- 1Department of Cardiology, The Second People’s Hospital of Hefei, Hefei Hospital Affiliated to Anhui Medical University, Hefei, Anhui, China
- 2Department of Cardiology, Hefei Second People’s Hospital Affiliated to Bengbu Medical College, Hefei, Anhui, China
Background: Emergency percutaneous coronary intervention (PCI) in patients with acute ST-segment elevation myocardial infarction (STEMI) helps to reduce the occurrence of major adverse cardiovascular events (MACEs) such as death, cardiogenic shock, and malignant arrhythmia, but in-hospital MACEs may still occur after emergency PCI, and their mortality is significantly increased once they occur. The aim of this study was to investigate the risk factors associated with MACE during hospitalization after PCI in STEMI patients, construct a nomogram prediction model and evaluate its effectiveness.
Methods: A retrospective analysis of 466 STEMI patients admitted to our hospital from January 2018 to June 2022. According to the occurrence of MACE during hospitalization, they were divided into MACE group (n = 127) and non-MACE group (n = 339), and the clinical data of the two groups were compared; least absolute shrinkage and selection operator (LASSO) regression was used to screen out the predictors with non-zero coefficients, and multivariate Logistic regression was used to analyze STEMI Independent risk factors for in-hospital MACE in patients after emergency PCI; a nomogram model for predicting the risk of in-hospital MACE in STEMI patients after PCI was constructed based on predictive factors, and the C-index was used to evaluate the predictive performance of the prediction model; the Bootstrap method was used to repeat sampling 1,000 Internal validation was carried out for the second time, the Hosmer-Lemeshow test was used to evaluate the model fit, and the calibration curve was drawn to evaluate the calibration degree of the model. Receiver operating characteristic (ROC) curves were drawn to evaluate the efficacy of the nomogram model and thrombolysis in myocardial infarction (TIMI) score in predicting in-hospital MACE in STEMI patients after acute PCI.
Results: The results of LASSO regression showed that systolic blood pressure, diastolic blood pressure, Killip grade II-IV, urea nitrogen and left ventricular ejection fraction (LVEF), IABP, NT-ProBNP were important predictors with non-zero coefficients, and multivariate logistic regression analysis was performed to analyze that Killip grade II-IV, urea nitrogen, LVEF, and NT-ProBNP were independent factors for in-hospital MACE after PCI in STEMI patients; a nomogram model for predicting the risk of in-hospital MACE after PCI in STEMI patients was constructed with the above independent predictors, with a C-index of 0.826 (95% CI: 0.785–0.868) having a good predictive power; the results of H-L goodness of fit test showed χ2 = 1.3328, P = 0.25, the model calibration curve was close to the ideal model, and the internal validation C-index was 0.818; clinical decision analysis also showed that the nomogram model had a good clinical efficacy, especially when the threshold probability was 0.1–0.99, the nomogram model could bring clinical net benefits to patients. The nomogram model predicted a greater AUC (0.826) than the TIMI score (0.696) for in-hospital MACE after PCI in STEMI patients.
Conclusion: Urea nitrogen, Killip class II-IV, LVEF, and NT-ProBNP are independent factors for in-hospital MACE after PCI in STEMI patients, and nomogram models constructed based on the above factors have high predictive efficacy and feasibility.
1 Introduction
In recent years, the incidence of acute ST-segment elevation myocardial infarction (STEMI) has been increasing, and it has become one of the most common and fatal cardiac emergencies in clinical practice (1). Percutaneous coronary intervention (PCI) is currently one of the most effective treatments for STEMI (2). Although PCI can timely open the infarcted vessel and achieve reperfusion, reperfusion itself aggravates myocardial injury and increases the incidence of major adverse cardiovascular events (MACEs), so it is particularly important to identify high-risk patients with STEMI who have a poor prognosis early in admission (3). Timely risk assessment of STEMI patients has a positive effect on improving patient outcomes. Establishing a convenient and effective prediction model is helpful to assess the risk of in-hospital MACE after emergency PCI in STEMI patients and has a positive effect on the early identification of patients at high risk of in-hospital MACE and timely intervention.
The nomogram is based on the analysis results of COX proportional hazards or logistic regression model, which is graphical and visualized for the prediction of individual disease risk and is more intuitive and easy to be popularized and applied in clinical practice. Compared with traditional risk scoring systems, nomogram models integrate more risk factors and obtain numerical probabilities of target events, more accurately quantify risk, and are more flexible to apply. Its application has been reported in predicting the risk of postoperative heart failure in patients with acute myocardial infarction (AMI) (4), the risk of in-hospital major cardiovascular events in patients with AMI after PCI (5), and the prognosis of patients with the acute coronary syndrome (6). In this study, we retrospectively analyzed the clinical characteristics of 466 STEMI patients before emergency PCI, and provided a reference for clinical assessment of the patient’s condition and guiding treatment by constructing a nomogram model to predict the risk of in-hospital MACE in STEMI patients after emergency PCI.
2 Materials and methods
2.1 Study population
A retrospective analysis of 466 STEMI patients who underwent emergency PCI at the Second People’s Hospital of Hefei from January 2018 to June 2022 was performed as the study subjects, all of whom were stented patients. Inclusion criteria (1) aged 18 years or older; (2) no previous history of atrial fibrillation; (3) admitted for emergency PCI within 24 h after onset; (4) demographic characteristics and complete clinical data. Exclusion criteria: (1) combined with malignant tumor; (2) accompanied by non-obstructive coronary heart disease, primary cardiomyopathy; (3) clinical evidence of infection; (4) accompanied by immune system disease; (5) combined with severe liver and kidney dysfunction. Patients were divided into the MACE group (n = 127) and the non-MACE group (n = 339) according to whether MACE occurred in the hospital after PCI. MACE defined the primary endpoint as cardiac death. Secondary endpoints were myocardial reinfarction, malignant arrhythmia, and acute heart failure. Myocardial reinfarction was defined as stent thrombosis in this study. Criteria for stent thrombosis diagnosis were according to those proposed by the Academic Research Consortium (ARC) (7). Diagnosis of acute heart failure: clinical manifestations such as shortness of breath, orthopnea, pulmonary rales, pink foamy sputum; NT-proBNP: >450 ng/L in patients under 50 years old, >900 ng/L in patients over 50 years old, >1,800 ng/L in patients over 75 years old, and >1,200 ng/L in patients with renal insufficiency (glomerular filtration rate <60 ml/min). Malignant arrhythmias include severe sinus bradycardia (≤40 beats/min), high-grade or third-degree atrioventricular block, ventricular tachycardia, ventricular fibrillation, etc., and classify cardiac arrest as a special type of malignant arrhythmia. This study has been approved by the Ethics Committee of the Second People’s Hospital of Hefei (Approval No.: 2020-ke-058). All methods were performed following the Declaration of Helsinki. PCI: refers to the treatment of transcatheter techniques to dredge the stenotic or even occluded coronary lumen, thereby improving the blood perfusion of the myocardium, including percutaneous coronary balloon angioplasty (PTCA), coronary stent implantation, coronary rotational atherectomy, intracoronary thrombus aspiration, and cutting balloon angioplasty.
