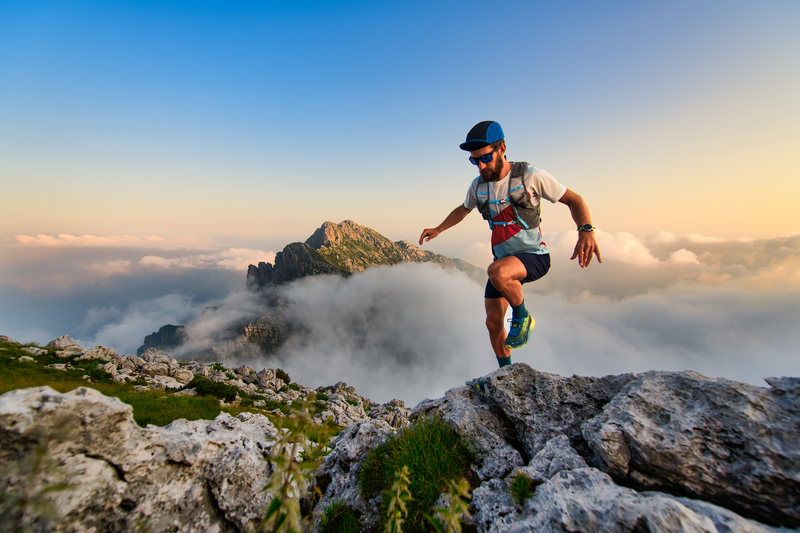
94% of researchers rate our articles as excellent or good
Learn more about the work of our research integrity team to safeguard the quality of each article we publish.
Find out more
ORIGINAL RESEARCH article
Front. Cardiovasc. Med. , 09 January 2023
Sec. Cardiovascular Epidemiology and Prevention
Volume 9 - 2022 | https://doi.org/10.3389/fcvm.2022.1072164
This article is part of the Research Topic Epidemiology and Clinical Researches in Atherosclerosis and Cardiovascular Disease View all 13 articles
Background: Interleukin (IL)-34 and IL-38 are associated with cardiovascular disease (CVD). However, their involvement in atrial fibrillation (AF) and AF-associated adverse events remains uncertain. Therefore, we aimed to investigate their association with various AF prognostic factors in a cohort study and assessed their predictive value for the prognosis of patients with AF.
Methods: Patients with new-onset non-valvular AF were consecutively enrolled between 2013 and 2015 at the Department of Cardiovascular Medicine of the Southwest Hospital of the Army Medical University (Third Military Medical University) in Chongqing, China. The endpoints included stroke and all-cause mortality. The baseline levels of plasma IL-34, IL-38, NT-proBNP, high-sensitivity cardiac troponin T (hs-cTnT), and GDF-15 were measured and their correlation with AF-related adverse events were analyzed in a Cox proportional-hazards regression model. The C-statistic, net reclassification improvement (NRI), and integrated discrimination improvement (IDI) were used to evaluate the performance of the AF prognostic models. Decision curve analysis (DCA) was used to evaluate the clinical net benefit of the original and modified models.
Results: A total of 299 patients with new-onset AF were enrolled. During the median follow-up time of 28 (IQR: 27, 29) months, the higher levels of IL-34 were associated with a lower risk of stroke, and the higher levels of IL-38 were associated with an increased risk of all-cause death (all adjusted P < 0.05). In addition, elevated hs-cTnT and NT-proBNP concentrations were associated with a higher risk of stroke and all-cause mortality (all adjusted P < 0.05). Furthermore, the CHA2DS2-VASc score combined with IL-38 and NT-proBNP significantly improved the C-statistic, IDI, and NRI (all P < 0.01). There was no statistically significant difference (all P > 0.05) in the discrimination power between the preference models and the ABC (age, biomarkers, and clinical history) score for the two prognostic outcomes.
Conclusion: Our results suggested that IL-34 and IL-38 were independently associated with stroke and all-cause mortality in patients with AF. Moreover, adding IL-38 and NT-proBNP to the CHA2DS2-VASc score significantly improved its predictive ability of AF-related all-cause death. Finally, the preference model performed equally well as the ABC score in predicting AF prognosis.
