- Department of Anesthesiology, Union Hospital, Tongji Medical College, Huazhong University of Science and Technology, Wuhan, China
Background: Successful weaning and extubation after cardiac surgery is an important step of postoperative recovery. Delayed extubation is associated with poor prognosis and high mortality, thereby contributing to a substantial economic burden. The aim of this study was to develop and validate a prediction model estimate the risk of delayed extubation after cardiac surgery based on perioperative risk factors.
Methods: We performed a retrospective cohort study of adult patients undergoing cardiac surgery from 2014 to 2019. Eligible participants were randomly assigned into the development and validation cohorts, with a ratio of 7:3. Variables were selected using least absolute shrinkage and selection operator (LASSO) logistic regression model with 10-fold cross-validation. Multivariable logistic regression was applied to develop a predictive model by introducing the predictors selected from the LASSO regression. Receiver operating characteristic (ROC) curve, calibration plot, decision curve analysis (DCA) and clinical impact curve were used to evaluate the performance of the predictive risk score model.
Results: Among the 3,919 adults included in our study, 533 patients (13.6%) experienced delayed extubation. The median ventilation time was 68 h in the group with delayed extubation and 21 h in the group without delayed extubation. A predictive scoring system was derived based on 10 identified risk factors based on 10 identified risk factors including age, BMI ≥ 28 kg/m2, EF < 50%, history of cardiac surgery, type of operation, emergency surgery, CPB ≥ 120 min, duration of surgery, IABP and eGFR < 60 mL/min/1.73 m2. According to the scoring system, the patients were classified into three risk intervals: low, medium and high risk. The model performed well in the validation set with AUC of 0.782 and a non-significant p-value of 0.901 in the Hosmer-Lemeshow test. The DCA curve and clinical impact curve showed a good clinical utility of this model.
Conclusions: We developed and validated a prediction score model to predict the risk of delayed extubation after cardiac surgery, which may help identify high-risk patients to target with potential preventive measures.
Introduction
With innovations and advances in medical management and surgical techniques, a growing number of patients undergo cardiac surgery, including critically ill patients with multiple comorbidities (1). Although most of the patients can resume spontaneous respiration as soon as possible after cardiac surgery, successful weaning from mechanical ventilation remains a challenge (2, 3). Due to prolonged ventilator support, up to 20% of patients experienced delayed extubation after cardiac surgery, which increased the risk of postoperative complications including acute kidney injury and delirium (4, 5). More notably, among patients requiring prolonged mechanical ventilation, the recently observed in-hospital mortality rate was as high as 50.3% (6, 7). Additionally, the need for prolonged postoperative mechanical ventilation imposes a burden on the allocation of critical care resources such as ventilator and intensive care unit (ICU) beds. Therefore, early identification of patients at risk of delayed extubation appears important.
A comprehensive assessment system incorporating preoperative and intraoperative factors can accurately predict the risk of delayed extubation after cardiac surgery and guide clinical decision-making. Although some predictive scoring systems for delayed extubation after cardiac surgery have been reported (8–11), specifically, sample size issues, single type of surgery and imperfection of assessment system in these studies have limited the application and promotion of the predictive models in clinical practice. Moreover, the majority of these participant were from developed countries. Considering the differences in lifestyle, income and medical resources, a novel predictive model that can be widely used in developing countries is still needed.
The risk factors for delayed extubation are mainly attributed to patient-related and surgery-related factors, but the optimal combination of predictors has not been identified. Therefore, the purpose of this study was first to evaluate the duration of postoperative mechanical ventilation and then identify the potential risk factors for delayed extubation. Ultimately, the results were used to establish and validate a model to help clinicians understand the likelihood of delayed extubation and allocate postoperative medical resources appropriately at the first time after cardiac surgery.
Methods
Ethical statement
Permission for this retrospective study was obtained from the Ethics Committee of Union Hospital affiliated to Tongji Medical College of Huazhong University of Science and Technology (No. 2022-0465). The written informed consent was waived by the institutional review boards because of the retrospective and anonymous nature of the data. This study was conducted in accordance with the ethical principles stated in the Declaration of Helsinki and reported according to TRIPOD guidelines (Supplementary Table 1) (12).
Study design and population
From January 2014 to December 2019, all consecutive adult patients undergoing cardiac surgery were enrolled in this retrospective observational study at Union Hospital in Wuhan, China. Surgical procedures were extensive, including coronary artery bypass graft (CABG), valve repair or replacement procedures (single or multiple), aortic surgery (due to aortic aneurysm and dissection) and combined surgeries. Because of the retrospective and observational nature, no formal sample size calculation was performed.
Participants were excluded from this study if they met any of the following criteria: (1) preoperative mechanical ventilation; (2) combined with non-cardiac surgeries like lobectomy; (3) second thoracotomy after surgery; (4) died or discharged within 48 h after surgery; (5) clinical data loss.
Data collection
Demographic and clinical information were extracted from the electronic medical record of the hospital. Preoperative variables included age, gender, body mass index (BMI), history of smoking and alcohol, diabetes, hypertension, lung disease, heart disease, ejection fraction (EF), cerebrovascular disease, cancer history, renal insufficiency, surgery history and use of vasoactive agents and antibiotics. Pulmonary arterial hypertension (PAH) was defined Doppler echocardiography or other results of the hemodynamic tests. Cerebrovascular disease included any history of stroke, transient ischemic attack, carotid endarterectomy, and carotid stenting. Preoperative medication was defined as 1 week prior to surgery and used for more than 3 consecutive days.
