- The Second Affiliated Hospital, Zhejiang University, Hangzhou, Zhejiang, China
Background: Risk scores for predicting in-hospital major bleeding in patients with acute myocardial infarction (AMI) are rare. The Swedish web-system for the enhancement and development of evidence-based care in heart disease evaluated according to recommended therapies (SWEDEHEART) score (SS), consisting of five common clinical variables, is a novel model for predicting in-hospital major bleeding. External validation of SS has not yet been completed.
Methods and results: A retrospective study recruiting consecutive East Asian patients diagnosed with AMI was conducted in the Second Affiliated Hospital, Zhejiang University. The primary endpoint was the ability of SS to predict in-hospital major bleeding, which was defined as Bleeding Academic Research Consortium (BARC) type 3 or 5 bleeding. To validate SS, the discrimination and calibration were assessed in the overall population and several subgroups. The receiver operating characteristic (ROC) curves and the areas under ROC curves (AUCs) were calculated for discrimination. The calibration of SS was evaluated with the unreliability U test. A total of 2,841 patients diagnosed with AMI during hospitalization were included, and 1.94% (55) of them experienced in-hospital major bleeding events. The AUC of SS for the whole population was only 0.60 [95% confidence interval (CI), 0.52–0.67], without an acceptable calibration (p = 0.001). Meanwhile, the highest AUC (0.72; 95% CI, 0.61–0.82) of SS for the primary endpoint was found in the diabetes subgroup, with an acceptable calibration (p = 0.87).
Conclusion: This external validation study showed that SS failed to exhibit sufficient accuracy in predicting in-hospital major bleeding among East Asian patients with AMI despite demonstrating acceptable performance in the diabetic subgroup of patients. Studies to uncover optimal prediction tools for in-hospital major bleeding risk in AMI are urgently warranted.
1. Introduction
Although the ischemic risk of acute coronary syndrome (ACS) has been considerably reduced with the development of percutaneous coronary intervention (PCI) and antithrombotic therapy over the last decade, bleeding has always been a common and non-neglectable complication associated with poor prognosis (1), especially in-hospital bleeding under the administration of intensive antiplatelet and anticoagulant medications (2–4). Identifying specific populations at high risk for bleeding is particularly important during daily clinical practice, and current guidelines recommend using the Can Rapid Risk Stratification of Unstable Angina Patients Suppress Adverse Outcomes with Early Implementation of the American College of Cardiology/American Heart Association Guidelines (CRUSADE) score as an acceptable model to deal with this issue (5, 6). However, the CRUSADE score is not suitable for the application among conservatively treated patients and is substantially limited due to the widespread use of radial access during PCI (6). Novel risk models for predicting in-hospital bleeding are rare.
The Swedish web-system for the enhancement and development of evidence-based care in heart disease evaluated according to recommended therapies (SWEDEHEART) score (SS), generated from the large-scale SWEDEHEART registry, is a novel and convenient model including only five clinical variables to predict in-hospital major bleeding following acute myocardial infarction (AMI) (7). Internal–external cross-validation showed that SS had better performance than the CRUSADE score in assessing the registry-defined in-hospital major bleeding risk (C-index, 0.80 vs. 0.72) among patients irrespective of a history of PCI (7). Both its favorable discriminative ability and good convenience have qualified SS to be a promising prediction model. However, the derivation cohort of SS included AMI patients from Sweden, and no external validation with a different population has been conducted to further confirm the performance of this model. East Asian patients with AMI, who are considered to have a relatively increased risk of bleeding, should be paid more attention to risk assessment (8, 9). Therefore, we aimed to externally validate SS for predicting in-hospital major bleeding in East Asian AMI patients in order to examine its transportability.
2. Materials and methods
2.1. Patient population
This was a retrospective cohort study that enrolled consecutive patients diagnosed with AMI in the Department of Cardiology at the Second Affiliated Hospital, Zhejiang University, Hangzhou, China, between June 2018 and June 2021 irrespective of a history of PCI. Major exclusion criteria included being transferred to undergo coronary artery bypass graft surgery during hospitalization and refusing to participate in the study. AMI was defined according to the third universal concept of infarction (10). The demographic data, medical history, laboratory tests, and in-hospital complications (including bleeding events) were strictly recorded in a computer database. The strategy of PCI procedures and periprocedural antithrombotic medications was chosen at physicians’ discretion according to standard guidelines and also recorded. This retrospective study was performed in accordance with the Declaration of Helsinki and was approved by the Ethics Committee of the Second Affiliated Hospital, Zhejiang University (reference no. EC-20220412-1020). All patients involved in this study provided written informed consent.