Atrial fibrillation (AF) is the most common cardiac arrhythmia which has become a major public health concern worldwide, and its prevalence is projected to increase further in the coming years (1). The onset of AF and its development is usually accompanied by many adverse events, which can lead to disability and mortality (2). Therefore, prediction and risk stratification of prognosis is particularly essential for better monitoring and management of AF. Risk stratification patterns recommended by current AF guidelines are mainly based on clinical risk factors, such as the CHA2DS2-VASc score (3). However, the identification power of CHA2DS2-VASc score is still limited in estimating the “truly high-risk” subjects, with a C-statistic of only 0.6 (4). Multiple studies have found biomarkers carrying rich prognostic information in AF (5–7). Hijazi et al. developed a new biomarker-based model in 2016, the ABC (age, biomarkers, and clinical history) score, which yielded a higher C-statistic than the CHA2DS2-VASc score (8).
Circulating interleukins (ILs) are a class of cytokines involved in information transmission, activation, and inflammatory responses, which have been intensively investigated in cardiovascular disease (CVD) over the last few years (9–11). Among them, IL-34 has been shown associated with CVD risk (12, 13), and IL-38 may be a key regulator of CVD (14). At present, relatively little is known about the role of IL-34 and IL-38 in AF-associated adverse events. In addition, the question remains to be answered whether IL-34 and IL-38 can improve the predictive power of the existing risk stratification scheme (CHA2DS2-VASc score) for AF-related prognosis.
Therefore, we aimed to explore the associations between ILs and stroke and all-cause death and to evaluate their predictive value in AF outcomes. Furthermore, we compared the prognostic predictive power of CHA2DS2-VASc score combined with IL-34 or IL-38 to that of the ABC score, to find new prognosis-associated biomarkers of AF and improve prognostic assessment of AF patients.
We conducted a cohort study. Patients aged greater than or equal to 18 years with new-onset AF were consecutively enrolled between December 2013 and August 2015 at the Department of Cardiovascular Medicine of the Southwest Hospital of the Army Medical University (Third Military Medical University) in Chongqing, China. The diagnostic criteria of AF were based on the definition by the 2020 ESC Guidelines (15). Patients were excluded if they met one of the following criteria: moderate-to-severe mitral stenosis, artificial valve replacement, malignant tumors, acute and chronic inflammatory diseases, connective tissue diseases, and/or infections. The study complied with the principles of the Declaration of Helsinki and was approved by the Ethics Committee of Southwest Hospital of the Army Medical University. Informed consents have been obtained from all subjects.
The CHA2DS2-VASc score was calculated using clinical information [heart failure, hypertension, diabetes, vascular disease, aged 65–74 years old, female accounted for one point. Age ≥ 75 years old, and stroke history/transient ischemic attack (TIA)/thromboembolism history accounted for two points] (3). The ABC-stroke score was calculated according to the study of Hijazi et al. [age, the levels of troponin (Tn) T/I, NT-proBNP, prior stroke/systemic embolism (SE), and 1-year stroke risk incidence rate of individual] (8). Also, the ABC-death score was calculated according to the study of Hijazi et al. (16) (age, the levels of Tn T/I, NT-proBNP, GDF-15, the history of heart failure, and 1-year mortality risk incidence rate of individual).
Fasting blood samples (5 ml) were drawn from all participants (within 48 h after admission and before any treatments). Plasma fractions were obtained immediately by centrifugation at 2,000 rpm/min for 15 min at 4°C and stored in a −80°C freezer until use.
NT-proBNP and high-sensitivity cardiac troponin T (hs-cTnT) levels were measured using an electro-chemiluminescence immunoassay (Cobas e601, Rocha Diagnostics, Manheim, Germany). The detection range of NT-proBNP and hs-cTnT were 5–35,000 pg/ml and 3–10,000 ng/L, respectively. GDF-15 levels were assessed by enzyme-linked immunosorbent assay (ELISA) kit (RayBiotech, Norcross, GA, USA), and the analytical range was 2–800 pg/ml, the inter-assay and intra-assay coefficient of variation were less than 10 and 12%, respectively. Measurements of IL-34 and IL-38 were performed with Bio-plex Pro™ plex xMAP array technology (Bio-Rad Corporation, Hercules, CA, USA) based on Luminex 200 system (Luminex Corporation, Austin, TX, USA), and the concentrations were calculated by fluorescence intensities from the corresponding standard curves, based on Bio-Plex Manager™ 6.1 (Bio-Rad) software. Each sample was tested once. The standard and reference products were used for quality control, all of which showed coefficients of variation of less than 12.1%.
The primary endpoint was stroke (ischemic and hemorrhagic) and the secondary endpoint was all-cause death. The follow-up time for each individual was recorded from the date of entry to the date of death, or the end of the trial. The patient’s physical conditions or causes of death were verified annually through electronic medical records, reports of close relatives, and national death registration system.