Intraoperative factors were as follows: type of surgery classified in six categories, emergency surgery, surgical approach (median sternotomy vs. minimally invasive), cardiopulmonary bypass (CPB) and aortic cross clamp (ACC) time, transfusion of blood product, operative duration and use of intra-aortic balloon pump (IABP). Autologous transfusion was excluded from the transfusion record.
Preoperative laboratory test results included neutrophil–lymphocyte ratio (NLR), platelet–lymphocyte ratio (PLR), lymphocyte-to-monocyte ratio (LMR), hemoglobin, albumin, estimated glomerular filtration rate (eGFR). Anemia was defined as hemoglobin < 130 g/L for men or < 120 g/L for women.
Study outcomes
Delayed extubation was defined as postoperative mechanical ventilation lasting >48 h or reintubation within the first 2 days after surgery. Also, other possible outcomes were recorded: length of ICU stay and postoperative hospital days, major postoperative complications and in-hospital mortality.
Neurological complications included cerebral ischemic infarct or intracerebral hemorrhage with the presence of imaging evidence. Pneumonia and cardiac arrest were defined according to European Perioperative Clinical Outcome (EPCO) definitions (13).
Statistical analysis
In the initial variable pool, two variables that were missing in >20% of patients were directly removed, which were forced expiratory volume in 1 s (FEV1) to forced expiratory volume (FCV) ratio and New York Heart Association (NYHA) classification. Then, we again excluded individuals with missing variables, including those with missing rate < 5%. As such, no statistical interpolation techniques were used in our dataset to ensure high clinical relevance. According to clinical relevance and practicality, we transformed the corresponding continuous variables into categorical variables. Data were not normally distributed. For demographic and clinical variables, continuous variables were described using medians with interquartile ranges (IQR), and categorical variables were summarized as frequencies with percentage. Differences between groups were compared using the chi-square tests for categorical data and nonparametric tests for continuous data as appropriate.
The full cohort was randomly assigned into the development and validation sets at a 7:3 ratio. We used the training cohort to derive a risk prediction score system and then externally validated the score system in the validation cohort. Least absolute shrinkage and selection operator (LASSO) regression is a very popular technique to shrink and select variables for regression models (14). First, we use LASSO regression to narrow the scope of the candidate predictors, using cross-validation as the criterion to select variables. The variables selected in the LASSO analysis were entered into multivariable logistic regression analysis. Then, variables with P-value < 0.05 were applied to construct a risk prediction score system for delayed extubation after cardiac surgery. In order to facilitate visualization, predicted risk scores were assigned by dividing the regression coefficient of each significant factor by the smallest β coefficient and then rounded to the closest integer. The total point for each patient was calculated by summing the scores of existing risk factors. Patients in the cohort were ranked and classified into 3 risk groups based on the tertiles of total risk scores: low risk (< 33%), medium risk (33–66%), and high risk >66%.
The risk prediction model was evaluated and validated using the data of training set and validation set, respectively. Receiver operating characteristic (ROC) curve was used to access the capability of the predictive model to discriminate true positives from false positives. The accuracy of model was evaluated by Hosmer-Lemeshow goodness-of-fit test and calibration curve. Clinical usefulness and net benefit of the model were estimated with decision curve analysis (DCA) and clinical impact curve. All statistical analysis was performed using R software (version 4.2.0). A P-value of < 0.05 was considered statistically significant.
Results
Characteristics of the study cohort
Data of 5,136 consecutive cases were retrieved from the hospital record database and 1,217 patients were excluded (Figure 1). A total of 3,919 patients were included in this study: 777 (19.8%) isolated coronary artery bypass grafting (CABG), 2,452 (62.6%) isolated valve surgery, 238 (6.1%) isolated aortic surgery, 380 (9.7%) combined CABG and valve procedures, 37 (0.9%) combined valve and aortic procedures and 35 (0.9%) combined CABG and aortic procedures. Of the 3,919 participants in our analysis, 533 (13.6%) patients experienced delayed extubation. Compared with patients without delayed extubation, patients with delayed extubation were older and had a higher percentage of comorbidities such as hypertension, coronary heart disease, atrial fibrillation, previous myocardial infarction, pulmonary disease, cerebrovascular disease and renal insufficiency (Table 1). All of the 3,919 patients were randomly assigned into two independent sets: the training cohort (n = 2,743) and the validation cohort (n = 1,176). We compared details of the demographic and clinical variables between these two groups and results show that there were no significant differences in demographic characteristics (Table 1). In summary, the training cohort and the validation cohort shared similar characteristics, including the incidence of delayed extubation (13.7 vs. 13.3%, P = 0.689).
