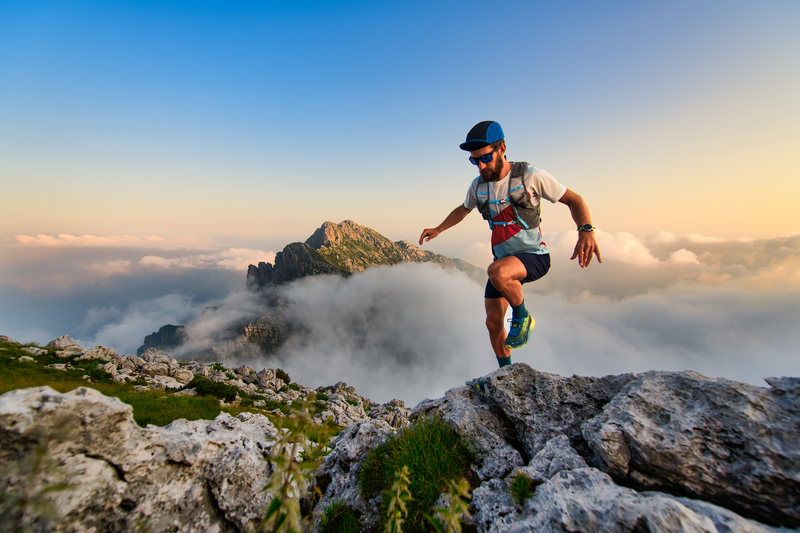
94% of researchers rate our articles as excellent or good
Learn more about the work of our research integrity team to safeguard the quality of each article we publish.
Find out more
ORIGINAL RESEARCH article
Front. Cardiovasc. Med. , 11 November 2021
Sec. Cardiovascular Genetics and Systems Medicine
Volume 8 - 2021 | https://doi.org/10.3389/fcvm.2021.735136
This article is part of the Research Topic Genetic Architecture of Quantitative Cardiovascular Imaging and Electrocardiographic Traits View all 8 articles
Major depressive disorder (MDD) is phenotypically associated with cardiovascular diseases (CVD). We aim to investigate mechanisms underlying relationships between MDD and CVD in the context of shared genetic variations. Polygenic overlap analysis was used to test genetic correlation and to analyze shared genetic variations between MDD and seven cardiovascular outcomes (coronary artery disease (CAD), heart failure, atrial fibrillation, stroke, systolic blood pressure, diastolic blood pressure, and pulse pressure measurement). Mendelian randomization analysis was used to uncover causal relationships between MDD and cardiovascular traits. By cross-trait meta-analysis, we identified a set of genomic loci shared between the traits of MDD and stroke. Putative causal genes for MDD and stroke were prioritized by fine-mapping of transcriptome-wide associations. Polygenic overlap analysis pointed toward substantial genetic variation overlap between MDD and CVD. Mendelian randomization analysis indicated that genetic liability to MDD has a causal effect on CAD and stroke. Comparison of genome-wide genes shared by MDD and CVD suggests 20q12 as a pleiotropic region conferring risk for both MDD and CVD. Cross-trait meta-analyses and fine-mapping of transcriptome-wide association signals identified novel risk genes for MDD and stroke, including RPL31P12, BORSC7, PNPT11, and PGF. Many genetic variations associated with MDD and CVD outcomes are shared, thus, pointing that genetic liability to MDD may also confer risk for stroke and CAD. Presented results shed light on mechanistic connections between MDD and CVD phenotypes.
Collectively, mental disorders and cardiovascular diseases (CVD) account for a large proportion of the total disability and morbidity worldwide (1, 2). Major depressive disorder (MDD), commonly referred to as depression, is characterized by the persistence of low mood. MDD is the most prevalent mental disorder and is accompanied by considerable morbidity, mortality, and a high risk of suicide (3). At some point during the lifetime, it affects 1 out of 5 adults (4). Major forms of CVD include hypertension, coronary heart disease, heart failure, stroke, and atrial fibrillation. High-rate of co-morbidity between depression and CVD is well-acknowledged; patients with depression are more likely to develop CVD, and patients with CVD have higher depression scores than the general population (5). Among patients with CVD, depression is a major contributor to increased healthcare cost, mortality, and reduced quality of life (6, 7), and is also considered an independent risk factor for major adverse cardiovascular events (8). Specifically in coronary heart disease patients, the prevalence of depression is reported at 15–23% (9).
A prevailing measure of quantifying the genetic relationship between two traits is a genetic correlation coefficient, with its sign indicating the direction of the shared genetic effect. However, when dealing with mixtures of effect directions across shared genetic variants, the genetic correlation analyses may be underpowered (10). Polygenic overlaps were recently proposed to measure the fraction of genetic variants causally associated with both traits over the total number of causal variants across a pair of traits involved (10).
In previous studies, MDD has been reported to be genetically correlated with coronary artery disease (CAD) (11). Nevertheless, whether these associations are causal remains to be seen. Mendelian randomization (MR) approach tests for causative association between an exposure and an outcome by utilizing genetic variants as instrumental variables (12, 13). Several frameworks have been proposed for MR analysis, including MR-Egger methods (14). Recently, a powerful GSMR (Generalized Summary-data-based Mendelian Randomization) suit was developed to account for linkage disequilibrium (LD) by leveraging power from multiple genetic variants (15). The GSMR framework is increasingly employed in recent analyses (16–20), with reports of the causal effects of MDD on small vessel stroke, ischemic heart disease, and CAD already available (21–23).
In this study, we evaluated genetic correlation and polygenic overlap between MDD and eight cardiovascular conditions and reported their causal associations. To achieve this, a multi-SNP MR analysis was run on summary GWAS datasets. Across MDD and CVD, pleiotropic genes were extracted by comparing genome-wide genes reported for each trait. Then, in cross-trait meta-analyses, pleiotropic genomic loci shared between MDD and stroke were identified, followed by prioritizing putative risk genes by leveraging a multi-tissue eQTL database.