2.2. Endpoints
The primary study endpoint was the predictive accuracy of SS for in-hospital major bleeding in AMI patients. Bleeding events that occurred after PCI during hospitalization were judged by a pair of independent cardiologists. Localization of bleeds and changes in hemoglobin (Hb) values were collected from the hospital information system. In-hospital major bleeding was defined as Bleeding Academic Research Consortium (BARC) type 3 or 5 bleeding, which is the most prevalently used bleeding criterion (11).
2.3. Statistical methods
Continuous data are presented as mean ± standard deviation values and were compared using Student’s t test if normally distributed or as median and interquartile range (IQR) values and compared by the Wilcoxon rank-sum test if non-parametric. Categorical data are presented as counts and percentages and were compared using Fisher’s exact test.
SWEDEHEART score includes the following five variables: sex, Hb, age, C-reaction protein (CRP) level, and serum creatinine concentration. For patients missing CRP information, a modified SS was also generated (7). For SS calculations, the estimated major bleeding probability was computed as 1/[1 + exp(−bX)], where “bX” varied according to whether CRP data were available or missing (7).
To validate SS, its discrimination was assessed in both the overall and the subgroup datasets. Subgroups included those established according to age (<65 or ≥65 years), the presence or absence of diabetes mellitus (DM), sex, the presence or absence of heart failure (HF), a history of PCI, and the occurrence of ST-segment elevation MI (STEMI) or non-STEMI (NSTEMI). By taking the predicted probability of major bleeding as the test variable and the observed proportion of major bleeding as the state variable, the receiver operating characteristic (ROC) curve was drawn, and the area under the ROC curve (AUC) was calculated (12, 13). Similar to the discrimination, the calibration was also assessed in both the overall and the subgroup datasets. The calibration curve of SS for major bleeding according to the predicted probabilities and the observed proportions was drawn (14), and the calibration of SS was evaluated with an unreliability test (15). A decision curve was plotted (16), and decision curve analysis was performed to judge the clinical use of SS by quantifying the net benefits with different probability thresholds in the overall dataset (17, 18). All p values were two-tailed, and p < 0.05 was considered to be statistically significant. All statistical analyses were conducted using Stata/SE version 15.0 (StataCorp LLC, College Station, TX, USA) and R version 3.3.1 (R Foundation for Statistical Computing, Vienna, Austria).
3. Results
3.1. Baseline characteristics
A total of 2,841 patients diagnosed with AMI during hospitalization were included in this retrospective study, of whom 2,324 patients (81.8%) received PCI during hospitalization. In-hospital major bleeding occurred in 55 patients (1.94%) (a flowchart is shown in Supplementary Figure 1). All bleeding events were BARC type 3 bleeding; no case of BARC type 5 fatal bleeding was found in this cohort.
The baseline characteristics of patients with and without in-hospital major bleeds are presented in Table 1. The proportion of a previous MI history in the major bleeding group was significantly greater than that in the no major bleeding group (18.2% vs. 9.4%, p = 0.04). Patients in the major bleeding group more often presented with signs or symptoms of HF (30.9% vs. 9.3%, p < 0.001) and atrial fibrillation (7.3% vs. 2.1%, p = 0.03) at admission. During hospitalization, complications such as shock, new-onset atrial fibrillation, cardiopulmonary resuscitation, and death also occurred more frequently in the major bleeding group (p < 0.05 for all). Patients in the major bleeding group had a significantly lower Hb concentration (129 ± 20 g/L vs. 135 ± 20 g/L, p = 0.02) and a significantly higher CRP level [median (IQR), 11.2 (5.4–45.1) mg/L vs. 4.2 (2.0–12.8) mg/L; p < 0.001] compared to those in the no major bleeding group.
3.2. Discrimination of SS
Figure 1 presents the ROCs of SS for in-hospital major bleeding in the whole population and each subgroup. The AUCs and relevant 95% confidence interval (CI) values are presented in Table 2.