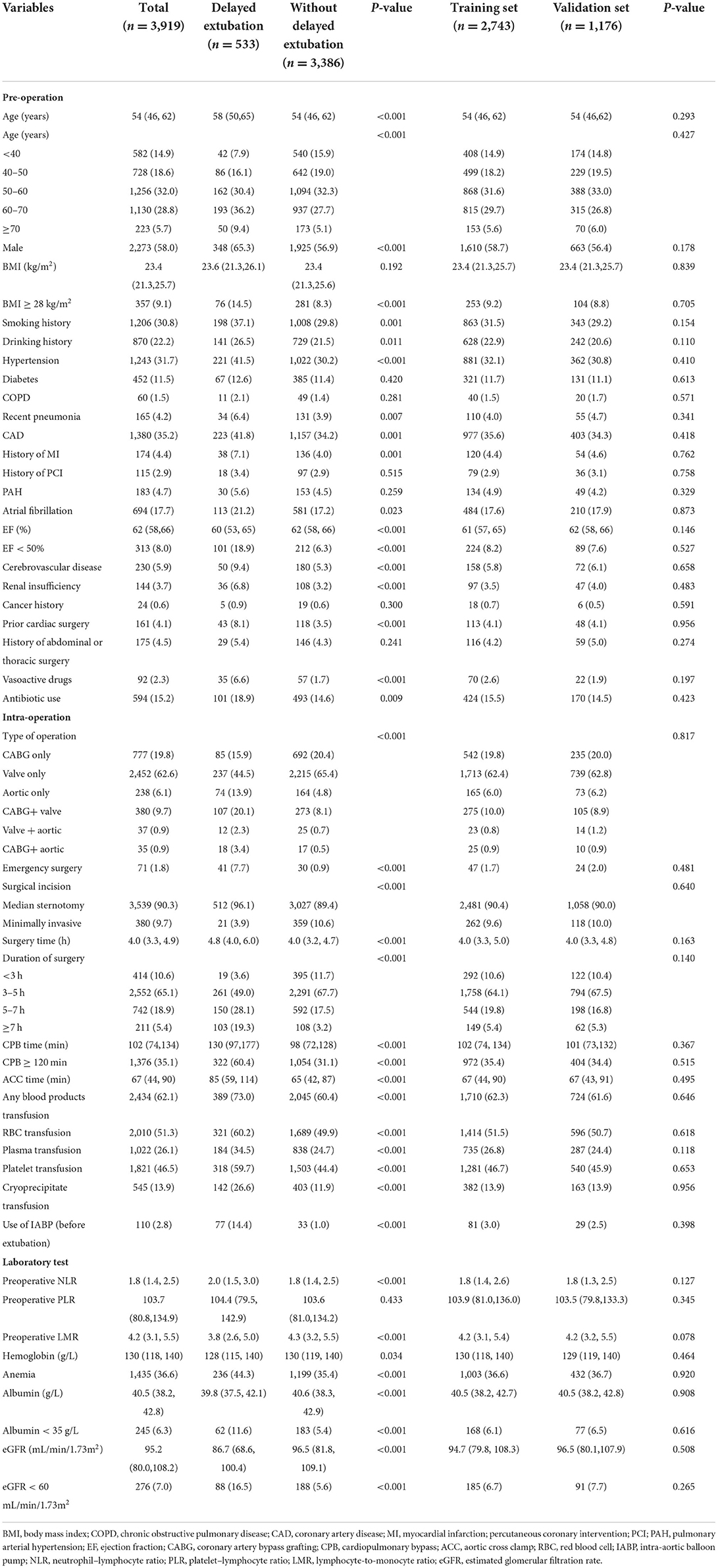
Table 1. The demographic and clinical characteristics of patients in the training and validation datasets.
Predictive model development
Based on the training data, a total of 40 candidate parameters were entered into the LASSO regression analysis, and variables were selected according to the optimal lambda value values after the 10-fold cross-validation procedure (Figure 2). After the initial screening, 13 potential variables, including age, BMI ≥ 28 kg/m2, EF < 50%, history of cardiac surgery, use of vasoactive drugs, type of operation, emergency surgery, CPB ≥ 120 min, duration of surgery, IABP, eGFR < 60 mL/min/1.73 m2, intraoperative infusion of platelets and cryoprecipitate, were incorporated into the next multivariable logistic regression analysis. The results indicated that age, BMI ≥ 28 kg/m2, EF < 50%, history of cardiac surgery, type of operation, emergency surgery, CPB ≥ 120 min, duration of surgery, IABP and eGFR < 60 mL/min/1.73 m2 were independently associated with delayed extubation after cardiac surgery. Finally, these 10 predictors were introduced to construct a risk prediction model and assigned specific weight scores according to the corresponding coefficients (Table 2). The assigned scores for these predictors ranged from 1 to 13 and the possible total scores for each patient ranged from 0 to 64.
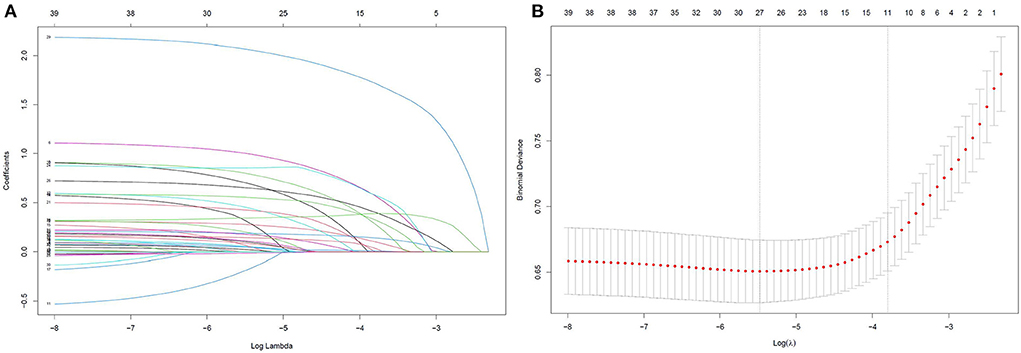
Figure 2. LASSO regression was used to select the potential risk factors. (A) LASSO coefficient curves for the 40 clinical variables; (B) Binomial Deviance of the LASSO model with different λ. The optimal value of λ (the vertical dashed line) for 10-fold cross-validation was used to select optimal variables. LASSO, least absolute shrinkage and selection operator.