The summary results of GWAS of MDD (20) and seven cardiovascular conditions—CAD (24), heart failure (25), atrial fibrillation (26), stroke (27), systolic blood pressure (28), diastolic blood pressure (28), and pulse pressure measurement (28)—were used for the analyses. The summary result of GWAS of CVD (29) was used in the validation stage. The CVD dataset included a mixture of multiple cardiovascular diseases recruited by the UKB (29). Participants from these datasets were either of European origins (for traits of MDD, stroke, heart failure, CVD, and blood pressure) or mainly of European origins (for atrial fibrillation and CAD). Condition-specific sample sizes have ranged from 332,477 to 977,323. Each SNP was analyzed across pairs of datasets after exclusion of all SNPs with conflicting alleles, and effect harmonization. Detailed information on the datasets included in this study is summarized in Table 1 and Supplementary File.
GWAS summary results were utilized to extract the genetic correlation of MDD with cardiovascular conditions using LD score regression software (LDSC, v1.0.1) (30, 31). Polygenic overlaps were analyzed by MiXeR v1.2 using default parameters (10). The MiXeR pipeline evaluates the number of shared and trait-specific causal variants between two traits, while accounting for effects of LD structure, minor allele frequency (MAF), sample size, cryptic relationships, and sample overlap. The total number of causal variants was 22.6% of the total estimate, which accounts for 90% of SNP heritability for each trait.
We examined causal effects between MDD and the seven cardiovascular conditions, namely, CAD, heart failure, atrial fibrillation, stroke, systolic blood pressure, diastolic blood pressure, and pulse pressure measurement. GSMR v1.0.9 was used to infer bidirectional causal associations between MDD and the cardiovascular conditions, with causal effects of cardiovascular conditions on MDD being called reverse Mendelian randomization (15). Instrumental variants were selected based on default P ≤ 5 × 10−8. When the threshold was surpassed by <10 SNPs, a P-value threshold of 1 × 10−5 was used. As pleiotropy is known to serve as a potential source of bias and, therefore, an inflated estimation in an MR analysis (32), we used the HEIDI-outlier approach, which detects and eliminates genetic instruments with apparent pleiotropic effects on both the risk factors and the disease (15, 33). Multiple tests were corrected by FDR, with significant causal association detected at FDR < 0.05. A detailed description of the MR is provided in the Supplementary Methods section.
GWAS results were obtained for MDD and four types of CVD from the GWAS Catalog database (34). For stroke, we combined the following labels: stroke as such, large artery stroke, small vessel stroke, cardioembolic stroke, and ischemic stroke. Analysis of gene overlaps among the five traits was conducted using the R package SuperExactTest (35), with the total gene number in the genome being set as 30,000.
Given that MDD has the closest relationship with stroke among the CVD, we performed a cross-trait meta-analysis of the MDD and the stroke using the subset-based fixed-effects method ASSET (version 2.4.0) (36). The meta-analysis pools the effect of a given SNP across K studies, weighting the effects by the size of the study under the default parameters. After subset-based meta-analysis, SNPs with P-values lower than 5 × 10−8 were considered statistically significant. FUMA was used for functional annotation and gene-mapping of variants and identify LD-independent genomic regions in the meta-analysis result (37). Enrichment of the shared genes in the GWAS catalog reported categories was calculated using FUMA (37). Gene property analysis for tissue specificity was performed by FUMA. To ensure that sample overlap did not contribute to inflated estimates of genetic overlap between MDD and stroke, λmeta statistics were calculated (38). The λmeta is a statistic that uses effect size concordance to detect sample overlap or heterogeneity. Under the null hypothesis, λmeta = 1 when the pair of cohorts are completely independent. When there are overlapping samples, λmeta <1. When there is heterogeneity between datasets, the expectation is λmeta > 1. In most GWAS meta-analyses, λmeta is likely to be slightly larger than 1 due to unknown heterogeneity.
To prioritize putatively causal genes, we used fine-mapping of causal gene sets (FOCUS v0.6.10) (39) to the meta-analysis MDD and stroke results in three relevant tissues, including the brain, whole blood, and heart. FOCUS models predict expression correlations and assign a posterior inclusion probability (PIP) for genes at each transcriptome-wide association study (TWAS) region and relevant tissue types. A multi-tissue eQTL reference weight database was employed, and LD information from LDSC was used as reference. Multiple testing corrections were used to account for all gene–tissue pairs using Benjamini–Hochberg adjusted TWAS P-values (FDR < 0.05).
Genetic correlation analyses indicated that MDD has a significant genetic correlation with CAD, heart failure, atrial fibrillation, and pulse pressure (Table 2). Polygenic overlap analysis indicated that 15.8 thousand variants causally influence MDD, while CVD was associated with much smaller numbers of causal variants, ranging from 0.5 thousand for the atrial fibrillation to 2.8 thousand for heart failure. Each of the tested CVD or cardiovascular measurements has shared a substantial set of causal variants with that of MDD (Figure 1A).
Figure 1. Shared causal variants and causal effects between MDD and CVD. MDD, major depressive disorder; HF, heart failure; AF, atrial fibrillation; CAD, coronary artery disease. (A) Venn diagrams of unique and shared causal variants between major depressive disorder and cardiovascular diseases. The numbers indicate the estimated quantity of causal variants (in thousands) per component, explaining 90% of SNP heritability in each phenotype. The size of the circles reflects the degree of polygenicity. (B) Causal effects of MDD on cardiovascular diseases. The dotted lines denote effect sizes (bxy). (C) Overlapped genes between major depressive disorder and cardiovascular disease from GWAS-catalog. The matrix of solid and empty circles at the bottom illustrates the “presence” (solid green) or “absence” (empty) of the gene sets in each intersection. The numbers to the right of the matrix are set sizes. The colored bars on the top of the matrix represent the intersection sizes with the color intensity showing the P-value significance. (D) Pleiotropic genes shared by MDD and CVD. (E) Mechanisms underlying associations between MDD and CVD. (F) Gene property analysis for tissue specificity in general GTEx tissues. (G) Fine-mapping of TWAS hits within 14:72890537-14:76444767 in heart_left_ventricle. Transcriptome-wide association signal indicating the strength of predicted expression associated with the trait.
MDD confers a causal effect on CAD, stroke, and pulse pressure (Table 2, Figure 1B). Positive causal effects of MDD on stroke (bxy = 0.19) were the largest among the cardiovascular conditions profiled. Of note, causal effects of MDD on pulse pressure were negative (bxy = −0.56), indicating that liability to MDD may result in decreased pulse pressure. However, in general, we show that cardiovascular conditions do not confer a causal effect on MDD.
