- 1UCD Diabetes Complications Research Centre, Conway Institute of Biomolecular and Biomedical Research, University College Dublin, Dublin, Ireland
- 2Nutrigenomics Research Group, UCD Institute of Food and Health, School of Public Health Physiotherapy and Sports Science, University College Dublin, Dublin, Ireland
- 3Centre of Excellence for Public Health, Queen's University Belfast, Belfast, United Kingdom
- 4UCD Institute of Food and Health, School of Public Health Physiotherapy and Sports Science, University College Dublin, Dublin, Ireland
- 5School of Medicine, University College Dublin, Dublin, Ireland
Finding genetic variants that cause functional disruption or regulatory change among the many implicated GWAs variants remains a key challenge to translating the findings from GWAs to therapeutic treatments. Defining the causal mechanisms behind the variants require functional screening experiments that can be complex and costly. Prioritizing variants for functional characterization using techniques that capture important functional and regulatory elements can assist this. The genetic architecture of complex traits such as cardiovascular disease and type II diabetes comprise an enormously large number of variants of small effect contributing to heritability and spread throughout the genome. This makes it difficult to distinguish which variants or core genes are most relevant for prioritization and how they contribute to the regulatory networks that become dysregulated leading to disease. Despite these challenges, recent GWAs for CAD prioritized genes associated with lipid metabolism, coagulation and adhesion along with novel signals related to innate immunity, adipose tissue and, vascular function as important core drivers of risk. We focus on three examples of novel signals associated with CAD which affect risk through missense or UTR mutations indicating their potential for therapeutic modification. These variants play roles in adipose tissue function vascular function and innate immunity which form the cornerstones of immuno-metabolism. In addition we have explored the putative, but potentially important interactions between the environment, specifically food and nutrition, with respect to key processes.
Introduction
CVD is increasing and the distribution of risk factors is changing with increasing prevalence of obesity and type II diabetes (T2D), particularly among young adults (aged 18–45) in developed countries (1–3). The burden of CVD risk factors remains very high because of unhealthy contemporary lifestyles, with dysregulated balance between energy intake and physical activity. In addition, malnutrition, wherein excess energy is coupled by micronutrient deficiencies, amplifies genetic risk (4). The major cardiovascular consequences of obesity and T2D predominantly derive from dysregulated and inflamed adipose tissue, particularly perivascular or visceral fat surrounding the organs (5). Visceral fat has limited expandability and becomes inflamed, with the resulting adipokine dysregulation adversely affecting vascular biology by promoting vasoconstriction, medial smooth muscle cell proliferation and endothelial dysfunction and is known as dysregulated immunometabolism (6). In the obese state, immune cells become activated and infiltrate metabolic tissues, chronic activation of inflammatory pathways in both vascular and immune components trigger stress kinase activation that impinge on the signaling of metabolic hormones such as insulin leading to impaired glucose and lipid homeostasis (7). Highly structured interactions between immune and metabolic responses are evolutionarily conserved and disruption of these interactions underlie many pathologies such as obesity and diabetes. Therapeutic solutions to tackle obesity, T2D and hypertension are drastically needed to reduce the overall burden of cardiovascular disease. However, many drugs or interventions have failed due to a lack of understanding of complex disease architecture (8, 9).
GWAs provided unique insights into the genetic architecture of complex diseases. Genetic architecture considers the overall composition of variants influencing a trait in terms of number, frequency and magnitude of effect and potential interactions, and can vary over traits (10). With increasing size and scope of GWAs, it has become clear that many complex traits are driven by enormously large numbers of variants of small effects. These variants are spread across the genome rather than in disease related pathways, include many without obvious connection to disease and/or related risk factors. These variants are potentially capturing most regulatory variants that are active in disease relevant tissues and the regulatory networks they form, may be so interconnected they affect the functions of core disease-related genes. This can be observed for variants that are heavily concentrated in regions that are transcribed or marked by active chromatin in disease-relevant tissues but with little enrichment for cell-type specific regulatory elements compared to broadly active regions. Boyle et al. (11) proposed that this pattern could be explained through an Omnigenic model of inheritance. This is an extension of RA Fischer's infinitesimal model of inheritance proposed nearly a century ago (12). The Omnigenic model considers that gene regulatory networks are so interconnected that all genes expressed in disease relevant cells are able to affect the functions of core disease-related genes. Most heritability is accounted for by effects of genes in peripheral pathways, outside of core pathways, which accounts for loci associated with multiple traits (pleiotrophy). Therefore, disease risk may be largely driven by genes with no direct relevance to disease and is propagated through regulatory networks to a much smaller number of core genes with direct effects.
In theory, the set of core genes must have a more pronounced effect on disease traits and proteins derived from these genes will drive pharmaceutical development and therapeutic strategies. However, how this works at the cellular regulatory network is incompletely understood. To understand the relevance of the variants for therapeutic development, it is crucial to understand their effect on protein level, activity or function. Even if a variant has a small effect on protein level and disease risk this protein may still be a suitable target for disease prevention if considered in context with its disease architecture (10). For instance, the SNP associated with (HMG-CoA reductase) explains 0.26% of variance in LDL levels, manipulating this gene can reduce LDL levels by 30–40% and reduce CAD risk (13). Even if the variants affect protein level or function, there are numerous challenges to drug development.