The Shapiro–Wilk test was used to test if continuous variables follow a normal distribution. Continuous variables were presented by mean ± SD or median with interquartile ranges (IQRs). Categorical variables were presented as numbers and percentages. The optimal cut-off values of biomarkers were determined by X-tile software (Yale University, New Haven, CT, USA) (17). To avoid multiple cut-off values in this study, we considered stroke and all-cause death as a composite endpoint.
To investigate the associations between biomarkers and AF prognosis, biomarkers were initially examined by univariate Cox proportional-hazard analysis. Then, the variables with P < 0.10 in univariate Cox proportional-hazard analysis were incorporated into the multivariate Cox proportional-hazard analysis by the forward and likelihood ratio (LR) method. In addition, we used the same method (forward and LR) for sensitivity analysis to verify the robustness of the above results. Sensitivity analysis was performed with adjustment for the AF-related covariates: warfarin, statins, and angiotensin receptor inhibitors (ARB) and the components of the CHA2DS2-VASc score. The variance inflation factor (VIF) was used to examine the multicollinearity of the variables.
The value of C-index (Harrell’s C) (18), integrated discrimination improvement (IDI), and net reclassification improvement (NRI) were calculated to evaluate the predictive power of the models in discrimination and reclassification (19). Decision curve analysis (DCA) as described by Vickers et al. (20) was used to quantify the clinical net benefit of each model and to visually compare models.
A two-sided p-value < 0.05 was considered statistically significant. All analyses were performed with SPSS statistical software version 25.0 (SPSS Inc., Chicago, IL, USA.) and R software version 4.1.2 (R Project for Statistical Computing, Vienna, Austria).
A total of 299 participants underwent this trial (Supplementary Figure 1). Among them, 132 (44.15%) were female, the median age was 66 years (IQR: 58, 73). 25 (8.36%) patients were lost to follow-up. The demographic and clinical characteristics of the participants at baseline were presented in Table 1. During the median follow-up time of 28 (IQR: 27, 29) months, we identified 26 strokes (4.12 per 100 person-years) and 30 deaths (4.48 per 100 person-years).
The optimal cut-off values of biomarkers were determined by the X-tile software based on follow-up time, composite endpoint event (stroke and all-cause death). Optimal cut-off values of IL-34, IL-38, NT-proBNP, hs-cTnT, and GDF-15 were 138.47 pg/ml, 58.25 pg/ml, 3,580.17 pg/ml, 11.00 ng/ml, and 1,813.62 pg/ml, respectively (Supplementary Table 1).
We incorporated variables with P < 0.10 in univariate Cox regression (Supplementary Table 2) into the multivariate Cox regression model. In addition, the treatments (warfarin, statins, and ARB) and the components of the CHA2DS2-VASc score were further adjusted in the sensitive analysis and no differences were found. The collinearity was ignored in this study as the VIFs of variables were less than 2.0 in all models. We found that IL-34 [hazard ratio (HR): 0.36, 95% confidence interval (CI): 0.17–0.78, P = 0.010] and hs-cTnT (HR: 3.09, 95% CI: 1.33−7.19, P = 0.009) were independently correlated with the risk of stroke. For all-cause mortality, patients with higher levels of IL-38 (HR: 3.11, 95% CI 1.16−8.29, P = 0.024) and NT-proBNP (HR: 2.77, 95% CI 1.13−6.78, P = 0.025) had an increased risk of all-cause death (Table 2). Although higher GDF-15 level was related to a higher risk of all-cause death in unadjusted analysis, we found that it was not an independent risk factor for AF-related prognosis in the adjusted model.
Table 2. Associations between biomarkers concentrations and events during follow-up in atrial fibrillation (AF) patients.
We assessed the predictive capabilities of the CHA2DS2-VASc model combined with biomarkers (Table 3). For all-cause death risk stratification, the CHA2DS2-VASc integrated IL-38 and NT-proBNP, achieved a C-statistic of 0.80 (95% CI 0.73−0.87), which had a significant improvement over the original model (C-statistic: 0.70, 95% CI 0.63−0.77, P = 0.005), and the reclassification ability was significantly better (IDI: 3.7%, 95% CI: 1.1−13.3%, P < 0.001; NRI: 77.6%, 95% CI: 21.9−82.7%, P < 0.001). On the other hand, the CHA2DS2-VASc score combined with IL-34 and hs-cTnT for stroke risk stratification achieved highest C-statistic of 0.75 (95% CI 0.66−0.83), which did not show a significant statistical difference from other scores. The DCA visualized the clinical net benefit of the original and modified CHA2DS2-VASc score (Figure 1). For predicting stroke, the clinical net benefit of modified CHA2DS2-VASc score adding IL-34 and hs-cTnT was slightly better than other scores (Figure 1A). For predicting all-cause death, adding IL-38 and NT-proBNP to CHA2DS2-VASc had the best clinical net benefit when the threshold probability was between 9 and 35% (Figure 1B).