In the validation stage, we examined the genetic correlation and causal associations between MDD and CVD. Our results indicate that MDD has a significant genetic correlation with CVD (rg = 0.357, s.e. = 0.056, P = 1.79 × 10−10). Genetic liability to MDD confers a causal effect on CVD (bxy = 0.26, s.e. = 0.10, P = 9.84 × 10−3), while genetic liability to CVD confers a causal effect on MDD (bxy = 0.07, s.e. = 0.03, P = 4.74 × 10−3). However, the causal effect conferred by CVD on MDD was relatively weak.
There were 675, 253, 328, 426, and 1,653 genome-wide significant genes for CAD, heart failure, atrial fibrillation, stroke, and MDD, respectively. There was an over-representation of shared genes between MDD and each of the four types of CVD (Figure 1C, Supplementary Table 1). A total of seven pleiotropic genes were implicated in MDD and at least three types of CVD, including SLC39A8, MAML3, FADS2, ZFHX3, PLCG1, ZHX3, and ADI1P1 (Figure 1D). Notably, ZHX3 and ADI1P1 genes were shared by MDD with all four types of CVD.
The cross-trait meta-analysis of MDD and stroke revealed 45 loci with 104 independent significant SNPs (IndSigSNPs), including 13 loci involving 19 pleiotropic IndSigSNPs and associated with both traits (Table 3, Figures 2A–D). Tissue expression analysis showed that the associations were significantly enriched in brain tissues (Figure 1F). For datasets on MDD and stroke, λmeta values were at 1.11 ± 0.01, indicating no significant overlap between disease-specific GWAS samples. Quantile-quantile (QQ) plots to display the observed meta-analysis statistics vs. the expected statistics under the null model of no associations in the -log10(p) scale are shown in Supplementary Figure 1.
Figure 2. Meta-analysis of major depressive disorder with stroke. (A) Manhattan plot of the meta-analysis. The x-axis is the chromosomal position of SNPs and the y-axis is the significance of the SNPs (-log10P). Genes implicated by independent significant SNPs were annotated. (B–D) The four highlighted genomic loci. Each SNP is color-coded based on the highest r2 to one of the independent significant SNPs if that is greater or equal to the r2 threshold of 0.6. Other SNPs (below the r2 of 0.6) are colored in gray.
To prioritize putatively causal genes from the meta-analysis of MDD and stroke, fine-mapping of TWAS associations was performed. A total of 100 gene-tissue pairs were identified as part of the 90% credible set for the three tissues, with 71 genes in total. Four genes were identified to be in the credible set with the highest posterior probabilities (PIP > 0.90), including RPL31P12, BORCS7, PTPN11, and PGF (Supplementary Table 2, Figure 1G, Supplementary Figures 2–5).
Depression is a major cause of morbidity and poor quality of life among CVD patients (6), and an independent risk factor for major adverse cardiovascular events (8). The comorbidity of depression and adverse cardiovascular outcomes typically forms a vicious cycle, known to significantly impact both the course and the management of these common conditions.
The polygenicity of MDD is much higher than that of CVD. Although the genetic correlation between MDD and CVD is relatively low, the substantial polygenic overlap between MDD and CVD was evident. For each CVD or related physiological parameter, more than 60% of genetic variants overlap with those of MDD. Notably, nearly all causal variants influencing atrial fibrillation risk also affect MDD. In addition, we observed an over-representation of shared genes between MDD and all types of CVD. Interestingly, two genes locating at chromosome 20q12, PLCG1, and ZHX3, were implicated in all the five traits, making the chromosome 20q12 region a major pleiotropic locus for both MDD and CVD.
The gene PLCG1 encodes protein PLCγ1, which plays a key role in the intracellular transduction of the signal from receptor-mediated tyrosine kinase activators. In the brain, PLCγ is primarily activated by neurotransmitters, neurotrophic factors, and hormones. Prior studies have reported the potential role of PLCG1 in both normal brain function and brain disorders, including MDD (40, 41). On the other hand, the PLCγ1-dependent signaling is critical for arterial development (42), the repair of the intima after vessel injury (43), and the myogenic constriction of cerebral arteries (44). The ZHX3 gene encodes a member of the zinc fingers and homeoboxes (ZHX) gene family. Dysregulation of ZHX factors has been reported in both neurological and hematological diseases (45).
Even as high comorbidity of MDD and CVD has long been acknowledged, and their associations have been well-studied and discussed (7, 11), causal relationships between these two conditions came into the focus just recently. In this work, genetic liability to MDD was shown to etiologically influence the development of CAD and stroke, while liability to cardiovascular outcomes exerted no or minimal influence on MDD. Genetic correlation evaluates the relationship between two traits, and the sign of the correlation coefficient is determined by whether the directions of the shared genetic effect are predominantly the same or opposite for the two traits. Two traits can have substantial polygenic overlap with a non-significant genetic correlation between them (10, 46), which may account for the causal effect of MDD on stroke in the context of no genetic correlation between them. This leads us to the argument that the high rate of cardiovascular events in MDD patients may, at least partially, follow genetic variations inherited by the patients. When compounded with an unhealthy lifestyle, including an overall reduction of the physical activity commonly seen in depressed patients, this pre-existing liability may lead to the acquisition of cardiovascular disease. On the contrary, the high rate of depression seen in CVD patients may largely be due to a psychological and physical reaction that occurs after cardiovascular events, rather than from inherited genetic liability to MDD.
A recent study by Tang et al. reported a causal association of MDD with CAD (23). As the present study was conducted in a CAD dataset which was almost twice larger than that utilized by Tang et al. (332,477 vs. 184,305 patients) and as analytic frameworks were different, our study may be interpreted as a piece of corroborating evidence for the causal effect of MDD on CAD. Another recent work reported that genetic risk factors for MDD may pleiotropically increase CAD risk in females (47). However, the causal effect of MDD on CAD uncovered in our study was relatively weak (bxy = 0.06) when compared with the effects of MDD on stroke (bxy = 0.19). Moreover, our results do not support a causal role of genetic liability to MDD in the development of hypertension but suggest that liability to MDD may result in a marked reduction of pulse pressure instead (bxy = −0.56).