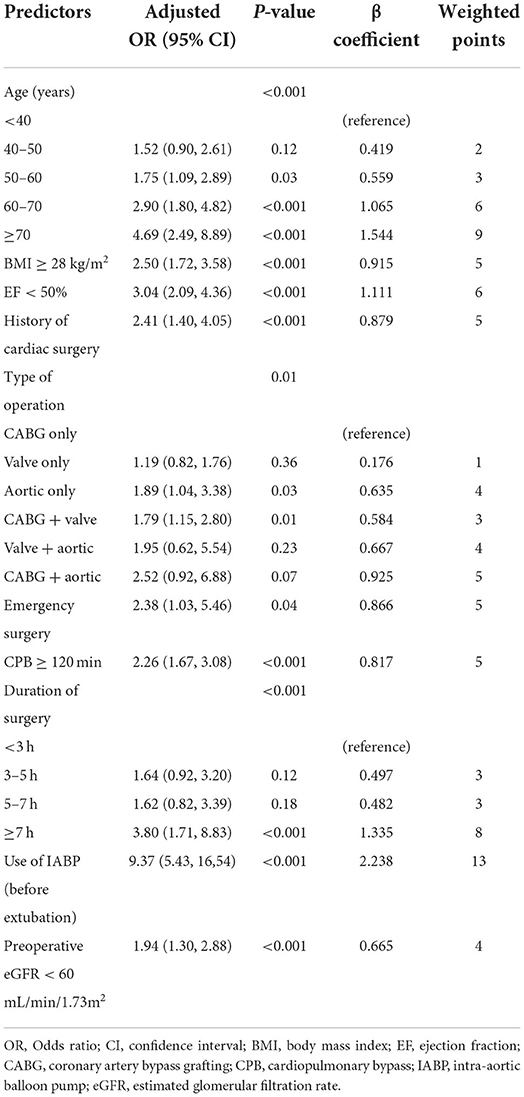
Table 2. Multivariable logistic regression model for predictors of delayed extubation and weighted score assignment.
Predictive model validation
In this study, the median risk score was 10 in both cohorts with the highest risk score of 43 in the development cohort and 39 in validation cohort. Based on the distribution of total points, patients in the development and validation cohorts were classified into low- (0–8 points), medium- (9–12 points), and high-risk (≥13 points) groups. In both cohorts, there was significant difference for the proportion of patients with delayed extubation among the three risk groups. Compared with the low-risk patients, patients in medium-risk had ~2 times greater risk of delayed extubation, and patients in medium-risk had ~10 times greater risk (Table 3).
For the predictive model, the reported area under the ROC is 0.807 (0.783, 0.832) in the development cohort and 0.782 (0.742, 0.823) in the validation cohort, indicating a good discrimination power (Figure 3). The overlapping of the actual and predicted calibration plots suggested good fit of the model (Figure 4). The calibration was further confirmed using the Hosmer-Lemeshow test, with a non-significant P-value of 0.401 in the development cohort and 0.901 in the validation cohort.
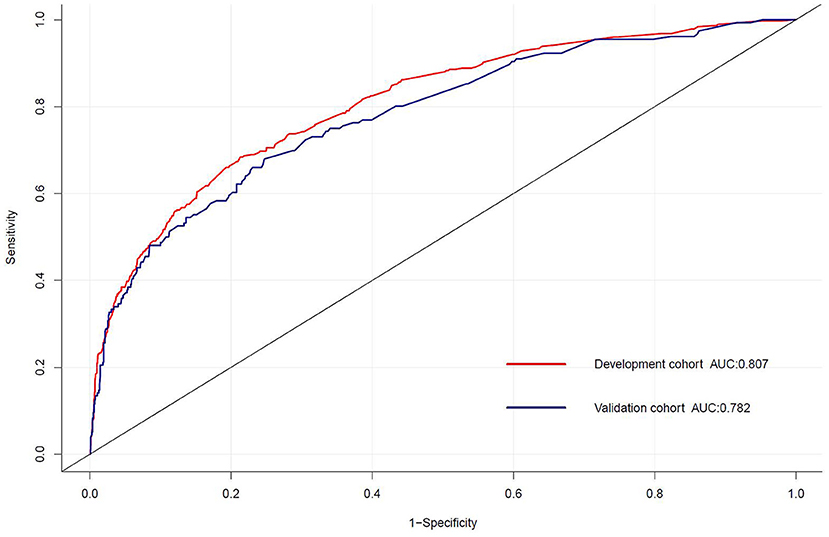
Figure 3. The receiver operating characteristic (ROC) curve for the prediction model of delayed extubation. The area under the curve (AUC) of the model exhibited good discriminating power in the development and validation cohorts.
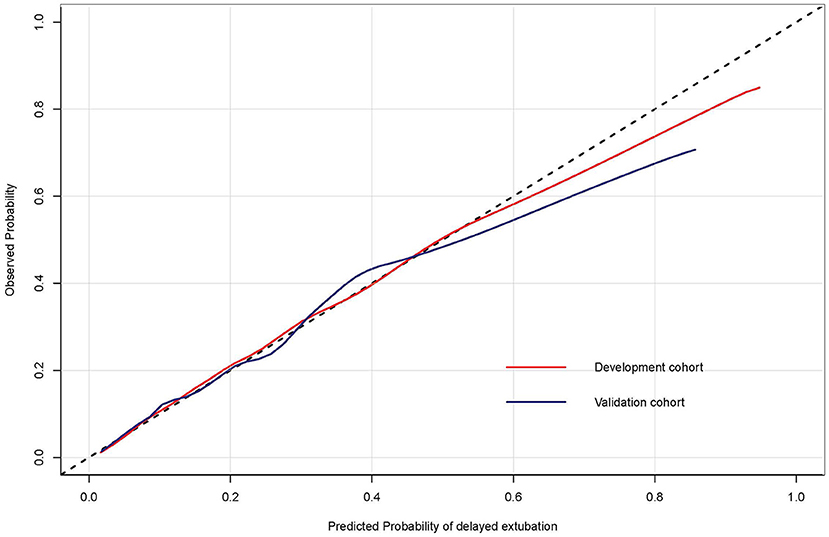
Figure 4. The calibration curves of the risk prediction model of delayed extubation. The diagonal dashed line indicated perfect prediction of the ideal model. The solid line represented the performance of the model in the development and validation cohorts, and being closer to the diagonal dashed line indicated that the model has better prediction ability.