2.2 Study method
2.2.1 Data collection
Demographic characteristics and clinical data of AMI patients at admission were collected through the hospital’s electronic case system, including age, gender, smoking history, heart rate at admission, systolic blood pressure, diastolic blood pressure, comorbidities (including hypertension and diabetes), Killip class II-IV, Gensini score, LVEF of echocardiography results, laboratory parameters (including neutrophils, lymphocytes, hemoglobin, platelets, total bilirubin, direct bilirubin, indirect bilirubin, albumin, triglycerides, total cholesterol, LDL-C, HDL-C, Apolipoprotein B, Apolipoprotein A1, urea, creatinine, uric acid, cystatin C, homocysteine, and fasting blood glucose, NT-ProBNP), Intervention-related data (Gensini score, D-to-B time, infarct location, number of diseased vessels, number of implanted stents, tirofiban, thrombus aspiration, IABP), and MACE data during hospitalization.
2.2.2 Nomogram establishment and verification
Least absolute shrinkage and selection operator (LASSO) regression was used to reduce the dimension of 31 clinical data in this study, predictors of non-zero coefficients were selected, and multivariate logistic regression was used to analyze independent predictors affecting in-hospital MACE after PCI in STEMI patients. Predictors were used to construct a nomogram model to predict the risk of in-hospital MACE after PCI in STEMI patients, and C-index was used to assess the predictive efficacy of the nomogram model for in-hospital MACE after PCI in STEMI patients. Bootstrap multiple sampling 1,000 times was used for model internal validation, model fit was evaluated by the Hosmer-Lemeshow test, and calibration curves were plotted to evaluate the calibration of the model. Decision curves were drawn to analyze the net benefit rate of this nomogram model in predicting in-hospital MACE after PCI in STEMI patients. Receiver operating characteristic (ROC) curves were plotted to assess the efficacy of nomogram models and thrombolysis in myocardial infarction (TIMI) scores in predicting in-hospital MACE after acute PCI in STEMI patients.
2.2.3 Statistical methods
Statistics and graphs were performed using SPSS 26.0, R4.2.1, and GraphPad Prism9.0. Kolmogorov-Smirnov normality test was performed on the measurement data, which conformed to the normal distribution and was expressed as mean ± standard deviation, and an independent sample t-test was used for comparison between the two groups; the measurement data without normal distribution were expressed as median M (P25, P75), and Mann-Whitney U test was used for comparison between the two groups; the adoption rate of enumeration data was expressed, and chi-square test was used for comparison between the two groups; LASSO regression was used to select the predictors of non-zero coefficients, and multivariate logistic regression was used to analyze the independent risk factors affecting MACE during hospitalization after PCI in STEMI patients; C-index, area under ROC curve, calibration curve, and clinical decision curve were calculated. All statistics were performed using two-sided tests, and P < 0.05 was considered statistically significant.
3 Results
3.1 Comparison of clinical data between MACE group and non-MACE patients
In this study, there were significant age differences, the proportion of women, history of hypertension, systolic and diastolic blood pressure at admission, neutrophils, hemoglobin, total bilirubin, indirect bilirubin, albumin, urea, creatinine, uric acid, cystatin C, fasting blood glucose, NT-ProBNP, LVEF, Killip class II-IV, Gensini score, number of diseased vessels, thrombus aspiration, and IABP between the MACE group and the non-MACE group (P < 0.05). There were no significant differences in diabetes history, smoking history, heart rate, lymphocytes, platelets, direct bilirubin, triglycerides, total cholesterol, LDL-C, HDL-C, Apo-B, Apo-A1, homocysteine, D-to-B time, infarct location, number of implanted stents, and tirofiban between the two groups (P > 0.05), as shown in Table 1.
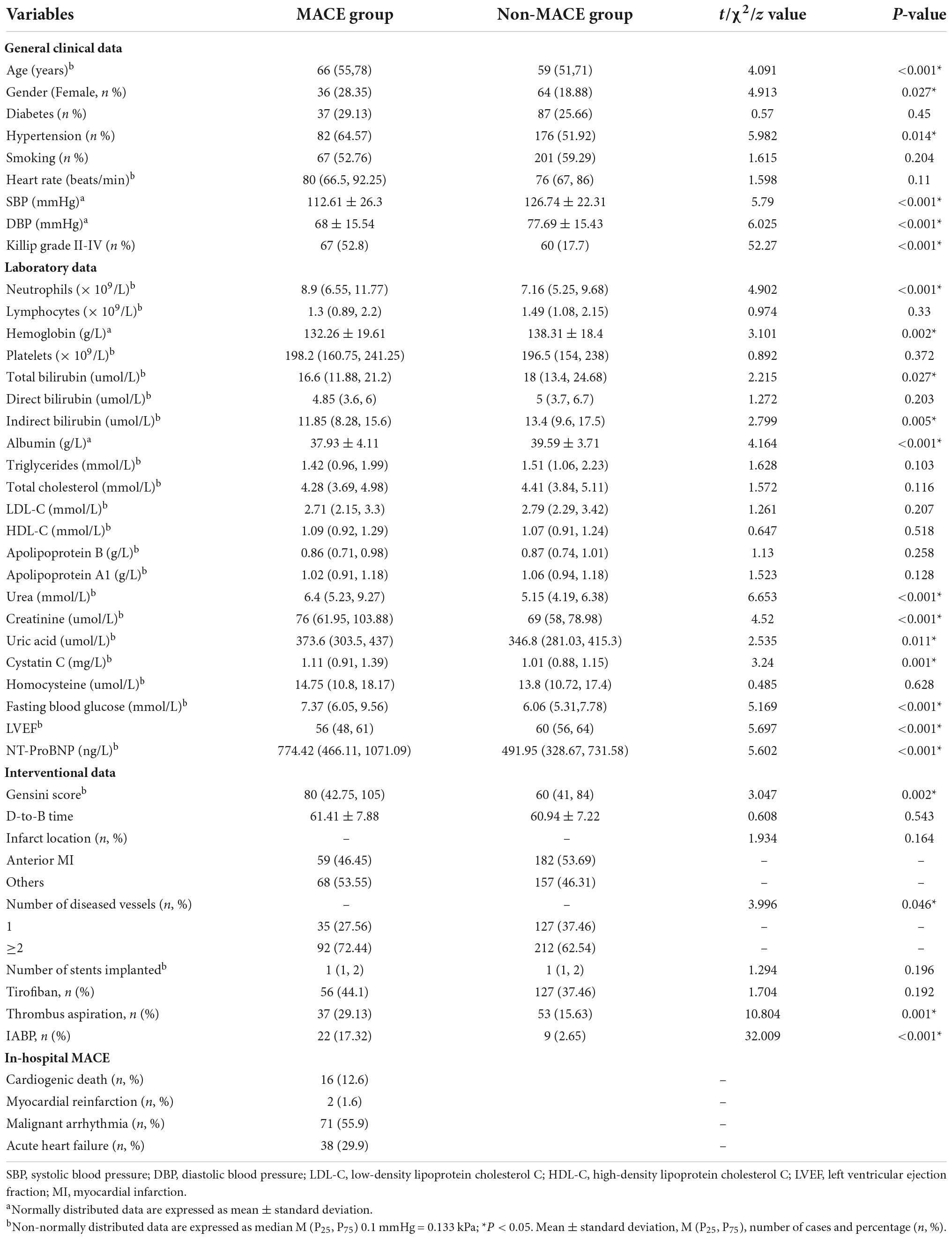
Table 1. Comparison of general clinical data between major adverse cardiovascular events (MACE) group and non-MACE group.