Table 3. Discrimination and reclassification for stroke, all-cause mortality, or cardiovascular death.
Figure 1. Decision curve analysis (DCA) of the original and modified CHA2DS2-VASc score. (A) Stroke model added hs-cTnT and/or IL-34; (B) all-cause mortality model added IL-38 and/or NT-proBNP. The black lines (original scores) and other lines (modified scores) represent the net benefit of each model graphically. The horizontal axis represents the threshold probability of the target adverse event risk. The longitudinal axis shows the net benefit. ILs, interleukins; NT-proBNP, N-terminal fragment B-type natriuretic peptide; hs-cTnT, high-sensitivity cardiac troponin.
In this study, we first explored the association of IL-34 and IL-38 with stroke and all-cause mortality in patients with new-onset AF and found that low levels of IL-34 were independent predictors of stroke, while high concentrations of IL-38 were correlated with all-cause death, which goes beyond earlier findings. In addition, we evaluated whether the addition of IL-34 and IL-38 could improve the predictive performance of the existing prognostic prediction model (CHA2DS2-VASc score). We showed significant improvement for the all-cause death risk prediction when IL-38 and NT-proBNP were added to the original model (CHA2DS2-VASc score) Finally, the preferred models in two adverse events predictive models performed equally well as the ABC score.
The pathophysiology of AF is complex and yet to be explored. Several studies have depicted correlations between AF and different inflammatory markers and mediators (21, 22). Tn elevation was initially seen as a sensitive indicator of myocardial damage and infarction. Recent data has shown that cardiac troponin I (cTnI) and T (cTnT) provide important prognostic information in anticoagulated patients with AF for predicting all-cause mortality, cardiac death, stroke or SE (23), which was consistent with our findings that hs-cTnT was independently related to stroke. NT-proBNP, which is degraded by pro-BNP, has been used as a biomarker for heart failure and renal insufficiency. In recent years, its prognostic values have also been applied to AF. The concentrations of NT-proBNP increased during states of hemodynamic stress such as in heart failure, acute coronary syndrome, and arrhythmias including AF (22). In a sub-study of RE-LY, increased concentrations of NT-proBNP were generally associated with the risk of stroke and mortality (24). The same conclusion was obtained in our study. GDF-15 is a member of the transforming growth factor-β (TGF-β) superfamily, which plays an important role as an inflammatory marker in tumor pathogenesis, and ischemic, and metabolic diseases. In addition, it has been widely studied in the field of CVDs. It is not expressed in healthy adult myocardium but significantly expressed in cardiomyocytes, adipocytes, macrophages, endothelial cells, and vascular smooth muscle cells after myocardial lesion (25). In our study, GDF-15 was a risk predictor for mortality on top of clinical characteristics. However, NT-proBNP, hs-TnT, and GDF-15 partly reflected the same processes, which was myocardial dysfunction and cardiovascular comorbidity (7). This might explain why in the adjusted model GDF-15 did not appear to be an independent risk indicator for all-cause death when other biomarkers and clinical characteristics have been taken into account.
Interleukin (IL)-34 as a novel ligand of CSF-1R was defined in 2008 (26), and its biology and function have been broadly studied. In physiologic cases, they perform critical roles in the development of microglia and Langerhans cells (LCs). They also play crucial roles in pathological conditions, such as inflammatory diseases. Over the past few years, its role in CVD has also been investigated and found to be significantly increased in patients with coronary heart disease (CHD), and its increase is positively correlated with the level of high-sensitivity C-reactive protein (hs-CRP), the results of which give evidence for IL-34 as a pro-inflammatory cytokine (27). Moreover, studies have shown that IL-34 may be an important predictor of CVD, heart failure hospitalization, and all-cause mortality in patients with chronic heart failure (CHF) (28). Concurrently, we found that it can be used as an independent predictor for stroke events in AF patients, which may provide a new insight connecting AF to stroke outcomes beyond other biomarkers. However, it was noteworthy that AF patients with lower IL-34 levels have a higher risk of stroke, which seemed counterintuitive to its pro-inflammatory role. On the one hand, the levels of IL-34 were measured only once at the baseline, failing to evaluate the impact of dynamic changes of them on the prognosis. Therefore, the effects of IL-34 level fluctuation on the outcomes of AF need to be further explored in the future. On the other hand, some previous studies also reported that elevated IL-34 may act as a protective factor. Esaki et al. (29) identified decreased expression of IL-34 in atopic dermatitis (AD) compared to non-lesional AD and normal epidermis. The study by Mizuno et al. (30) demonstrated that in vitro, microglia treated with IL-34 attenuated the neurotoxic effects of oligomeric amyloid-β (oAβ), which mediates synaptic dysfunction and neuronal damage in Alzheimer’s disease. Moreover, intracerebroventricular administration of IL-34 improves deficits in associative learning. In addition, IL-34 also has a protective role in some cancer, such as non-small cell lung cancer (31), colorectal cancer (32), breast cancer (33, 34), and lung cancer (32), hematologic malignancies (32), and head and neck cancer (33, 34). Therefore, the issue of whether IL-34 is beneficial or harmful in stroke-attacked AF patients merits further study.
