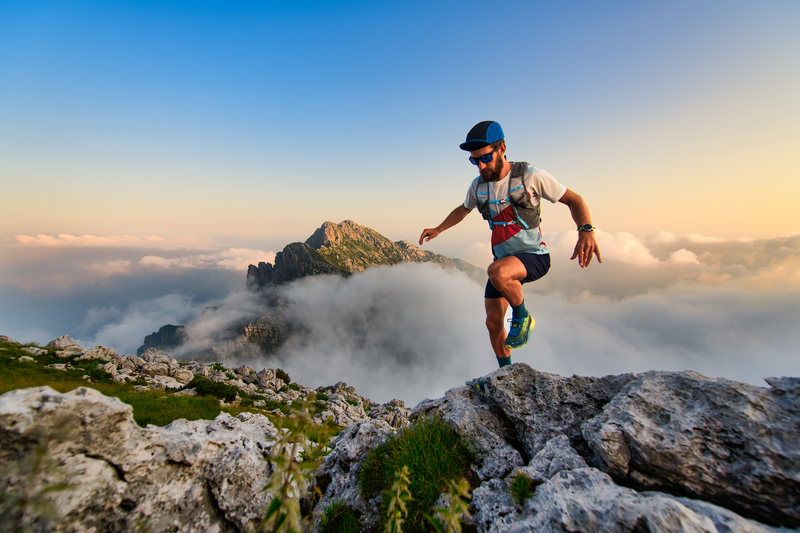
94% of researchers rate our articles as excellent or good
Learn more about the work of our research integrity team to safeguard the quality of each article we publish.
Find out more
ORIGINAL RESEARCH article
Front. Cardiovasc. Med. , 08 December 2021
Sec. Sex and Gender in Cardiovascular Medicine
Volume 8 - 2021 | https://doi.org/10.3389/fcvm.2021.707070
Background: Coronary heart disease (CHD) is the major cause of mortality in the world with a significant impact on the younger population. The aim of this study was to identify prematurity among patients with coronary artery bypass graft surgery (CABG) based on the clustering of CHD risk factors.
Methods: Patients were recruited from an existing cohort of candidates for CABG surgery named Tehran Heart Center Coronary Outcome Measurement (THC-COM). A latent class analysis (LCA) model was formed using 11 potential risk factors as binary variables: cigarette smoking, obesity, diabetes, family history of CHD, alcohol use, opium addiction, hypertension, history of stroke, history of myocardial infarction (MI), peripheral vascular disease (PVD), and hyperlipidemia (HLP). We analyzed our data to figure out how the patients are going to be clustered based on their risk factors.
Results: For 566 patients who were studied, the mean age (SD) and BMI of patients were 59.1 (8.9) and 27.3 (4.1), respectively. The LCA model fit with two latent classes was statistically significant (G2 = 824.87, df = 21, p < 0.0001). The mean (SD) age of patients for Class I and Class II was 55.66 (8.55) and 60.87 (8.66), respectively. Class I (premature) was characterized by a high probability of smoking, alcohol consumption, opium addiction, and a history of MI (P < 0.05), and class II by a high probability of obesity, diabetes, and hypertension.
Conclusion: Latent class analysis calculated two groups of severe CHD with distinct risk markers. The younger group, which is characterized by smoking, addiction, and the history of MI, can be regarded as representative of premature CHD.
- Clustering of patients with coronary artery diseases provide a better understanding of the disease behavior.
- Clustering patients based on individual and clinical characteristics help health counselors to predict premature CHD.
- Findings are applicable to policy makers and health professionals for coronary artery diseases (CHD) management.
Coronary heart disease (CHD) is the major cause of mortality in the world and one of the leading causes of disease burden in developing and developed countries (1). Of the 17 million deaths from cardiovascular diseases (CVDs) in 2015, 7.4 million people died of CHD. It is responsible for over a third of premature deaths globally (1). The number of CABG operations performed to treat CHD has increased more than five-fold since 1980, and the general trend has been an almost steady rise in the number of operations performed each year (2, 3). With increasing the number of patients undergoing CABG, their healthcare costs have quadrupled in the last decade (4, 5).