Decision curve analysis (DCA) was performed to explore the clinical usefulness of the model by quantifying the net benefits at different threshold probabilities. The probability threshold of the model was 3–74% in the development cohort and 3–62% in the validation cohort (Figure 5). Compared with the “all happened” and “none happened” assumptions, the prediction model could provide good predictive net benefit for a wide range of decision threshold. The clinical impact curves also suggested the higher usefulness of the prediction model in a realistic clinical setting (Figure 6).
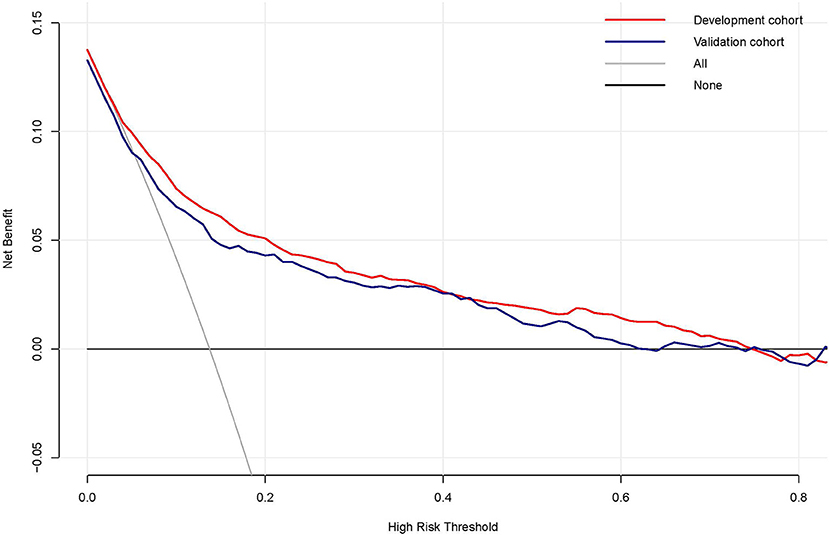
Figure 5. The decision curve analysis of the risk prediction model of delayed extubation. The vertical axis measured the net benefit. The gray solid line represented the assumption that no patient has delayed extubation. The black solid line represented the assumption that all patients appeared delayed extubation. The red line and blue line represented the prediction model from the development and validation cohorts, respectively.
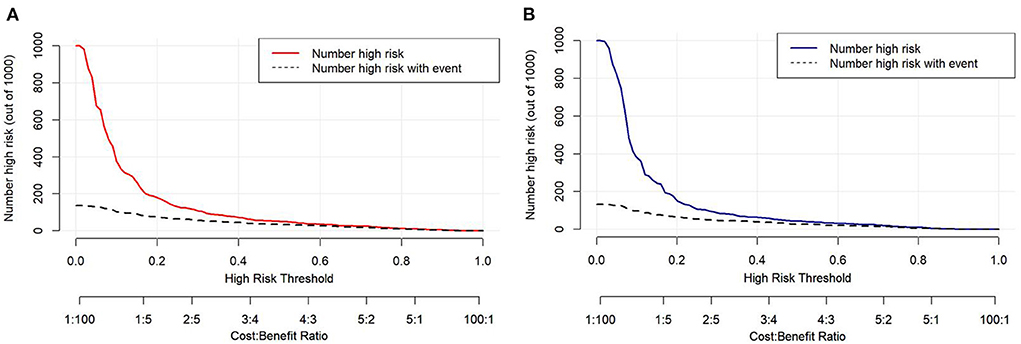
Figure 6. The clinical impact curves of the risk prediction model in the development cohort (A) and validation cohort (B). The number of high-risk patients and the number of high-risk patients with delayed extubation were plotted at different threshold probabilities within a given population.
Clinical outcomes
To assess the potential impact of delayed extubation on patient prognosis, we compared several clinical outcomes including major postoperative complications and hospital length of stay (Table 4). Patients with delayed extubation had a median duration of postoperative mechanical ventilation of 68 (52, 92) h, compared with 21 (15, 16) h in patients without delayed extubation. Obviously, patients required delayed extubation tended to have a longer stay in the ICU and hospital than patients extubated within 48 h. Additionally, patients required delayed extubation in our study exhibited significantly higher extrapulmonary complications such as cardiac arrest, neurological complications and continuous renal replacement therapy (CRRT). Also, the significant differences of mortality in-hospital between two groups were observed (3.8 vs. 0.5%, P < 0.001).
Clinical risk assessment form
Considering the availability and practicality of the model, the final 10 predictors are formatted as a short and simple risk assessment form, which formally combines the risk score and risk stratification (Table 5). In addition to being reproduced for clinical use, the form provided is easy to document and preserve the risk assessment findings in clinical setting.
Discussion
In this study, we identified 10 risk factors associated with delayed extubation: age, BMI ≥ 28 kg/m2, EF < 50%, history of cardiac surgery, type of operation, emergency surgery, CPB ≥ 120 min, duration of surgery, IABP and eGFR < 60 mL/min/1.73 m2. Then, we developed and internally validated the prediction model to generate individualized risk score of delayed extubation in cardiac surgery patients. The total risk score enables stratification of patients into low-risk, medium-risk and high-risk groups. Compared with the low-risk group ( ≤ 8 points), there was a significant trend toward increased incidence of delayed extubation in the medium-risk group (9–12 points) and high-risk group (≥13 points). The model was based on demographic and perioperative clinical variables for which information would be routinely obtained at the end of surgery. Consequently, this scoring model will contribute to an earlier assessment of extubation time and enable clinicians to develop specific treatment strategies as quickly as possible.