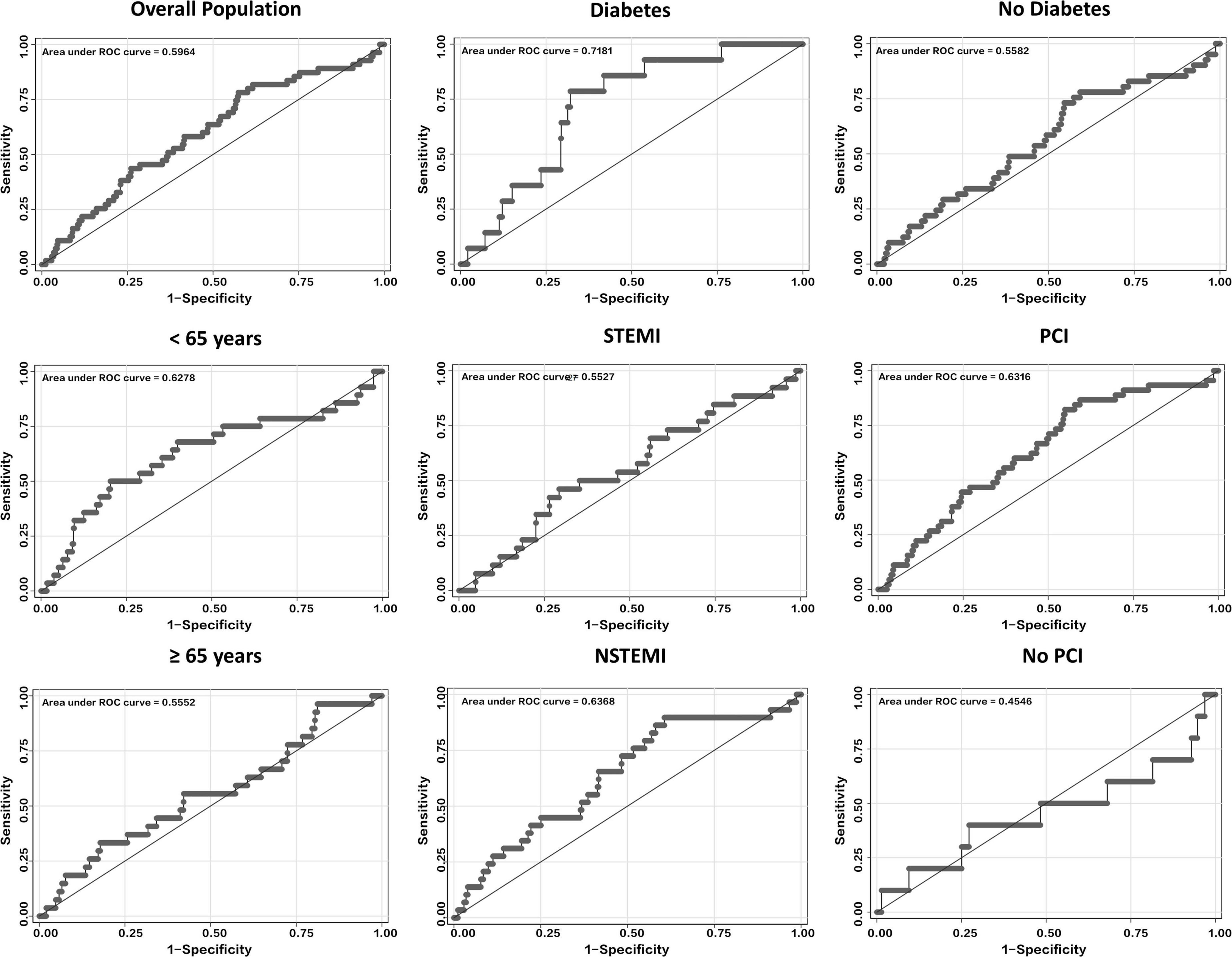
Figure 1. ROCs curves of SS for predicting in-hospital major bleeding in the overall population and several subgroups, including those of age, diabetes, MI type, and PCI. NSTEMI, non-ST-segment elevation myocardial infarction; PCI, percutaneous coronary intervention; ROC, receiver operating characteristics; SS, SWEDEHEART score; STEMI, ST-segment elevation myocardial infarction.
The AUC of SS for in-hospital major bleeding in the whole population was 0.60 (95% CI, 0.52–0.67) (Figure 1 and Table 2). After subgroup analysis, however, the highest AUC of the score for the primary endpoint, 0.72 (95% CI, 0.61–0.82) (Figure 1 and Table 2), was found in the DM subgroup. Meanwhile, in the non-DM subgroup, the AUC of SS was 0.56 (95% CI, 0.47–0.65) (Figure 1 and Table 2). Across the three subgroups of age < 65 years, a history of PCI, and NSTEMI subgroups, the AUCs ranged from 0.63 to 0.64 (Figure 1 and Table 2). Conversely, the AUCs for the age ≥ 65 years, no history of PCI, and STEMI subgroups were all <0.60, with the lowest AUC of SS for in-hospital major bleeding found in the no history of PCI subgroup (0.45; 95% CI, 0.22–0.68) (Figure 1 and Table 2). The AUCs were all <0.60 in subgroups stratified by sex and HF or not (Table 2 and Supplementary Figure 2).
3.3. Calibration of SS
The calibration curves of SS for in-hospital major bleeding in the whole cohort (Figure 2) and subgroups (Figure 2 and Supplementary Figure 3) are shown. For the whole population, SS did not reach a level of acceptable calibration for in-hospital major bleeding (p = 0.001) (Figure 2). An unacceptable degree of calibration was also found in the male sex, STEMI, non-DM, a history of PCI, and HF diagnosis subgroups, indicating that the observed probabilities were insufficient and the estimated probabilities were low (p < 0.05 for all) (Figure 2 and Supplementary Figure 3). However, we confirmed an agreement between observed and predicted in-hospital major bleeding rates in the DM subgroup (p = 0.87) (Figure 2). The NSTEMI, no history of PCI, no HF diagnosis, and female sex subgroups also showed an acceptable calibration of SS for the primary endpoint (p > 0.05 for all) (Figure 2 and Supplementary Figure 3).