Interleukin (IL)-38 (IL-1F10 or IL-1HY2) belongs to IL-36, one of the family members of IL-1. Its primary biological function is to block the activation of the IL-36R signaling pathway and influence the proinflammation function of IL-36, which is similar to IL-36Ra (35). IL-38 is expressed in the thymus, heart, placenta, and fetal liver in healthy conditions (36), whereas in disease it is predominantly expressed in settings in IL-1-driven inflammatory response, such as CVD (37). Previous study indicated that the expression of the IL-38 gene was increased in peripheral blood mononuclear cells (PBMCs) of patients with ST-segment elevation myocardial infarction (STEMI) and had been positively correlated with CRP, cTnI, and NT-proBNP (37).
The CHA2DS2-VASc score, the main method recommended by current guidelines for predicting stroke outcomes in AF, is derived from the CHADS2 score [congestive heart failure, hypertension, age ≥ 75 years, diabetes mellitus, previous stroke (double weight)], and it is better at identifying “truly low risk” (CHA2DS2-VASc score = 0–1) than the CHADS2 score (38). However, as research continues to unfold, it has been found that there was still room for improvement in terms of predicting the “truly high-risk” patients since the C-statistic of the CHA2DS2-VASc score was only 0.60 (95% CI 0.57−0.64) (3). The discovery that biomarkers provide rich information for AF prognosis has led to new strategies to improve prognosis stratification. Hijazi et al. developed an ABC score based on age, biomarkers and clinical history, which had a C-statistic of 0.65 (95% CI: 0.61−0.69) and were well-validated both internally and externally (8). In our findings, the C-statistic of stroke prediction model integrated IL-34 and/or hs-cTnT were all superior to the original model, and NRI of the CHA2DS2-VASc score combined with hs-cTnT had a significantly improved. Furthermore, when NT-proBNP was added to the CHA2DS2-VASc score for predicting all-cause mortality, the C-statistic was significantly improved in the new model. In addition, when IL-38 and NT-proBNP were simultaneously added to the CHA2DS2-VASc score, the C-statistic increased, and NRI, and IDI both showed significant improvements of the new model regarding prognostic prediction value. Finally, we also compared the new model with the largest C-statistic to the ABC score and found that they have comparable discrimination capacity, which indicated that our improvements to the current clinical model were acceptable.
There were some limitations of our study. First, we selected participants from a single center which lacks external validity. Therefore, large-scale prospective validation is required in multicenter studies. Second, our follow-up time was relatively short, and the observation of end-point events was insufficient, so it is still necessary to extend the follow-up time to obtain more accurate results. Finally, further studies are needed on the exact biological mechanism of IL-34 and IL-38 in the pathology of AF.
In conclusion, serum IL-34 and IL-38 may serve as biomarkers of prognostic evaluation for stroke and all-cause mortality in patients with AF. The addition of IL-38 improved the predictive power of the existing model (CHA2DS2-VASc score) and increases the net clinical benefit. The new model showed comparable prognostic value to the ABC score. Our findings may help with clinical management and prognostic prediction of patients with AF.
The original contributions presented in this study are included in the article/Supplementary material, further inquiries can be directed to the corresponding authors.
The studies involving human participants were reviewed and approved by the Army Medical University. The patients/participants provided their written informed consent to participate in this study.
LZ, ZZ, and YL designed this study. JM, NW, and ZY drafted the article and analyzed the data. YC and CL were in charge of data collection. WX did the critical revision of article. All authors have read and approved the final manuscript.
This work was supported by the National Natural Science Foundation of China (No. 82073649 to NW).
The authors declare that the research was conducted in the absence of any commercial or financial relationships that could be construed as a potential conflict of interest.