There is no consensus on the definition, risk factors, and age limits for premature CHD in the clinical setting. The Task Force of the European Society of Cardiology (ESC), European Atherosclerosis Society (EAS), and European Society of Hypertension (ESH) have recommended screening for coronary risk factors to the close relatives of patients with premature CHD. They have been defined premature CHD as having CHDs for men <55 years and women <65 years (6). Sutter et al. have employed this definition to identify premature CHD in their study (7). Some have proposed <51 years for men and <56 for women as a cutoff point for premature CHD (8). Mulders et al. used this definition to classify the patients and showed that patients with a genetic predisposition for CVD are at risk for recurrent events (9). However, the age limit as low as 55 years for women and 45 years for men was suggested as well (10). Clinicians need a definitive definition as well to identify patients with premature CHD and prevent any misdiagnosis in the clinical setting.
Latent class analysis (LCA) is a commonly used empirical approach for identifying subpopulations by shared item response patterns. It is also a commonly used data reduction tool for analyzing multivariate categorical data (11). A finite number of exclusive classes of individuals categorized as latent variables are assumed to compose this population (12). The LCA is a statistical procedure that allows the researcher to identify groups of individuals (or clusters) due to their differences within a dataset that would otherwise not be apparent. Traditional cluster and factor analyses are designed for continuous variables, but LCA is suitable for discrete and dichotomous variables. Awareness of these clusters may provide us with a better understanding of the disease behavior and identify patients at higher risk of morbidity (13, 14). Hence, LCA sounds like an appropriate approach for classifying patients according to their individual and clinical characteristics to predict premature CHD. This study was aimed to identify latent classes of patients with severe CHD who were candidates for CABG based on the clustering of proposed risk markers for premature CHD.
Participants in this cross-sectional study were candidates for CABG surgery recruited for Tehran Heart Center Coronary Outcome Measurement (THC-COM) study. Baseline data were collected as part of the large study started in May 2006 (15). The sample size calculation, recruitment method, and definitions have been explained elsewhere (16). Briefly, a total of 535 patients were recruited to this study. Based on numerous studies, Nylund-Gibson and Choi suggest that 300 or more cases are desirable for sampling (17). Written informed consent was obtained from each participant. This study was approved by the Institutional Research Board of Tehran Heart Center, Tehran University of Medical Sciences.
Data collection was conducted through interviews, physical examinations, and paramedical evaluation. Patients were asked about demographic characteristics, including gender, age, family history of CHD [a first-degree relative with CHD was defined as positive family history (18)], cigarette smoking (patient regularly smokes a tobacco product/products one or more times per day or has smoked in the 30 days prior to admission) (19), alcohol consumption (the use of alcohol despite recurrent adverse consequences) (20), drug addiction (opium dependence according to the DSM-IV criteria for substance dependence, daily regular use of substances) (12), and having diabetes mellitus [symptoms of diabetes plus at least one of the following: plasma glucose concentration = 200 mg/dl (11.1 mmol/l), fasting plasma glucose = 126 mg/dl (7.0 mmol/l), plasma glucose 2 h postprandial = 200 mg/dl (11.1 mmol/l)] (21), and hypertension (systolic blood pressure = 140 mmHg and/or diastolic = 90 mmHg and/or on antihypertensive treatment) (22). Anthropometric variables, including weight and height, were measured, and BMI was calculated (23). Weight was measured while patients wearing light clothes. Total cholesterol, HDL cholesterol (HDL-C mg/dl), triglycerides (TG mg/dl), and LDL cholesterol (LDL-C mg/dl) were measured. They were also evaluated for having a history of stroke, myocardial infarction (MI), peripheral vascular disease (PVD), and hyperlipidemia (HLP).
The continuous variables were compared using a t-test, and categorical variables were analyzed using a chi-square test between male patients and female patients. An LCA model was built using 11 known and proposed risk factors for CHD as binary variables: cigarette smoking, obesity, diabetes, family history of CHD, alcohol consumption, opium addiction, hypertension, PVD, HLP, history of stroke, and MI. The included risk factors were added to the statistical model based on premature systematic review studies (24, 25). The LCA analyses were conducted using the “poLCA” package in R 3.4.2. The LCA outcomes include the number of latent classes, the probability of each indicator in each class, and the classification of individuals based on their most likely latent class membership. The assumption underlying the LCA model is that the conditional response probabilities for each individual are to be the same. Also, the assumption of conditional independence declares that within each class, the indicators are independent of one another. The data were checked to ensure that the mentioned assumptions were established. It was assumed that within latent classes, manifest variables are independent of each other. Unmodeled dependence among indicators may induce poor model fit and incorrect values of information criteria [e.g., Bayesian information criterion (BIC)], Akaike information criterion (AIC), resulting in spurious latent classes (usually with an overestimated number of classes). Because our results had reasonable values of information criteria, we can conclude that in our data, the assumption of conditional independence and conditional-response probability in LCA was established.
