- Department of Cardiovasology, Shijiazhuang People's Hospital, Shijiazhuang, China
Background: Morbidity and mortality of heart failure (HF) post-myocardial infarction (MI) remain elevated. The aim of this study was to find potential long non-coding RNAs (lncRNAs) and mRNAs in the progression from acute myocardial infarction (AMI) to myocardial fibrosis (MF) to HF.
Methods: Firstly, blood samples from AMI, MF, and HF patients were used for RNA sequencing. Secondly, differentially expressed lncRNAs and mRNAs were obtained in MF vs. AMI and HF vs. MF, followed by functional analysis of shared differentially expressed mRNAs between two groups. Thirdly, interaction networks of lncRNA-nearby targeted mRNA and lncRNA-co-expressed mRNA were constructed in MF vs. AMI and HF vs. MF. Finally, expression validation and diagnostic capability analysis of selected lncRNAs and mRNAs were performed.
Results: Several lncRNA-co-expressed/nearby targeted mRNA pairs including AC005392.3/AC007278.2-IL18R1, AL356356.1/AL137145.2-PFKFB3, and MKNK1-AS1/LINC01127-IL1R2 were identified. Several signaling pathways including TNF and cytokine–cytokine receptor interaction, fructose and mannose metabolism and HIF-1, hematopoietic cell lineage and fluid shear stress, and atherosclerosis and estrogen were selected. IL1R2, IRAK3, LRG1, and PLAC4 had a potential diagnostic value for both AMI and HF.
Conclusion: Identified AC005392.3/AC007278.2-IL18R1, AL356356.1/AL137145.2-PFKFB3, and MKNK1-AS1/LINC01127-IL1R2 lncRNA-co-expressed/nearby targeted mRNA pairs may play crucial roles in the development of AMI, MF, and HF.
Introduction
Heart failure (HF), the terminal stage of a wide range of cardiovascular diseases, leads to the decompensation of the heart's ability to contract or relax (1). In most clinical cases, HF is caused by myocardial infarction (MI). Following MI, a lot of cardiac cells will die in response to ischemia (2). As the adult heart has a very limited capacity to regenerate after injury, the lost cardiac cells will be replaced by fibrotic scars. The process is accompanied by remodeling of the surrounding myocardium and eventually results in impaired cardiac function. HF is significantly associated with cardiac hypertrophy, valvular disease, dilated cardiomyopathy, hypertrophic cardiomyopathy, age, obesity, diabetes, and hypertension (3, 4). Despite effective treatments targeted at improving HF, the 5-year mortality rate of the disease varies from 45 to 60% (5). Therefore, in-depth understanding of pathological mechanisms of HF is very important to develop effective therapy and assessment of patient prognosis.
Long non-coding RNAs (lncRNAs) regulate mRNA expression at the transcriptional and post-transcriptional levels. It has been shown that lncRNAs are emerging as essential players in heart development, atherosclerosis, and HF (6). In addition, the major component of HF disease management is diagnosis and monitoring of disease progression. In this study, we tried to find potential differentially expressed lncRNAs and mRNAs in the blood of patients with acute myocardial infarction (AMI), myocardial fibrosis (MF), and HF. We supposed that these potential differentially expressed lncRNAs and interacted mRNAs may be involved in the disease progression from AMI, MF and HF.
Materials and Methods
Subjects
In this study, three patients with AMI, three patients with MF, and three patients with HF were enrolled. The inclusion criteria of AMI patients were as follows: (1) time of chest pain or distress > 30 min within 24 h, and the level of the myocardial enzyme of creatine kinase (CK)-MB and cardiac troponin T (cTnT) was higher than the normal range; (2) patients had their first episode; (3) patients received no medical or surgical treatment prior to admission; (4) patients had blood samples before admission, at discharge, and 6 months after MI; and (5) patients had complete clinical data, including gender, age, height, and weight. The exclusion criteria of AMI patients were as follows: (1) patients with myocarditis and other diseases caused by chest pain or distress; (2) patients with a history of renal failure, advanced liver disease, malignant tumors, and other inflammatory diseases such as psoriasis and rheumatoid arthritis; (3) recurrent patients; (4) patients with incomplete clinical data; and (5) patients with missing blood samples before hospitalization, at discharge, and 6 months after MI. Those patients diagnosed with MF (1 year after MI) were enrolled. Depending on the diagnostic criteria, those patients with HF caused by MI were also enrolled.
Blood Samples
The blood of AMI, MF, and HF patients was collected. Ethical approval was obtained from the ethics committee of the Shijiazhuang People's Hospital. In addition, informed written consent was obtained from all individuals.
RNA Isolation and Sequencing
The total RNA was extracted from the blood sample using the RNAliquid Reagent according to the manufacturer's protocols. Agilent 2100 was utilized to test the RNA integrity number (RIN) (7). RNA conditions to be met for sequencing were as follows: (1) the total amount (required for single database construction) is 5 μg; (2) the concentration was ≥200 ng/μl; and (3) the value of OD260/280 was between 1.8 and 2.2. After total RNA DNase I digestion, removal of rRNA, RNA interruption, synthesis of reverse transcriptional one and two strands, end repair, cDNA with an “A” at the 3′ end, connection of cDNA 5′ adapter, digestion of two strands of cDNA, PCR reaction, and product recovery and library quality inspection, the Illumina Hiseq x-ten platform (PE150 strategy) (8) was used to perform RNA sequencing for lncRNA. After RNA fragment selection, 3' connector connection, reverse primer annealing, 5' connector connection, the synthesis of a strand of cDNA, PCR amplification, library fragment selection, library quantification, and pooling annularization, BGISEQ-500 platform (SE50 strategy) (9) was used to perform RNA sequencing for mRNA. The Combat function for the SVA package was used to eliminate batch effect (10). The Fastx-Toolkit was used to trim 5' and 3' segments of reads to remove bases with mass <20 and delete reads with N >10%. For lncRNA analysis, the cleaned gene sequencing reads were aligned to the reference genome (GRCh38) for matching via HISAT2 (11) (https://ccb.jhu.edu/software/hisat2/index.shtml). Stringtie (12) (http://www.ccb.jhu.edu/software/stringtie/) was utilized to quantify the expression levels of lncRNA and mRNA. For mRNA analysis, the Rfam (13) was used for annotation analysis on measured small RNA. The mature mRNA and mRNA precursor sequences were downloaded from miRBase (14). The expression of mRNA was quantified with miRDeep2 (15). Finally, DEGSeq2 package (16) was used to compare the expression difference of lncRNA and mRNA between the two groups in the R environment. The value of fragments per kilobase of exon per million mapped reads (FPKM) of each gene/transcript in the sample was calculated according to the comparison results of all samples with the reference genome. The value was regarded as the expression amount of gene/transcript in the sample.
Identification of Differentially Expressed lncRNAs and mRNAs in MF vs. AMI and HF vs. MF
Differential expression analysis of lncRNAs and mRNAs in MF vs. AMI and HF vs. MF was evaluated in the R-bioconductor package DESeq2. Firstly, in order to normalize for sequencing depth, the original read count performed normalization. Then, the hypothesis testing probability (p-value) was obtained by the statistical model. Thirdly, multiple hypothesis test correction was carried out to obtain the corrected p-value [false discovery rate (FDR)]. Those differentially expressed lncRNAs and mRNAs were identified under the criterion of p-value < 0.05 and |log2Fold change| ≥ 1.
Functional Analysis of Common Differentially Expressed mRNAs in MF vs. AMI and HF vs. MF
In order to study the biological function of common differentially expressed mRNAs in MF vs. AMI and HF vs. MF, functional analysis including Gene Ontology (GO) classification (17) and Kyoto Encyclopedia of Genes and Genomes (KEGG) pathway enrichment analysis (18) was performed through CPDB (http://cpdb.molgen.mpg.de/CPDB). Significantly enriched GO and KEGG terms were identified under the criterion of p-value < 0.05.
Nearby Target and Co-expression Analysis Between lncRNA and mRNA in MF vs. AMI and HF vs. MF
In order to identify nearby targeted differentially expressed mRNAs of differentially expressed lncRNAs in MF vs. AMI and HF vs. MF, the mRNAs transcribed within a 100-kb window up- or downstream of lncRNAs (19) were searched by using BEDTools (20). In addition, the Pearson correlation coefficient (cor) of lncRNAs and mRNA was calculated using the cor.test function in R. The correlation pairs with |cor| ≥ 0.98 and p-value < 0.05 were considered in that lncRNAs were significantly co-expressed with mRNAs. Interaction networks of lncRNA-nearby mRNA and lncRNA-co-expressed mRNA were visualized by using Cytoscape (21).
In vitro Validation of Key Differentially Expressed lncRNAs and mRNAs
In total, five patients with AMI (three patients from RNA sequencing), three patients with MF (two patients from RNA sequencing), and five patients with HF (three patients from RNA sequencing) were enrolled. The inclusion criteria of patients with AMI, MF, and HF were consistent with that for RNA sequencing. Ethical approval was obtained from the ethics committee of the Shijiazhuang People's Hospital and informed written consent was obtained from all individuals.
Total RNA of the blood samples was extracted according to the manufacturer's protocols. Then, qPCR was performed in an ABI 7300 qPCR system with SYBR® Green PCR Master Mix. All reactions were performed in triplicate. ACTB and GAPDH were used for the internal reference. Relative gene expression was analyzed by the fold change method. p < 0.05 is the significance level.
Electronic Validation and Diagnostic Analysis of Key Differentially Expressed lncRNAs and mRNAs
In order to further validate the expression of the identified key differentially expressed lncRNAs and mRNAs, the GSE21125 dataset (HF cases:normal controls = 18:9) and the GSE48060 dataset (AMI cases:normal controls = 31:21) were used for expression validation. The expression pattern was displayed in the box plots. In addition, we also performed the ROC analyses to assess the diagnostic value of lncRNAs and mRNAs in the above two datasets.
Results
Clinical Information of Enrolled Individuals for RNA Sequencing and in vitro Validation
A total of 14 patients (5 AMI patients, 4 MF patients, and 5 HF patients) were enrolled in both RNA sequencing and in vitro validation. The clinical information of these individuals is presented in Table 1. The chisq.test of gender showed that there was no statistical difference of gender between male and female patients in three groups (p-value = 0.638).