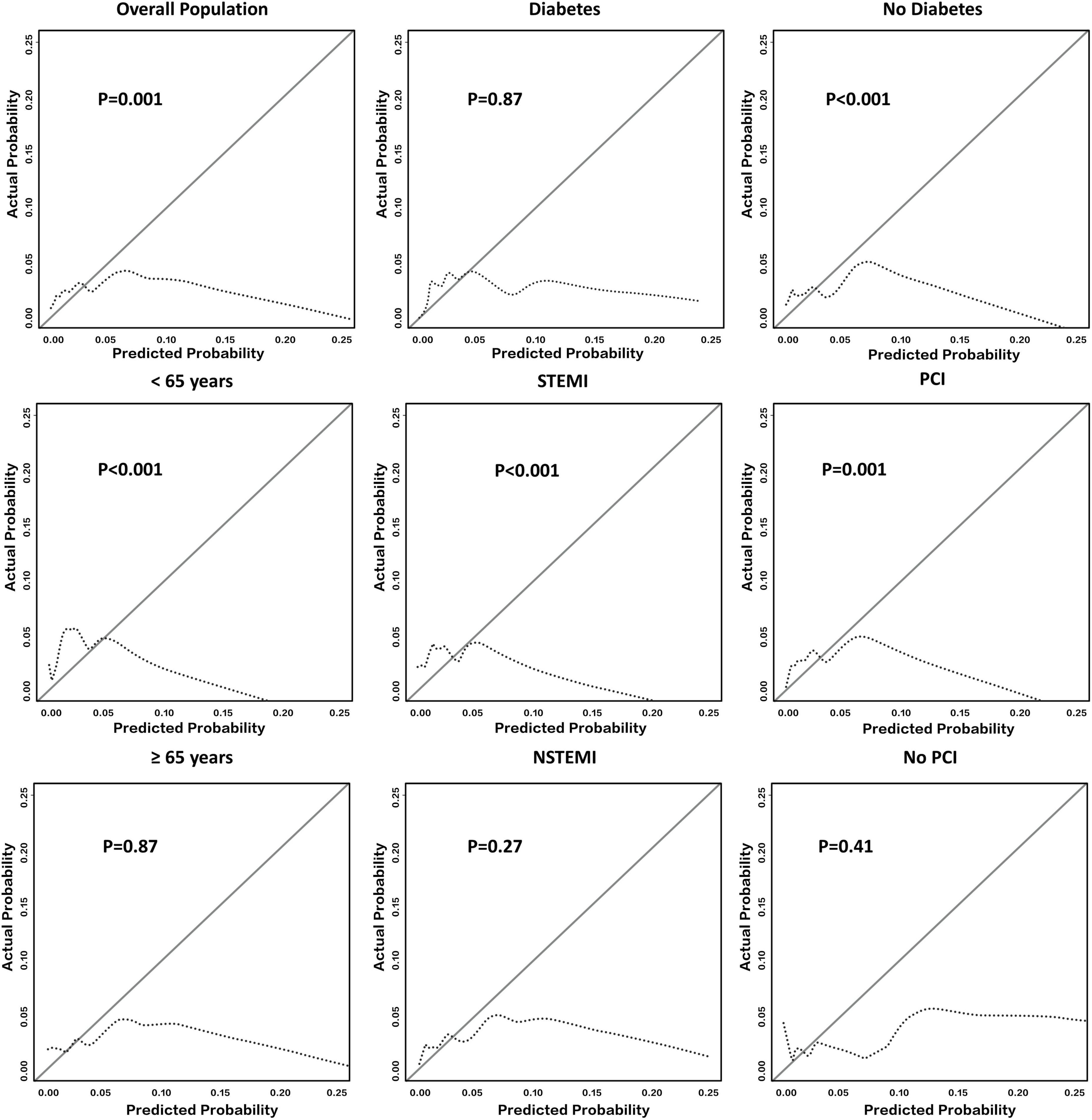
Figure 2. Calibration of SS in all East Asian patients with AMI and their subgroups. It shows the calibration plot of SS for predicting in-hospital major bleeding among the whole East Asian population with AMI and several representative subgroups. Deviations from ideal calibration represent bias in the predicted probability. The figure indicates that the calibration of SS in the overall population was not acceptable (p = 0.001). Note that p > 0.05 represents an acceptable calibration ability among a single subgroup. AMI, acute myocardial infarction; NSTEMI, non-ST-segment elevation myocardial infarction; PCI, percutaneous coronary intervention; SS, SWEDEHEART score; STEMI, ST-segment elevation myocardial infarction.