There are various iterations for the number of identified latent classes. Latent class analysis determines the best model by comparing the frequencies of the observed and expected response patterns, and it calculates G2. For comparing the relative fit of the model according to the different number of classes, G2 (likelihood ratio chi-square) is used. Then, AIC and BIC can be calculated for model selection. The smaller the value of AIC and BIC, the more fit the model. The fit model contains the optimum number of latent classes. Then, we can nominate these classes based on their characteristics (26, 27). In this analysis, expectation-maximization and Newton–Raphson algorithms were used to find the maximum-likelihood estimates of the model parameters (28).
The patients' clinical characteristics are presented in Table 1. The mean age (SD) and BMI of patients were 59.1 (8.9) and 27.3 (4.1), respectively. Women had a significantly greater BMI and higher fasting blood sugar (FBS), blood urea nitrogen (BUN), cholesterol, and LDL-C compared to men. Hematocrit and the level of creatinine were significantly higher in men. The prevalence of major CHD risk factors among the patients is presented in Table 2. Smoking was more prevalent among men, while diabetes and hypertension rates were significantly higher in women.
Latent class membership and response probabilities for each indicator are summarized in Table 2. A model with two latent classes was the best fit for our patients (G2 = 824.87, df = 21, p < 0.0001). The probability of membership was 33.8 and 66.2% for Class (I) and Class (II), respectively. Latent Class I was characterized by a high probability of smoking, a history of MI, alcohol consumption, and opium addiction (P < 0.05). Latent class II was characterized by a high probability of obesity, type 2 diabetes, and hypertension (P < 0.05). Family history, stroke, PVD, and HLP were not significantly different between the two classes. The models with 2, 3, 4, and 5 latent classes are shown in Supplementary Table 1.
Latent class membership and response probabilities for each indicator for men and women are presented in Table 3. The model indices showed that it is statistically significant for both men and women (G2 = 731.7, df = 379, p < 0.0001 for men and G2 = 162.5, df = 112, p < 0.0001 for women). Larger conditional probabilities are in boldface to highlight the overall pattern. While latent class I was characterized by a high probability of smoking, alcohol consumption, opium addiction, and a history of MI in men, it was characterized by a history of MI among women.
Table 3. The latent class analysis and the calculated model for the prevalence of the indicators among men and women.
The mean (SD) age for classes I and II was 55.66 (8.55) and 60.87 (8.66), respectively. There were significant differences in the age of the two groups of patients (P < 0.001). Patients in class I were about 5 years younger than patients in class II. Therefore, we named class I “premature” and class II “non-premature.” The rate of membership in class I for men and women was 44.3 and 2.7%, respectively. Also, the mean age (SD) of people who were categorized in class I was 55.58 (8.57) for men and 60 (6.55) for women. Only three women were categorized as class I or premature.
In this cross-sectional study, using LCA, two clusters of patients with severe CHD were identified with respect to 11 CHD risk markers. Class I is characterized by lower age, higher probability of smoking, alcohol consumption, opium addiction, and history of MI. Hence, we nominated class I as premature and class II as non-premature CHD. There was a difference between men and women with regard to the probability of their risk markers in the two classes.
There was a significant difference in the age of participants among the two classes. Class I included patients with younger age was named premature, and class II was named non-premature. A study used predefined criteria for addressing prematurity based on recommendations of other studies (7) before the age of 55 for men and 65 years for women (29). We employed LCA for clustering risk factors among CABG patients with similar results (55 years for men and 60 years for women) (Table 4). Some studies have shown that the risk of CHD increases with age >45 years in men and >55 years in women (7, 30). Another study applied the parental premature CHD definition when CHD occurred before 55/65 years in the father/mother patients with metabolic syndrome (8), suggesting that metabolic syndrome risk factors play a role in familial patterns for premature CHD.