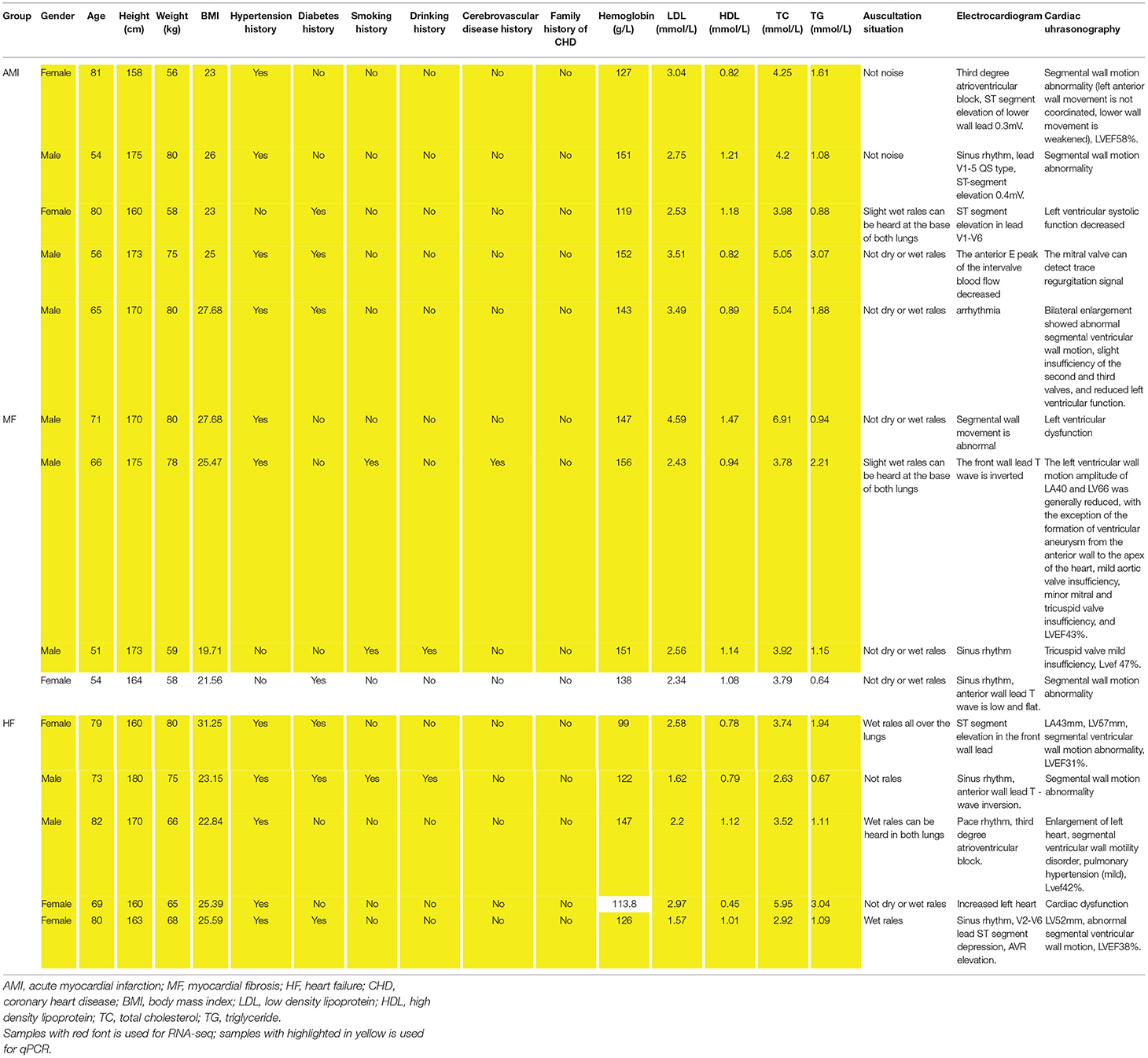
Table 1. The clinical information of all individuals enrolled in RNA sequencing and in vitro validation.
Differentially Expressed lncRNAs and mRNAs in MF vs. AMI and HF vs. MF
A total of 248 differentially expressed lncRNAs (Supplementary Table 1) and 936 differentially expressed mRNAs (Supplementary Table 2) were obtained in MF vs. AMI. The volcano plot and hierarchical clustering diagram of lncRNAs and mRNAs are shown in Figure 1. The top 10 up- and downregulated differentially expressed lncRNAs and mRNAs are shown in Tables 2, 3, respectively. In addition, a total of 377 differentially expressed lncRNAs (Supplementary Table 3) and 877 differentially expressed mRNAs (Supplementary Table 4) were obtained in HF vs. MF. The volcano plot and hierarchical clustering diagram of lncRNAs and mRNAs are shown in Figure 2. In addition, the top 10 up- and downregulated differentially expressed lncRNAs and mRNAs are listed in Tables 4, 5, respectively. Interestingly, a total of 87 common differentially expressed lncRNAs (such as AC005392.3, AC007278.2, AL356356.1, AL137145.2, MKNK1-AS1, LINC01127, and PLAC4) and 341 differentially expressed mRNAs (such as IL18R1, PFKFB3, IL1R2, IRAK3, FKBP5, LRG1, IKZF2, and RNASE1) were identified between MF vs. AMI and HF vs. MF. Beside PLAC4 and IKZF2, all above common lncRNAs and mRNAs were downregulated in MF vs. AMI, while they were upregulated in HF vs. MF. On the contrary, PLAC4 and IKZF2 were upregulated in MF vs. AMI, while they were downregulated in HF vs. MF.
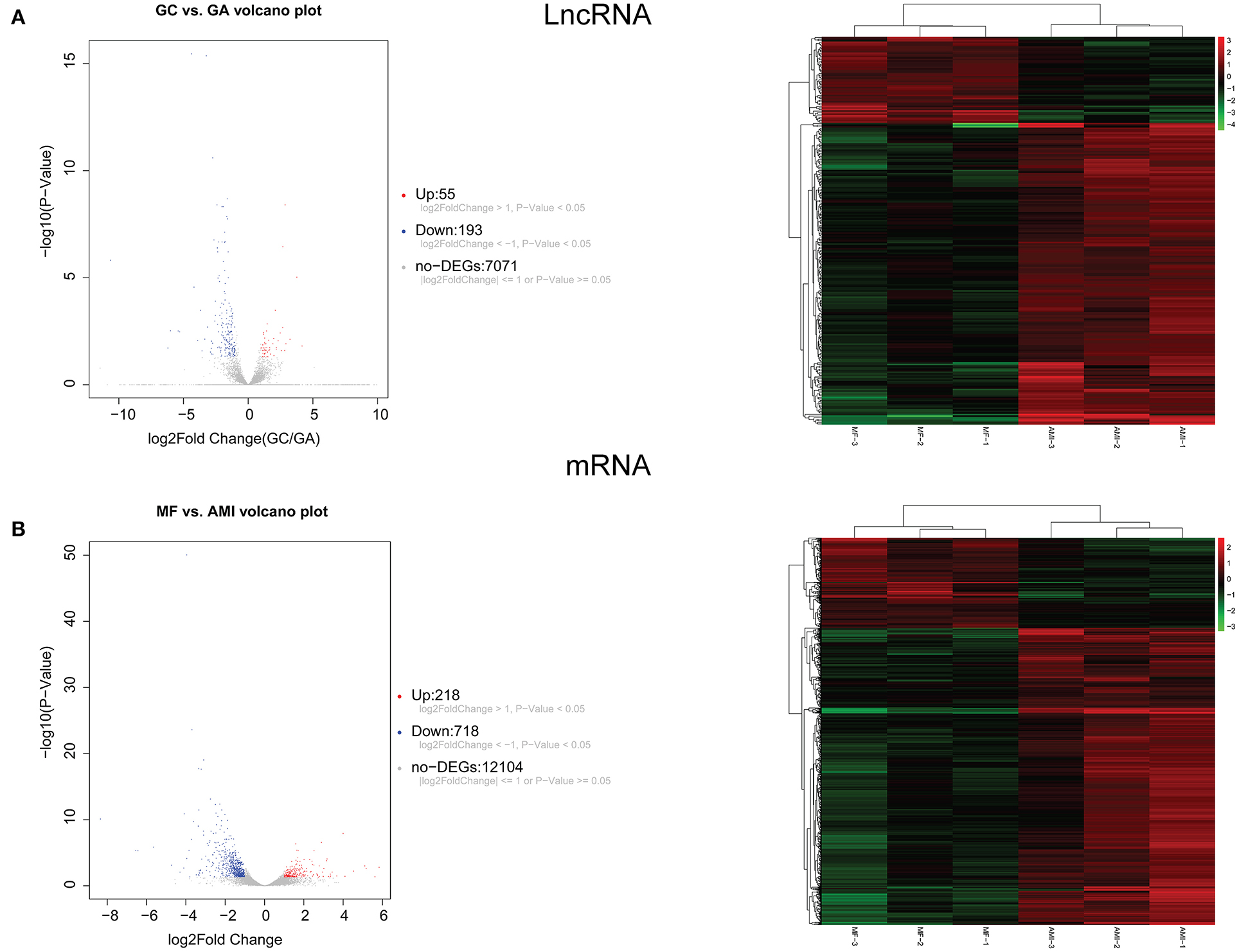
Figure 1. The volcano plot and hierarchical clustering diagram of lncRNAs (A) and mRNAs (B) in MF vs. AMI. In the volcano, the x-axis and y-axis present Log2 fold change and –log10 p-value, respectively. Red and blue color represent upregulation and downregulation, respectively. In the hierarchical clustering diagram, the diagram presents the result of a two-way hierarchical clustering of lncRNAs/mRNAs and sample. Each row represents a differentially expressed lncRNA/mRNA, and each column represents a sample. Differentially expressed lncRNAs/mRNAs clustering tree is shown on the top right corner. The color scale illustrates the relative level of differentially expressed genes: red, below the reference channel; green, higher than the reference.