After evaluating discrimination in combination with calibration, SS did not display an eligible level of performance for predicting the risk of in-hospital major bleeding in the whole population. However, among diabetic patients, both the discrimination and calibration of SS were acceptable for the primary endpoint. Results from the NSTEMI subgroup were also acceptable, but the performance of SS was not acceptable among the other subgroups.
3.4. Clinical utility of SS
Figure 3 presents the decision curve of SS for the primary endpoint, which showed that, if the threshold probability of a patient ranges from 0.4 to 1.3%, the patient would benefit from using SS to predict the in-hospital major bleeding risk (Figure 3). After analysis, 1,085 patients had a threshold probability ranging from 0.4 to 1.3%.
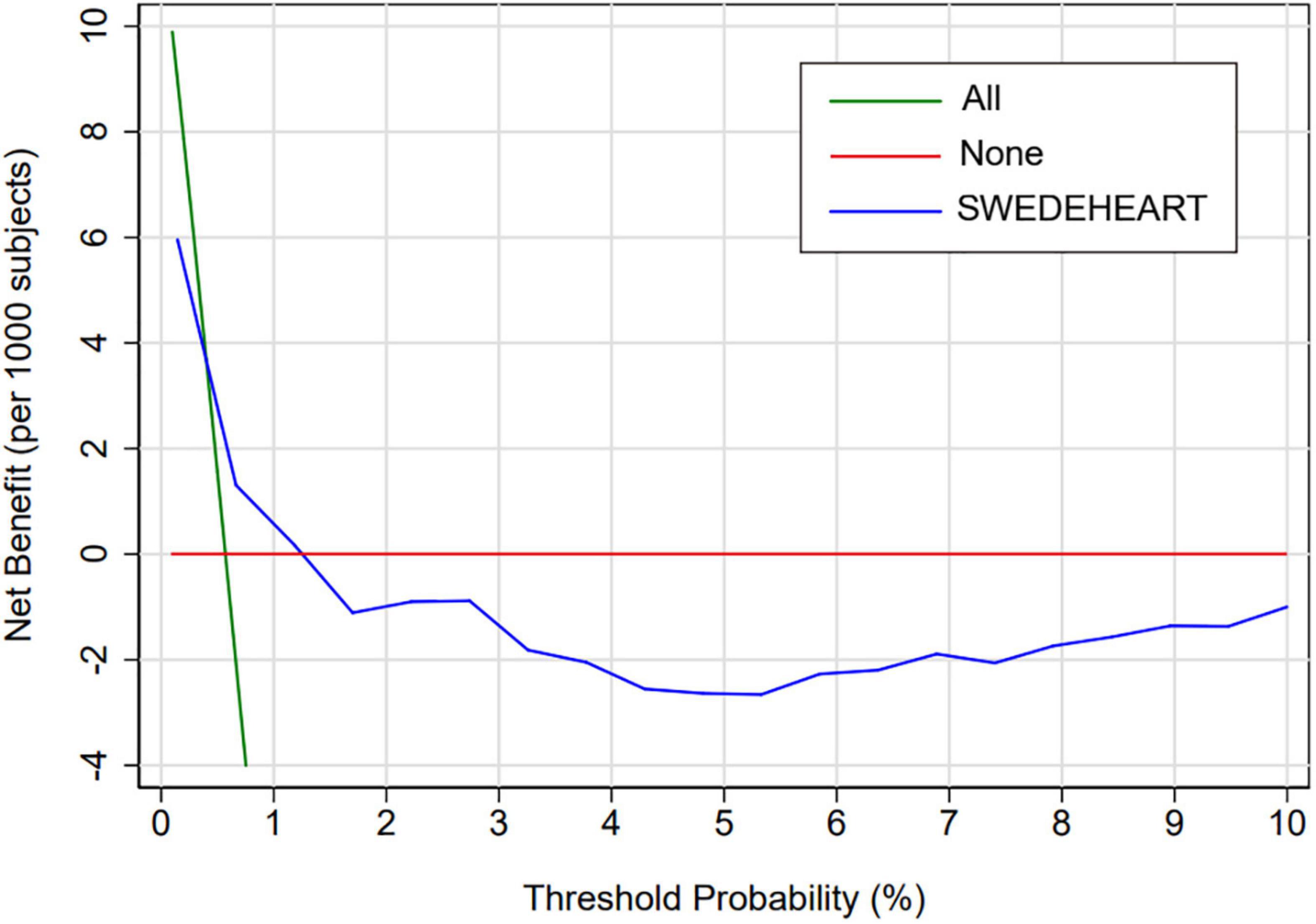
Figure 3. Decision curve analysis of SS among the overall population. Decision curve analysis for SS. The y-axis measures the net benefit. The x-axis measures the threshold probability. The blue line represents SS. The green line represents the assumption that all patients have an absolute risk of bleeding. The red line represents the assumption that no patients have any risk of bleeding. The decision curve shows that, if the threshold probability of a patient ranges from 0.4 to 1.3%, the patient would benefit from using SS to predict the in-hospital major bleeding risk. SS, SWEDEHEART score.
