs. 128.1°, p < 0.05) than the no-neoatherosclerosis group. Most FC features were significantly associated with neoatherosclerosis. Particularly, the maximum FC area, FC surface area, and FC burden showed the strongest correlations (p < 0.00001). Features related to lumen area and calcium depth were not significantly different between the two groups.
Table 2. Comparison of intravascular optical coherence tomography (IVOCT) plaque features between the neoatherosclerosis (n = 19) and no-neoatherosclerosis (n = 161) groups Student t-test was applied.
To reduce features that correlated with each other, we performed a correlation heatmap analysis. Figure 3 displays a correlation plot of the 17 IVOCT plaque features, with hierarchical clustering showing distinct clusters of feature variance. With hierarchical clustering, we identified six clusters of IVOCT plaque features that were highly correlated with each other (green areas in the heatmap) but showed minimum relevancy with other features (black areas in the heatmap). Using a Spearman correlation coefficient threshold of 0.9, we eliminated eight IVOCT features (e.g., lumen area, and calcium depth) from further analyses.
Figure 3. Heatmap of 17 intravascular optical coherence tomography (IVOCT) plaque features with hierarchical clustering. R2 values are plotted against each other and ordered to show the statistically different clusters (red boxes). The color key indicates the value of explained variances: R2 values<0.5 are black, whereas greater values are shown in green or red with increasing intensity. Six clusters (red boxes) identified by hierarchical clustering and the IVOCT plaque features with the smallest p-values are shown on the left. Using a Spearman correlation coefficient threshold of 0.9, a total of 8 features were excluded from further analysis.
We performed univariate and multivariate logistic regression analyses on the remaining 9 IVOCT plaque features to identify predictors of neoatherosclerosis development. Table 3 lists the IVOCT plaque features associated with neoatherosclerosis by univariate and multivariate logistic regression analyses, including the lesion length and specific lumen features, calcium features, and FC features. In univariate logistic regression analysis, maximum calcium angle, minimum calcium thickness, maximum FC angle, maximum FC area, FC surface area, and FC burden were independent predictors of neoatherosclerosis. However, lesion length and features related to the lumen were not significantly associated. In multivariate logistic regression analysis, only the FC surface area [odds ratio (OR) 1.38, 95% CI 1.05–1.80, p < 0.05] was strongly associated with neoatherosclerosis development (Table 3). In a ROC analysis, the AUC was 0.901 (95% CI 0.859–0.946, p < 0.05) for FC surface area (Figure 4). In the box plot analysis, FC surface area was significantly larger in the neoatherosclerosis group (p < 0.00001, Figure 5). Figure 6 shows 3D visualizations of FC surface area on the representative IVOCT pullbacks (neoatherosclerosis vs. no-neoatherosclerosis).
Table 3. Univariate/multivariate logistic regression analyses for predicting in-stent neoatherosclerosis.
Figure 4. Receiver operating characteristic (ROC) curve analysis of fibrous cap (FC) surface area for predicting in-stent neoatherosclerosis. Area under the curve (AUC) for FC surface area was 0.901 (95% CI 0.859–0.946, p < 0.05).
Figure 5. Box plot of the best predictive intravascular optical coherence tomography (IVOCT) plaque feature (FC surface area) showing that the FC surface area was significantly larger in neoatherosclerosis group than that in no-neoatherosclerosis group (p < 0.00001). Red indicates neoatherosclerosis and green indicates no-neoatherosclerosis.
Figure 6. Three-dimensional (3D) visualization of FC surface area on the representative IVOCT pullbacks. Panels are FC distributions of cases (top) with and (bottom) without future neoatherosclerosis. Neoatherosclerosis case had a lesion with a length of 53.4 mm and an FC surface area of 33.1 mm2 at baseline, and no-neoatherosclerosis case had a lesion with a length of 37 mm and an FC surface area of 0.73 mm2 at baseline. The red is lumen, and the green is FC.