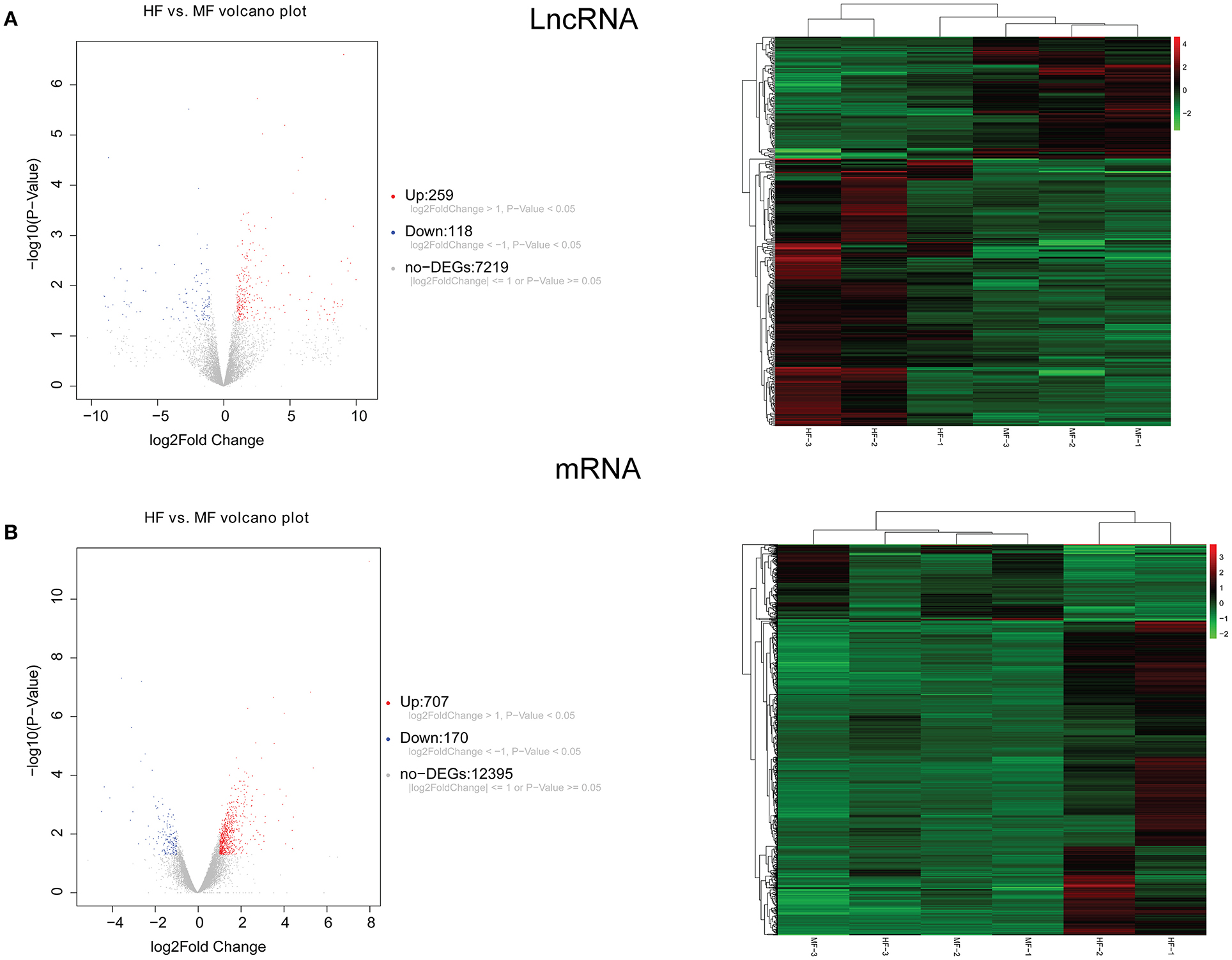
Figure 2. The volcano plot and hierarchical clustering diagram of lncRNAs (A) and mRNAs (B) in HF vs. MF. In the volcano, the x-axis and y-axis present Log2 fold change and –log10 p-value, respectively. Red and blue color represent upregulation and downregulation, respectively. In the hierarchical clustering diagram, the diagram presents the result of a two-way hierarchical clustering of lncRNAs/mRNAs and sample. Each row represents a differentially expressed lncRNA/mRNA, and each column represents a sample. Differentially expressed lncRNAs/mRNAs clustering tree is shown on the top right corner. The color scale illustrates the relative level of differentially expressed genes: red, below the reference channel; green, higher than the reference.
Functional Analysis of Common Differentially Expressed mRNAs Between MF vs. AMI and HF vs. MF
Functional analysis of common differentially expressed mRNAs between MF vs. AMI and HF vs. MF showed that cell activation, whole membrane, and drug binding were the most significantly enriched GO terms (Figure 3). In addition, several enriched signaling pathways in the KEGG analysis were identified, including TNF signaling pathway and cytokine–cytokine receptor interaction (involving IL18R1), fructose and mannose metabolism and HIF-1 signaling pathway (involving PFKFB3), hematopoietic cell lineage and fluid shear stress and atherosclerosis (involving IL1R2), and estrogen signaling pathway (involving FKBP5). All significantly enriched KEGG signaling pathways and involved mRNAs are listed in Table 6.
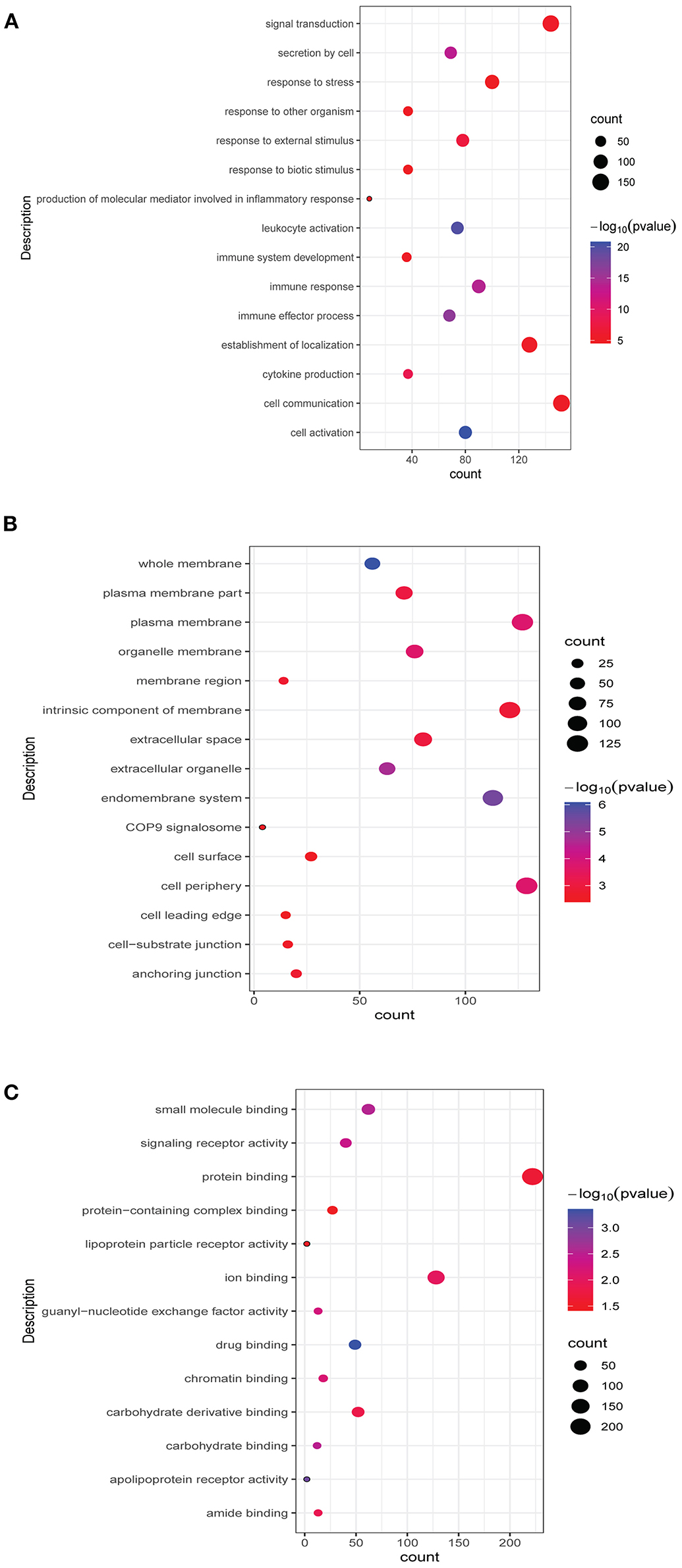
Figure 3. Top 15 significant enrichment GO terms of common differentially expressed mRNAs between MF vs. AMI and HF vs. MF. The x-axis and y-axis present the number of enriching mRNAs and GO terms, respectively. (A), biological progress; (B), cellular component; (C), molecular function.
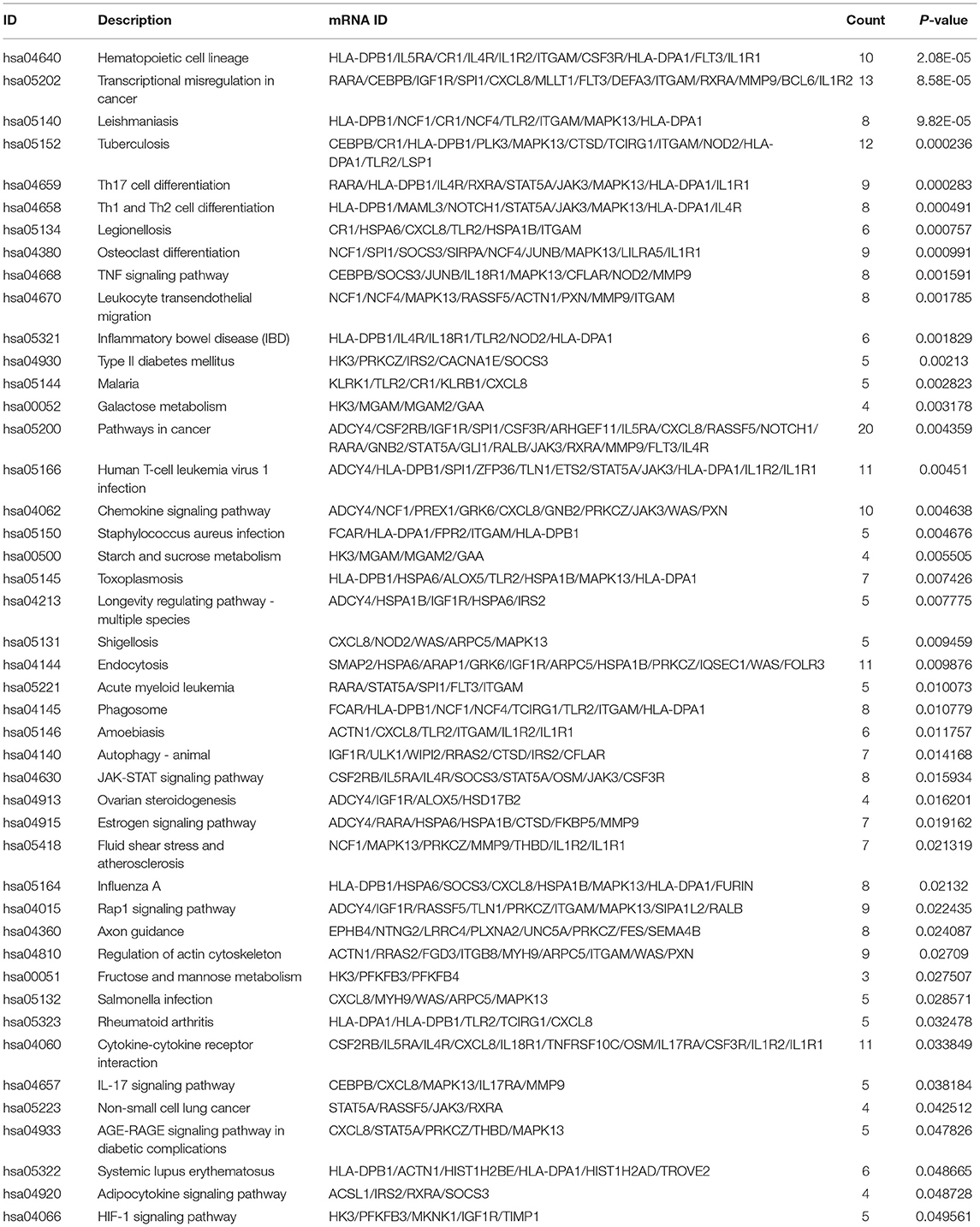
Table 6. All significantly enriched KEGG signaling pathways of common differentially expressed mRNAs between MF vs. AMI and HF vs. MF.