4. Discussion
This retrospective cohort study first externally validated the predictive accuracy of SS, a novel bleeding risk model, for in-hospital major bleeding among East Asian patients with AMI. This study found that SS did not have good accuracy in predicting the in-hospital major bleeding risk among the whole population. SS had an acceptable performance of discrimination and calibration only among diabetic patients with AMI.
Bleeding events, as the most important adverse events associated with antithrombotic therapy and revascularization strategies, are associated with a relatively higher mortality rate in the management of ACS (19, 20). A recently published cohort study from the large-scale SWEDEHEART registry also determined that upper gastrointestinal bleeding was common and independently associated with all-cause death in AMI patients. The balance of ischemic and bleeding risks for patients with ACS is permanently pursued by contemporary cardiologists. Several recent studies have reported that the rate of in-hospital bleeding remains 3–6% in ACS patients, while the present study found that the rate of in-hospital BARC-defined major bleeding was 1.94% (21, 22). Our study and previous studies collectively prove that bleeding events during hospitalization remain worthy of attention. However, risk scores predicting in-hospital major bleeding are still rare; an existing option, the CRUSADE score, due to its intrinsic deficiency, is now recommended only for patients with angiography by current guidelines (6).
SWEDEHEART score was derived and internally validated from a large-scale real-world SWEDEHEART registry including Caucasian patients and is a new model for predicting the risk of in-hospital major bleeding. In a derivation study, SS showed a better predictive accuracy for in-hospital major bleeding compared to the CRUSADE score among European patients with AMI (C-index, 0.80 vs. 0.72) (7). Moreover, SS includes only five common clinical parameters (sex, Hb concentration, age, CRP level, and serum creatinine concentration), making it more convenient than the CRUSADE score (which includes eight parameters). Importantly, the derivation study of SS did not conduct external validation, especially for populations other than Caucasian individuals. Moreover, a judgment of the primary endpoint, in-hospital bleeding, by the study authors was done in accordance with their criterion (readmission due to bleeding), rendering the usefulness of this risk score under universal standardized criteria of bleeding, such as the BARC definition, questionable (11). We, therefore, conducted this retrospective study to externally validate the predictive ability of SS for in-hospital BARC-defined major bleeding (type 3 or 5 bleeding) among East Asian patients with AMI from our site. The East Asian population, which is different from the Caucasian population, is regarded to have a relatively lower ischemic risk but a higher risk of bleeding (referred to as the “East Asian paradox”), and this group deserves to have more attention paid to the bleeding risk (8, 9). However, our results showed that the AUC of SS was only 0.60, and the calibration was also poor, with a great discrepancy between the observed and predicted probabilities (p = 0.001). This outcome failed to identify SS as an acceptable risk score for predicting the risk of major bleeding during hospitalization of East Asian patients.
A subgroup analysis among diabetic patients in our study revealed that SS had acceptable discrimination in predicting in-hospital major bleeding, with an AUC value of 0.72, while also presenting an eligible calibration. However, the predictive ability of SS in diabetic AMI patients was also limited. DM is known to be a risk factor not only for recurrent ischemic events but also bleeding among patients with ACS (11, 23, 24). The CRUSADE score may be considered as a tool for predicting in-hospital bleeding events among ACS patients (5). Therefore, an incremental effect of DM on the predictive accuracy of SS from our findings is theoretically reasonable. Although not suitable for the whole population, SS showed an acceptable predictive ability among diabetic East Asian patients in the present external validation study. Further exploration of SS for evaluating the in-hospital major bleeding risk among AMI patients with DM is needed. In total, this external validation study demonstrated that SS may be an alternative method for evaluating the in-hospital major bleeding risk of AMI patients with DM. Meanwhile, the unacceptable predictive ability of SS in other sub-populations supports the need to uncover optimally pragmatic tools for predicting the bleeding risk.
There are several limitations to this study. First, although it met the minimal requirements according to the TRIPOD guideline (25), the sample size of recruited patients was still too small. A large-scale, real-world study focusing on the East Asian population deserves to be conducted. Second, we did not compare the predictive accuracy between SS and the CRUSADE score due to insufficient data availability, but in the earlier derivation study, this comparison was performed (5). Finally, an incremental exploration of the effects of DM on SS was not performed in our study and requires further investigation.
This retrospective external validation study demonstrated that SS does not perform effectively in predicting in-hospital major bleeding among East Asian patients with AMI. However, an acceptable predictive ability of SS was found in AMI patients with DM. Large-scale studies are needed to further validate the accuracy of SS.