Importantly, our results point to a causal effect of MDD on stroke, thus, extending findings from Cai et al.'s study that have reported the causal effect of MDD on an increased risk of small vessel stroke, but not on a stroke of large arteries (21). The high comorbidity between MDD and stroke has long been observed, with post-stroke depression constituting a common mental health issue (48, 49). However, biological mechanisms underlying the phenotypic relationships between MDD and stroke remain largely elusive. Our meta-analysis of MDD and stroke identified 16 protein-coding genes as shared by the two traits. Among these genes, nine have been previously implicated in GWASs of depression, namely, AREL1, DENND1A, NR4A2, PAX5, RPS6KL1, SOX5, TMEM106B, VRK2, and YLPM1; none of these genes have been identified in any GWAS for stroke. Five genes have been described as genome-wide associated with cardiovascular traits, including PGF, PROX2, DLST, TMEM106B, and VWDE. Notably, TMEM106B was repeatedly identified as a risk gene for frontotemporal lobar degeneration (50–52). Evidence for the involvement of TMEM106B in depression is also compelling (20, 53, 54). Incidentally, one recent study reported TMEM106B as a genome-wide risk gene for CAD (55).
To identify potentially causal genes involved in MDD and stroke, we used the fine-mapping of TWAS hits implemented in FOCUS. In course of estimating the causality in three relevant tissues, a total of 71 genes were included in the 90%-credible set, including four genes with high PIP. Specifically, the genomic region 1p31.1 (Figure 2B) containing RPL31P12 was included in the 90%-credible gene set with a posterior probability of 1.00 in the brain cerebellum. It was reported that the SNP rs10789336 in the NEGR1 gene is associated with the expression level of RPL31P12 in brain tissues, and also confers the risk for MDD (56). In the 10q24.32 region, BORCS7, a genome-wide risk gene for schizophrenia (57, 58), blood pressure (59, 60), body mass index (61), and CAD (55), had the highest PIP of 0.97 in the dorsolateral prefrontal cortex. Notably, in a PET imaging study, a SNP in this gene was associated with the altered dopaminergic function (62). Given that both stroke and MDD affect the brain, both RPL31P12 and BORCS7 loci are attractive as candidates conferring genetic liability for both diseases.
In the 12q24.13 region (Figure 2C), PTPN11 entered in the credible gene set with a PIP of 0.92 for the left ventricle of the heart. Previous GWASs have implicated PTPN11 in peripheral artery disease (63), blood pressure (64, 65), and multiple sclerosis (66). Locus PTPN11 encodes SHP2, a member of the protein tyrosine phosphatase family that regulates a wide variety of cellular functions including cell growth, differentiation, mitotic cycle, and oncogenic transformation. In particular, SHP2 serves as a pivotal regulator of normal cardiac development and function (67). PTPN11 mutations are the most common cause of Noonan syndrome, a relatively common autosomal dominant disorder, classified as a RASopathy (68), a disorder of RAS signaling commonly associated with hypertrophic cardiomyopathy, or other malformations of the blood vessels. Our study provides evidence supporting the potential causal role of PTPN11 in stroke.
In the genomic locus 14q24.3 (Figure 2D), PGF had a PIP of 0.96 for the left ventricle of the heart. PGF encodes a secreted placental growth factor (PGF), which belongs to the vascular endothelial growth factor (VEGF) superfamily. PGF regulates cardiac adaptation through the hypertrophy of the heart tissue by inducing capillary growth and fibroblast proliferation (69). In the heart, PGF serves as a protective paracrine effector (70). One animal study demonstrated that the deficiency of Pgf in rodents affects cognitive functions, brain neuroanatomy, and cerebrovasculature (71). In human patients, reduced expression of PGF was linked to preeclampsia and cerebrovascular and neurological aberrations occurring in fetuses; in turn, preeclampsia may impair cognitive functioning, increase the risk for stroke and lead to adverse stroke outcomes (72). Previous genome-wide analyses identified PGF as a candidate gene both for CAD (55) and for mood instability (73). Our meta-analysis identified PGF as a risk gene for both MDD and stroke, and fine-mapping of TWAS signals further asserted that PGF is a possible causal gene for stroke.
In 2008, the American Heart Association (AHA) issued an advisory to screen all patients with CAD for depression (74). Later it was demonstrated that, in this group of patients, a standardized screening pathway for the assessment of depression offers the potential for early identification and improved management (75, 76). Similarly, recognition of shared genetic liability between MDD and CVD suggests the need to evaluate cardiovascular risk in patients with MDD, for example, by using polygenic risk scores (PRS). Since medical comorbidities are also known to contribute to either poor response to antidepressants or treatment resistance (77), it is tempting to speculate that a stratified allocation of treatment for MDD patients with higher genetic risk for CVD may help both to achieve a better response to SSRIs and to lower the risk for an adverse outcome of CVD.
Together, our study reveals novel mechanisms by which MDD influences the risk for the development of CVD (Figure 1E). Identification of shared genetic foundations for MDD and CVD may guide drug discovery and inform early prediction and personalized treatment for these commonly comorbid conditions.
The presented study has several strengths. First, to evaluate the shared genetic liability between MDD and CVD multiple cardiovascular outcomes were analyzed. Second, for each trait, we typically prioritized the largest available dataset as a study backbone. Furthermore, to avoid potential population heterogeneity across the studies, whenever possible, we limited our analysis to individuals of European ancestry. Finally, the genetic relationships between MDD and CVD were evaluated using multiple analytic strategies, corroborating each other.
We should acknowledge several limitations of this work. As our analyses were limited to a genetic component of the traits and European ancestry population, the presented results should be interpreted cautiously. It is also worth noticing that TWAS associations are not free of noise, since the gene expression levels were imputed from weighted linear combinations of SNPs. Considering that the observed causal effect of MDD on CAD was relatively weak, only stroke was included in the further gene-hunting analyses, thus, minimizing the possibility of overreaching for causal inference.
MDD and major types of CVD share substantial genetic variations. Genetic liability to MDD may confer risk for stroke and CAD. Presented results shed light on mechanisms underlying phenotypic relationships between MDD, CVD, and prioritize several candidate genes for future studies.