3.2 Construction of a risk prediction model for in-hospital MACE after PCI in STEMI patients
3.2.1 Predictor variables were filtered by lasso regression
Lasso regression analysis was performed with the presence or absence of MACE (assigned value: NO = 0, YES = 1) during hospitalization after PCI in STEMI patients as the dependent variable and the clinical data and laboratory parameters of the patients as independent variables [categorical variable (assigned value: NO = 0, YES = 1); continuous variable (assigned value: measured value)]. The 39 included variables were dimensionality reduced by Lasso regression, λ values were calculated using 10-fold cross-validation, and finally, λ values within one standard deviation of the least mean square prediction error was selected as optimal values, as shown in Figure. Final Lasso regression analysis screened seven predictors of non-zero coefficients (systolic and diastolic blood pressure at admission, Killip class II-IV, LVEF, urea, NT-ProBNP, IABP) from 39 variables. As shown in Figure 1.
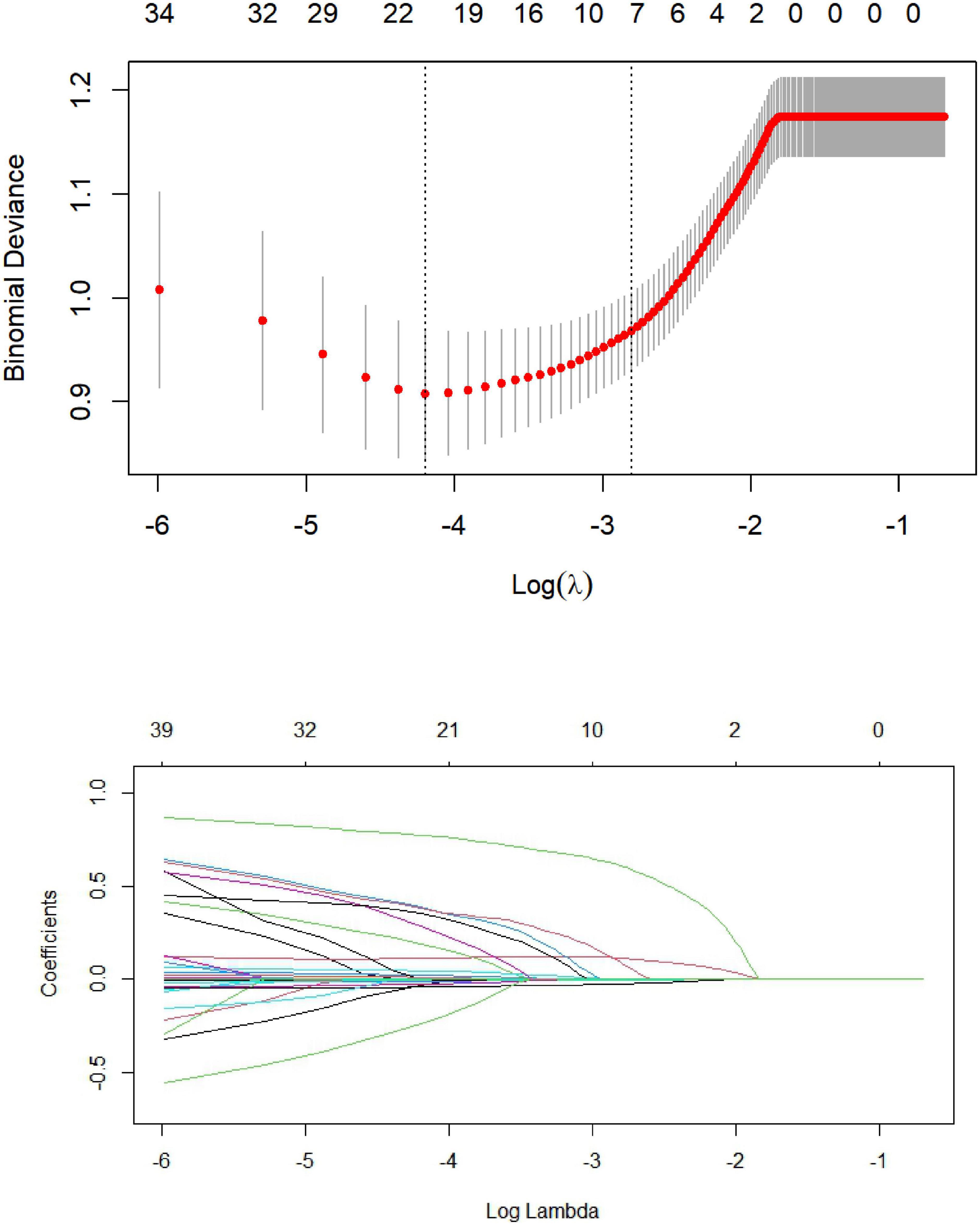
Figure 1. Predictor plots screened by least absolute shrinkage and selection operator (LASSO) regression analysis.
3.2.2 Multivariate logistic regression model construction
Seven predictive variables, systolic blood pressure (assigned value: measured value), diastolic blood pressure (assigned value: measured value), Killip class II-IV (assigned value: NO = 0, YES = 1), LVEF (assigned value: measured value), urea (assigned value: measured value), and IABP (assigned value: NO = 0, YES = 1), selected by Lasso regression, were used as dependent variables whether MACE occurred during hospitalization after PCI in STEMI patients (assigned value: NO = 0, YES = 1). The optimal Cut-Off value for MACE prediction according to NT-ProBNP was 700 ng/L, with values assigned as 0 for values less than 700 and 1 for values greater than or equal to 700. Multivariate logistic regression models were constructed using these variables as independent variables, and the results showed that Killip class II-IV, urea nitrogen, LVEF, and NT-ProBNP were independent factors for in-hospital MACE after PCI in STEMI patients (P < 0.05) as shown in Table 2. A nomogram of the predictive model for the development of in-hospital MACE after PCI in STEMI patients, the Nomogram, was drawn according to the predictive variables and is shown in Figure 2. Each predictor variable corresponds to a specific score on the horizontal axis of the nomogram score, and the scores corresponding to the three predictor variables are summed to obtain a total score. Through the total score corresponding to the risk prediction value of adverse cardiovascular events at the bottom of the nomogram, it can be seen from the figure that the patients with higher total scores are more likely to have in-hospital MACE.