Data availability statement
The raw data supporting the conclusions of this article will be made available by the authors, without undue reservation.
Ethics statement
Written informed consent was obtained from the individual(s) for the publication of any potentially identifiable images or data included in this article.
Author contributions
YL was mainly in charge of conducting this observational study. FL was responsible for writing the manuscript. QW contributed to recruiting subjects during the study period. QG was responsible for statistical analysis. JJ led the study conduct and took on the key role of designing, initiating, and conducting this study. All authors contributed to the article and approved the submitted version.
Acknowledgments
We acknowledged all contributors from The Second Affiliated Hospital of Zhejiang University to this study.
Conflict of interest
The authors declare that the research was conducted in the absence of any commercial or financial relationships that could be construed as a potential conflict of interest.
Publisher’s note
All claims expressed in this article are solely those of the authors and do not necessarily represent those of their affiliated organizations, or those of the publisher, the editors and the reviewers. Any product that may be evaluated in this article, or claim that may be made by its manufacturer, is not guaranteed or endorsed by the publisher.
Supplementary material
The Supplementary Material for this article can be found online at: https://www.frontiersin.org/articles/10.3389/fcvm.2022.1001261/full#supplementary-material
References
1. Sarajlic P, Simonsson M, Jernberg T, Back M, Hofmann R. Incidence, associated outcomes, and predictors of upper gastrointestinal bleeding following acute myocardial infarction: a SWEDEHEART-based nationwide cohort study. Eur Heart J Cardiovasc Pharmacother. (2022) 8:483–91. doi: 10.1093/ehjcvp/pvab059
2. Simonsson M, Wallentin L, Alfredsson J, Erlinge D, Hellstrom Angerud K, Hofmann R, et al. Temporal trends in bleeding events in acute myocardial infarction: insights from the SWEDEHEART registry. Eur Heart J. (2020) 41:833–43. doi: 10.1093/eurheartj/ehz593
3. Ducrocq G, Schulte PJ, Becker RC, Cannon CP, Harrington RA, Held C, et al. Association of spontaneous and procedure-related bleeds with short- and long-term mortality after acute coronary syndromes: an analysis from the PLATO trial. EuroIntervention. (2015) 11:737–45. doi: 10.4244/EIJY14M09_11
4. Giustino G, Mehran R, Dangas GD, Kirtane AJ, Redfors B, Genereux P, et al. Characterization of the average daily ischemic and bleeding risk after primary PCI for STEMI. J Am Coll Cardiol. (2017) 70:1846–57. doi: 10.1016/j.jacc.2017.08.018
5. Subherwal S, Bach RG, Chen AY, Gage BF, Rao SV, Newby LK, et al. Baseline risk of major bleeding in non-ST-segment-elevation myocardial infarction: the CRUSADE (Can Rapid risk stratification of Unstable angina patients Suppress ADverse outcomes with Early implementation of the ACC/AHA Guidelines) Bleeding Score. Circulation. (2009) 119:1873–82.
6. Collet JP, Thiele H, Barbato E, Barthelemy O, Bauersachs J, Bhatt DL, et al. 2020 ESC Guidelines for the management of acute coronary syndromes in patients presenting without persistent ST-segment elevation. Eur Heart J. (2021) 42:1289–367.
7. Simonsson M, Winell H, Olsson H, Szummer K, Alfredsson J, Hall M, et al. Development and validation of a novel risk score for in-hospital major bleeding in acute myocardial infarction:-The SWEDEHEART Score. J Am Heart Assoc. (2019) 8:e012157. doi: 10.1161/JAHA.119.012157
8. Kim HK, Tantry US, Smith SC Jr., Jeong MH, Park SJ, Kim MH, et al. The East Asian paradox: an updated position statement on the challenges to the current antithrombotic strategy in patients with cardiovascular disease. Thromb Haemost. (2021) 121:422–32. doi: 10.1055/s-0040-1718729
9. Levine GN, Jeong YH, Goto S, Anderson JL, Huo Y, Mega JL, et al. Expert consensus document: World Heart Federation expert consensus statement on antiplatelet therapy in East Asian patients with ACS or undergoing PCI. Nat Rev Cardiol. (2014) 11:597–606.
10. Thygesen K, Alpert JS, Jaffe AS, Simoons ML, Chaitman BR, White HD, et al. Third universal definition of myocardial infarction. J Am Coll Cardiol. (2012) 60:1581–98.
11. Mehran R, Rao SV, Bhatt DL, Gibson CM, Caixeta A, Eikelboom J, et al. Standardized bleeding definitions for cardiovascular clinical trials: a consensus report from the Bleeding Academic Research Consortium. Circulation. (2011) 123:2736–47. doi: 10.1161/CIRCULATIONAHA.110.009449
12. Cook NR. Use and misuse of the receiver operating characteristic curve in risk prediction. Circulation. (2007) 115:928–35.