To the best of our knowledge, this is the first study to examine pre-PCI IVOCT plaque characteristics as predictors of in-stent neoatherosclerosis. We built on our previous studies (38–49) to evaluate IVOCT-derived plaque features and their association with the development of neoatherosclerosis in follow-up IVOCT examinations. Considering the impact of neoatherosclerosis on patient outcomes, it is important to identify factors that may influence the PCI result. In this study, we automatically extracted multiple IVOCT plaque features prior to PCI, including lesion length, lumen, calcium, and FC features, using a dedicated software (OCTOPUS). Multivariate analysis identified only FC surface area as strongly associated with neoatherosclerosis. In a ROC analysis, the AUC of FC surface area for predicting neoatherosclerosis was 0.901 (95% CI 0.859–0.946, p < 0.05).
Optical Coherence TOmography PlaqUe and Stent-enabled automated extraction of IVOCT plaque features was done easily with a reasonable computational time (38). Briefly, the software includes several important functions such as lumen and plaque segmentation, identification of stent struts, and registration of pullbacks for sequential comparisons. The SegNet-based deep learning method (41) was used to segment the lumen boundary in IVOCT images. The calcified plaque was segmented using a two-step deep learning model (46) consisting of preprocessing, determining the major calcification lesions using a 3D convolutional neural network, and segmenting calcified plaques using SegNet. In the context of lipid plaque, we segmented FC by identifying abluminal boundary using dynamic programming (42, 48). Using OCTOPUS, it only took up to 4 min to process a single IVOCT pullback, with manual editing completed within a couple of minutes. Most manual editing was done to optimize the calcium border and lipid arc. The user specifications for OCTOPUS were created in collaboration with interventional cardiologists from the Cardiovascular Imaging Core Laboratory at University Hospitals Cleveland Medical Center, Cleveland, Ohio, a leading laboratory for IVOCT image analysis. OCTOPUS was trained on a large dataset including more than 20,000 clinical images from 150 patients. The software greatly reduces the effort needed to accurately label IVOCT images and is currently used for various offline clinical research purposes by interventional cardiologists. With faster implementation, OCTOPUS-enabled IVOCT data extraction is expected to provide important information for real-time treatment planning.
We observed a strong association between FC surface area and the occurrence of neoatherosclerosis. This finding underscores the importance of automated IVOCT image analysis which can provide quantitative surface area measurements as opposed to a few point estimates of fibrous cap thickness. Although a few studies have investigated the potential contributions of various clinical, stent, and plaque features to the development of neoatherosclerosis, precise understanding of the underlying mechanisms remains unknown. There are multiple potential factors associated with in-stent neoatherosclerosis, such as stent type/age (15, 17, 23, 27–32), clinical factors (24, 27, 33), plaque characteristics (11, 34–36), and stent characteristics (37). Pathological studies first demonstrated a potential association between the prevalence of neoatherosclerosis and stent type and stent age. Nakazawa et al. reported that the prevalence of neoatherosclerosis was significantly greater in lesions with drug-eluting stents than those with bare metal stents, while in-stent neoatherosclerosis occurred more commonly with drug-eluting stents (17). In addition to the stent type and stent age, several clinical characteristics have been related to neoatherosclerosis, such as chronic kidney disease (24, 27), diabetes mellitus (33), low-density lipoprotein cholesterol (27), and lack of treatment with angiotensin-converting enzyme inhibitors or angiotensin II receptor blockers (24). Plaque characteristics have also been implicated in the development of neoatherosclerosis. Studies have suggested that microvessels might be a trigger for neoatherosclerosis development due to a higher prevalence in late in-stent restenotic tissue (11, 35, 36). Neoatherosclerosis has been found to be related to the morphology of the vessel segment (36). Particularly, the presence of lipidous plaque in the stent edges was associated with the occurrence of neoatherosclerosis. Although IVOCT provides an opportunity to conduct comprehensive assessment of coronary plaque, our study is the first to quantitatively analyze plaque characteristics in IVOCT images and their association with outcomes (e.g., neoatherosclerosis).