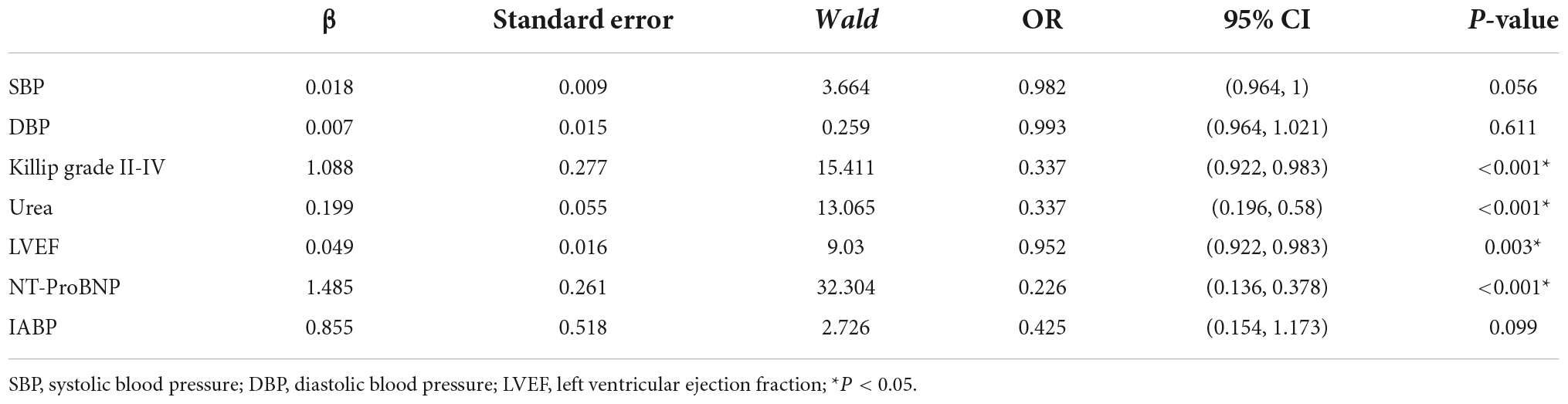
Table 2. Multivariate logistic regression analysis of influencing factors in major adverse cardiovascular events (MACE) group.
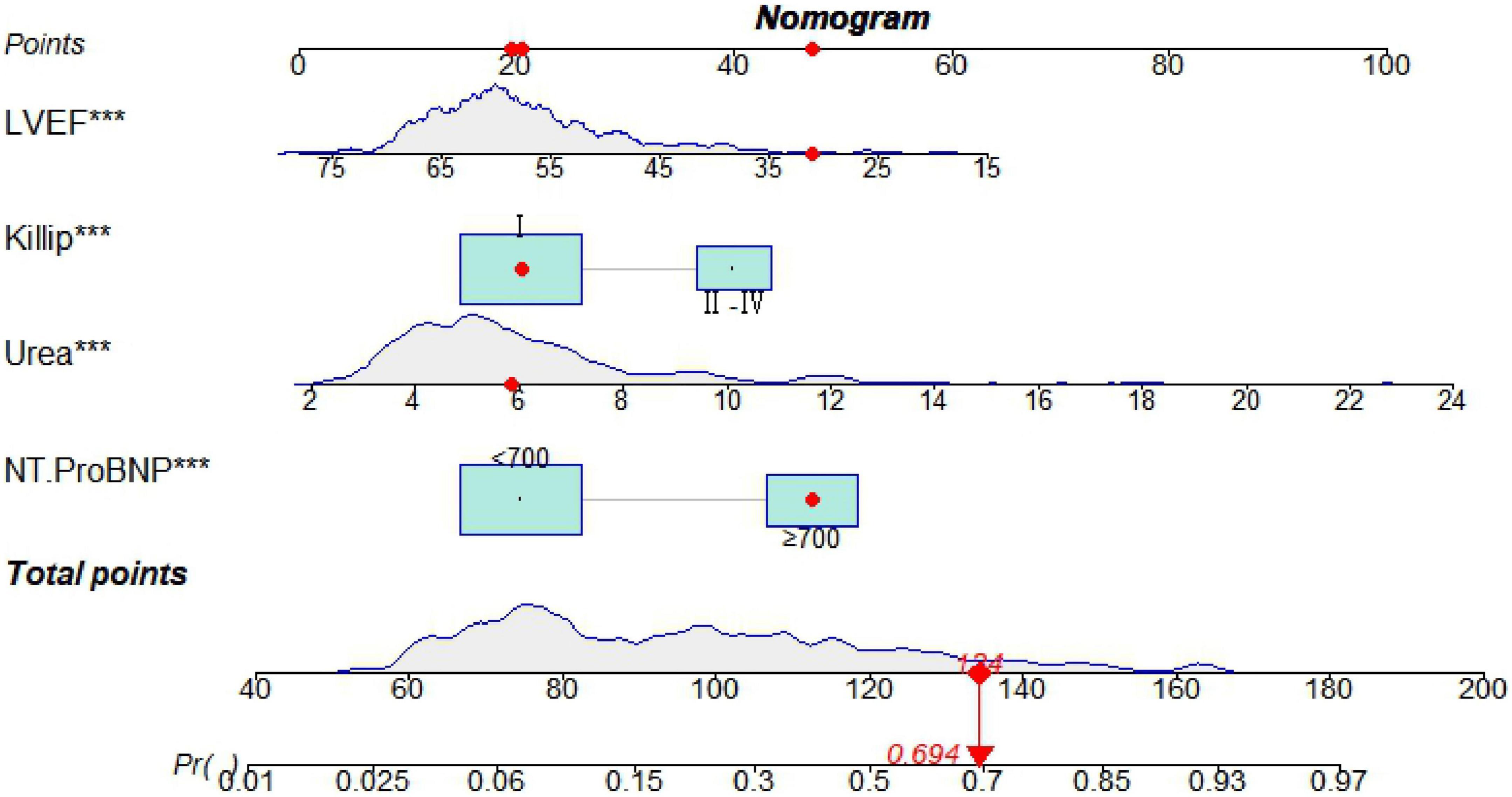
Figure 2. A nomogram predicting in-hospital major adverse cardiovascular events (MACE) after percutaneous coronary intervention (PCI) in patients with ST-segment elevation myocardial infarction (STEMI). ***P < 0.05.
3.2.3 Nomogram model validation
The nomogram model concordance index, C-index (equivalent area under the ROC curve AUC), was 0.826 (95% CI: 0.785–0.868); The sensitivity was 0.709, the specificity was 0.802, and the accuracy was 78.7% which had good predictive power. The results of the H-L goodness of fit test of the nomogram model for predicting in-hospital MACE after PCI in STEMI patients showed χ2 = 0.44, P = 0.51, and the model calibration curve was close to the ideal model, as shown in Figure 3. The internal validated C-index was 0.818 (95% CI: 0.78–0.87), suggesting that the model had good calibration; The Brier score was 0.137, suggesting that the nomogram model predicted in-hospital MACE occurrence in acute ST-segment elevation myocardial infarction with good correlation and strong calibration with internal sampling. ROC curve analysis results showed that the AUC of the nomogram model for predicting in-hospital MACE after PCI in STEMI patients was 0.826 greater than that of the TIMI score 0.696 (Z =3.567, P < 0.05) nomogram model had better predictive performance than TIMI score, as shown in Figure 4.