Duration of postoperative mechanical ventilation can serve as a meaningful end point for clinical trials, and successful ventilator weaning and extubation in the ICU is an important step of promoting postoperative recovery for all cardiac surgery patients. Furthermore, the poor prognosis of patients with delayed extubation also emphasized the importance of early identification in patients at risk. Although some risk factors in this model are similar to those reported in previous studies (8–11), our study differs from the perspective of statistical methods and has several advantages over previous conventional multivariable regression analysis. With the use of robust statistical modeling techniques, DCA and clinical impact curves, we present an effective, contemporary, yet simple predictive model of delayed extubation after cardiac surgery. As for the problem of multicollinearity between clinical variables, LASSO regression analysis in this study could minimize this problem and provide more accurate results. ROC curve, calibration plot and DCA and clinical impact curve were used for internal validation to ensure the stability and accuracy of this model.
Existing models of delayed extubation provide important findings about the risk factors associated with delayed extubation after cardiac surgery, including previous cardiac surgery, ejection fraction and CPB time (8–11). However, previous models lacked grade information on important variables such as age, type of surgery, and duration of surgery, which limited the generalizability and application value of these models. Moreover, until now, risk models able to risk stratify patients of delayed extubation after cardiac surgery appear to be less common in the literature. Risk stratification of predictive models is critical and helpful for clinicians to be more prepared and confident in the postoperative treatment of medium-risk and high-risk patients, as well as reasonable allocation of medical resources. In contrast, our model fills these gaps and highlights clinically relevant risk factors for delayed extubation, which greatly facilitates the clinician to better understand individual risk and decision-making.
Currently, there is no common threshold for the ideal extubation time after cardiac surgery, previous studies accepted very different time thresholds, ranging from 6 h to 21 days postoperatively (17). In the results of the analysis of the world literature, most investigators accepted a threshold of 24 or 48 h for extubation time to determine prolonged mechanical ventilation (18). Although the Society of Thoracic Surgeons (STS) defines prolonged mechanical ventilation as more than 24 h, this applies only to patient populations who underwent CABG alone. Considering that the study population of this study involved multiple types of cardiac surgery, we used a threshold of 48 h to define the delayed extubation. Due to different definitions and populations, the reported prevalence of delayed extubation varies widely in the literature, ranging from 6 to 12% (8–11). We divided the type and duration of surgery to determine its potential impact on delayed extubation. For this study, 13.6% of adult patients required delayed extubation for ventilatory support during ICU observation. The relatively high rates of delayed extubation may be related to the specific characteristics in our study population such as complex types of surgery. Patients undergoing more than two types of heart surgery tend to require longer duration of surgery and CPB time, and may be more unstable and at greater risk during surgery. In addition to longer mechanical ventilation and length of stay in the ICU, higher occurrence of major adverse cardiac or cerebrovascular events were also observed in patients with combined cardiac surgery (15, 19). We observed that nearly half of the patients undergoing aortic dissection surgery were mechanically ventilated for more than 48 h after surgery, with a similar incidence to previous reports in the literature (28.9–48.5%) (20–22). Our findings demonstrated that the risk of delayed extubation in patients undergoing aortic surgery was doubled compared to isolated CABG or valve surgery, which was similar to that of patients undergoing combined cardiac surgery.
In addition to common cardiac risk factors in previous models such as left ventricular ejection fraction and history of cardiac surgery, our model included patient-specific factors like age and BMI. Age-related changes in cardiac structure and function are well recognized (23), as are substantial changes in lung function (24). Risk of delayed extubation with each age group was explored by grouping patients by age. The results showed that the risk for delayed extubation after cardiac surgery increased significantly with age, especially after the age of 60 years. Reduced lung compliance and functional residual capacity are major causes of impaired lung function in obese patients, which is exacerbated by general anesthesia and mechanical ventilation (25). According to the Chinese guide (26), individuals with BMI ≥ 28 kg/m2 were considered obesity. Corresponding scores were assigned to each group to facilitate visualization of risks.
Activation of systemic inflammatory mediators induced by CPB increases the susceptibility to lung injury (16). Prolonged CPB time has repeatedly been reported as a risk factor for delayed extubation in the literature. As expected, in our model, the risk of delayed postoperative extubation increased ~2-fold when the duration of CPB exceeded 120 min. Hessels et al. (8) reported that Patients who received more than 210 min of CPB were nearly 4 times more likely to suffer delayed postoperative extubation. Obviously, the risk was related to the duration and complexity of the operation.
Patients undergoing emergency cardiac surgery are often in unstable condition and even life-threatening. Incomplete preoperative evaluation and preparation exposes patients to unknown risks, making it necessary for clinicians to carefully consider the risks before making extubation decisions. Meanwhile, the use of intra- or post-operative IABP means poorer cardiac function. Therefore, the need for longer ventilation times is usually a consequence of cardiopulmonary dysfunction or slower recovery after cardiac surgery.
Impaired preoperative renal function was classified as a presence eGFR < 60 mL/min/1.73 m2 (27). In the present study, patients with renal impairment had twice the risk of delayed extubation as those with normal renal function. Intraoperative exposure to CPB and concomitant use of multiple drugs further increase the total burden of renal metabolism (28), which may slow the excretion of drugs and their metabolites and early recovery from anesthesia.