All datasets analyzed during this study are publicly available at PGC (https://www.med.unc.edu/pgc/) and GWAS catalog (https://www.ebi.ac.uk/gwas/).
FZ conceived the project and analyzed the data. FZ and AB wrote the manuscript. FZ, AB, and HC contributed to the revision of the manuscript. All authors read and approved the final manuscript.
This work was supported by the National Natural Science Foundation of China (81471364).
The authors declare that the research was conducted in the absence of any commercial or financial relationships that could be construed as a potential conflict of interest.
All claims expressed in this article are solely those of the authors and do not necessarily represent those of their affiliated organizations, or those of the publisher, the editors and the reviewers. Any product that may be evaluated in this article, or claim that may be made by its manufacturer, is not guaranteed or endorsed by the publisher.
We thank members of the Psychiatric Genomics Consortium, the UK Biobank, the 23andMe, and other teams, who generously shared the GWAS data.
The Supplementary Material for this article can be found online at: https://www.frontiersin.org/articles/10.3389/fcvm.2021.735136/full#supplementary-material
1. GBD 2017 DALYs and HALE Collaborators. Global, regional, and national disability-adjusted life-years (DALYs) for 359 diseases and injuries and healthy life expectancy (HALE) for 195 countries and territories, 1990-2017: a systematic analysis for the Global Burden of Disease Study 2017. Lancet. (2018) 392:1859–922. doi: 10.1016/S0140-6736(18)32335-3
2. Whiteford HA, Degenhardt L, Rehm J, Baxter AJ, Ferrari AJ, Erskine HE, et al. Global burden of disease attributable to mental and substance use disorders: findings from the Global Burden of Disease Study 2010. Lancet. (2013) 382:1575–86. doi: 10.1016/S0140-6736(13)61611-6
3. Ferrari AJ, Charlson FJ, Norman RE, Patten SB, Freedman G, Murray CJ, et al. Burden of depressive disorders by country, sex, age, and year: findings from the global burden of disease study 2010. PLoS Med. (2013) 10:e1001547. doi: 10.1371/journal.pmed.1001547
4. Disease GBD, Injury I, Prevalence C. Global, regional, and national incidence, prevalence, and years lived with disability for 328 diseases and injuries for 195 countries, 1990-2016: a systematic analysis for the Global Burden of Disease Study 2016. Lancet. (2017) 390:1211–259. doi: 10.1016/S0140-6736(17)32154-2
5. Hare DL, Toukhsati SR, Johansson P, Jaarsma T. Depression and cardiovascular disease: a clinical review. Eur Heart J. (2014) 35:1365–72. doi: 10.1093/eurheartj/eht462
6. Correll CU, Solmi M, Veronese N, Bortolato B, Rosson S, Santonastaso P, et al. Prevalence, incidence and mortality from cardiovascular disease in patients with pooled and specific severe mental illness: a large-scale meta-analysis of 3,211,768 patients and 113,383,368 controls. World Psychiatry. (2017) 16:163–80. doi: 10.1002/wps.20420
7. Barth J, Schumacher M, Herrmann-Lingen C. Depression as a risk factor for mortality in patients with coronary heart disease: a meta-analysis. Psychosom Med. (2004) 66:802–13. doi: 10.1097/01.psy.0000146332.53619.b2
8. Yusuf S, Hawken S, Ounpuu S, Dans T, Avezum A, Lanas F, et al. Effect of potentially modifiable risk factors associated with myocardial infarction in 52 countries (the INTERHEART study): case-control study. Lancet. (2004) 364:937–52. doi: 10.1016/S0140-6736(04)17018-9
9. Spijkerman T, de Jonge P, van den Brink RH, Jansen JH, May JF, Crijns HJ, et al. Depression following myocardial infarction: first-ever versus ongoing and recurrent episodes. Gen Hosp Psychiatry. (2005) 27:411–7. doi: 10.1016/j.genhosppsych.2005.05.007
10. Frei O, Holland D, Smeland OB, Shadrin AA, Fan CC, Maeland S, et al. Bivariate causal mixture model quantifies polygenic overlap between complex traits beyond genetic correlation. Nat Commun. (2019) 10:2417. doi: 10.1038/s41467-019-10310-0
11. Kendler KS, Gardner CO, Fiske A, Gatz M. Major depression and coronary artery disease in the Swedish twin registry: phenotypic, genetic, and environmental sources of comorbidity. Arch Gen Psychiatry. (2009) 66:857–63. doi: 10.1001/archgenpsychiatry.2009.94
12. Lawlor DA, Harbord RM, Sterne JA, Timpson N, Davey Smith G. Mendelian randomization: using genes as instruments for making causal inferences in epidemiology. Stat Med. (2008) 27:1133–63. doi: 10.1002/sim.3034
13. Burgess S, Scott RA, Timpson NJ, Davey Smith G, Thompson SG, Consortium E-I. Using published data in Mendelian randomization: a blueprint for efficient identification of causal risk factors. Eur J Epidemiol. (2015) 30:543–52. doi: 10.1007/s10654-015-0011-z
14. Hemani G, Zheng J, Elsworth B, Wade KH, Haberland V, Baird D, et al. The MR-Base platform supports systematic causal inference across the human phenome. Elife. (2018) 7:e34408. doi: 10.7554/eLife.34408
15. Zhu Z, Zheng Z, Zhang F, Wu Y, Trzaskowski M, Maier R, et al. Causal associations between risk factors and common diseases inferred from GWAS summary data. Nat Commun. (2018) 9:224. doi: 10.1038/s41467-017-02317-2
16. Jansen IE, Savage JE, Watanabe K, Bryois J, Williams DM, Steinberg S, et al. Genome-wide meta-analysis identifies new loci and functional pathways influencing Alzheimer's disease risk. Nat Genet. (2019) 51:404–13. doi: 10.1038/s41588-018-0311-9