Nearby Target and Co-expressed Analysis Between lncRNAs and mRNAs in MF vs. AMI and HF vs. MF
A total of 209 lncRNA-nearby targeted mRNA pairs (including 150 lncRNA and 155 mRNAs) and 171 lncRNA-nearby targeted mRNA pairs (including 109 lncRNA and 139 mRNAs) were obtained in MF vs. AMI and HF vs. MF, respectively. Interestingly, some common lncRNA-nearby targeted mRNA pairs between MF vs. AMI and HF vs. MF were found, such as AC007278.2-IL18R1, AL137145.2-PFKFB3, and LINC01127-IL1R2. In addition, a total of 1214 lncRNA-co-expressed mRNA pairs (including 80 lncRNA and 300 mRNAs) and 2069 lncRNA-co-expressed mRNA pairs (including 78 lncRNA and 288 mRNAs) were obtained in MF vs. AMI and HF vs. MF, respectively. Significantly, a total of 186 common lncRNA-co-expressed mRNA pairs were identified between MF vs. AMI and HF vs. MF. For instance, AC005392.3-IL18R1, AL356356.1-PFKFB3, and MKNK1-AS1-IL1R2 were found. The interaction network of common lncRNA-co-expressed mRNA pairs between MF vs. AMI and HF vs. MF is shown in Figure 4.
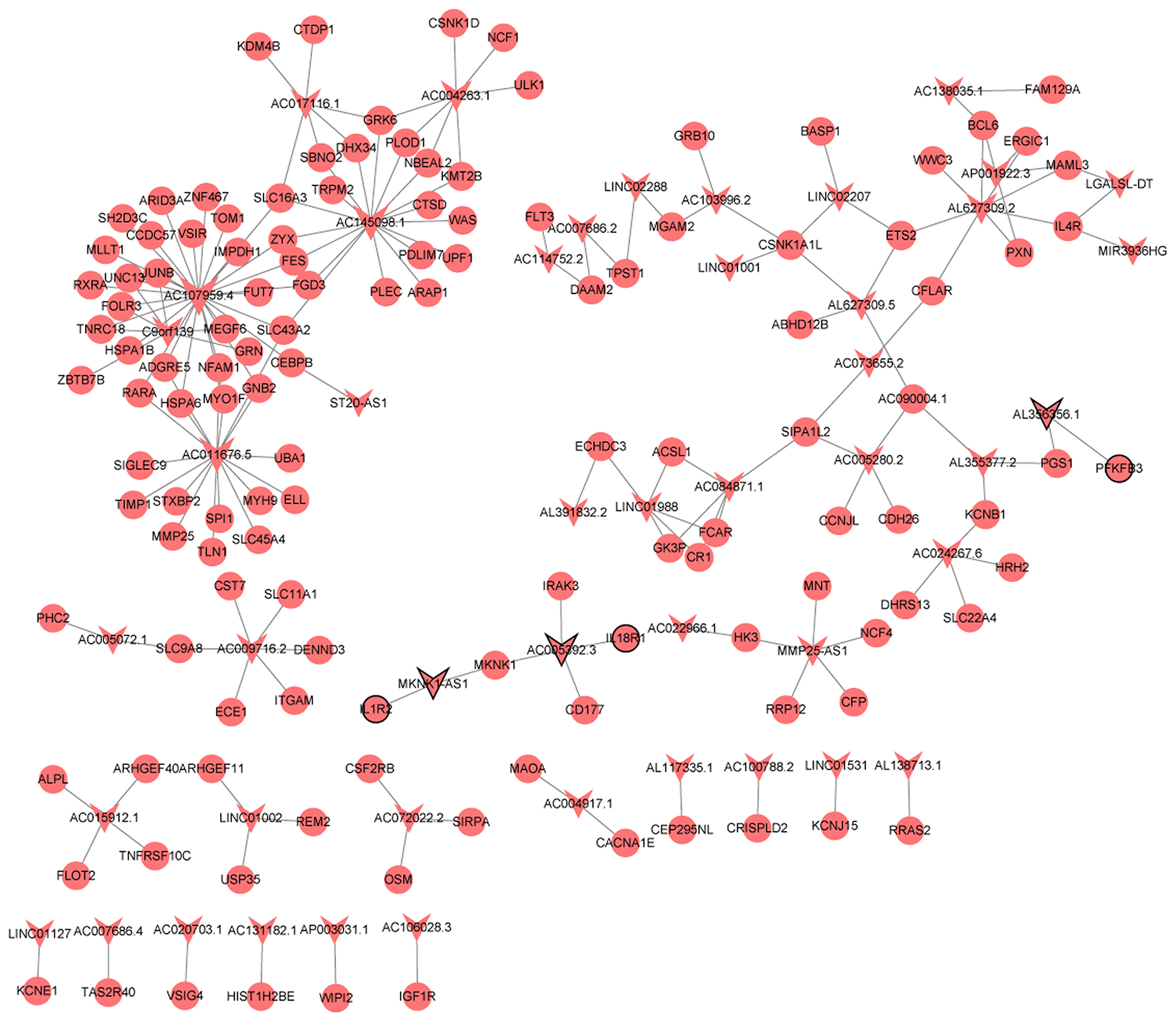
Figure 4. The interaction network of common lncRNA-co-expressed mRNA pairs between MF vs. AMI and HF vs. MF. The arrow and circle represent lncRNA and co-expressed mRNA, respectively. Arrow/circle with a black border represents core lncRNA/mRNA.
In vitro Validation
Five patients with AMI (three patients from RNA sequencing), three patients with MF (two patients from RNA sequencing), and five patients with HF (three patients from RNA sequencing) were included. Based on bioinformatic analysis and literature retrieval results, we selected one differentially expressed lncRNA (PLAC4) and eight differentially expressed mRNA (IL18R1, PFKFB3, IL1R2, IRAK3, FKBP5, LRG1, IKZF2, and RNASE1) for validation (Figure 5). PLAC4 was upregulated in MF vs. AMI, while it was downregulated in HF vs. MF. Besides FKBP5, all above mRNAs were downregulated in MF vs. AMI, while they were upregulated in HF vs. MF. The validated result was basically in line with the RNA sequencing analysis. In addition, the expression validation of IL18R1, IL1R2, LRG1, and RNASE1 in additional HF and MF patients was also performed (Supplementary Figure 1). The method was similar to the description before. The expression trend of these genes was upregulated. Some genes were not expressed significantly. Small sample size may lead to the bias. Larger numbers of samples are further needed.
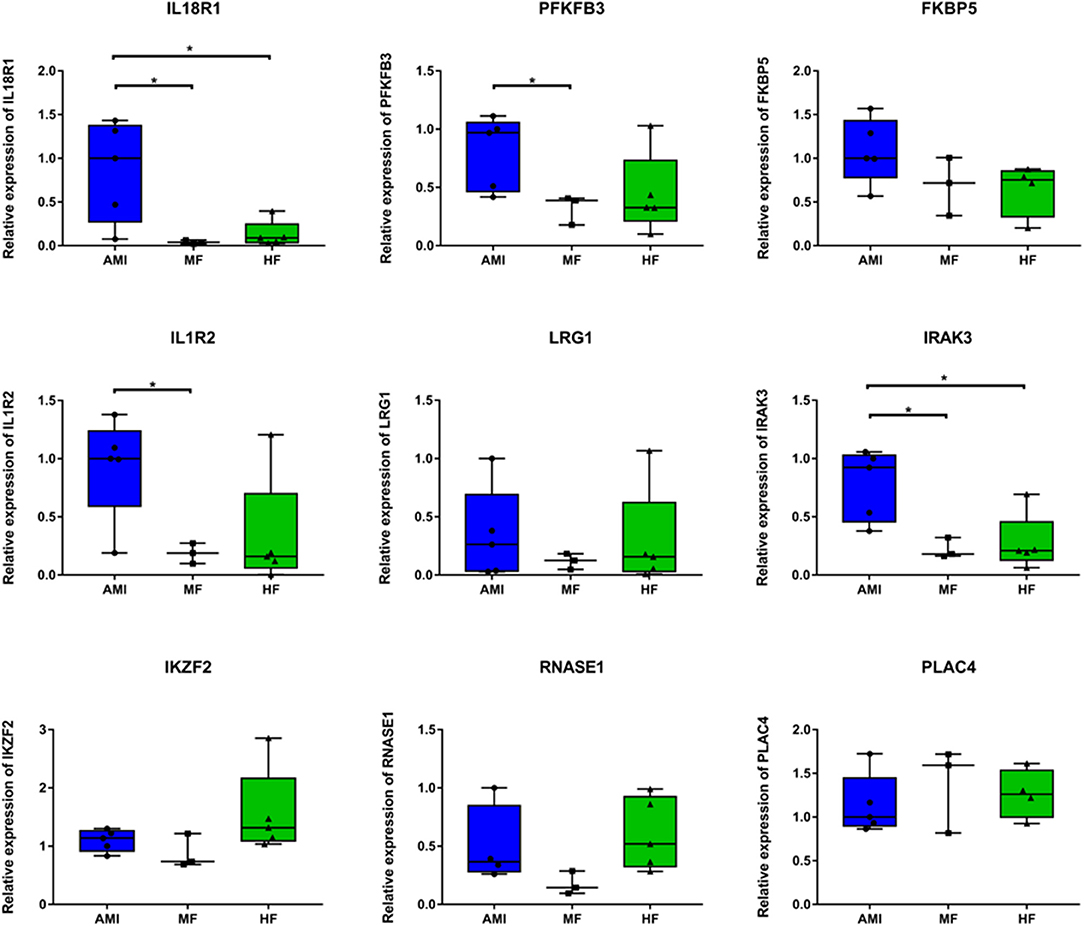
Figure 5. The in vitro qPCR validation of key differentially expressed lncRNAs and mRNAs. *p < 0.05.