Although these predictors reported here have clinical implications, there are some limitations to our work that require further study. First, all patients were from a single tertiary care center, which could limit the translation of our results to other centers. Second, due to the problem of missing data inherent in retrospective studies, a relatively small sample size was used in our study. Although our score model performed well in terms of discrimination and calibration, external populations are needed to determine whether our results are representative of those from other centers. Future prospective studies are needed to replicate our results and to determine the clinical implications of these retrospective findings. Third, detailed intraoperative data on mechanical ventilation, vasopressors and anesthetics were not included in our analysis.
Conclusions
In this study, we observed that delayed extubation after cardiac surgery was associated with poorer postoperative recovery and higher risk of death. Using 10 easily obtainable and objective variables, we developed and validated a prediction score model to identify population at high risk for delayed extubation and stratify risk. We believe that this model will be a useful predictive tool to support informed clinical decision making and risk discussion.
Data availability statement
The raw data supporting the conclusions of this article will be made available by the authors, without undue reservation.
Ethics statement
The studies involving human participants were reviewed and approved by Ethics Committee of Union Hospital affiliated to Tongji Medical College of Huazhong University of Science and Technology. Written informed consent for participation was not required for this study in accordance with the national legislation and the institutional requirements.
Author contributions
QW, WX, JL, and XL: study concept and design. YB and LC: acquisition and collection of data. XL and JL: analyzed the data. ZX, YW, JL, and XL: interpretation of data. XL: drafted the manuscript. QW and WX: manuscript revision and editing. All authors: final approval of manuscript.
Funding
This study was supported by the National Key Research and Development Program of China (Grant No. 2018YFC2001903) and the National Natural Science Foundation of China (Grant Nos. 81873952 and 81901948).
Acknowledgments
We express our appreciation to the department members for their great support and assistance.
Conflict of interest
The authors declare that the research was conducted in the absence of any commercial or financial relationships that could be construed as a potential conflict of interest.
Publisher's note
All claims expressed in this article are solely those of the authors and do not necessarily represent those of their affiliated organizations, or those of the publisher, the editors and the reviewers. Any product that may be evaluated in this article, or claim that may be made by its manufacturer, is not guaranteed or endorsed by the publisher.
Supplementary material
The Supplementary Material for this article can be found online at: https://www.frontiersin.org/articles/10.3389/fcvm.2022.1002768/full#supplementary-material
References
1. Udzik J, Sienkiewicz S, Biskupski A, Szylinska A, Kowalska Z, Biskupski P. Cardiac complications following cardiac surgery procedures. J Clin Med. (2020) 9:3347. doi: 10.3390/jcm9103347
2. Herlihy JP, Koch SM, Jackson R, Nora H. Course of weaning from prolonged mechanical ventilation after cardiac surgery. Tex Heart Inst J. (2006) 33:122–9.
3. Liu LL, Gropper MA. Respiratory and hemodynamic management after cardiac surgery. Curr Treat Opt Cardiovasc Med. (2002) 4:161–9. doi: 10.1007/s11936-002-0036-y
4. Kotfis K, Szylinska A, Listewnik M, Lechowicz K, Kosiorowska M, Drozdzal S, et al. Balancing intubation time with postoperative risk in cardiac surgery patients: a retrospective cohort analysis. Ther Clin Risk Manag. (2018) 14:2203–12. doi: 10.2147/TCRM.S182333
5. Heringlake M, Nowak Y, Schon J, Trautmann J, Berggreen AE, Charitos EI, et al. Postoperative intubation time is associated with acute kidney injury in cardiac surgical patients. Crit Care. (2014) 18:547. doi: 10.1186/s13054-014-0547-4
6. Suarez-Pierre A, Fraser CD, Zhou X, Crawford TC, Lui C, Metkus TS, et al. Predictors of operative mortality among cardiac surgery patients with prolonged ventilation. J Card Surg. (2019) 34:759–66. doi: 10.1111/jocs.14118
7. Fernandez-Zamora MD, Gordillo-Brenes A, Banderas-Bravo E, Arboleda-Sanchez JA, Hinojosa-Perez R, Aguilar-Alonso E, et al. Prolonged mechanical ventilation as a predictor of mortality after cardiac surgery. Respir Care. (2018) 63:550–7. doi: 10.4187/respcare.04915
8. Hessels L, Coulson TG, Seevanayagam S, Young P, Pilcher D, Marhoon N, et al. Development and validation of a score to identify cardiac surgery patients at high risk of prolonged mechanical ventilation. J Cardiothorac Vasc Anesth. (2019) 33:2709–16. doi: 10.1053/j.jvca.2019.03.009
9. Totonchi Z, Baazm F, Chitsazan M, Seifi S, Chitsazan M. Predictors of prolonged mechanical ventilation after open heart surgery. J Cardiovasc Thorac Res. (2014) 6:211–6. doi: 10.15171/jcvtr.2014.014
10. Sharma V, Rao V, Manlhiot C, Boruvka A, Fremes S, Wasowicz M, et al. derived and validated score to predict prolonged mechanical ventilation in patients undergoing cardiac surgery. J Thorac Cardiovasc Surg. (2017) 153:108–15. doi: 10.1016/j.jtcvs.2016.08.020