The findings of the present study have potential clinical relevance. Current interpretation methods for IVOCT images, endorsed by European Society of Cardiology, American Heart Association, and American College of Cardiology, lack assessment of the potential drivers of in-stent neoatherosclerosis. Similar to our findings with IVOCT, intravascular assessment of coronary plaques has been associated with immediate stent deployment outcomes and future events (49, 53). A few studies have investigated the clinical significance of neoatherosclerosis detected by intravascular imaging as a predictor of long-term adverse outcomes in patients undergoing PCI (54, 55). The findings of our study provide insights into the anatomical and inflammatory mechanisms that may link FC surface area with the occurrence of neoatherosclerosis.
This study has some limitations. First, the sample size (N = 90) in this serial imaging study was somewhat limited. Therefore, results might be changed with a larger population size. Second, only lipidic neoatherosclerosis was included in this study. Third, it is possible that patient-level factors, such as overall risk of atherosclerosis, might influence the development of neoatherosclerosis after stent placement. Importantly, all patients in this study had multi-vessel disease. Fourth, there may be unmeasured pre-stent plaque characteristics (e.g., microchannel, macrophage infiltration, and cholesterol crystal) that might influence neoatherosclerosis.
The ability to predict the likelihood of developing neoatherosclerosis after PCI can be used by interventional cardiologists to improve treatment planning. Identification of baseline plaque characteristics suggestive of neoatherosclerosis may prompt optimization of treatment to avoid future adverse events such as restenosis (56). Such approaches may inform appropriate planning for optimal stent expansion (20, 57) or the use of new-generation drug-eluting stents (58). Further studies will be necessary to address the clinical utility of IVOCT predictors in improving treatment planning and patient outcomes.
Neoatherosclerosis can be predicted from pre-stent IVOCT images using quantitative IVOCT plaque characteristics, specifically FC surface area. Our findings highlight the potential clinical benefits of utilizing IVOCT imaging in the catheterization laboratory to inform treatment decision making and optimizing treatment.
The original contributions presented in this study are included in the article/supplementary material, further inquiries can be directed to the corresponding author.
The studies involving human participants were reviewed and approved by Institutional Review Board of University Hospitals Cleveland Medical Center (Cleveland, OH, USA). The patients/participants provided their written informed consent to participate in this study.
JL has contributed to the ideation, overview, experiment, and drafting of the manuscript. GP and IM contributed the formal analysis and data preparation. JK contributed to the experiment and drafting of the manuscript. VZ, LD, and SA-K contributed to the data analyses. AH contributed to the ideation. GG contributed to the data curation, review, and editing. DW contributed to the drafting of the manuscript, review, editing, supervision, and funding acquisition. All authors have read and agreed to the submitted version of the manuscript.
This project was supported by the National Heart, Lung, and Blood Institute through grants NIH R21 HL108263, NIH R01 HL114406, NIH R01 HL143484, and NIH R01 RES219220. This work was also supported by the American Heart Association Grant #20POST35210974/JL/2020. This research was conducted in space renovated using funds from an NIH construction grant (C06 RR12463) awarded to Case Western Reserve University.
The grants were obtained via collaboration between Case Western Reserve University and University Hospitals of Cleveland. This work made use of the High-Performance Computing Resource in the Core Facility for Advanced Research Computing at Case Western Reserve University. The veracity guarantor (JK) affirms to the best of his knowledge that all aspects of this manuscript are accurate.
The authors declare that the research was conducted in the absence of any commercial or financial relationships that could be construed as a potential conflict of interest.
All claims expressed in this article are solely those of the authors and do not necessarily represent those of their affiliated organizations, or those of the publisher, the editors and the reviewers. Any product that may be evaluated in this article, or claim that may be made by its manufacturer, is not guaranteed or endorsed by the publisher.
The content of this report is solely the responsibility of the authors and does not necessarily represent the official views of the National Institutes of Health.