Electronic Validation and Diagnostic Analysis of Key Differentially Expressed lncRNAs and mRNAs
Firstly, four key differentially expressed mRNAs including FKBP5, IL1R2, IRAK3, and LRG1 were randomly selected for expression validation in the GSE21125 of HF dataset (Figure 6). FKBP5, IL1R2, IRAK3, and LRG1 were upregulated in HF patients compared with normal controls. This further suggested that upregulation of these mRNAs may play a critical role in the development of HF. In addition, ROC curve analysis was carried out to assess the diagnosis ability of FKBP5, IL1R2, IRAK3, LRG1, RNASE1, and PLAC4 for HF (Figure 7). AUC values of FKBP5, IL1R2, IRAK3, LRG1, RNASE1, and PLAC4 were all more than 0.6, which suggested that they have a potential diagnostic value for HF. Secondly, six key differentially expressed mRNAs including IKZF2, IL1R2, IL18R1, IRAK3, LRG1, and PFKFB3 were randomly selected for expression validation in the GSE48060 of the AMI dataset (Figure 8). IL1R2, IRAK3, LRG1, and PFKFB3 were significantly upregulated in AMI patients compared with normal controls. IKZF2 and IL18R1 were remarkably downregulated in AMI patients compared with normal controls. This indicated that these mRNAs may play a significant role in AMI. In addition, ROC curve analysis was performed to assess the diagnosis ability of IKZF2, IL1R2, IL18R1, IRAK3, LRG1, PFKFB3, LINC01127, and PLAC4 for AMI (Figure 9). AUC values of IKZF2, IL1R2, IL18R1, IRAK3, LRG1, PFKFB3, LINC01127, and PLAC4 were all more than 0.6, which suggested that they have a potential diagnostic value for AMI. It is noted that IL1R2, IRAK3, LRG1, and PLAC4 could be considered as potential diagnostic markers for both AMI and HF.
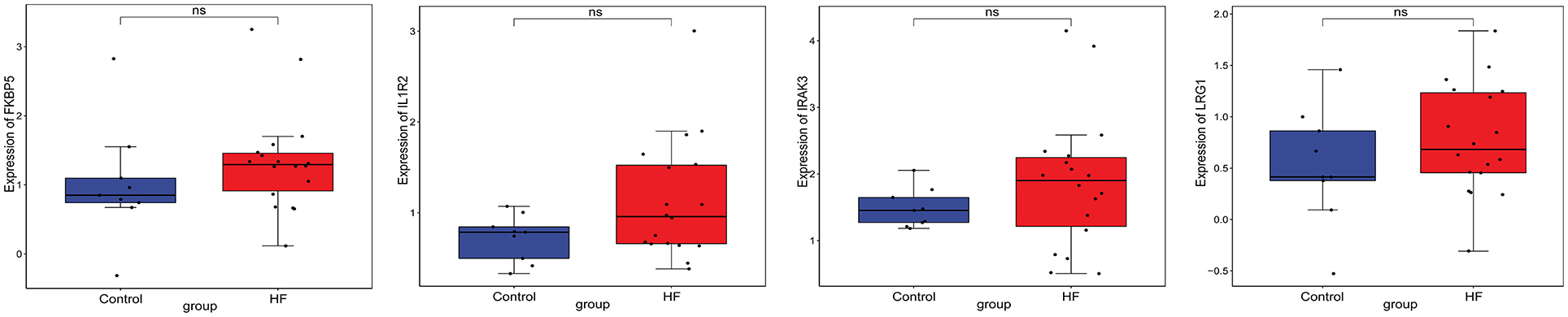
Figure 6. The box plot of FKBP5, IL1R2, IRAK3, and LRG1 in GSE21125 of the HF dataset. Ns, no significant difference.
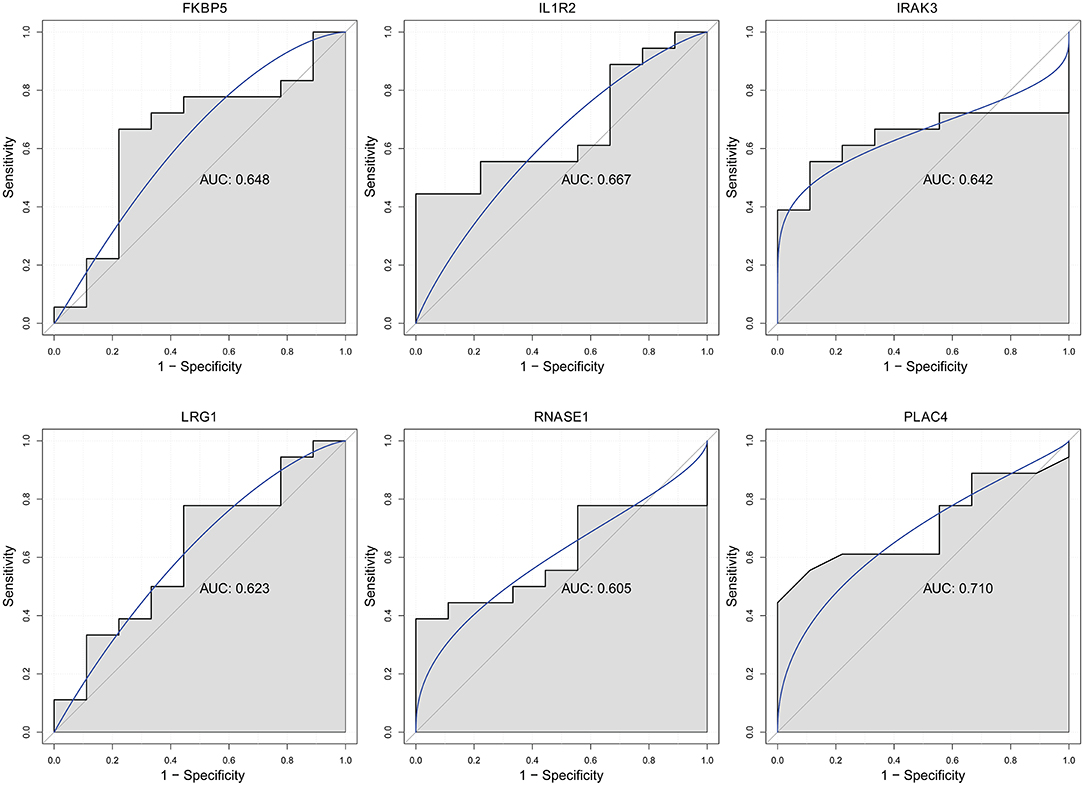
Figure 7. The ROC curves of FKBP5, IL1R2, IRAK3, LRG1, RNASE1, and PLAC4 in HF. The ROC curves were used to show the diagnostic ability with 1 – specificity and sensitivity.
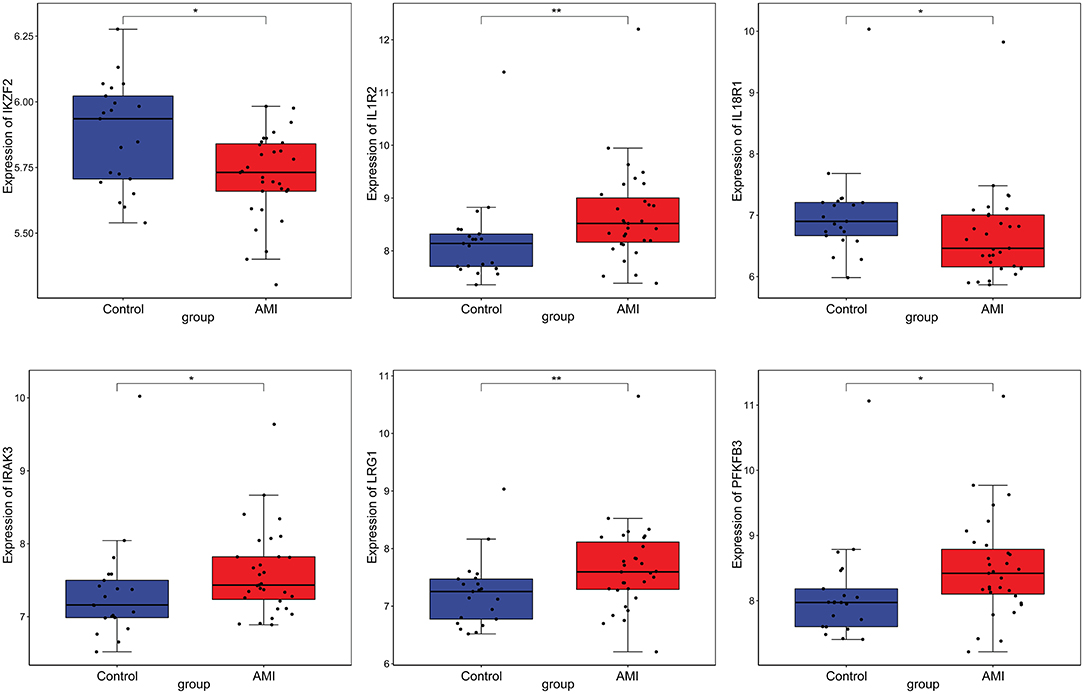
Figure 8. The box plot of IKZF2, IL1R2, IL18R1, IRAK3, LRG1, and PFKFB3 in GSE48060 of the AMI dataset. *p < 0.05; **p < 0.01.
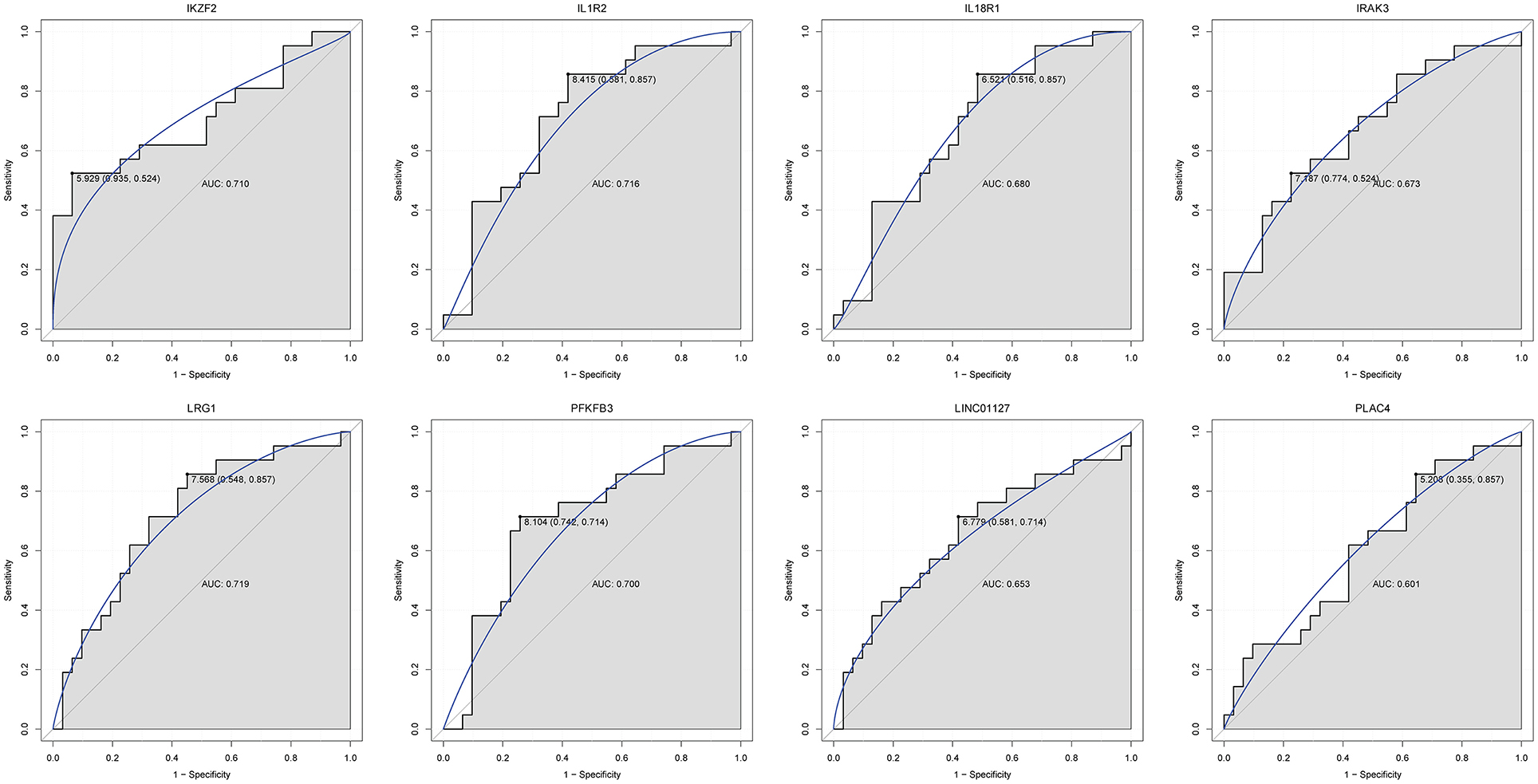
Figure 9. The ROC curves of IKZF2, IL1R2, IL18R1, IRAK3, LRG1, PFKFB3, LINC01127, and PLAC4 in AMI. The ROC curves were used to show the diagnostic ability with 1 – specificity and sensitivity.