11. Michaud L, Dureau P, Kerleroux B, Charfeddine A, Regan M, Constantin JM, et al. Development and validation of a predictive score for prolonged mechanical ventilation after cardiac surgery. J Cardiothorac Vasc Anesth. (2022) 36:825–32. doi: 10.1053/j.jvca.2021.07.016
12. Moons KG, Altman DG, Reitsma JB, Ioannidis JP, Macaskill P, Steyerberg EW, et al. Transparent reporting of a multivariable prediction model for individual prognosis or diagnosis (tripod): explanation and elaboration. Ann Intern Med. (2015) 162:W1–73. doi: 10.7326/M14-0698
13. Jammer I, Wickboldt N, Sander M, Smith A, Schultz MJ, Pelosi P, et al. Standards for definitions and use of outcome measures for clinical effectiveness research in perioperative medicine: European perioperative clinical outcome (epco) definitions: a statement from the esa-esicm joint taskforce on perioperative outcome measures. Eur J Anaesthesiol. (2015) 32:88–105. doi: 10.1097/EJA.0000000000000118
14. Pavlou M, Ambler G, Seaman S, De Iorio M, Omar RZ. Review and evaluation of penalised regression methods for risk prediction in low-dimensional data with few events. Stat Med. (2016) 35:1159–77. doi: 10.1002/sim.6782
15. Fellahi JL, Hedoire F, Le Manach Y, Monier E, Guillou L, Riou B. Determination of the threshold of cardiac troponin i associated with an adverse postoperative outcome after cardiac surgery: a comparative study between coronary artery bypass graft, valve surgery, and combined cardiac surgery. Crit Care. (2007) 11:R106. doi: 10.1186/cc6126
16. Apostolakis E, Filos KS, Koletsis E, Dougenis D. Lung dysfunction following cardiopulmonary bypass. J Card Surg. (2010) 25:47–55. doi: 10.1111/j.1540-8191.2009.00823.x
17. Rose L, McGinlay M, Amin R, Burns KE, Connolly B, Hart N, et al. Variation in definition of prolonged mechanical ventilation. Respir Care. (2017) 62:1324–32. doi: 10.4187/respcare.05485
18. MacIntyre NR, Epstein SK, Carson S, Scheinhorn D, Christopher K, Muldoon S. Management of patients requiring prolonged mechanical ventilation: report of a namdrc consensus conference. Chest. (2005) 128:3937–54. doi: 10.1378/chest.128.6.3937
19. Smith PK, Puskas JD, Ascheim DD, Voisine P, Gelijns AC, Moskowitz AJ, et al. Surgical treatment of moderate ischemic mitral regurgitation. N Engl J Med. (2014) 371:2178–88. doi: 10.1056/NEJMoa1410490
20. Maisat W, Siriratwarangkul S, Charoensri A, Wongkornrat W, Lapmahapaisan S. Perioperative risk factors for delayed extubation after acute type a aortic dissection surgery. J Thorac Dis. (2020) 12:4796–804. doi: 10.21037/jtd-20-742
21. Jin M, Ma WG, Liu S, Zhu J, Sun L, Lu J, et al. Predictors of prolonged mechanical ventilation in adults after acute type-a aortic dissection repair. J Cardiothorac Vasc Anesth. (2017) 31:1580–7. doi: 10.1053/j.jvca.2017.03.036
22. Kimura N, Tanaka M, Kawahito K, Sanui M, Yamaguchi A, Ino T, et al. Risk factors for prolonged mechanical ventilation following surgery for acute type a aortic dissection. Circ J. (2008) 72:1751–7. doi: 10.1253/circj.CJ-08-0306
23. Dai DF, Rabinovitch PS. Cardiac aging in mice and humans: the role of mitochondrial oxidative stress. Trends Cardiovasc Med. (2009) 19:213–20. doi: 10.1016/j.tcm.2009.12.004
24. Vaz FC, Enright PL, McAvay G, Van Ness PH, Gill TM. Frailty and respiratory impairment in older persons. Am J Med. (2012) 125:79–86. doi: 10.1016/j.amjmed.2011.06.024
25. De Jong A, Chanques G, Jaber S. Mechanical ventilation in obese ICU patients: from intubation to extubation. Crit Care. (2017) 21:63. doi: 10.1186/s13054-017-1641-1
26. Chen C, Lu FC. The guidelines for prevention and control of overweight and obesity in Chinese adults. Biomed Environ Sci. (2004) 17(Suppl):1–36.
27. Froissart M, Rossert J, Jacquot C, Paillard M, Houillier P. Predictive performance of the modification of diet in renal disease and cockcroft-gault equations for estimating renal function. J Am Soc Nephrol. (2005) 16:763–73. doi: 10.1681/ASN.2004070549
Keywords: delayed extubation, risk factor, cardiac surgery, risk score, prediction model
Citation: Li X, Liu J, Xu Z, Wang Y, Chen L, Bai Y, Xie W and Wu Q (2022) Early identification of delayed extubation following cardiac surgery: Development and validation of a risk prediction model. Front. Cardiovasc. Med. 9:1002768. doi: 10.3389/fcvm.2022.1002768
Received: 25 July 2022; Accepted: 15 September 2022;
Published: 04 October 2022.
Edited by:
Jinfeng Xu, The University of Hong Kong, Hong Kong SAR, ChinaReviewed by:
Huiwu Han, Xiangya Hospital, Central South University, ChinaZachary Enumah, Johns Hopkins Medicine, United States
Copyright © 2022 Li, Liu, Xu, Wang, Chen, Bai, Xie and Wu. This is an open-access article distributed under the terms of the Creative Commons Attribution License (CC BY). The use, distribution or reproduction in other forums is permitted, provided the original author(s) and the copyright owner(s) are credited and that the original publication in this journal is cited, in accordance with accepted academic practice. No use, distribution or reproduction is permitted which does not comply with these terms.
*Correspondence: Wanli Xie, d2FubGlfeGllQGh1c3QuZWR1LmNu; Qingping Wu, d3FwMTk2OEAxMjYuY29t
†These authors have contributed equally to this work