1. Kim LK, Feldman DN, Swaminathan RV, Minutello RM, Chanin J, Yang DC, et al. Rate of percutaneous coronary intervention for the management of acute coronary syndromes and stable coronary artery disease in the United States (2007 to 2011). Am J Cardiol. (2014) 114:1003–10. doi: 10.1016/j.amjcard.2014.07.013
2. Tada T, Byrne RA, Simunovic I, King LA, Cassese S, Joner M, et al. Risk of stent thrombosis among bare-metal stents, first-generation drug-eluting stents, and second-generation drug-eluting stents: results from a registry of 18,334 patients. JACC Cardiovasc Interv. (2013) 6:1267–74. doi: 10.1016/j.jcin.2013.06.015
3. Cassese S, Byrne RA, Tada T, Pinieck S, Joner M, Ibrahim T, et al. Incidence and predictors of restenosis after coronary stenting in 10 004 patients with surveillance angiography. Heart. (2014) 100:153–9. doi: 10.1136/heartjnl-2013-304933
4. Wenaweser P, Daemen J, Zwahlen M, van Domburg R, Jüni P, Vaina S, et al. Incidence and correlates of drug-eluting stent thrombosis in routine clinical practice: 4-year results from a large 2-institutional cohort study. J Am Coll Cardiol. (2008) 52:1134–40. doi: 10.1016/j.jacc.2008.07.006
5. Natsuaki M, Morimoto T, Furukawa Y, Nakagawa Y, Kadota K, Yamaji K, et al. Late adverse events after implantation of sirolimus-eluting stent and bare-metal stent. Circ Cardiovasc Interv. (2014) 7:168–79. doi: 10.1161/CIRCINTERVENTIONS.113.000987
6. Yamaji K, Kimura T, Morimoto T, Nakagawa Y, Inoue K, Soga Y, et al. Very long-term (15 to 20 years) clinical and angiographic outcome after coronary bare metal stent implantation. Circ Cardiovasc Interv. (2010) 3:468–75. doi: 10.1161/CIRCINTERVENTIONS.110.958249
7. Doyle B, Rihal CS, O’Sullivan CJ, Lennon RJ, Wiste HJ, Bell M, et al. Outcomes of stent thrombosis and restenosis during extended follow-up of patients treated with bare-metal coronary stents. Circulation. (2007) 116:2391–8. doi: 10.1161/CIRCULATIONAHA.107.707331
8. Otsuka F, Byrne RA, Yahagi K, Mori H, Ladich E, Fowler DR, et al. Neoatherosclerosis: overview of histopathologic findings and implications for intravascular imaging assessment. Eur Heart J. (2015) 36:2147–59. doi: 10.1093/eurheartj/ehv205
9. Nusca A, Viscusi MM, Piccirillo F, De Filippis A, Nenna A, Spadaccio C, et al. In stent neo-atherosclerosis: pathophysiology, clinical implications, prevention, and therapeutic approaches. Life. (2022) 12:393. doi: 10.3390/life12030393
10. Joner M, Koppara T, Byrne RA, Castellanos MI, Lewerich J, Novotny J, et al. Neoatherosclerosis in patients with coronary stent thrombosis: findings from optical coherence tomography imaging (a report of the PRESTIGE consortium). JACC Cardiovasc Interv. (2018) 11:1340–50. doi: 10.1016/j.jcin.2018.02.029
11. Ino Y, Kubo T, Kitabata H, Ishibashi K, Tanimoto T, Matsuo Y, et al. Difference in neointimal appearance between early and late restenosis after sirolimus-eluting stent implantation assessed by optical coherence tomography. Coron Artery Dis. (2013) 24:95–101. doi: 10.1097/MCA.0b013e32835c872b
12. Park S-J, Kang S-J, Virmani R, Nakano M, Ueda Y. In-stent neoatherosclerosis: a final common pathway of late stent failure. J Am Coll Cardiol. (2012) 59:2051–7. doi: 10.1016/j.jacc.2011.10.909