All claims expressed in this article are solely those of the authors and do not necessarily represent those of their affiliated organizations, or those of the publisher, the editors and the reviewers. Any product that may be evaluated in this article, or claim that may be made by its manufacturer, is not guaranteed or endorsed by the publisher.
The Supplementary Material for this article can be found online at: https://www.frontiersin.org/articles/10.3389/fcvm.2022.1072164/full#supplementary-material
1. Du X, Guo L, Xia S, Du J, Anderson C, Arima H, et al. Atrial fibrillation prevalence, awareness and management in a nationwide survey of adults in China. Heart. (2021). doi: 10.1136/heartjnl-2020-317915 [Epub ahead of print].
2. Benjamin E, Wolf P, D’Agostino R, Silbershatz H, Kannel W, Levy D. Impact of atrial fibrillation on the risk of death: the framingham heart study. Circulation. (1998) 98:946–52. doi: 10.1161/01.cir.98.10.946
3. January C, Wann L, Calkins H, Chen L, Cigarroa J, Cleveland J, et al. 2019 AHA/ACC/HRS focused update of the 2014 AHA/ACC/HRS guideline for the management of patients with atrial fibrillation: a report of the American college of cardiology/American heart association task force on clinical practice guidelines and the heart rhythm society in collaboration with the society of thoracic surgeons. Circulation. (2019) 140:e125–51. doi: 10.1161/CIR.0000000000000665
4. January C, Wann L, Alpert J, Calkins H, Cigarroa J, Cleveland J, et al. 2014 AHA/ACC/HRS guideline for the management of patients with atrial fibrillation: a report of the American college of cardiology/American heart association task force on practice guidelines and the heart rhythm society. J Am Coll Cardiol. (2014) 64:e1–76. doi: 10.1016/j.jacc.2014.03.022
5. Hijazi Z, Siegbahn A, Andersson U, Granger C, Alexander J, Atar D, et al. High-sensitivity troponin I for risk assessment in patients with atrial fibrillation: insights from the apixaban for reduction in stroke and other thromboembolic events in atrial fibrillation (ARISTOTLE) trial. Circulation. (2014) 129:625–34. doi: 10.1161/CIRCULATIONAHA.113.006286
6. Hijazi Z, Wallentin L, Siegbahn A, Andersson U, Alexander J, Atar D, et al. High-sensitivity troponin T and risk stratification in patients with atrial fibrillation during treatment with apixaban or warfarin. J Am Coll Cardiol. (2014) 63:52–61. doi: 10.1016/j.jacc.2013.07.093
7. Wallentin L, Hijazi Z, Andersson U, Alexander J, De Caterina R, Hanna M, et al. Growth differentiation factor 15, a marker of oxidative stress and inflammation, for risk assessment in patients with atrial fibrillation: insights from the apixaban for reduction in stroke and other thromboembolic events in atrial fibrillation (ARISTOTLE) trial. Circulation. (2014) 130:1847–58. doi: 10.1161/CIRCULATIONAHA.114.011204
8. Oldgren J, Hijazi Z, Lindbäck J, Alexander J, Connolly S, Eikelboom J, et al. Performance and validation of a novel biomarker-based stroke risk score for atrial fibrillation. Circulation. (2016) 134:1697–707.
9. Yuan S, Lin A, He Q, Burgess S, Larsson S. Circulating interleukins in relation to coronary artery disease, atrial fibrillation and ischemic stroke and its subtypes: a two-sample mendelian randomization study. Int J Cardiol. (2020) 313:99–104. doi: 10.1016/j.ijcard.2020.03.053
10. Guo Y, Lip G, Apostolakis S. Inflammation in atrial fibrillation. J Am Coll Cardiol. (2012) 60:2263–70. doi: 10.1016/j.jacc.2012.04.063
11. Smith C, Hulme S, Vail A, Heal C, Parry-Jones A, Scarth S, et al. SCIL-STROKE (subcutaneous interleukin-1 receptor antagonist in ischemic stroke): a randomized controlled phase 2 trial. Stroke. (2018) 49:1210–6. doi: 10.1161/STROKEAHA.118.020750
12. Fan Q, Yan X, Zhang H, Lu L, Zhang Q, Wang F, et al. IL-34 is associated with the presence and severity of renal dysfunction and coronary artery disease in patients with heart failure. Sci Rep. (2016) 6:39324. doi: 10.1038/srep39324
13. Li Z, Jin D, Wu Y, Zhang K, Hu P, Cao X, et al. Increased serum interleukin-34 in patients with coronary artery disease. J Int Med Res. (2012) 40:1866–70.