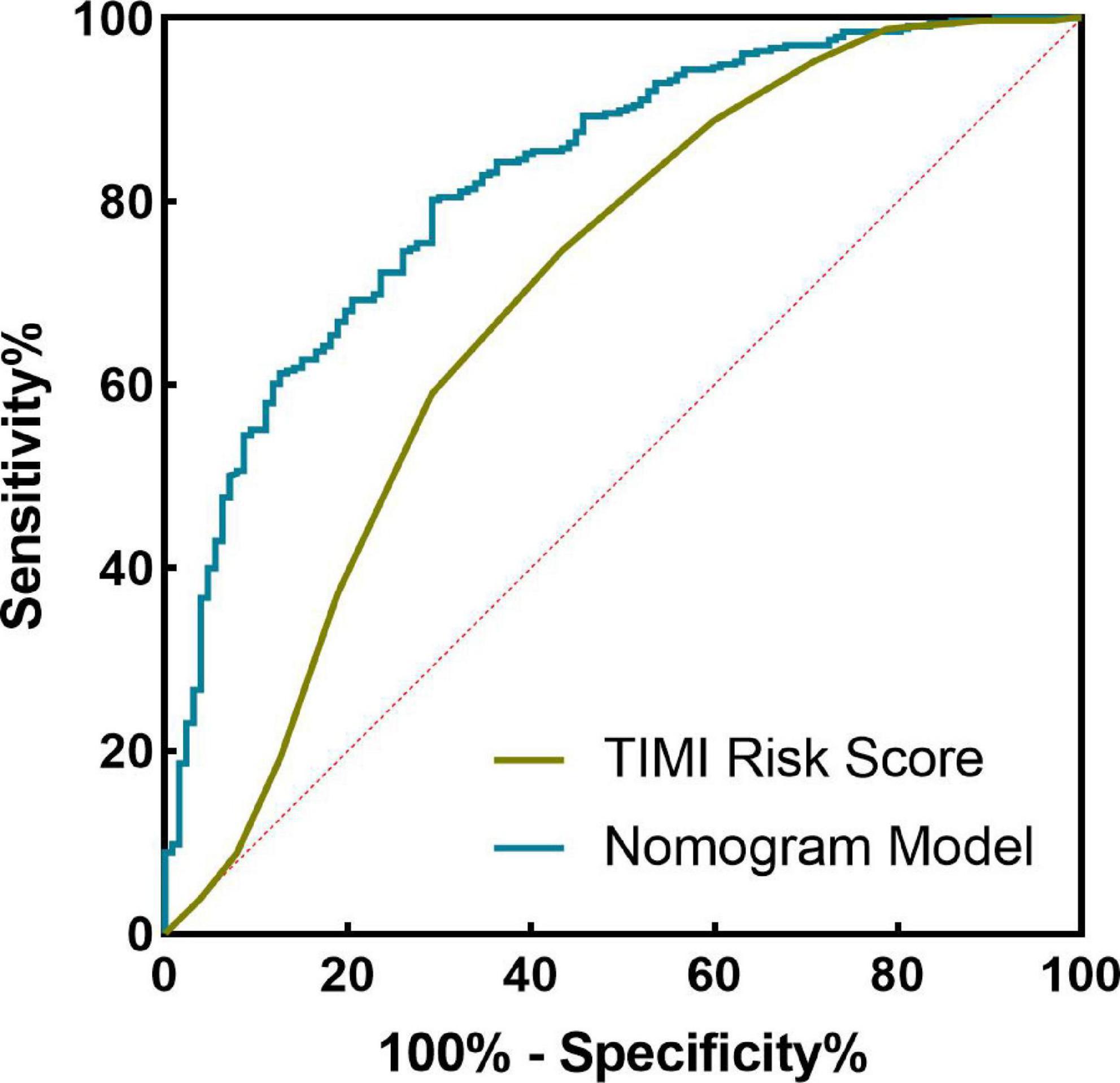
Figure 4. Receiver operating characteristic (ROC) curve of nomogram model and thrombolysis in myocardial infarction (TIMI) score in predicting the efficacy of in-hospital major adverse cardiovascular events (MACE) after percutaneous coronary intervention (PCI) in ST-segment elevation myocardial infarction (STEMI) patients.
3.2.4 Clinical decision analysis for nomogram models
The clinical decision curve (DCA) was plotted with the probability of the high-risk threshold as the abscissa and the net benefit rate as the ordinate, in which the probability of the high-risk threshold was set at (0, 1), the black solid line represented the net benefit rate of in-hospital MACE in all patients, the gray solid line represented the net benefit rate of in-hospital MACE in all patients, the sky blue curve represented the single model decision curve taking TIMI score as an example, the red curve represented the decision curve of this nomogram model, which was positive or negative relative to all study subjects, and the nomogram predicted the net benefit of the model in the interval of 0.1–0.99 with a threshold probability of 0.1–0.99, suggesting that the nomogram model could bring net clinical benefit to patients when the threshold probability was 0.1–0.99, as shown in Figure 5. The clinical impact curve (CIC) can further reflect the use of the nomogram model to predict the risk stratification of 1,000 people, showing the coordinate axis of “loss: benefit,” assigned with eight scales, green in the figure is a single factor model represented by TIMI score, red is a nomogram model, gray represents the actual occurrence of in-hospital MACE, it is seen that compared with the single model of TIMI score, the difference between the nomogram model curve and the actual occurrence curve is smaller, suggesting that the nomogram model is more suitable for the actual occurrence of in-hospital MACE in clinical practice, as shown in Figure 6.
4 Discussion
This study aimed to investigate the occurrence of MACE during hospitalization in patients with STEMI, so the primary study endpoint in the definition of MACE was cardiac death. Secondary endpoints included myocardial reinfarction, first acute or subacute thrombosis, which could be induced at the site of stent implantation due to intimal injury. In addition, when interventional therapy is performed for major vessels, it can compress branch vessels to a certain extent, resulting in vascular occlusion, and also causing some myocardial necrosis with symptoms of acute myocardial infarction. During the onset of acute myocardial infarction, various arrhythmias, especially ventricular arrhythmias, can occur due to myocardial ischemia, and in severe cases, cardiorespiratory arrest in patients. In patients with inferior myocardial infarction, inhibition of the sinoatrial node and atrioventricular node function can lead to heart rate reduction and atrioventricular block. For patients with longer ischemic events, due to more myocardial cell necrosis, patients still cannot save the necrotic myocardium after opening the vessel, resulting in decreased cardiac pump function during hospitalization, resulting in acute heart failure or even cardiogenic shock. Therefore, in this study, these indicators were selected as meeting the endpoints, and predictors were selected to construct a nomogram model to provide a reference for clinical assessment of the patient’s condition and guiding treatment.
Acute myocardial infarction (AMI) is a serious and fatal disease with high mortality and poor prognosis. Although PCI can restore myocardial perfusion associated with an infarcted artery as soon as possible and improve the prognosis of patients, the risk of major adverse cardiovascular events in patients after PCI is still very high, mainly including cardiac death, heart failure, stroke, revascularization, malignant arrhythmia, etc. (8–10). Studies have shown that poor prognosis after PCI in patients with acute myocardial infarction is associated with several indicators, such as LVEF, Killip class, Hb, and red blood cell distribution width (11–13).
Therefore, early assessment of short-term risk in STEMI patients, including assessment of the extent of myocardial injury, presence of clinical features at high risk of developing MACE, risk of reperfusion therapy, and success, is important. To obtain more effective prognostic information, the scoring system for patient risk assessment mainly includes the TIMI risk score (14), GRACE risk score (15), PAMI risk score (16), etc., of which TIMI risk score is widely used in clinical practice (14). The TIMI risk score was based on data from the TIMI-II study, which enrolled STEMI patients who presented within 6 h and underwent thrombolytic therapy, taking into account risk factors and reperfusion time, and therefore has important prognostic value for STEMI patients, especially STEMI patients receiving reperfusion therapy. The GRACE risk score model was derived from the Global Registry of Acute Coronary Events (17), which primarily enrolled patients with non-ST-segment elevation acute coronary syndrome, and the score was used to risk stratify patients based primarily on their basic clinical signs and ancillary tests and did not include the impact of reperfusion on prognosis. Therefore, it is generally believed in clinical work that the TIMI risk score is more accurate for risk stratification of STEMI patients, while the GRACE risk score is more reasonable for risk stratification of non-ST-segment elevation acute coronary syndrome patients. Therefore, early screening of patients at high risk of MACE and individualized management are beneficial to improve patient outcomes.