17. Pasman JA, Verweij KJH, Gerring Z, Stringer S, Sanchez-Roige S, Treur JL, et al. GWAS of lifetime cannabis use reveals new risk loci, genetic overlap with psychiatric traits, and a causal influence of schizophrenia. Nat Neurosci. (2018) 21:1161–70. doi: 10.1038/s41593-018-0206-1
18. Savage JE, Jansen PR, Stringer S, Watanabe K, Bryois J, de Leeuw CA, et al. Genome-wide association meta-analysis in 269,867 individuals identifies new genetic and functional links to intelligence. Nat Genet. (2018) 50:912–9. doi: 10.1038/s41588-018-0152-6
19. Colodro-Conde L, Couvy-Duchesne B, Whitfield JB, Streit F, Gordon S, Kemper KE, et al. Association between population density and genetic risk for schizophrenia. JAMA Psychiatry. (2018) 75:901–10. doi: 10.1001/jamapsychiatry.2018.1581
20. Wray NR, Ripke S, Mattheisen M, Trzaskowski M, Byrne EM, Abdellaoui A, et al. Genome-wide association analyses identify 44 risk variants and refine the genetic architecture of major depression. Nat Genet. (2018) 50:668–81. doi: 10.1038/s41588-018-0090-3
21. Cai H, Cai B, Zhang H, Sun W, Wang Y, Zhou S, et al. Major depression and small vessel stroke: a Mendelian randomization analysis. J Neurol. (2019) 266:2859–66. doi: 10.1007/s00415-019-09511-w
22. Mulugeta A, Zhou A, King C, Hypponen E. Association between major depressive disorder and multiple disease outcomes: a phenome-wide Mendelian randomisation study in the UK Biobank. Mol Psychiatry. (2019) 25:1469–76. doi: 10.1038/s41380-019-0486-1
23. Tang B, Yuan S, Xiong Y, He Q, Larsson SC. Major depressive disorder and cardiometabolic diseases: a bidirectional Mendelian randomisation study. Diabetologia. (2020) 63:1305–11. doi: 10.1007/s00125-020-05131-6
24. Nelson CP, Goel A, Butterworth AS, Kanoni S, Webb TR, Marouli E, et al. Association analyses based on false discovery rate implicate new loci for coronary artery disease. Nat Genet. (2017) 49:1385–91. doi: 10.1038/ng.3913
25. Shah S, Henry A, Roselli C, Lin H, Sveinbjornsson G, Fatemifar G, et al. Genome-wide association and Mendelian randomisation analysis provide insights into the pathogenesis of heart failure. Nat Commun. (2020) 11:163. doi: 10.1038/s41467-019-13690-5
26. Roselli C, Chaffin MD, Weng LC, Aeschbacher S, Ahlberg G, Albert CM, et al. Multi-ethnic genome-wide association study for atrial fibrillation. Nat Genet. (2018) 50:1225–33. doi: 10.1038/s41588-018-0133-9
27. Malik R, Chauhan G, Traylor M, Sargurupremraj M, Okada Y, Mishra A, et al. Multiancestry genome-wide association study of 520,000 subjects identifies 32 loci associated with stroke and stroke subtypes. Nat Genet. (2018) 50:524–37. doi: 10.1038/s41588-018-0058-3
28. Evangelou E, Warren HR, Mosen-Ansorena D, Mifsud B, Pazoki R, Gao H, et al. Genetic analysis of over 1 million people identifies 535 new loci associated with blood pressure traits. Nat Genet. (2018) 50:1412–25. doi: 10.1038/s41588-018-0205-x
29. Sudlow C, Gallacher J, Allen N, Beral V, Burton P, Danesh J, et al. UK biobank: an open access resource for identifying the causes of a wide range of complex diseases of middle and old age. PLoS Med. (2015) 12:e1001779. doi: 10.1371/journal.pmed.1001779
30. Bulik-Sullivan BK, Loh PR, Finucane HK, Ripke S, Yang J, Schizophrenia Working Group of the Psychiatric Genomics C, et al. LD Score regression distinguishes confounding from polygenicity in genome-wide association studies. Nat Genet. (2015) 47:291–5. doi: 10.1038/ng.3211
31. Bulik-Sullivan B, Finucane HK, Anttila V, Gusev A, Day FR, Loh PR, et al. An atlas of genetic correlations across human diseases and traits. Nat Genet. (2015) 47:1236–41. doi: 10.1038/ng.3406
32. Solovieff N, Cotsapas C, Lee PH, Purcell SM, Smoller JW. Pleiotropy in complex traits: challenges and strategies. Nat Rev Genet. (2013) 14:483–95. doi: 10.1038/nrg3461
33. Ong JS, MacGregor S. Implementing MR-PRESSO and GCTA-GSMR for pleiotropy assessment in Mendelian randomization studies from a practitioner's perspective. Genet Epidemiol. (2019) 43:609–16. doi: 10.1002/gepi.22207
34. Buniello A, MacArthur JAL, Cerezo M, Harris LW, Hayhurst J, Malangone C, et al. The NHGRI-EBI GWAS catalog of published genome-wide association studies, targeted arrays and summary statistics 2019. Nucleic Acids Res. (2019) 47:D1005–12. doi: 10.1093/nar/gky1120
35. Wang M, Zhao Y, Zhang B. Efficient test and visualization of multi-set intersections. Sci Rep. (2015) 5:16923. doi: 10.1038/srep16923
36. Bhattacharjee S, Rajaraman P, Jacobs KB, Wheeler WA, Melin BS, Hartge P, et al. A subset-based approach improves power and interpretation for the combined analysis of genetic association studies of heterogeneous traits. Am J Hum Genet. (2012) 90:821–35. doi: 10.1016/j.ajhg.2012.03.015
37. Watanabe K, Taskesen E, van Bochoven A, Posthuma D. Functional mapping and annotation of genetic associations with FUMA. Nat Commun. (2017) 8:1826. doi: 10.1038/s41467-017-01261-5
38. Chen GB, Lee SH, Robinson MR, Trzaskowski M, Zhu ZX, Winkler TW, et al. Across-cohort QC analyses of GWAS summary statistics from complex traits. Eur J Hum Genet. (2016) 25:137–46. doi: 10.1038/ejhg.2016.106
39. Mancuso N, Freund MK, Johnson R, Shi H, Kichaev G, Gusev A, et al. Probabilistic fine-mapping of transcriptome-wide association studies. Nat Genet. (2019) 51:675–82. doi: 10.1038/s41588-019-0367-1
40. Yang YR, Kang DS, Lee C, Seok H, Follo MY, Cocco L, et al. Primary phospholipase C and brain disorders. Adv Biol Regul. (2016) 61:80–5. doi: 10.1016/j.jbior.2015.11.003