Many studies have addressed the differences between men and women with regard to the risk factors for premature CVD (16, 31). A study from Iran reported the incidence rate of premature CVD about five and four percent for men and women, respectively (32). A similar study from Iran revealed that modifiable lifestyle risk factors, hypercholesterolemia, low HDL-C, and diabetes were associated with premature CHD in both women and men patients (31, 33). In the recent study, being overweight, having less physical activity, and prediabetes were specific risk factors for premature CHD among women and smoking, and low HDL-C were associated with premature CHD among men. The current study used LCA and clustered 11 risk markers in two distinct class I (premature 33.8%) and class II (non-premature: 66.2%) groups in patients with severe CHD. Class I (premature) was characterized by the high prevalence of smoking (98.4%), alcohol consumption (38.6%), and opium addiction (38.3%). While in the other class, older patients were people with clinical risk factors, including diabetes (45.5%), obesity (75%), and hypertension (55.9%). Although positive family history has been reported as an important predictor for premature CHD (29, 30), we did not find such a relationship.
The known risk factors (such as diabetes, hypertension, dietary factors, obesity) and lipoprotein-associated risk (low HDL-C levels, higher TGs, and elevated apolipoprotein B levels) could explain premature CAD among South Asians (34). Studies have shown that opium addiction aggravates the known risk factors for CVD and has been considered an independent predictor for CHD mortality (8, 34, 35). According to a clinical study in Iran, about 5% of patients with CABG use opium. There is evidence that Iranian patients have a misconception of opium consumption that opium may decrease the risk of diabetes and hypertension (36). Similar studies determined that opium consumption decreases the age for MI and predisposes to cardiac death (37, 38).
To have a better knowledge of premature CHD risk factors, simultaneous classification of patients according to their conditions appears to be an important contributor.
Our findings have important implications for clinical practice and in particular, underline the importance of screening in lower age populations who have risk markers for premature CHD to adopt prevention strategies in primary and secondary care settings. This may be of benefit in the larger group of patients compared to those looking for a family history of CHD.
We used LCA for distinguishing the pattern of risk factor distribution among our patients, and it worked well. A model of two groups fitted best and was in accordance with two age groups with the significantly different incidence of risk factors. However, there are some points to be addressed. The prevalence rates of smoking, alcohol consumption, and opium addiction were lower among women compared to those among men in our study. Latent class analysis categorized only three women in class I (premature CHD). A larger sample size is probably needed before we can judge the predictors and cutoff point for the age of premature CHD in women. Given the low rate of smoking, alcohol consumption, and opium addiction among women in studies (32), it is probable that CHD in younger women is represented by different determinants like PVD.
The average age of 55 years for men and 60 years for women that we found in our study is related to the Iranian patients with severe CHD who were candidates for CABG, and it cannot be applied for the diagnosis of premature CHD in other groups of patients. Moreover, we did not ask the patients about the time they were diagnosed as patients with CHD even though it is not practical to document the exact time the CHD has begun. However, coronary artery involvement was severe among all participants of this study and they were candidates for CABG, which means they were at the same level regarding the progress of CHD. Additionally, the cross-sectional design of this study restricts causal interpretation of the association between unhealthy behaviors in the development of CHD. The current study was the dataset of the THC-COM study, and it was not possible for authors to include other variables such as the duration of the study.
In this study, we employed LCA to identify clusters of patients with regard to their risk markers and recognize different categories of CHD. The best model fitted two classes that we named premature and non-premature CHD. The latent class I (premature CHD) was characterized by smoking, alcohol consumption, opium addiction, and the history of MI, while class II was characterized by known major risk factors like obesity, diabetes, and hypertension. There was a difference between men and women regarding CHD risk markers.
The original contributions presented in the study are included in the article/Supplementary Material, further inquiries can be directed to the corresponding author/s.
The studies involving human participants were reviewed and approved by Tehran University of Medical Sciences. The patients/participants provided their written informed consent to participate in this study. The study was approved by the Ethics Committee of Tehran University of Medical Sciences and Tabriz University of Medical Sciences (NO. IR.TBZMED.REC.1400.748). All participants gave written informed consent. The data and material is available.
LJ collected the data and wrote the first draft. MN was the main investigator who contributed to designing and helping in recruitment. MA helped in the interpretation of results and manuscript drafting. PS conducted study analysis. All the authors read and approved the manuscript.