To better individualize patient outcomes, we used Lasso regression to screen five risk factors most associated with major adverse cardiovascular events during hospitalization: systolic blood pressure (SBP) and diastolic blood pressure (DBP) at admission, Killip class II-IV, urea, left ventricular ejection fraction (LVEF), IABP, and NT-ProBNP as significant predictors with coefficients that were not zero. Previous studies have shown that blood pressure at admission has also been shown to be a risk factor for in-hospital outcomes in STEMI patients. Acute myocardial infarction patients with hypertension have a poor prognosis (18), while other studies have shown that AMI patients with low SBP and low DBP levels at admission are significantly associated with the risk of in-hospital mortality (19, 20). In this study, we found that SBP and DBP at admission were lower and statistically significantly different in patients affecting the MACE group than in the non-MACE group. We believe that lower SBP and/or lower DBP impacts myocardial perfusion and thus adversely impacts prognosis. In this study, multivariate logistic regression analysis was performed with five predictor variables SBP, DBP, Killip class II-IV, LVEF (assigned value: measured value), and urea selected by Lasso regression as independent variables, and the results showed that Killip class II-IV and urea were independent risk factors for in-hospital MACE after PCI in STEMI patients (P < 0.05), and LVEF was an independent protective factor for in-hospital MACE after PCI in STEMI patients (P < 0.05).
Killip classification is an index to assess the severity of heart failure after acute myocardial infarction (21). Previous studies have reported that myocardial infarction patients with higher Killip grades tend to have more severe coronary artery disease and larger myocardial infarct size, which means more myocardial cell necrosis and necrotic cells are subsequently replaced by fibrotic scars, which are difficult to reverse once formed, in addition to their effects on cardiac contractility, which interfere with normal cardiac electrical activity and thus lead to arrhythmia, which may be a factor in the poor long-term prognosis of patients with higher Killip grades (22, 23). At the same time, DeGeare et al. (24) reported that patients with a higher Killip class were more likely to develop renal failure after PCI, and some patients required long-term dialysis therapy, which may also be partly responsible.
Patients with acute myocardial infarction complicated by heart failure (HF) or left ventricular dysfunction have a poor prognosis and are at high risk of rehospitalization and death (25). Assessment of left ventricular function using echocardiographic measurements of LVEF after acute myocardial infarction is an important predictor of clinical outcome (26) and can well distinguish between low and high risk of cardiac events after acute myocardial infarction. In a study of 417 patients with AMI, LVEF <40% was an independent predictor of the combined end point of death, congestive heart failure, and recurrent AMI 30 years after AMI (27). In another large prospective cohort study (28), 4,122 patients with acute myocardial infarction undergoing PCI were followed up for 4 years and found to have a significantly increased risk of sudden cardiac death and all-cause mortality in patients with LVEF ≤30 and 30< LVEF ≤40% compared with those with LVEF >40%. Another study (29), involving 28,771 patients with HF, left ventricular dysfunction, or both after acute myocardial infarction, showed that the risk of death increased with decreasing LVEF for all types of death.
NT-proBNP is an endogenous hormone produced by ventricular myocytes, and it has been shown that its peripheral content is not significantly changed in the early stages of ventricular dysfunction, but is significantly increased in patients with acute heart failure (30). In clinical practice, LVEF, NYHA functional classification, A-D stage, and other indicators are the main indicators to determine heart failure, but these indicators have a certain subjective color, in reflecting the severity of heart failure, it is bound to be subjectively affected, resulting in a certain degree of inaccuracy. NT-proBNP levels have been reported to more accurately reflect the severity of heart failure and correlate well with NYHA functional class (31). When acute heart failure occurs, NT-ProBNP levels rise dramatically in the patient’s plasma. NT-ProBNP levels have a close correlation with left ventricular systolic dysfunction (30, 32) and can be used as a sensitive indicator to determine the ventricular function and the degree of cardiac insufficiency, as well as to evaluate the clinical treatment effect and prognosis (33, 34). This study also found that serum NT-proBNP was significantly increased in patients who developed acute heart failure, consistent with the trend in the above studies.
Previous studies have shown that renal dysfunction in STEMI patients is one of the most important predictors of in-hospital and long-term mortality (35). And serum creatinine levels are closely related to prognosis after treatment (36). This study showed that creatinine and urea levels were significantly higher in the MACE group than in the non-MACE group (P < 0.05), while elevated blood urea nitrogen levels were independent risk factors for in-hospital MACE in STEMI patients. Early restoration of effective myocardial reperfusion in STEMI patients is critical to reducing acute mortality and improving prognosis; however, interventional or medical therapy is often limited by renal function and serum creatinine levels. Therefore, serum creatinine and urea nitrogen levels should be used as important predictors of prognosis when individualizing treatment regimens, and risk stratification should be performed according to renal function status as well as blood urea nitrogen and creatinine levels, which ultimately effectively reduces mortality and improves hospital outcomes.
A nomogram is a visual graph composed of line segments of different lengths that are used to predict the probability of a clinical event, is based on a multivariate regression model, and is drawn after integrating multiple clinical indicators. In this study, we constructed a nomogram model for risk prediction of in-hospital MACE after PCI in STEMI patients based on indicators that were statistically different in multivariate logistic regression analysis. The results showed that the AUC of the nomogram model for predicting in-hospital MACE after PCI in STEMI patients was greater than that of the TIMI score, indicating that the nomogram model constructed in this study had a higher predictive value for in-hospital MACE after PCI in STEMI patients compared with TIMI score. The nomogram model concordance index (C-index) was 0.826 (95% CI: 0.785–0.868), with a sensitivity of 0.709 and a specificity of 0.802, which had good predictive power. The results of the H-L goodness-of-fit test for predicting in-hospital MACE after PCI in STEMI patients showed χ2 = 1.3328, P = 0.25, and the model calibration curve was close to the ideal model, with an internally validated C-index of 0.818 and good discrimination. Clinical decision curve (DCA) analysis showed that the net benefit of the nomogram prediction model was higher in the interval of 0.1–0.99 for threshold probability, suggesting that when the threshold probability was 0.11–0.99, the nomogram model could bring net clinical benefit to patients; clinical impact curve (CIC) analysis showed that compared with the TIMI score single model, the difference between the nomogram model curve and the actual disease curve was smaller, suggesting that the nomogram model was more suitable for the actual occurrence of in-hospital MACE in clinical practice, and the prediction model judged that STEMI patients at high risk of in-hospital MACE were highly matched with STEMI patients who developed in-hospital MACE, confirming that the prediction model had a high clinical effective rate.