41. Zhang G, Xu S, Zhang Z, Zhang Y, Wu Y, An J, et al. Identification of key genes and the pathophysiology associated with major depressive disorder patients based on integrated bioinformatics analysis. Front Psychiatry. (2020) 11:192. doi: 10.3389/fpsyt.2020.00192
42. Lawson ND, Mugford JW, Diamond BA, Weinstein BM. phospholipase C gamma-1 is required downstream of vascular endothelial growth factor during arterial development. Genes Dev. (2003) 17:1346–51. doi: 10.1101/gad.1072203
43. Jiang D, Zhuang J, Peng W, Lu Y, Liu H, Zhao Q, et al. Phospholipase Cgamma1 mediates intima formation through Akt-Notch1 signaling independent of the phospholipase activity. J Am Heart Assoc. (2017) 6:e005537. doi: 10.1161/JAHA.117.005537
44. Gonzales AL, Yang Y, Sullivan MN, Sanders L, Dabertrand F, Hill-Eubanks DC, et al. A PLCgamma1-dependent, force-sensitive signaling network in the myogenic constriction of cerebral arteries. Sci Signal. (2014) 7:ra49. doi: 10.1126/scisignal.2004732
45. Liu Y, Ma D, Ji C. Zinc fingers and homeoboxes family in human diseases. Cancer Gene Ther. (2015) 22:223–6. doi: 10.1038/cgt.2015.16
46. Smeland OB, Bahrami S, Frei O, Shadrin A, O'Connell K, Savage J, et al. Genome-wide analysis reveals extensive genetic overlap between schizophrenia, bipolar disorder, and intelligence. Mol Psychiatry. (2020) 25:844–53. doi: 10.1038/s41380-018-0332-x
47. Dennis J, Sealock J, Levinson RT, Farber-Eger E, Franco J, Fong S, et al. Genetic risk for major depressive disorder and loneliness in sex-specific associations with coronary artery disease. Mol Psychiatry. (2019). doi: 10.1038/s41380-019-0614-y. [Epub ahead of print].
48. Towfighi A, Ovbiagele B, El Husseini N, Hackett ML, Jorge RE, Kissela BM, et al. Poststroke depression: a scientific statement for healthcare professionals from the American Heart Association/American Stroke Association. Stroke. (2017) 48:e30–43. doi: 10.1161/STR.0000000000000113
49. Das J, G KR. Post stroke depression: the sequelae of cerebral stroke. Neurosci Biobehav Rev. (2018) 90:104–14. doi: 10.1016/j.neubiorev.2018.04.005
50. Van Deerlin VM, Sleiman PM, Martinez-Lage M, Chen-Plotkin A, Wang LS, Graff-Radford NR, et al. Common variants at 7p21 are associated with frontotemporal lobar degeneration with TDP-43 inclusions. Nat Genet. (2010) 42:234–9. doi: 10.1038/ng.536
51. Gallagher MD, Suh E, Grossman M, Elman L, McCluskey L, Van Swieten JC, et al. TMEM106B is a genetic modifier of frontotemporal lobar degeneration with C9orf72 hexanucleotide repeat expansions. Acta Neuropathol. (2014) 127:407–18. doi: 10.1007/s00401-013-1239-x
52. Pottier C, Zhou X, Perkerson RB, 3rd, Baker M, Jenkins GD, Serie DJ, et al. Potential genetic modifiers of disease risk and age at onset in patients with frontotemporal lobar degeneration and GRN mutations: a genome-wide association study. Lancet Neurol. (2018) 17:548–58. doi: 10.1016/S1474-4422(18)30126-1
53. Howard DM, Adams MJ, Shirali M, Clarke TK, Marioni RE, Davies G, et al. Genome-wide association study of depression phenotypes in UK Biobank identifies variants in excitatory synaptic pathways. Nat Commun. (2018) 9:1470. doi: 10.1101/168732
54. Howard DM, Adams MJ, Clarke TK, Hafferty JD, Gibson J, Shirali M, et al. Genome-wide meta-analysis of depression identifies 102 independent variants and highlights the importance of the prefrontal brain regions. Nat Neurosci. (2019) 22:343–52. doi: 10.1038/s41593-018-0326-7
55. van der Harst P, Verweij N. Identification of 64 novel genetic loci provides an expanded view on the genetic architecture of coronary artery disease. Circ Res. (2018) 122:433–43. doi: 10.1161/CIRCRESAHA.117.312086
56. Wang X, Cheng W, Zhu J, Yin H, Chang S, Yue W, et al. Integrating genome-wide association study and expression quantitative trait loci data identifies NEGR1 as a causal risk gene of major depression disorder. J Affect Disord. (2020) 265:679–86. doi: 10.1016/j.jad.2019.11.116
57. Ripke S, O'Dushlaine C, Chambert K, Moran JL, Kahler AK, Akterin S, et al. Genome-wide association analysis identifies 13 new risk loci for schizophrenia. Nat Genet. (2013) 45:1150–9. doi: 10.1038/ng.2742
58. Li Z, Chen J, Yu H, He L, Xu Y, Zhang D, et al. Genome-wide association analysis identifies 30 new susceptibility loci for schizophrenia. Nat Genet. (2017) 49:1576–83. doi: 10.1038/ng.3973
59. Lu X, Wang L, Lin X, Huang J, Charles Gu C, He M, et al. Genome-wide association study in Chinese identifies novel loci for blood pressure and hypertension. Hum Mol Genet. (2015) 24:865–74. doi: 10.1093/hmg/ddu478
60. Takeuchi F, Akiyama M, Matoba N, Katsuya T, Nakatochi M, Tabara Y, et al. Interethnic analyses of blood pressure loci in populations of East Asian and European descent. Nat Commun. (2018) 9:5052. doi: 10.1038/s41467-018-07345-0
61. Hoffmann TJ, Choquet H, Yin J, Banda Y, Kvale MN, Glymour M, et al. A large multiethnic genome-wide association study of adult body mass index identifies novel loci. Genetics. (2018) 210:499–15. doi: 10.1534/genetics.118.301479
62. D'Ambrosio E, Dahoun T, Pardinas AF, Veronese M, Bloomfield MAP, Jauhar S, et al. The effect of a genetic variant at the schizophrenia associated AS3MT/BORCS7 locus on striatal dopamine function: a PET imaging study. Psychiatry Res Neuroimaging. (2019) 291:34–41. doi: 10.1016/j.pscychresns.2019.07.005