Discussion
Up to now, no reports about AC005392.3 have been recorded. The expression level of AC007278.2 is upregulated in peripheral blood mononuclear cells in multiple sclerosis (22). The IL-18 cytokine, coded by interleukin 18 receptor 1 (IL18R1), has been found to be involved in atherosclerosis and cardiovascular complications (23). Bochmann et al. found that IL18R1 is a high-confidence marker for epicardial cells (24). The single nucleotide polymorphism of the IL18R1 gene has been found in cardiovascular disease, such as coronary artery disease (25, 26). In addition, the expression level of IL18R1 is significantly altered in systemic and arterial bed-dependent atherosclerosis plaques (27). IL18R1 is upregulated in patients with acute ST elevation MI (28, 29). In this study, we first found the association between AC005392.3, AC007278.2, and IL18R1. This suggested that AC007278.2 and IL18R1 may be associated with atherosclerosis and cardiovascular complications, which may provide a novel field in understanding the disease progression of AMI, MF, and HF. Moreover, IL18R1 could be considered as a potential diagnostic biomarker for AMI patients.
AL356356.1 is significantly related to the clinical traits of clear cell renal cell carcinoma patients and could serve as a predictor for the disease (30). The highest percentage of candidate fusion transcripts of AL137145.2-6-phosphofructo-2-kinase/fructose-2, 6-biphosphatase 3 (PFKFB3) was found in non-small cell lung cancer (31). In cerebrovascular disease of moyamoya disease, AL137145.2 acts as ceRNAs to regulate C-X-C motif chemokine ligand 16 (CXCL16) through miR-107 (31). It is noted that PFKFB3-regulated glycolysis in endothelial cells also plays an important role in vascular sprouting (32, 33). PFKFB3 also maintains endothelial cells in a quiescent state to reduce injury- and inflammation-induced pathological processes including atherosclerosis (34–36). Higher levels of PFKFB3 have been detected in patients with symptomatic atherosclerosis and incident MI (37, 38). In the present study, we found that PFKFB3 was co-expressed with AL356356.1. Moreover, it is a nearby target mRNA of AL137145.2. Furthermore, PFKFB3 had a potential diagnostic value for AMI patients. Interaction pairs of AL356356.1/AL137145.2-PFKFB3 may be involved in maintaining the function of endothelial cells in the process of AMI, MF, and HF.
Dysregulated MKNK1-AS1 could predict adverse cardiovascular outcomes in patients with end-stage renal disease (39). It is reported that LINC01127 promotes the development of ovarian tumors (40). Upregulation of LINC01127 has been observed in clear cell renal cell carcinoma (41). Interleukin 1 receptor type 2 (IL1R2) is associated with the inflammatory response, which leads to the development of atherosclerotic plaques (42, 43). Additionally, DNA methylation and single nucleotide polymorphism are respectively found in subclinical cardiovascular disease and coronary heart disease (44, 45). It is pointed out that IL1R2 is upregulated in vasculitis and AMI patients (46, 47). Herein, we found the interaction pairs of MKNK1-AS1/LINC01127-IL1R2 in AMI, MF, and HF, which indicated that these lncRNAs and mRNAs play a role in atherosclerosis. In addition, IL1R2 could be considered as a potential diagnostic biomarker for both AMI and HF patients.
Besides interaction with co-expressed/nearby lncRNAs, IL18R1, PFKFB3, and IL1R2 were also significantly involved in some signaling pathways according to the KEGG analysis. For example, IL18R1 was enriched in the TNF signaling pathway and cytokine–cytokine receptor interaction; PFKFB3 was enriched in fructose and mannose metabolism and HIF-1 signaling pathway; IL1R2 was enriched in hematopoietic cell lineage and fluid shear stress and atherosclerosis. The TNF signaling pathway is linked to cardiac remodeling following MI (48). It has been found that TNF-α is involved in the pathogenesis and progression of MF (49). The clinical trial testing of anti-TNF-α drugs has been performed in patients with chronic HF (50, 51). It has been demonstrated that cytokine–cytokine receptor interaction plays an important role in the occurrence and development of AMI and HF (47, 52). Increased activity of fructose and mannose metabolism is found in acute myocardial ischemia injury (53). Parisi et al. found that HIF-1 had the diagnostic potential effect of ischemia, which can effectively reflect the process of AMI to a certain extent (54). Moreover, lack of HIF-1 causes angiogenesis disorders and MF, which leads to HF (55). Hematopoietic stem cells have a potential regenerative ability in the AMI xenotransplantation (56). In addition, hematopoietic cells can trans-differentiate into cardiac fibroblasts when ischemic injury occurs (57). Westenbrink et al. found that HF affected multiple hematopoietic lineages (58). Reduced shear stress and low wall shear stress gradient are considered to increase the possibility of atherosclerosis (59, 60). Atherosclerosis is the most common cause of MI and HF (61, 62). Thus, it can be seen that the abovementioned signaling pathways and enriched genes play an important role in cardiovascular disease.
Between MF vs. AMI and HF vs. MF, we also found several other common differentially expressed lncRNAs (such as PLAC4) and differentially expressed mRNAs including interleukin 1 receptor associated kinase 3 (IRAK3), leucine-rich alpha-2-glycoprotein 1 (LRG1), ribonuclease A family member 1 (RNASE1), FKBP prolyl isomerase 5 (FKBP5), and IKAROS family zinc finger (IKZF2). PLAC4 is associated with congenital heart disease and hypertrophic cardiomyopathy (63, 64). The expression level of IRAK3 is significantly increased 2 days post-MI (65). Upregulation of LRG1 has been found in incident MI (38). In mouse models of MI, LRG1 ablation leads to aggravate MF and heart dysfunction (66). In addition, LRG1 participates in vascular remodeling during HF (67). RNASE1 is upregulated in patients with a history of early MI (68). It is speculated that RNASE1 could be considered as a novel prognostic biomarker for the process of HF after AMI (69). It has been demonstrated that FKBP5 is upregulated and plays a crucial role in endothelial platelet aggregation in AMI patients (70, 71). IKZF2 is overexpressed in the left ventricular myocardium (72). In this study, we found that IRAK3, LRG1, RNASE1, and FKBP5 were downregulated in MF vs. AMI, while they were upregulated in HF vs. MF. On the contrary, PLAC4 and IKZF2 were upregulated in MF vs. AMI, while they downregulated in HF vs. MF. Thus, it can be seen that these genes may be involved in the function of vascular endothelial cells. In addition, FKBP5 was remarkably enriched in the estrogen signaling pathway. In the serum, estrogen receptor β activation reduces the infarct size and lowers the levels of myocardial enzymes (73). In addition, estrogen attenuates MF in HF mainly via estrogen receptor β (74). It is indicated that the estrogen signaling pathway (in which FKBP5 is involved) could reduce the infarct size. It is worth mentioning that PLAC4, IRAK3, and LRG1 had a potential diagnostic value for both AMI and HF in our study.
In summary, identified lncRNA-co-expressed/nearby targeted mRNAs pairs including AC005392.3/AC007278.2-IL18R1, AL356356.1/AL137145.2-PFKFB3, and MKNK1-AS1/LINC01127-IL1R2 and several signaling pathways (TNF signaling pathway, cytokine–cytokine receptor interaction, fructose and mannose metabolism, HIF-1 signaling pathway, hematopoietic cell lineage, fluid shear stress and atherosclerosis, and estrogen signaling pathway) may be involved in the progression from AMI to MF to HF. Significantly, IL1R2, IRAK3, LRG1, and PLAC4 had a potential diagnostic value for both AMI and HF. Our study may provide a theoretical basis for the diagnosis and mechanism of research for the development process of AMI–MF–HF patients, which can be valuable for patient prognosis.
However, there are limitations to our study. Firstly, the sample size in RNA sequencing and qPCR is small. Large numbers of blood samples are further needed for RNA sequencing and in vitro validation. Secondly, we did not validate the expression of identified lncRNAs and mRNAs and explore deeper pathologic mechanisms of the disease progression. More functional and biological validation experiments, such as immunostaining, ELISA, or Western blot, are further needed. In addition, further mice model experiments are needed to validate and investigate the detailed molecular mechanism of these lncRNAs and mRNAs.
Data Availability Statement
We have deposited the RNA seq data in a public, community-supported repository of GEO (GSE168281).
Ethics Statement
The studies involving human participants were reviewed and approved by Shijiazhuang People's Hospital. The patients/participants provided their written informed consent to participate in this study.
Author Contributions
EW, QC, and YY analyzed the data. LX, XZ, RW, and ZW interpreted the data. SW was a major contributor in writing the manuscript. XH designed the project. All authors read and approved the final manuscript.
Funding
This study was funded by Hebei Medical Science Research Project in 2021 “Related molecular mechanisms between lncRNA/mRNA/miRNA/circRNA and development progression from myocardial fibrosis to myocardial infarction to heart failure based on high flux transcriptome sequencing technology” (20210683).
Conflict of Interest
The authors declare that the research was conducted in the absence of any commercial or financial relationships that could be construed as a potential conflict of interest.
Supplementary Material
The Supplementary Material for this article can be found online at: https://www.frontiersin.org/articles/10.3389/fcvm.2021.664044/full#supplementary-material
Supplementary Figure 1. The in vitro qPCR validation of IL18R1, IL1R2, LRG1, and RNASE1 in additional HF and MF patients. *p < 0.05.