13. Hanley JA, McNeil BJ. The meaning and use of the area under a receiver operating characteristic (ROC) curve. Radiology. (1982) 143:29–36. doi: 10.1148/radiology.143.1.7063747
14. Alba AC, Agoritsas T, Walsh M, Hanna S, Iorio A, Devereaux PJ, et al. Discrimination and calibration of clinical prediction models: users’ guides to the medical literature. JAMA. (2017) 318:1377–84. doi: 10.1001/jama.2017.12126
15. Fenlon C, O’Grady L, Doherty ML, Dunnion J. A discussion of calibration techniques for evaluating binary and categorical predictive models. Prev Vet Med. (2018) 149:107–14. doi: 10.1016/j.prevetmed.2017.11.018
16. Vickers AJ, Elkin EB. Decision curve analysis: a novel method for evaluating prediction models. Med Decis Mak. (2006) 26:565–74. doi: 10.1177/0272989X06295361
17. Fitzgerald M, Saville BR, Lewis RJ. Decision curve analysis. JAMA. (2015) 313:409–10. doi: 10.1001/jama.2015.37
18. Van Calster B, Wynants L, Verbeek JFM, Verbakel JY, Christodoulou E, Vickers AJ, et al. Reporting and interpreting decision curve analysis: a guide for investigators. Eur Urol. (2018) 74:796–804. doi: 10.1016/j.eururo.2018.08.038
19. Valgimigli M, Costa F, Lokhnygina Y, Clare RM, Wallentin L, Moliterno DJ, et al. Trade-off of myocardial infarction vs. bleeding types on mortality after acute coronary syndrome: lessons from the Thrombin Receptor Antagonist for Clinical Event Reduction in Acute Coronary Syndrome (TRACER) randomized trial. Eur Heart J. (2017) 38:804–10. doi: 10.1093/eurheartj/ehw525
20. Manoukian SV, Feit F, Mehran R, Voeltz MD, Ebrahimi R, Hamon M, et al. Impact of major bleeding on 30-day mortality and clinical outcomes in patients with acute coronary syndromes: an analysis from the ACUITY Trial. J Am Coll Cardiol. (2007) 49:1362–8. doi: 10.1016/j.jacc.2007.02.027
21. Dworeck C, Redfors B, Angeras O, Haraldsson I, Odenstedt J, Ioanes D, et al. Association of pretreatment with P2Y12 receptor antagonists preceding percutaneous coronary intervention in non-st-segment elevation acute coronary syndromes with outcomes. JAMA Netw Open. (2020) 3:e2018735. doi: 10.1001/jamanetworkopen.2020.18735
22. Martin-Asenjo R, Gregson J, Rossello X, Van de Werf F, Medina J, Danchin N, et al. Number of antithrombotic drugs used early and in-hospital outcomes in acute coronary syndromes. J Cardiovasc Transl Res. (2021) 14:790–8. doi: 10.1007/s12265-020-10094-5
23. James S, Angiolillo DJ, Cornel JH, Erlinge D, Husted S, Kontny F, et al. Ticagrelor vs. clopidogrel in patients with acute coronary syndromes and diabetes: a substudy from the PLATelet inhibition and patient Outcomes (PLATO) trial. Eur Heart J. (2010) 31:3006–16. doi: 10.1093/eurheartj/ehq325
24. Rivas Rios JR, Franchi F, Rollini F, Angiolillo DJ. Diabetes and antiplatelet therapy: from bench to bedside. Cardiovasc Diagn Ther. (2018) 8:594–609. doi: 10.21037/cdt.2018.05.09
Keywords: SWEDEHEART score, in-hospital major bleeding, AMI, East Asian, diabetes
Citation: Liu Y, Lv F, Wei Q, Gao Q and Jiang J (2023) External validation of the SWEDEHEART score for predicting in-hospital major bleeding among East Asian patients with acute myocardial infarction. Front. Cardiovasc. Med. 9:1001261. doi: 10.3389/fcvm.2022.1001261
Received: 23 July 2022; Accepted: 19 December 2022;
Published: 11 January 2023.
Edited by:
Ayman Elbadawi, University of Texas Medical Branch at Galveston, United StatesReviewed by:
Philip Sarajlic, Karolinska Institutet, SwedenAldina Pivodic, University of Gothenburg, Sweden
Copyright © 2023 Liu, Lv, Wei, Gao and Jiang. This is an open-access article distributed under the terms of the Creative Commons Attribution License (CC BY). The use, distribution or reproduction in other forums is permitted, provided the original author(s) and the copyright owner(s) are credited and that the original publication in this journal is cited, in accordance with accepted academic practice. No use, distribution or reproduction is permitted which does not comply with these terms.
*Correspondence: Jun Jiang, amlhbmctanVuQHpqdS5lZHUuY24=