63. Klarin D, Lynch J, Aragam K, Chaffin M, Assimes TL, Huang J, et al. Genome-wide association study of peripheral artery disease in the Million Veteran Program. Nat Med. (2019) 25:1274–9. doi: 10.1038/s41591-019-0492-5
64. Giri A, Hellwege JN, Keaton JM, Park J, Qiu C, Warren HR, et al. Trans-ethnic association study of blood pressure determinants in over 750,000 individuals. Nat Genet. (2019) 51:51–62. doi: 10.1038/s41588-018-0303-9
65. Sung YJ, Winkler TW, de Las Fuentes L, Bentley AR, Brown MR, Kraja AT, et al. A large-scale multi-ancestry genome-wide study accounting for smoking behavior identifies multiple significant loci for blood pressure. Am J Hum Genet. (2018) 102:375–400. doi: 10.1016/j.ajhg.2018.01.015
66. International Multiple Sclerosis Genetics Consortium. Multiple sclerosis genomic map implicates peripheral immune cells and microglia in susceptibility. Science. (2019) 365:eaav7188. doi: 10.1126/science.aav7188
67. Lauriol J, Jaffre F, Kontaridis MI. The role of the protein tyrosine phosphatase SHP2 in cardiac development and disease. Semin Cell Dev Biol. (2015) 37:73–81. doi: 10.1016/j.semcdb.2014.09.013
68. Siegfried A, Cances C, Denuelle M, Loukh N, Tauber M, Cave H, et al. Noonan syndrome, PTPN11 mutations, and brain tumors. a clinical report and review of the literature. Am J Med Genet A. (2017) 173:1061–5. doi: 10.1002/ajmg.a.38108
69. Accornero F, van Berlo JH, Benard MJ, Lorenz JN, Carmeliet P, Molkentin JD. Placental growth factor regulates cardiac adaptation and hypertrophy through a paracrine mechanism. Circ Res. (2011) 109:272–80. doi: 10.1161/CIRCRESAHA.111.240820
70. Accornero F, Molkentin JD. Placental growth factor as a protective paracrine effector in the heart. Trends Cardiovasc Med. (2011) 21:220–4. doi: 10.1016/j.tcm.2012.05.014
71. Kay VR, Ratsep MT, Cahill LS, Hickman AF, Zavan B, Newport ME, et al. Effects of placental growth factor deficiency on behavior, neuroanatomy, and cerebrovasculature of mice. Physiol Genomics. (2018) 50:862–75. doi: 10.1152/physiolgenomics.00076.2018
72. Ratsep MT, Hickman AF, Croy BA. The Elsevier trophoblast research award lecture: impacts of placental growth factor and preeclampsia on brain development, behaviour, and cognition. Placenta. (2016) 48(Suppl. 1):S40–6. doi: 10.1016/j.placenta.2016.02.001
73. Ward J, Strawbridge RJ, Bailey MES, Graham N, Ferguson A, Lyall DM, et al. Genome-wide analysis in UK Biobank identifies four loci associated with mood instability and genetic correlation with major depressive disorder, anxiety disorder and schizophrenia. Transl Psychiatry. (2017) 7:1264. doi: 10.1038/s41398-017-0012-7
74. Lichtman JH, Bigger JT Jr, Blumenthal JA, Frasure-Smith N, Kaufmann PG, Lespérance F, et al. Depression and coronary heart disease: recommendations for screening, referral, and treatment: a science advisory from the American Heart Association Prevention Committee of the Council on Cardiovascular Nursing, Council on Clinical Cardiology, Council on Epidemiology and Prevention, and Interdisciplinary Council on Quality of Care and Outcomes Research: endorsed by the American Psychiatric Association. Circulation. (2008) 118:1768–75. doi: 10.1161/CIRCULATIONAHA.108.190769
75. Berkman LF, Blumenthal J, Burg M, Carney RM, Catellier D, Cowan MJ, et al. Effects of treating depression and low perceived social support on clinical events after myocardial infarction: the Enhancing Recovery in Coronary Heart Disease Patients (ENRICHD) Randomized Trial. JAMA. (2003) 289:3106–16. doi: 10.1001/jama.289.23.3106
76. Kim JM, Stewart R, Lee YS, Lee HJ, Kim MC, Kim JW, et al. Effect of escitalopram vs placebo treatment for depression on long-term cardiac outcomes in patients with acute coronary syndrome: a randomized clinical trial. JAMA. (2018) 320:350–8. doi: 10.1001/jama.2018.9422
Keywords: major depressive disorder, cardiovascular disease, Mendelian randomization, polygenic overlap, stroke
Citation: Zhang F, Cao H and Baranova A (2021) Shared Genetic Liability and Causal Associations Between Major Depressive Disorder and Cardiovascular Diseases. Front. Cardiovasc. Med. 8:735136. doi: 10.3389/fcvm.2021.735136
Received: 02 July 2021; Accepted: 08 October 2021;
Published: 11 November 2021.
Edited by:
Shefali S. Verma, University of Pennsylvania, United StatesReviewed by:
Xu Lin, Southern Medical University, ChinaCopyright © 2021 Zhang, Cao and Baranova. This is an open-access article distributed under the terms of the Creative Commons Attribution License (CC BY). The use, distribution or reproduction in other forums is permitted, provided the original author(s) and the copyright owner(s) are credited and that the original publication in this journal is cited, in accordance with accepted academic practice. No use, distribution or reproduction is permitted which does not comply with these terms.
*Correspondence: Fuquan Zhang, emhhbmdmcUBuam11LmVkdS5jbg==
Disclaimer: All claims expressed in this article are solely those of the authors and do not necessarily represent those of their affiliated organizations, or those of the publisher, the editors and the reviewers. Any product that may be evaluated in this article or claim that may be made by its manufacturer is not guaranteed or endorsed by the publisher.
Research integrity at Frontiers
Learn more about the work of our research integrity team to safeguard the quality of each article we publish.