References
1. McMurray JJ, Adamopoulos S, Anker SD, Auricchio A, Böhm M, Dickstein K, et al. [ESC guidelines for the diagnosis and treatment of acute and chronic heart failure 2012]. Turk Kardiyoloji Dernegi arsivi: Turk Kardiyoloji Derneginin Yayin Organidir. (2012) 40:77–137.
2. Laflamme MA, Murry CE. Regenerating the heart. Nat Biotechnol. (2005) 23:845–56. doi: 10.1038/nbt1117
3. Piek A, de Boer RA, Silljé HH. The fibrosis-cell death axis in heart failure. Heart Fail Rev. (2016) 21:199–211. doi: 10.1007/s10741-016-9536-9
4. Mosterd A, Hoes AW. Clinical epidemiology of heart failure. Heart. (2007) 93:1137–46. doi: 10.1136/hrt.2003.025270
5. Levy D, Kenchaiah S, Larson MG, Benjamin EJ, Kupka MJ, Ho KK, et al. Long-term trends in the incidence of and survival with heart failure. N Engl J Med. (2002) 347:1397–402. doi: 10.1056/NEJMoa020265
6. Schonrock N, Harvey RP, Mattick JS. Long noncoding RNAs in cardiac development and pathophysiology. Circ Res. (2012) 111:1349–62. doi: 10.1161/CIRCRESAHA.112.268953
7. Schroeder A, Mueller O, Stocker S, Salowsky R, Leiber M, Gassmann M, et al. The RIN: an RNA integrity number for assigning integrity values to RNA measurements. BMC Mol Biol. (2006) 7:3. doi: 10.1186/1471-2199-7-3
8. Nair SS, Luu PL, Qu W, Maddugoda M, Huschtscha L, Reddel R, et al. Guidelines for whole genome bisulphite sequencing of intact and FFPET DNA on the illumina HiSeq X Ten. Epigenetics Chromatin. (2018) 11:24. doi: 10.1186/s13072-018-0194-0
9. Zhu FY, Chen MX, Ye NH, Qiao WM, Gao B, Law WK, et al. Comparative performance of the BGISEQ-500 and illumina HiSeq4000 sequencing platforms for transcriptome analysis in plants. Plant Methods. (2018) 14:69. doi: 10.1186/s13007-018-0337-0
10. Leek JT, Johnson WE, Parker HS, Jaffe AE, Storey JD. The sva package for removing batch effects and other unwanted variation in high-throughput experiments. Bioinformatics. (2012) 28:882–3. doi: 10.1093/bioinformatics/bts034
11. Kim D, Paggi JM, Park C, Bennett C. Graph-based genome alignment and genotyping with HISAT2 and HISAT-genotype. Nat Biotechnol. (2019) 37:907–15. doi: 10.1038/s41587-019-0201-4
12. Pertea M, Pertea GM, Antonescu CM, Chang TC, Mendell JT, Salzberg SL. StringTie enables improved reconstruction of a transcriptome from RNA-seq reads. Nat Biotechnol. (2015) 33:290–5. doi: 10.1038/nbt.3122
13. Kalvari I, Nawrocki EP, Ontiveros-Palacios N, Argasinska J, Lamkiewicz K, Marz M, et al. Rfam 14: expanded coverage of metagenomic, viral and microRNA families. Nucleic Acids Res. (2021) 49:D192–200. doi: 10.1093/nar/gkaa1047
14. Griffiths-Jones S, Grocock RJ, van Dongen S, Bateman A, Enright AJ. miRBase: microRNA sequences, targets and gene nomenclature. Nucleic Acids Res. (2006) 34:D140–4. doi: 10.1093/nar/gkj112
15. Mackowiak SD. Identification of novel and known miRNAs in deep-sequencing data with miRDeep2. Curr Protoc Bioinformatics. (2011) Chapter 12:Unit 12.10. doi: 10.1002/0471250953.bi1210s36
16. Wang X, Zhu J, Tong J, Wu F, Gao Y, Wang X, et al. [Bioinformatical analysis of differentially expressed genes in alveolar macrophages of chronic obstructive pulmonary disease (COPD)]. Chin J Cell Mol Immunol. (2020) 36:961–6.
17. Gene Ontology Consortium: going forward. Nucleic Acids Res. (2015) 43:D1049–56. doi: 10.1093/nar/gku1179
18. Kanehisa M, Goto S. KEGG: kyoto encyclopedia of genes and genomes. Nucleic Acids Res. (2000) 28:27–30. doi: 10.1093/nar/28.1.27
19. Fei Q, Bai X, Lin J, Meng H, Yang Y, Guo A. Identification of aberrantly expressed long non-coding RNAs in postmenopausal osteoporosis. Int J Mol Med. (2018) 41:3537–50. doi: 10.3892/ijmm.2018.3575
20. Quinlan AR. BEDTools: the Swiss-army tool for genome feature analysis. Curr Protoc Bioinform. (2014) 47:11.12.11–34. doi: 10.1002/0471250953.bi1112s47
21. Shannon P, Markiel A, Ozier O, Baliga NS, Wang JT, Ramage D, et al. Cytoscape: a software environment for integrated models of biomolecular interaction networks. Genome Res. (2003) 13:2498–2504. doi: 10.1101/gr.1239303
22. Hosseini A, Teimuri S, Ehsani M, Rasa SMM, Etemadifar M, Nasr Esfahani MH, et al. LncRNAs associated with multiple sclerosis expressed in the Th1 cell lineage. J Cell Physiol. (2019) 234:22153–62. doi: 10.1002/jcp.28779
23. Thompson SR, Humphries SE. Interleukin-18 genetics and inflammatory disease susceptibility. Genes Immun. (2007) 8:91–9. doi: 10.1038/sj.gene.6364366
24. Bochmann L, Sarathchandra P, Mori F, Lara-Pezzi E, Lazzaro D, Rosenthal N. Revealing new mouse epicardial cell markers through transcriptomics. PLoS ONE. (2010) 5:e11429. doi: 10.1371/journal.pone.0011429
25. Grisoni ML, Proust C, Alanne M, Desuremain M, Salomaa V, Kuulasmaa K, et al. Lack of association between polymorphisms of the IL18R1 and IL18RAP genes and cardiovascular risk: the MORGAM project. BMC Med Genetics. (2009) 10:44. doi: 10.1186/1471-2350-10-44
26. Tiret L, Godefroy T, Lubos E, Nicaud V, Tregouet DA, Barbaux S, et al. Genetic analysis of the interleukin-18 system highlights the role of the interleukin-18 gene in cardiovascular disease. Circulation. (2005) 112:643–650. doi: 10.1161/CIRCULATIONAHA.104.519702
27. Levula M, Oksala N, Airla N, Zeitlin R, Salenius JP, Järvinen O, et al. Genes involved in systemic and arterial bed dependent atherosclerosis–Tampere vascular study. PLoS ONE. (2012) 7:e33787. doi: 10.1371/journal.pone.0033787
28. van der Pouw Kraan TC, Bernink FJ, Yildirim C, Koolwijk P, Baggen JM, Timmers L, et al. Systemic toll-like receptor and interleukin-18 pathway activation in patients with acute ST elevation myocardial infarction. J Mol Cell Cardiol. (2014) 67:94–102. doi: 10.1016/j.yjmcc.2013.12.021
29. Vanhaverbeke M, Vausort M, Veltman D, Zhang L, Wu M, Laenen G, et al. Peripheral blood RNA levels of QSOX1 and PLBD1 are new independent predictors of left ventricular dysfunction after acute myocardial infarction. Circ Genom Precision Med. (2019) 12:e002656. doi: 10.1161/CIRCGEN.119.002656
30. Yang K, Lu XF, Luo PC. Identification of six potentially long noncoding RNAs as biomarkers involved competitive endogenous RNA in clear cell renal cell carcinoma. Biomed Res Int. (2018) 2018:9303486. doi: 10.1155/2018/9303486
31. Hong Y, Kim WJ, Bang CY, Lee JC, Oh YM. Identification of alternative splicing and fusion transcripts in non-Small cell lung cancer by RNA sequencing. Tuberc Respir Dis. (2016) 79:85–90. doi: 10.4046/trd.2016.79.2.85
32. De Bock K, Georgiadou M, Schoors S, Kuchnio A, Wong BW, Cantelmo AR, et al. Role of PFKFB3-driven glycolysis in vessel sprouting. Cell. (2013) 154:651–63. doi: 10.1016/j.cell.2013.06.037
33. De Bock K, Georgiadou M, Carmeliet P. Role of endothelial cell metabolism in vessel sprouting. Cell Metab. (2013) 18:634–47. doi: 10.1016/j.cmet.2013.08.001
34. Teuwen LA, Draoui N, Dubois C, Carmeliet P. Endothelial cell metabolism: an update anno 2017. Curr Opin Hematol. (2017) 24:240–7. doi: 10.1097/MOH.0000000000000335
35. Schoors S, De Bock K, Cantelmo AR, Georgiadou M, Ghesquière B, Cauwenberghs S, et al. Partial and transient reduction of glycolysis by PFKFB3 blockade reduces pathological angiogenesis. Cell Metab. (2014) 19:37–48. doi: 10.1016/j.cmet.2013.11.008
36. Tawakol A, Singh P, Mojena M, Pimentel-Santillana M, Emami H, MacNabb M, et al. HIF-1α and PFKFB3 mediate a tight relationship between proinflammatory activation and anerobic metabolism in atherosclerotic macrophages. Arterioscler Thromb Vasc Biol. (2015) 35:1463–71. doi: 10.1161/ATVBAHA.115.305551
37. Bekkering S, van den Munckhof I, Nielen T, Lamfers E, Dinarello C, Rutten J, et al. Innate immune cell activation and epigenetic remodeling in symptomatic and asymptomatic atherosclerosis in humans in vivo. Atherosclerosis. (2016) 254:228–36. doi: 10.1016/j.atherosclerosis.2016.10.019
38. Qiu L, Liu X. Identification of key genes involved in myocardial infarction. Eur J Med Res. (2019) 24:22. doi: 10.1186/s40001-019-0381-x
39. Lai CF, Chen YT, Gu J, Nerbonne JM, Lin CH, Yang KC. Circulating long noncoding RNA DKFZP434I0714 predicts adverse cardiovascular outcomes in patients with end-stage renal disease. Int J Cardiol. (2019) 277:212–19. doi: 10.1016/j.ijcard.2018.08.013
40. Jing L, Gong M, Lu X, Jiang Y, Li H, Cheng W. LINC01127 promotes the development of ovarian tumors by regulating the cell cycle. Am J Transl Res. (2019) 11:406–17.