This study still has shortcomings: firstly, this study is a single-center study with a limited sample size, the risk factors included in the study are not comprehensive and bias cannot be avoided; secondly, in terms of model validation, only internal validation has been performed. Third, due to the limitation of our medical institution level, all enrolled patients in this study were patients who underwent coronary artery stenting, so the effect of different PCI procedures on MACE still needs further study. Lack of external validation results from other sites. Therefore, in terms of the clinical application and promotion of this model, large-sample, multicenter clinical data are still needed to provide more external evidence to support further exploring the influencing factors of in-hospital MACE after PCI in STEMI patients and optimize the nomogram model.
5 Conclusion
In summary, Killip class II-IV, urea nitrogen, and LVEF NT-ProBNP are independent factors for in-hospital MACE after PCI in STEMI patients, and a nomogram model for in-hospital MACE risk prediction after PCI in STEMI patients constructed based on the above factors has good discrimination, calibration, and clinical effectiveness and can be used as an effective tool for early clinical prediction of in-hospital MACE risk after PCI in STEMI patients.
Data availability statement
The raw data supporting the conclusions of this article will be made available by the authors, without undue reservation.
Ethics statement
The studies involving human participants were reviewed and approved by the Second People’s Hospital of Hefei Ethics Committee. Written informed consent for participation was not required for this study in accordance with the national legislation and the institutional requirements.
Author contributions
CF and ZC wrote the main manuscript text. XJ and MY prepared Tables 1, 2 and Figures 1–6. All authors reviewed the manuscript and approved the submitted version.
Conflict of interest
The authors declare that the research was conducted in the absence of any commercial or financial relationships that could be construed as a potential conflict of interest.
Publisher’s note
All claims expressed in this article are solely those of the authors and do not necessarily represent those of their affiliated organizations, or those of the publisher, the editors and the reviewers. Any product that may be evaluated in this article, or claim that may be made by its manufacturer, is not guaranteed or endorsed by the publisher.
References
1. Çınar T, Çağdaş M, Rencüzoğulları İ, Karakoyun S, Karabağ Y, Yesin M, et al. Prognostic efficacy of C-reactive protein/albumin ratio in ST elevation myocardial infarction. Scand Cardiovasc J. (2019). 53:83–90. doi: 10.1080/14017431.2019.1590628
2. Zhang H, Qiu B, Zhang Y, Cao Y, Zhang X, Wu Z, et al. The value of pre-infarction angina and plasma D-dimer in predicting no-reflow after primary percutaneous coronary intervention in st-segment elevation acute myocardial infarction patients. Med Sci Monit. (2018) 24:4528–35. doi: 10.12659/MSM.909360
3. Klingenberg R, Aghlmandi S, Räber L, Gencer B, Nanchen D, Heg D, et al. Improved risk stratification of patients with acute coronary syndromes using a combination of hsTnT, NT-proBNP and hsCRP with the GRACE score. Eur Heart J Acute Cardiovasc Care. (2018) 7:129–38. doi: 10.1177/2048872616684678
4. Zheng S, Lyu J, Han D, Xu F, Li C, Yang R, et al. Establishment of a prognostic model based on the Sequential Organ Failure Assessment score for patients with first-time acute myocardial infarction. J Int Med Res. (2021) 49:3000605211011976. doi: 10.1177/03000605211011976
5. Bai Z, Ma Y, Shi Z, Li T, Hu S, Shi B. Nomogram for the prediction of intrahospital mortality risk of patients with ST-segment elevation myocardial infarction complicated with hyperuricemia: a multicenter retrospective study. Therap Clin Risk Manag. (2021) 17:863–75. doi: 10.2147/TCRM.S320533
6. Kong S, Chen C, Zheng G, Yao H, Li J, Ye H, et al. A prognostic nomogram for long-term major adverse cardiovascular events in patients with acute coronary syndrome after percutaneous coronary intervention. BMC Cardiovasc Disord. (2021) 21:253. doi: 10.1186/s12872-021-02051-0
7. Cutlip DE, Windecker S, Mehran R, Boam A, Cohen DJ, van Es GA, et al. Clinical end points in coronary stent trials: a case for standardized definitions. Circulation. (2007) 115:2344–51. doi: 10.1161/CIRCULATIONAHA.106.685313
8. Roe MT, Messenger JC, Weintraub WS, Cannon CP, Fonarow GC, Dai D, et al. Treatments, trends, and outcomes of acute myocardial infarction and percutaneous coronary intervention. J Am Coll Cardiol. (2010) 56:254–63.
9. Krumholz HM, Normand SL, Wang Y. Trends in hospitalizations and outcomes for acute cardiovascular disease and stroke, 1999-2011. Circulation. (2014) 130:966–75. doi: 10.1161/CIRCULATIONAHA.113.007787
10. Panduranga P, Al-Rashidi M, Al-Hajri F. In-hospital and one-year clinical outcome of percutaneous coronary intervention in a tertiary hospital in Oman: Oman PCI registry. Oman Med J. (2017) 32:54–61. doi: 10.5001/omj.2017.10
11. Shiraishi J, Kohno Y, Nakamura T, Yanagiuchi T, Hashimoto S, Ito D, et al. Predictors of in-hospital outcomes after primary percutaneous coronary intervention for acute myocardial infarction in patients with a high Killip class. Internal Med. (2014) 53:933–9. doi: 10.2169/internalmedicine.53.1144
12. Saito D, Nakanishi R, Watanabe I, Yabe T, Okubo R, Amano H, et al. Combined assessment of left ventricular end-diastolic pressure and ejection fraction by left ventriculography predicts long-term outcomes of patients with ST-segment elevation myocardial infarction. Heart Vessels. (2018) 33:453–61. doi: 10.1007/s00380-017-1080-6
13. Brener SJ, Mehran R, Dangas GD, Ohman EM, Witzenbichler B, Zhang Y, et al. Relation of baseline hemoglobin levels and adverse events in patients with acute coronary syndromes (from the acute catheterization and urgent intervention triage strategY and harmonizing outcomes with revasculariZatiON and stents in acute myocardial infarction trials). Am J Cardiol. (2017) 119:1710–6. doi: 10.1016/j.amjcard.2017.02.052
14. Morrow DA, Antman EM, Charlesworth A, Cairns R, Murphy SA, de Lemos JA, et al. TIMI risk score for ST-elevation myocardial infarction: a convenient, bedside, clinical score for risk assessment at presentation: an intravenous nPA for treatment of infarcting myocardium early II trial substudy. Circulation. (2000) 102:2031–7. doi: 10.1161/01.cir.102.17.2031
15. Eagle KA, Lim MJ, Dabbous OH, Pieper KS, Goldberg RJ, Van de Werf F, et al. A validated prediction model for all forms of acute coronary syndrome: estimating the risk of 6-month postdischarge death in an international registry. JAMA. (2004) 291:2727–33. doi: 10.1001/jama.291.22.2727