13. Vergallo R, Yonetsu T, Uemura S, Park S-J, Lee S, Kato K, et al. Correlation between degree of neointimal hyperplasia and incidence and characteristics of neoatherosclerosis as assessed by optical coherence tomography. Am J Cardiol. (2013) 112:1315–21. doi: 10.1016/j.amjcard.2013.05.076
14. Habara M, Terashima M, Nasu K, Kaneda H, Yokota D, Ito T, et al. Morphological differences of tissue characteristics between early, late, and very late restenosis lesions after first generation drug-eluting stent implantation: an optical coherence tomography study. Eur Heart J Cardiovasc Imaging. (2013) 14:276–84. doi: 10.1093/ehjci/jes183
15. Otsuka F, Vorpahl M, Nakano M, Foerst J, Newell JB, Sakakura K, et al. Pathology of second-generation everolimus-eluting stents versus first-generation sirolimus- and paclitaxel-eluting stents in humans. Circulation. (2014) 129:211–23. doi: 10.1161/CIRCULATIONAHA.113.001790
16. Yamaji K, Inoue K, Nakahashi T, Noguchi M, Domei T, Hyodo M, et al. Bare metal stent thrombosis and in-stent neoatherosclerosis. Circ Cardiovasc Interv. (2012) 5:47–54. doi: 10.1161/CIRCINTERVENTIONS.111.964965
17. Nakazawa G, Otsuka F, Nakano M, Vorpahl M, Yazdani SK, Ladich E, et al. The pathology of neoatherosclerosis in human coronary implants bare-metal and drug-eluting stents. J Am Coll Cardiol. (2011) 57:1314–22. doi: 10.1016/j.jacc.2011.01.011
18. Neumann F-J, Sousa-Uva M, Ahlsson A, Alfonso F, Banning AP, Benedetto U, et al. 2018 ESC/EACTS Guidelines on myocardial revascularization. Eur Heart J. (2019) 40:87–165. doi: 10.1093/eurheartj/ehy394
19. Lawton JS, Tamis-Holland JE, Bangalore S, Bates ER, Beckie TM, Bischoff JM, et al. 2021 ACC/AHA/SCAI guideline for coronary artery revascularization: executive summary: a report of the American College of Cardiology/American Heart Association Joint Committee on Clinical Practice Guidelines. Circulation. (2022) 145:e4–17. doi: 10.1161/CIR.0000000000001039
20. Dallan LAP, Pereira GTR, Zimin V, Zago EI, Vergara-Martel A, Fares A, et al. Comparison of stent expansion using a volumetric versus the conventional method through optical coherence tomography in an all-comers population. Cardiovasc Revasc Med. (2021) 24:48–54. doi: 10.1016/j.carrev.2020.09.015
21. Zhang B-C, Karanasos A, Regar E. OCT demonstrating neoatherosclerosis as part of the continuous process of coronary artery disease. Herz. (2015) 40:845–54. doi: 10.1007/s00059-015-4343-y
22. Hong S-J, Lee S-Y, Hong M-K. Clinical implication of optical coherence tomography-based neoatherosclerosis. J Korean Med Sci. (2017) 32:1056. doi: 10.3346/jkms.2017.32.7.1056
23. Yonetsu T, Kim J-S, Kato K, Kim S-J, Xing L, Yeh RW, et al. Comparison of incidence and time course of neoatherosclerosis between bare metal stents and drug-eluting stents using optical coherence tomography. Am J Cardiol. (2012) 110:933–9. doi: 10.1016/j.amjcard.2012.05.027
24. Yonetsu T, Kato K, Kim S-J, Xing L, Jia H, McNulty I, et al. Predictors for neoatherosclerosis. Circ Cardiovasc Imaging. (2012) 5:660–6. doi: 10.1161/CIRCIMAGING.112.976167