41. Tian ZH, Yuan C, Yang K, Gao XL. Systematic identification of key genes and pathways in clear cell renal cell carcinoma on bioinformatics analysis. Ann Transl Med. (2019) 7:89. doi: 10.21037/atm.2019.01.18
42. He F, Teng X, Gu H, Liu H, Zhou Z, Zhao Y, et al. Interleukin-6 receptor rs7529229 t/C polymorphism is associated with left main coronary artery disease phenotype in a Chinese population. Int J Mol Sci. (2014) 15:5623–33. doi: 10.3390/ijms15045623
43. McGeachie M, Ramoni RL, Mychaleckyj JC, Furie KL, Dreyfuss JM, Liu Y, et al. Integrative predictive model of coronary artery calcification in atherosclerosis. Circulation. (2009) 120:2448–54. doi: 10.1161/CIRCULATIONAHA.109.865501
44. Chen YC, Chen TW, Su MC, Chen CJ, Chen KD, Liou CW, et al. Whole genome DNA methylation analysis of obstructive sleep apnea: IL1R2, NPR2, AR, SP140 methylation and clinical phenotype. Sleep. (2016) 39:743–55. doi: 10.5665/sleep.5620
45. Chen X, Chen X, Xu Y, Yang W, Wu N, Ye H, et al. Association of six CpG-SNPs in the inflammation-related genes with coronary heart disease. Hum Genom. (2016) 10:21. doi: 10.1186/s40246-016-0067-1
46. Kobayashi S, Ito A, Okuzaki D, Onda H, Yabuta N, Nagamori I, et al. Expression profiling of PBMC-based diagnostic gene markers isolated from vasculitis patients. DNA Res. (2008) 15:253–65. doi: 10.1093/dnares/dsn014
47. Chen DQ, Kong XS. Identification of differentially expressed genes and signaling pathways in acute myocardial infarction based on integrated bioinformatics analysis. Cardiovasc Ther. (2019) 2019:8490707. doi: 10.1155/2019/8490707
48. Xiao J, Moon M, Yan L, Nian M, Zhang Y, Liu C, et al. Cellular FLICE-inhibitory protein protects against cardiac remodelling after myocardial infarction. Basic Res Cardiol. (2012) 107:239. doi: 10.1007/s00395-011-0239-z
49. Long J, Liu M, Liu S, Tang F, Tan W, Xiao T, et al. H2S attenuates the myocardial fibrosis in diabetic rats through modulating PKC-ERK1/2MAPK signaling pathway. Technol Health Care. (2019) 27:307–316. doi: 10.3233/THC-199029
50. Chung ES, Packer M, Lo KH, Fasanmade AA, Willerson JT. Randomized, double-blind, placebo-controlled, pilot trial of infliximab, a chimeric monoclonal antibody to tumor necrosis factor-alpha, in patients with moderate-to-severe heart failure: results of the anti-TNF therapy against congestive heart failure (ATTACH) trial. Circulation. (2003) 107:3133–40. doi: 10.1161/01.CIR.0000077913.60364.D2
51. Mann DL, McMurray JJ, Packer M, Swedberg K, Borer JS, Colucci WS, et al. Targeted anticytokine therapy in patients with chronic heart failure: results of the randomized etanercept worldwide evaluation (RENEWAL). Circulation. (2004) 109:1594–602. doi: 10.1161/01.CIR.0000124490.27666.B2
52. Sala V, Gatti S, Gallo S, Medico E, Cantarella D, Cimino J, et al. A new transgenic mouse model of heart failure and cardiac cachexia raised by sustained activation of met tyrosine kinase in the heart. BioMed Res Int. (2016) 2016:9549036. doi: 10.1155/2016/9549036
53. Bai H, Sun K, Wu JH, Zhong ZH, Xu SL, Zhang HR, et al. Proteomic and metabolomic characterization of cardiac tissue in acute myocardial ischemia injury rats. PLoS ONE. (2020) 15:e0231797. doi: 10.1371/journal.pone.0231797
54. Parisi Q, Biondi-Zoccai GG, Abbate A, Santini D, Vasaturo F, Scarpa S, et al. Hypoxia inducible factor-1 expression mediates myocardial response to ischemia late after acute myocardial infarction. Int J Cardiol. (2005) 99:337–9. doi: 10.1016/j.ijcard.2003.11.038
55. Wei H, Bedja D, Koitabashi N, Xing D, Chen J, Fox-Talbot K, et al. Endothelial expression of hypoxia-inducible factor 1 protects the murine heart and aorta from pressure overload by suppression of TGF-β signaling. Proc Natl Acad Sci USA. (2012) 109:E841–50. doi: 10.1073/pnas.1202081109
56. Sondergaard CS, Hess DA, Maxwell DJ, Weinheimer C, Rosová I, Creer MH, et al. Human cord blood progenitors with high aldehyde dehydrogenase activity improve vascular density in a model of acute myocardial infarction. J Transl Med. (2010) 8:24. doi: 10.1186/1479-5876-8-24
57. Fu X, Liu Q, Li C, Li Y, Wang L. Cardiac fibrosis and cardiac fibroblast lineage-tracing: recent advances. Front Physiol. (2020) 11:416. doi: 10.3389/fphys.2020.00416
58. Westenbrink BD, Voors AA, de Boer RA, Schuringa JJ, Klinkenberg T, van der Harst P, et al. Bone marrow dysfunction in chronic heart failure patients. Eur J Heart Failure. (2010) 12:676–84. doi: 10.1093/eurjhf/hfq061
59. Farmakis TM, Soulis JV, Giannoglou GD, Zioupos GJ, Louridas GE. Wall shear stress gradient topography in the normal left coronary arterial tree: possible implications for atherogenesis. Curr Med Res Opin. (2004) 20:587–96. doi: 10.1185/030079904125003340
60. Eitenmüller I, Volger O, Kluge A, Troidl K, Barancik M, Cai WJ, et al. The range of adaptation by collateral vessels after femoral artery occlusion. Circ Res. (2006) 99:656–62. doi: 10.1161/01.RES.0000242560.77512.dd
61. Mythili S, Malathi N. Diagnostic markers of acute myocardial infarction (review). Biomed Rep. (2015) 3:743–8. doi: 10.3892/br.2015.500
62. Cannon MV, van Gilst WH, de Boer RA. Emerging role of liver X receptors in cardiac pathophysiology and heart failure. Basic Res Cardiol. (2016) 111:3. doi: 10.1007/s00395-015-0520-7
63. Pelleri MC, Gennari E, Locatelli C, Piovesan A, Caracausi M, Antonaros F, et al. Genotype-phenotype correlation for congenital heart disease in down syndrome through analysis of partial trisomy 21 cases. Genomics. (2017) 109:391–400. doi: 10.1016/j.ygeno.2017.06.004
64. Xu J, Li Z, Ren X, Dong M, Li J, Shi X, et al. Investigation of pathogenic genes in Chinese sporadic hypertrophic cardiomyopathy patients by whole exome sequencing. Sci Rep. (2015) 5:16609. doi: 10.1038/srep16609
65. Wang Y, Huang Y, Zhang M, Zhang X, Tang X, Kang Y. Bioinformatic analysis of the possible regulative network of miR-30a/e in cardiomyocytes 2 days post myocardial infarction. Acta Cardiologica Sinica. (2018) 34:175–88. doi: 10.6515/ACS.201803_34(2).20170926A
66. Kumagai S, Nakayama H, Fujimoto M, Honda H, Serada S, Ishibashi-Ueda H, et al. Myeloid cell-derived lRG attenuates adverse cardiac remodelling after myocardial infarction. Cardiovasc Res. (2016) 109:272–82. doi: 10.1093/cvr/cvv273
67. Yang FJ, Hsieh CY, Shu KH, Chen IY, Pan SY, Chuang YF, et al. Plasma leucine-Rich α-2-glycoprotein 1 predicts cardiovascular disease risk in end-stage renal disease. Sci Rep. (2020) 10:5988. doi: 10.1038/s41598-020-62989-7
68. Szauter KM, Jansen MK, Okimoto G, Loomis M, Kimura JH, Heller M, et al. Persistent inflammatory pathways associated with early onset myocardial infarction in a medicated multiethnic hawaiian cohort. Biochem Insights. (2011) 2011:13–27. doi: 10.4137/BCI.S6976
69. Maciejak A, Kiliszek M, Michalak M, Tulacz D, Opolski G, Matlak K, et al. Gene expression profiling reveals potential prognostic biomarkers associated with the progression of heart failure. Genome Med. (2015) 7:26. doi: 10.1186/s13073-015-0149-z
70. Wu K, Zhao Q, Li Z, Li N, Xiao Q, Li X, et al. Bioinformatic screening for key miRNAs and genes associated with myocardial infarction. FEBS Open Bio. (2018) 8:897–913. doi: 10.1002/2211-5463.12423
71. Eicher JD, Wakabayashi Y, Vitseva O, Esa N, Yang Y, Zhu J, et al. Characterization of the platelet transcriptome by RNA sequencing in patients with acute myocardial infarction. Platelets. (2016) 27:230–9. doi: 10.3109/09537104.2015.1083543
72. Muehlschlegel JD, Christodoulou DC, McKean D, Gorham J, Mazaika E, Heydarpour M, et al. Using next-generation RNA sequencing to examine ischemic changes induced by cold blood cardioplegia on the human left ventricular myocardium transcriptome. Anesthesiology. (2015) 122:537–50. doi: 10.1097/ALN.0000000000000582
73. Du M, Shan J, Feng A, Schmull S, Gu J, Xue S. Oestrogen receptor β activation protects against myocardial infarction via notch1 signalling. (2020) 34:165–78. doi: 10.1007/s10557-020-06949-3
Keywords: acute myocardial infarction, myocardial fibrosis, heart failure, RNA sequencing, lncRNAs, mRNAs, signaling pathways, diagnosis
Citation: Wang S, Wang E, Chen Q, Yang Y, Xu L, Zhang X, Wu R, Hu X and Wu Z (2021) Uncovering Potential lncRNAs and mRNAs in the Progression From Acute Myocardial Infarction to Myocardial Fibrosis to Heart Failure. Front. Cardiovasc. Med. 8:664044. doi: 10.3389/fcvm.2021.664044
Received: 18 March 2021; Accepted: 15 June 2021;
Published: 16 July 2021.
Edited by:
Christoph Dieterich, Heidelberg University, GermanyReviewed by:
Cheng-Kai Huang, Hannover Medical School, GermanyTobias Jakobi, University of Arizona, United States
Copyright © 2021 Wang, Wang, Chen, Yang, Xu, Zhang, Wu, Hu and Wu. This is an open-access article distributed under the terms of the Creative Commons Attribution License (CC BY). The use, distribution or reproduction in other forums is permitted, provided the original author(s) and the copyright owner(s) are credited and that the original publication in this journal is cited, in accordance with accepted academic practice. No use, distribution or reproduction is permitted which does not comply with these terms.
*Correspondence: Xitian Hu, aHV4aXRpYW4wMDcmI3gwMDA0MDsxNjMuY29t