The authors declare that the research was conducted in the absence of any commercial or financial relationships that could be construed as a potential conflict of interest.
All claims expressed in this article are solely those of the authors and do not necessarily represent those of their affiliated organizations, or those of the publisher, the editors and the reviewers. Any product that may be evaluated in this article, or claim that may be made by its manufacturer, is not guaranteed or endorsed by the publisher.
We acknowledge the contributions of Tehran Heart Center (Tehran University of Medical Sciences, Tehran, Iran) for providing facilities for this study.
The Supplementary Material for this article can be found online at: https://www.frontiersin.org/articles/10.3389/fcvm.2021.707070/full#supplementary-material
BMI, body mass index; CVD, cardiovascular diseases; CABG, LCA, latent class analysis; MI, myocardial infarction; CHD, coronary heart disease; PVD, peripheral vascular disease; HLP, hyperlipidemia.
1. Naghavi M, Wang H, Lozano R, Davis A, Liang X, Zhou M, et al. Global, regional, and national age-sex specific all-cause and cause-specific mortality for 240 causes of death, 1990-2013: a systematic analysis for the Global Burden of Disease Study (2013). Lancet. (2015) 385:117–71. doi: 10.1016/S0140-6736(14)61682-2
2. Eagle KA, Guyton RA, Davidoff R, Ewy GA, Fonger J, Gardner TJ, et al. ACC/AHA guidelines for coronary artery bypass graft surgery: a report of the American College of Cardiology/American Heart Association task force on Practice Guidelines (Committee to revise the 1991 Guidelines for Coronary Artery Bypass Graft Surgery). J Amer Coll Cardiol. (1999) 34:1262–347.
3. Darba S, Safaei N, Mahboub-Ahari A, Nosratnejad S, Alizadeh G, Ameri H, et al. Direct and indirect costs associated with coronary artery (heart) disease in Tabriz, Iran. Risk Manag Healthc Policy. (2020) 13:969–78. doi: 10.2147/RMHP.S261612
4. Schlatter RP, Hirakata VN, Polanczyk CA. Estimating the direct costs of ischemic heart disease: evidence from a teaching hospital in Brazil, a retrospective cohort study. BMC Cardiovasc Disord. (2017) 17:180. doi: 10.1186/s12872-017-0615-1
5. Pyörälä K, De Backer G, Graham I, Poole-Wilson P, Wood D. Prevention of coronary heart disease in clinical practice. Eur Heart J. (1994) 15:1300–31. doi: 10.1093/oxfordjournals.eurheartj.a060388
6. De Sutter J, De Bacquer D, Kotseva K, Sans S, Pyörälä K, Wood D, et al. Screening of family members of patients with premature coronary heart disease. Results from the EUROASPIRE II family survey. Eur Heart J. (2003) 24:249–57. doi: 10.1016/S0195-668X(02)00386-X
7. Dallongeville J, Grupposo MC, Cottel D, Ferrieres J, Arveiler D, Bingham A, et al. Association between the metabolic syndrome and parental history of premature cardiovascular disease. Eur Heart J. (2006) 27:722–8. doi: 10.1093/eurheartj/ehi717
8. Mulders TA, Meyer Z, van der Donk C, Kroon AA, Ferreira I, Stehouwer CD, et al. Patients with premature cardiovascular disease and a positive family history for cardiovascular disease are prone to recurrent events. Int J Cardiol. (2011) 153:64–7. doi: 10.1016/j.ijcard.2010.08.040
9. Akosah KO, Gower E, Groon L, Rooney BL, Schaper A. Mild hypercholesterolemia and premature heart disease: do the national criteria underestimate disease risk? J Am Coll Cardiol. (2000) 35:1178–84. doi: 10.1016/S0735-1097(00)00556-8
10. Funderburk JS, Maisto SA, Sugarman DE, Wade M. The covariation of multiple risk factors in primary care: a latent class analysis. J Behav Med. (2008) 31:525–35. doi: 10.1007/s10865-008-9176-1
11. Lanza ST, Flaherty BP, Collins LM. Latent class and latent transition analysis. In: Schinka JA, Velicer WF, editors. Handbook of Psychology: Research Methods in Psychology, Vol. 2. Hoboken, NJ: John Wiley & Sons Inc. (2003). p. 663–85.
12. Association AP. Diagnostic and Statistical Manual of Mental Disorders, Revised. Washington DC: American Psychiatric Association. (2000) 943:2000.