25. Tearney GJ, Regar E, Akasaka T, Adriaenssens T, Barlis P, Bezerra HG, et al. Consensus standards for acquisition, measurement, and reporting of intravascular optical coherence tomography studies: a report from the international working group for intravascular optical coherence tomography standardization and validation. J Am Coll Cardiol. (2012) 59:1058–72. doi: 10.1016/j.jacc.2011.09.079
26. Prati F, Regar E, Mintz GS, Arbustini E, Di Mario C, Jang I-K, et al. Expert review document on methodology, terminology, and clinical applications of optical coherence tomography: physical principles, methodology of image acquisition, and clinical application for assessment of coronary arteries and atherosclerosis. Eur Heart J. (2010) 31:401–15. doi: 10.1093/eurheartj/ehp433
27. Lee S-Y, Hur S-H, Lee S-G, Kim S-W, Shin D-H, Kim J-S, et al. Optical coherence tomographic observation of in-stent neoatherosclerosis in lesions with more than 50% neointimal area stenosis after second-generation drug-eluting stent implantation. Circ Cardiovasc Imaging. (2015) 8:e001878. doi: 10.1161/CIRCINTERVENTIONS.114.001878
28. Kim J-S, Hong M-K, Shin D-H, Kim B-K, Ko Y-G, Choi D, et al. Quantitative and qualitative changes in DES-related neointimal tissue based on serial OCT. JACC Cardiovasc Imaging. (2012) 5:1147–55. doi: 10.1016/j.jcmg.2012.01.024
29. Kozuki A, Shinke T, Otake H, Shite J, Nakagawa M, Nagoshi R, et al. Temporal course of vessel healing and neoatherosclerosis after DES implantation. JACC Cardiovasc Imaging. (2013) 6:1121–3. doi: 10.1016/j.jcmg.2013.06.005
30. Kuramitsu S, Sonoda S, Yokoi H, Iwabuchi M, Nishizaki Y, Shinozaki T, et al. Long-term coronary arterial response to biodegradable polymer biolimus-eluting stents in comparison with durable polymer sirolimus-eluting stents and bare-metal stents: five-year follow-up optical coherence tomography study. Atherosclerosis. (2014) 237:23–9. doi: 10.1016/j.atherosclerosis.2014.08.031
31. Joner M, Nakazawa G, Finn AV, Quee SC, Coleman L, Acampado E, et al. Endothelial cell recovery between comparator polymer-based drug-eluting stents. J Am Coll Cardiol. (2008) 52:333–42. doi: 10.1016/j.jacc.2008.04.030
32. Kim J-S, Kim J-S, Shin D-H, Kim B-K, Ko Y-G, Choi D, et al. Optical coherence tomographic comparison of neointimal coverage between sirolimus- and resolute zotarolimus-eluting stents at 9 months after stent implantation. Int J Cardiovasc Imaging. (2012) 28:1281–7. doi: 10.1007/s10554-011-9943-x
33. Tian F, Chen Y, Liu H, Zhang T, Guo J, Jin Q. Assessment of characteristics of neointimal hyperplasia after drug-eluting stent implantation in patients with diabetes mellitus: an optical coherence tomography analysis. Cardiology. (2014) 128:34–40. doi: 10.1159/000357612
34. Virmani R, Kolodgie FD, Burke AP, Finn AV, Gold HK, Tulenko TN, et al. Atherosclerotic plaque progression and vulnerability to rupture: angiogenesis as a source of intraplaque hemorrhage. Arterioscler Thromb Vasc Biol. (2005) 25:2054–61. doi: 10.1161/01.ATV.0000178991.71605.18
35. Kim B-K, Kim J-S, Shin D-H, Ko Y-G, Choi D, Jang Y, et al. Optical coherence tomography evaluation of in-stent restenotic lesions with visible microvessels. J Invasive Cardiol. (2012) 24:116–20.