16. Addala S, Grines CL, Dixon SR, Stone GW, Boura JA, Ochoa AB, et al. Predicting mortality in patients with ST-elevation myocardial infarction treated with primary percutaneous coronary intervention (PAMI risk score). Am J Cardiol. (2004) 93:629–32. doi: 10.1016/j.amjcard.2003.11.036
17. Granger CB, Goldberg RJ, Dabbous O, Pieper KS, Eagle KA, Cannon CP, et al. Predictors of hospital mortality in the global registry of acute coronary events. Arch Internal Med. (2003) 163:2345–53. doi: 10.1001/archinte.163.19.2345
18. Kenchaiah S, Pfeffer MA, St John Sutton M, Plappert T, Rouleau JL, Lamas GA, et al. Effect of antecedent systemic hypertension on subsequent left ventricular dilation after acute myocardial infarction (from the survival and ventricular enlargement trial). Am J Cardiol. (2004) 94:1–8. doi: 10.1016/j.amjcard.2004.03.020
19. Yap YG, Duong T, Bland JM, Malik M, Torp-Pederson C, Køber L, et al. Prognostic value of blood pressure measured during hospitalization after acute myocardial infarction: an insight from survival trials. J Hypertens. (2007) 25:307–13. doi: 10.1097/HJH.0b013e3280115bae
20. Shiraishi J, Kohno Y, Sawada T, Ito D, Kimura M, Ariyoshi M, et al. Systolic blood pressure at admission, clinical manifestations, and in-hospital outcomes in patients with acute myocardial infarction. J Cardiol. (2011) 58:54–60. doi: 10.1016/j.jjcc.2011.04.003
21. Killip T III, Kimball JT. Treatment of myocardial infarction in a coronary care unit. A two year experience with 250 patients. Am J Cardiol. (1967) 20:457–64. doi: 10.1016/0002-914990023-9
22. Khot UN, Jia G, Moliterno DJ, Lincoff AM, Khot MB, Harrington RA, et al. Prognostic importance of physical examination for heart failure in non-ST-elevation acute coronary syndromes: the enduring value of Killip classification. JAMA. (2003) 290:2174–81. doi: 10.1001/jama.290.16.2174
23. Francis Stuart SD, De Jesus NM, Lindsey ML, Ripplinger CM. The crossroads of inflammation, fibrosis, and arrhythmia following myocardial infarction. J Mol Cell Cardiol. (2016) 91:114–22. doi: 10.1016/j.yjmcc.2015.12.024
24. DeGeare VS, Boura JA, Grines LL, O’Neill WW, Grines CL. Predictive value of the Killip classification in patients undergoing primary percutaneous coronary intervention for acute myocardial infarction. Am J Cardiol. (2001) 87:1035–8. doi: 10.1016/s0002-914901457-6
25. Metra M, Cotter G, El-Khorazaty J, Davison BA, Milo O, Carubelli V, et al. Acute heart failure in the elderly: differences in clinical characteristics, outcomes, and prognostic factors in the VERITAS study. J Card Fail. (2015) 21:179–88. doi: 10.1016/j.cardfail.2014.12.012
26. Prastaro M, Pirozzi E, Gaibazzi N, Paolillo S, Santoro C, Savarese G, et al. Expert review on the prognostic role of echocardiography after acute myocardial infarction. J Am Soc Echocardiogr. (2017) 30:431–43.e2. doi: 10.1016/j.echo.2017.01.020
27. Schwammenthal E, Adler Y, Amichai K, Sagie A, Behar S, Hod H, et al. Prognostic value of global myocardial performance indices in acute myocardial infarction: comparison to measures of systolic and diastolic left ventricular function. Chest. (2003) 124:1645–51. doi: 10.1378/chest.124.5.1645
28. Shiga T, Hagiwara N, Ogawa H, Takagi A, Nagashima M, Yamauchi T, et al. Sudden cardiac death and left ventricular ejection fraction during long-term follow-up after acute myocardial infarction in the primary percutaneous coronary intervention era: results from the HIJAMI-II registry. Heart. (2009) 95:216–20. doi: 10.1136/hrt.2008.145243
29. Hall TS, von Lueder TG, Zannad F, Rossignol P, Duarte K, Chouihed T, et al. Relationship between left ventricular ejection fraction and mortality after myocardial infarction complicated by heart failure or left ventricular dysfunction. Int J Cardiol. (2018) 272:260–6. doi: 10.1016/j.ijcard.2018.07.137
30. Pemberton CJ, Johnson ML, Yandle TG, Espiner EA. Deconvolution analysis of cardiac natriuretic peptides during acute volume overload. Hypertension. (2000) 36:355–9. doi: 10.1161/01.hyp.36.3.355
31. Pizzolo F, Zorzi F, Chiecchi L, Consoli L, Aprili I, Guarini P, et al. NT-proBNP, a useful tool in hypertensive patients undergoing a diagnostic evaluation for primary aldosteronism. Endocrine. (2014) 45:479–86. doi: 10.1007/s12020-013-0028-6
32. Chen AA, Wood MJ, Krauser DG, Baggish AL, Tung R, Anwaruddin S, et al. NT-proBNP levels, echocardiographic findings, and outcomes in breathless patients: results from the ProBNP Investigation of Dyspnoea in the Emergency Department (PRIDE) echocardiographic substudy. Eur Heart J. (2006) 27:839–45. doi: 10.1093/eurheartj/ehi811
33. Januzzi JL Jr, Camargo CA, Anwaruddin S, Baggish AL, Chen AA, Krauser DG, et al. The N-terminal pro-BNP Investigation of Dyspnea in the Emergency Department (PRIDE) study. Am J Cardiol. (2005) 95:948–54. doi: 10.1016/j.amjcard.2004.12.032
34. Weber M, Hamm C. Role of B-type natriuretic peptide (BNP) and NT-proBNP in clinical routine. Heart. (2006) 92:843–9. doi: 10.1136/hrt.2005.071233
35. Zhao L, Wang L, Zhang Y. Elevated admission serum creatinine predicts poor myocardial blood flow and one-year mortality in ST-segment elevation myocardial infarction patients undergoing primary percutaneous coronary intervention. J Invasive Cardiol. (2009) 21:493–8.
36. O’Gara PT, Kushner FG, Ascheim DD, Casey DE Jr, Chung MK, de Lemos JA, et al. 2013 ACCF/AHA guideline for the management of ST-elevation myocardial infarction: executive summary: a report of the American College of Cardiology Foundation/American Heart Association Task Force on Practice Guidelines. Circulation. (2013) 127:529–55. doi: 10.1161/CIR.0b013e3182742c84
Keywords: acute ST-segment elevation myocardial infarction, STEMI, percutaneous coronary intervention, PCI, MACE, nomogram model
Citation: Fang C, Chen Z, Zhang J, Jin X and Yang M (2022) Construction and evaluation of nomogram model for individualized prediction of risk of major adverse cardiovascular events during hospitalization after percutaneous coronary intervention in patients with acute ST-segment elevation myocardial infarction. Front. Cardiovasc. Med. 9:1050785. doi: 10.3389/fcvm.2022.1050785
Received: 22 September 2022; Accepted: 29 November 2022;
Published: 21 December 2022.
Edited by:
Gianluca Rigatelli, Hospital Santa Maria della Misericordia of Rovigo, ItalyReviewed by:
Jun Chen, Hubei University of Medicine, ChinaZhaoqing Sun, Shengjing Hospital of China Medical University, China
Copyright © 2022 Fang, Chen, Zhang, Jin and Yang. This is an open-access article distributed under the terms of the Creative Commons Attribution License (CC BY). The use, distribution or reproduction in other forums is permitted, provided the original author(s) and the copyright owner(s) are credited and that the original publication in this journal is cited, in accordance with accepted academic practice. No use, distribution or reproduction is permitted which does not comply with these terms.
*Correspondence: Zhenfei Chen, MTYwMTk5NDQ5MkBxcS5jb20=