13. Najafi M, Jahangiry L, Mortazavi SH, Jalali A, Karimi A, Bozorgi A. Outcomes and long-term survival of coronary artery surgery: the controversial role of opium as risk marker. World J Cardiol. (2016) 8:676. doi: 10.4330/wjc.v8.i11.676
14. Ghanbari J, Mohammadpoorasl A, Jahangiry L, Farhangi MA, Amirzadeh J, Ponnet K. Subgroups of lifestyle patterns among hypertension patients: a latent-class analysis. BMC Med Res Methodol. (2018) 18:127. doi: 10.1186/s12874-018-0607-6
15. Najafi M, Sheikhvatan M, Ataie-Jafari A. Effects of opium use among coronary artery disease patients in Iran. Subst Use Misuse. (2010) 45:2579–81. doi: 10.3109/10826081003793904
16. Najafi M, Sheikhvatan M. Gender differences in coronary artery disease: correlational study on dietary pattern and known cardiovascular risk factors. Int Cardiovasc Res J. (2013) 7:124–9.
17. Nylund-Gibson K, Choi AY. Ten frequently asked questions about latent class analysis. Transl Issues Psychol Sci. (2018) 4:440–61. doi: 10.1037/tps0000176
18. Lloyd-Jones DM, Nam BH, D'Agostino RB Sr, Levy D, Murabito JM, Wang TJ, et al. Parental cardiovascular disease as a risk factor for cardiovascular disease in middle-aged adults: a prospective study of parents and offspring. JAMA. (2004) 291:2204–11. doi: 10.1001/jama.291.18.2204
19. Cannon CP, Battler A, Brindis RG, Cox JL, Ellis SG, Every NR, et al. American College of Cardiology key data elements and definitions for measuring the clinical management and outcomes of patients with acute coronary syndromes. A report of the American College of Cardiology Task Force on Clinical Data Standards (Acute Coronary Syndromes Writing Committee). J Am Coll Cardiol. (2001) 38:2114–30. doi: 10.1016/s0735-1097(01)01702-8
20. Barrett-Connor E, Giardina E-GV, Gitt AK, Gudat U, Steinberg HO, Tschoepe D. Women and heart disease: the role of diabetes and hyperglycemia. Arch Intern Med. (2004) 164:934–42. doi: 10.1001/archinte.164.9.934
21. lberti KG, Zimmet PZ. Definition, diagnosis and classification of diabetes mellitus and its complications. Part 1: diagnosis and classification of diabetes mellitus provisional report of a WHO consultation. Diabet Med. (1998) 15:539–53. doi: 10.1002/(SICI)1096-9136(199807)15:7<539::AID-DIA668>3.0.CO;2-S
22. Whitworth JA, Chalmers J. World health organisation-international society of hypertension (WHO/ISH) hypertension guidelines. Clin Exp Hypertens. (2004) 26:747–52. doi: 10.1081/ceh-200032152
23. WHO. BMI classification. In: Global Database on Body Mass Index 2006. (Retrieved July 27, 2012).
24. Dugani SB, Hydoub YM, Ayala AP, Reka R, Nayfeh T, Ding JF, et al. Risk factors for premature myocardial infarction: a systematic review and meta-analysis of 77 studies. Mayo Clin Proc Innov Qual Outcomes. (2021). 5:783–94. doi: 10.1016/j.mayocpiqo.2021.03.009
25. Dugani SB, Ayala Melendez AP, Reka R, Hydoub YM, McCafferty SN, Murad MH, et al. Risk factors associated with premature myocardial infarction: a systematic review protocol. BMJ Open. (2019) 9(2):e023647. doi: 10.1136/bmjopen-2018-023647
26. Muthén B, Muthén LK. Integrating person-centered and variable-centered analyses: growth mixture modeling with latent trajectory classes. Alcoholism Clin Exp Res. (2000) 24:882–91. doi: 10.1111/j.1530-0277.2000.tb02070.x
27. Muthen BO. Latent variable mixture modeling. In: Marcoulides GA, Schumacker RE, editors. New Developments and Techniques in Structural Equation Modeling. Hillsdale, NJ: Lawrence Erlbaum Associates (2001). p. 1–34.