36. Tian J, Ren X, Uemura S, Dauerman H, Prasad A, Toma C, et al. Spatial heterogeneity of neoatherosclerosis and its relationship with neovascularization and adjacent plaque characteristics: optical coherence tomography study. Am Heart J. (2014) 167:884–92.e2. doi: 10.1016/j.ahj.2014.03.013
37. Kitabata H, Kubo T, Komukai K, Ishibashi K, Tanimoto T, Ino Y, et al. Effect of strut thickness on neointimal atherosclerotic change over an extended follow-up period (= 4 years) after bare-metal stent implantation: intracoronary optical coherence tomography examination. Am Heart J. (2012) 163:608–16. doi: 10.1016/j.ahj.2012.01.007
38. Lee J, Kim JN, Gharaibeh Y, Zimin VN, Dallan LAP, Pereira GTR, et al. OCTOPUS – optical coherence tomography plaque and stent analysis software. arXiv [Preprint]. (2022) doi: 10.48550/arXiv.2204.10212
39. Gharaibeh Y, Prabhu DS, Kolluru C, Lee J, Zimin V, Bezerra HG, et al. Coronary calcification segmentation in intravascular OCT images using deep learning: application to calcification scoring. JMI. (2019) 6:045002. doi: 10.1117/1.JMI.6.4.045002
40. Kolluru C, Prabhu D, Gharaibeh Y, Bezerra H, Guagliumi G, Wilson D. Deep neural networks for A-line-based plaque classification in coronary intravascular optical coherence tomography images. J Med Imaging. (2018) 5:44504. doi: 10.1117/1.JMI.5.4.044504
41. Lee J, Prabhu D, Kolluru C, Gharaibeh Y, Zimin VN, Bezerra HG, et al. Automated plaque characterization using deep learning on coronary intravascular optical coherence tomographic images. Biomed Opt Express. (2019) 10:6497–515. doi: 10.1364/BOE.10.006497
42. Wang Z, Chamie D, Bezerra HG, Yamamoto H, Kanovsky J, Wilson DL, et al. Volumetric quantification of fibrous caps using intravascular optical coherence tomography. Biomed Opt Express. (2012) 3:1413–26. doi: 10.1364/BOE.3.001413
43. Lee J, Prabhu D, Kolluru C, Gharaibeh Y, Zimin VN, Dallan LAP, et al. Fully automated plaque characterization in intravascular OCT images using hybrid convolutional and lumen morphology features. Sci Rep. (2020) 10:2596. doi: 10.1038/s41598-020-59315-6
44. Lu H, Lee J, Jakl M, Wang Z, Cervinka P, Bezerra HG, et al. Application and evaluation of highly automated software for comprehensive stent analysis in intravascular optical coherence tomography. Sci Rep. (2020) 10:2150. doi: 10.1038/s41598-020-59212-y
45. Lu H, Lee J, Ray S, Tanaka K, Bezerra HG, Rollins AM, et al. Automated stent coverage analysis in intravascular OCT (IVOCT) image volumes using a support vector machine and mesh growing. Biomed Opt Express. (2019) 10:2809–28. doi: 10.1364/BOE.10.002809
46. Lee J, Gharaibeh Y, Kolluru C, Zimin VN, Dallan LAP, Kim JN, et al. Segmentation of coronary calcified plaque in intravascular OCT images using a two-step deep learning approach. IEEE Access. (2020) 8:225581–93. doi: 10.1109/ACCESS.2020.3045285
47. Kolluru C, Lee J, Gharaibeh Y, Bezerra HG, Wilson DL. Learning with fewer images via image clustering: application to intravascular OCT image segmentation. IEEE Access. (2021) 9:37273–80. doi: 10.1109/ACCESS.2021.3058890
48. Lee J, Pereira GTR, Gharaibeh Y, Kolluru C, Zimin VN, Dallan LAP, et al. Automated analysis of fibrous cap in intravascular optical coherence tomography images of coronary arteries. arXiv [Preprint]. (2022) doi: 10.48550/arXiv.2204.10162
49. Gharaibeh Y, Lee J, Zimin VN, Kolluru C, Dallan LAP, Pereira GTR, et al. Prediction of stent under-expansion in calcified coronary arteries using machine-learning on intravascular optical coherence tomography. arXiv [Preprint]. (2022) doi: 10.48550/arXiv.2205.10354
50. Lee J, Kim J, Pereira GTR, Gharaibeh Y, Kolluru C, Zimin VN, et al. Automatic microchannel detection using deep learning in intravascular optical coherence tomography images. Proceedings of the Medical Imaging 2020: Biomedical Applications in Molecular, Structural, and Functional Imaging. San Diego, CA (2022).