14. Pfeiler S, Winkels H, Kelm M, Gerdes N. IL-1 family cytokines in cardiovascular disease. Cytokine. (2019) 122:154215. doi: 10.1016/j.cyto.2017.11.009
15. Hindricks G, Potpara T, Dagres N, Arbelo E, Bax J, Blomström-Lundqvist C, et al. 2020 ESC guidelines for the diagnosis and management of atrial fibrillation developed in collaboration with the European association for cardio-thoracic surgery (EACTS): the task force for the diagnosis and management of atrial fibrillation of the European society of cardiology (ESC) developed with the special contribution of the european heart rhythm association (EHRA) of the ESC. Eur Heart J. (2021) 42:373–498. doi: 10.1093/eurheartj/ehaa612
16. Hijazi Z, Oldgren J, Lindbäck J, Alexander J, Connolly S, Eikelboom J, et al. A biomarker-based risk score to predict death in patients with atrial fibrillation: the ABC (age, biomarkers, clinical history) death risk score. Eur Heart J. (2018) 39:477–85. doi: 10.1093/eurheartj/ehx584
17. Camp R, Dolled-Filhart M, Rimm DL. X-tile: a new bio-informatics tool for biomarker assessment and outcome-based cut-point optimization. Clin Cancer Res. (2004) 10:7252–9. doi: 10.1158/1078-0432.CCR-04-0713
18. Harrell F, Lee K, Mark D. Multivariable prognostic models: issues in developing models, evaluating assumptions and adequacy, and measuring and reducing errors. Stat Med. (1996) 15:361–87. doi: 10.1002/(SICI)1097-0258(19960229)15:4<361::AID-SIM168>3.0.CO;2-4
19. Pencina M, D’Agostino R, D’Agostino R, Vasan R. Evaluating the added predictive ability of a new marker: from area under the ROC curve to reclassification and beyond. Stat Med. (2008) 27:157–72.
20. Vickers AJ, Cronin AM, Elkin EB, Gonen M. Extensions to decision curve analysis, a novel method for evaluating diagnostic tests, prediction models and molecular markers. BMC Med Inform Decis Mak. (2008) 8:53. doi: 10.1186/1472-6947-8-53
21. Patel P, Dokainish H, Tsai P, Lakkis N. Update on the association of inflammation and atrial fibrillation. J Cardiovasc Electrophysiol. (2010) 21:1064–70. doi: 10.1111/j.1540-8167.2010.01774.x
22. Hijazi Z, Oldgren J, Siegbahn A, Wallentin L. Application of biomarkers for risk stratification in patients with atrial fibrillation. Clin Chem. (2017) 63:152–64. doi: 10.1373/clinchem.2016.255182
23. Hijazi Z, Siegbahn A, Andersson U, Lindahl B, Granger C, Alexander J, et al. Comparison of cardiac troponins I and T measured with high-sensitivity methods for evaluation of prognosis in atrial fibrillation: an ARISTOTLE substudy. Clin Chem. (2015) 61:368–78. doi: 10.1373/clinchem.2014.226936
24. Hijazi Z, Oldgren J, Andersson U, Connolly S, Ezekowitz M, Hohnloser S, et al. Cardiac biomarkers are associated with an increased risk of stroke and death in patients with atrial fibrillation: a randomized evaluation of long-term anticoagulation therapy (RE-LY) substudy. Circulation. (2012) 125:1605–16. doi: 10.1161/CIRCULATIONAHA.111.038729
25. Wischhusen J, Melero I, Fridman W. Growth/Differentiation factor-15 (GDF-15): from biomarker to novel targetable immune checkpoint. Front Immunol. (2020) 11:951. doi: 10.3389/fimmu.2020.00951
26. Lin H, Lee E, Hestir K, Leo C, Huang M, Bosch E, et al. Discovery of a cytokine and its receptor by functional screening of the extracellular proteome. Science. (2008) 320:807–11. doi: 10.1126/science.1154370
27. Xi R, Fan Q, Yan X, Zhang H, Xie H, Gu G, et al. Increased serum interleukin-34 levels are related to the presence and severity of cardiac dysfunction in patients with ischemic cardiomyopathy. Front Physiol. (2018) 9:904. doi: 10.3389/fphys.2018.00904
28. Tao R, Fan Q, Zhang H, Xie H, Lu L, Gu G, et al. Prognostic significance of interleukin-34 (IL-34) in patients with chronic heart failure with or without renal insufficiency. J Am Heart Assoc. (2017) 6:e004911. doi: 10.1161/JAHA.116.004911