28. Linzer DA, Lewis JB. poLCA: an R package for polytomous variable latent class analysis. J Stat Softw. (2011) 42:1–29. doi: 10.18637/jss.v042.i10
29. Abdi-Ali A, Shaheen A, Southern D, Zhang M, Knudtson M, White J, et al. Relation between family history of premature coronary artery disease and the risk of death in patients with coronary artery disease. Am J Cardiol. (2016) 117:353–8. doi: 10.1016/j.amjcard.2015.11.008
30. Hajar R. Risk factors for coronary artery disease: historical perspectives. Heart Views. (2017) 18:109–14. doi: 10.4103/HEARTVIEWS.HEARTVIEWS_106_17
31. Farhangi MA, Ataie-Jafari A, Najafi M, Foroushani GS, Tehrani MM, Jahangiry L. Gender differences in major dietary patterns and their relationship with cardio-metabolic risk factors in a year before coronary artery bypass grafting (CABG) surgery period. Arch Iran Med. (2016) 19:470–9.
32. Eslami A, Mozaffary A, Derakhshan A, Azizi F, Khalili D, Hadaegh F. Sex-specific incidence rates and risk factors of premature cardiovascular disease. A long term follow up of the Tehran lipid and glucose study. Int J Cardiol. (2017) 227:826–32. doi: 10.1016/j.ijcard.2016.10.037
33. Sadeghi R, Adnani N, Erfanifar A, Gachkar L, Maghsoomi Z. Premature coronary heart disease and traditional risk factors-can we do better? Int Cardiovasc Res J. (2013) 7:46–50.
34. Sivapalaratnam S, Boekholdt SM, Trip MD, Sandhu MS, Luben R, Kastelein JJ, et al. Family history of premature coronary heart disease and risk prediction in the EPIC-Norfolk prospective population study. Heart. (2010) 96:1985–9. doi: 10.1136/hrt.2010.210740
35. Khademi H, Malekzadeh R, Pourshams A, Jafari E, Salahi R, Semnani S, et al. Opium use and mortality in Golestan Cohort Study: prospective cohort study of 50 000 adults in Iran. Bmj. (2012) 344:e2502. doi: 10.1136/bmj.e2502
36. Alavi SS, Mehrdad R, Makarem J. Prevalence of substance abuse/alcohol consumption and their predictors among patients admitted in operating rooms of a General Educational Hospital, Tehran, Iran. Asian J Pharmaceut Res Health Care. (2016). 8:63. doi: 10.18311/ajprhc/2016/7651
37. Safaii N, Kazemi B. Effect of opium use on short-term outcome in patients undergoing coronary artery bypass surgery. Gen Thorac Cardiovasc Surg. (2010) 58:62–7. doi: 10.1007/s11748-009-0529-7
Keywords: coronary heart diseases, risk markers, premature, cluster analysis, gender
Citation: Jahangiry L, Abbasalizad Farhangi M, Najafi M and Sarbakhsh P (2021) Clusters of the Risk Markers and the Pattern of Premature Coronary Heart Disease: An Application of the Latent Class Analysis. Front. Cardiovasc. Med. 8:707070. doi: 10.3389/fcvm.2021.707070
Received: 08 May 2021; Accepted: 03 November 2021;
Published: 08 December 2021.
Edited by:
Saskia C. A. De Jager, Utrecht University, NetherlandsReviewed by:
Senta Graf, Medical University of Vienna, AustriaCopyright © 2021 Jahangiry, Abbasalizad Farhangi, Najafi and Sarbakhsh. This is an open-access article distributed under the terms of the Creative Commons Attribution License (CC BY). The use, distribution or reproduction in other forums is permitted, provided the original author(s) and the copyright owner(s) are credited and that the original publication in this journal is cited, in accordance with accepted academic practice. No use, distribution or reproduction is permitted which does not comply with these terms.
*Correspondence: Mahdi Najafi, bWFoZGkubmFqYWZpQHV3by5jYQ==; bWFoZGluYWphZmlrb29AZ21haWwuY29t
Disclaimer: All claims expressed in this article are solely those of the authors and do not necessarily represent those of their affiliated organizations, or those of the publisher, the editors and the reviewers. Any product that may be evaluated in this article or claim that may be made by its manufacturer is not guaranteed or endorsed by the publisher.
Research integrity at Frontiers
Learn more about the work of our research integrity team to safeguard the quality of each article we publish.