51. Lee J, Kim JN, Gomez-Perez L, Gharaibeh Y, Motairek I, Pereira GTR, et al. Automated Segmentation of Microvessels in Intravascular OCT Images Using Deep Learning. Bioengineering. (2022) 9:648. doi: 10.3390/bioengineering9110648
52. Guagliumi G, Shimamura K, Sirbu V, Garbo R, Boccuzzi G, Vassileva A, et al. Temporal course of vascular healing and neoatherosclerosis after implantation of durable- or biodegradable-polymer drug-eluting stents. Eur Heart J. (2018) 39:2448–56. doi: 10.1093/eurheartj/ehy273
53. Nakamura D, Wijns W, Price MJ, Jones MR, Barbato E, Akasaka T, et al. New volumetric analysis method for stent expansion and its correlation with final fractional flow reserve and clinical outcome: an ILUMIEN I Substudy. JACC Cardiovasc Interv. (2018) 11:1467–78. doi: 10.1016/j.jcin.2018.06.049
54. Sumino Y, Yonetsu T, Ueno H, Nogami K, Misawa T, Hada M, et al. Clinical significance of neoatherosclerosis observed at very late phase between 3 and 7 years after coronary stent implantation. J Cardiol. (2021) 78:58–65. doi: 10.1016/j.jjcc.2021.01.005
55. Kuroda M, Otake H, Shinke T, Takaya T, Nakagawa M, Osue T, et al. The impact of in-stent neoatherosclerosis on long-term clinical outcomes: an observational study from the Kobe University Hospital optical coherence tomography registry. EuroIntervention. (2016) 12:e1366–74. doi: 10.4244/EIJY15M12_05
56. Nakamura D, Dohi T, Ishihara T, Kikuchi A, Mori N, Yokoi K, et al. Predictors and outcomes of neoatherosclerosis in patients with in-stent restenosis. EuroIntervention. (2021) 17:489–96. doi: 10.4244/EIJ-D-20-00539
57. Dallan LAP, Zimin VN, Lee J, Gharaibeh Y, Kim JN, Pereira GTR, et al. Assessment of post-dilatation strategies for optimal stent expansion in calcified coronary lesions: ex vivo analysis with optical coherence tomography. Cardiovasc Revasc Med. (2022) 43:62–70. doi: 10.1016/j.carrev.2022.05.004
58. Iglesias JF, Heg D, Roffi M, Tüller D, Noble S, Muller O, et al. Long-term effect of ultrathin-strut versus thin-strut drug-eluting stents in patients with small vessel coronary artery disease undergoing percutaneous coronary intervention: a subgroup analysis of the BIOSCIENCE randomized trial. Circ Cardiovasc Interv. (2019) 12:e008024. doi: 10.1161/CIRCINTERVENTIONS.119.008024
Keywords: intravascular optical coherence tomography, neoatherosclerosis, plaque characteristics, OCTOPUS, fibrous cap surface area
Citation: Lee J, Pereira GTR, Motairek I, Kim JN, Zimin VN, Dallan LAP, Hoori A, Al-Kindi S, Guagliumi G and Wilson DL (2022) Neoatherosclerosis prediction using plaque markers in intravascular optical coherence tomography images. Front. Cardiovasc. Med. 9:1079046. doi: 10.3389/fcvm.2022.1079046
Received: 24 October 2022; Accepted: 29 November 2022;
Published: 14 December 2022.
Edited by:
Ernst Wellnhofer, Charité–Universitätsmedizin Berlin, GermanyReviewed by:
Taku Asano, St. Luke’s International Hospital, JapanCopyright © 2022 Lee, Pereira, Motairek, Kim, Zimin, Dallan, Hoori, Al-Kindi, Guagliumi and Wilson. This is an open-access article distributed under the terms of the Creative Commons Attribution License (CC BY). The use, distribution or reproduction in other forums is permitted, provided the original author(s) and the copyright owner(s) are credited and that the original publication in this journal is cited, in accordance with accepted academic practice. No use, distribution or reproduction is permitted which does not comply with these terms.
*Correspondence: David L. Wilson, ZGx3QGNhc2UuZWR1
Disclaimer: All claims expressed in this article are solely those of the authors and do not necessarily represent those of their affiliated organizations, or those of the publisher, the editors and the reviewers. Any product that may be evaluated in this article or claim that may be made by its manufacturer is not guaranteed or endorsed by the publisher.
Research integrity at Frontiers
Learn more about the work of our research integrity team to safeguard the quality of each article we publish.