29. Esaki H, Ewald D, Ungar B, Rozenblit M, Zheng X, Xu H, et al. Identification of novel immune and barrier genes in atopic dermatitis by means of laser capture microdissection. J Allergy Clin Immunol. (2015) 135:153–63. doi: 10.1016/j.jaci.2014.10.037
30. Mizuno T, Doi Y, Mizoguchi H, Jin S, Noda M, Sonobe Y, et al. Interleukin-34 selectively enhances the neuroprotective effects of microglia to attenuate oligomeric amyloid-β neurotoxicity. Am J Pathol. (2011) 179:2016–27. doi: 10.1016/j.ajpath.2011.06.011
31. Lee E, Son D, Kim S, Lee J, Jo J, Han J, et al. Prediction of recurrence-free survival in postoperative non-small cell lung cancer patients by using an integrated model of clinical information and gene expression. Clin Cancer Res. (2008) 14:7397–404. doi: 10.1158/1078-0432.CCR-07-4937
32. Wang B, Xu W, Tan M, Xiao Y, Yang H, Xia T. Integrative genomic analyses of a novel cytokine, interleukin-34 and its potential role in cancer prediction. Int J Mol Med. (2015) 35:92–102. doi: 10.3892/ijmm.2014.2001
33. Mizuno H, Kitada K, Nakai K, Sarai A. PrognoScan: a new database for meta-analysis of the prognostic value of genes. BMC Med Genom. (2009) 2:18. doi: 10.1186/1755-8794-2-18
34. Uhlen M, Zhang C, Lee S, Sjöstedt E, Fagerberg L, Bidkhori G, et al. A pathology atlas of the human cancer transcriptome. Science. (2017) 357:eaan2507. doi: 10.1126/science.aan2507
35. van de Veerdonk F, Stoeckman A, Wu G, Boeckermann A, Azam T, Netea M, et al. IL-38 binds to the IL-36 receptor and has biological effects on immune cells similar to IL-36 receptor antagonist. Proc Natl Acad Sci USA. (2012) 109:3001–5. doi: 10.1073/pnas.1121534109
36. Bensen J, Dawson P, Mychaleckyj J, Bowden D. Identification of a novel human cytokine gene in the interleukin gene cluster on chromosome 2q12-14. J Int Cytokine Res. (2001) 21:899–904. doi: 10.1089/107999001753289505
37. Zhong Y, Yu K, Wang X, Wang X, Ji Q, Zeng Q. Elevated plasma IL-38 concentrations in patients with acute ST-Segment elevation myocardial infarction and their dynamics after reperfusion treatment. Med Inflamm. (2015) 2015:490120. doi: 10.1155/2015/490120
Keywords: interleukins, prognosis, CHA2DS2-VASc score, ABC score, atrial fibrillation
Citation: Ma J, Wu N, Yuan Z, Chen Y, Li C, Xie W, Zhang Z, Li Y and Zhong L (2023) Prognostic value of interleukin-34 and interleukin-38 in patients with newly diagnosed atrial fibrillation. Front. Cardiovasc. Med. 9:1072164. doi: 10.3389/fcvm.2022.1072164
Received: 17 October 2022; Accepted: 22 December 2022;
Published: 09 January 2023.
Edited by:
Wuxiang Xie, Clinical Research Institute, Peking University, ChinaReviewed by:
Yi-Gang Li, Shanghai Jiao Tong University, ChinaCopyright © 2023 Ma, Wu, Yuan, Chen, Li, Xie, Zhang, Li and Zhong. This is an open-access article distributed under the terms of the Creative Commons Attribution License (CC BY). The use, distribution or reproduction in other forums is permitted, provided the original author(s) and the copyright owner(s) are credited and that the original publication in this journal is cited, in accordance with accepted academic practice. No use, distribution or reproduction is permitted which does not comply with these terms.
*Correspondence: Li Zhong, emhvbmdsaTI4QGhvdG1haWwuY29t; Zhihui Zhang,
eHl6cGpAdG1tdS5lZHUuY24=; Yafei Li,
bGl5YWZlaTIwMDhAaG90bWFpbC5jb20=
†These authors have contributed equally to this work
Disclaimer: All claims expressed in this article are solely those of the authors and do not necessarily represent those of their affiliated organizations, or those of the publisher, the editors and the reviewers. Any product that may be evaluated in this article or claim that may be made by its manufacturer is not guaranteed or endorsed by the publisher.
Research integrity at Frontiers
Learn more about the work of our research integrity team to safeguard the quality of each article we publish.