An Omnigenic Architecture For Cardiovascular Disease?
GWAs has reproducibly associated over 160 variants with cardiovascular disease (14–20). By combining data from UK Biobank (34,541 cases and 261,984 non-cases) followed by replication in CardiogramplusC4D (88,192 cases and 162,544 controls), an additional 64 novel loci were recently prioritized (20). This identified a total of 163 loci associated with coronary artery disease (CAD) (21). Consistent with the omnigenic model for CAD genetic architecture, many novel candidate genes did not have an obvious connection to CAD and the genetic contribution was concentrated in regions transcribed or marked by active chromatin in relevant tissues (blood vessels and liver) but with little enrichment for cell-type specific regulatory elements. While they reconstituted a larger number of gene pathways/networks for CAD, increasing from 4 to 14%, overall the variants were spread throughout the genome and only 14% forming disease relevant pathways.
Prioritizing Variants Using Information From Functional and Regulatory Regions
To prioritize core CAD-related genes, they fine mapped the regions characterizing the functional, cellular and regulatory contribution of the variants (22) and prioritized their significance using probabilistic models (23) to derive a set of genes with converging evidence of potential functional SNP-gene mechanisms for functional follow up studies. The fine mapping methods that they used are compared against related methods summarized in Table 1, reviewed in Schaid et al. (30). Integrating information from multiple omics approaches in this way provides a more comprehensive understanding of the flow of information from the disease driver to its functional consequence or interactions. Methods can now test the mediating mechanism of these genetic variants on complex traits (31). Their analysis prioritized 161 variants to candidate genes based on proximity, expression quantitative loci data, DEPICT analysis and long-range chromatin interactions of variants with gene promotors for signals of regulation using stringent conditions and identified 28 loci with convincing arguments for causal variation, 22 known and 6 novel or 19 potential core genes (with missense, intergenic, downstream or UTR mutations). Among known genes; APOE, PCSK9, ANGPTL4 and SORT1 are implicated as core genes in lipid metabolism (a key component of immunometabolism) and targeting the effects of these genes can reduce CAD risk (32–34). Of the 6 novel signals, 3 are intergenic while 3 affect change through missense mutation or occur in a UTR3 region; these are TRIM5, FNDC3B, and CCM2 which are implicated in innate immunity, adipogenesis and vascular function, respectively, and all require functional follow up (Figure 1). Their study aimed to prioritize the CAD associations and elucidate regulatory connections that may influence the mechanism behind the associations, but according to the omnigenic model, broader regulatory connections between core genes must exist but are difficult to elucidate.
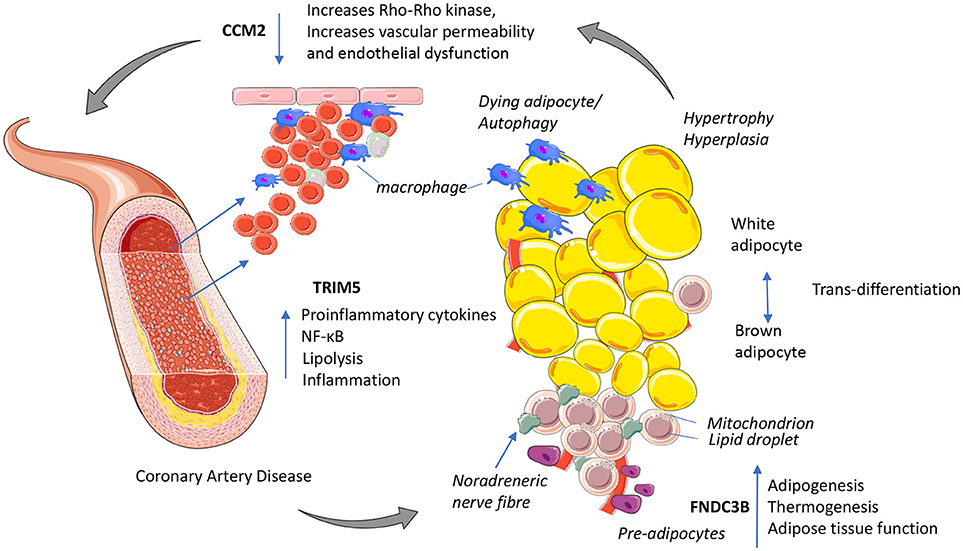
Figure 1. Putative mechanisms for three novel GWAs signals with functional links to immuno-metabolism and coronary artery disease. TRIM5 released from activated macrophages could increase proinflammatory cytokines NF-κB and shifts cellular energy from oxidative phosphorylation to lipolysis. CCM2 maintains endothelial function, decreased CCM2 increases Rho_Rho kinase activity increasing vascular permeability increasing inflammation. FNDC3B potentially enhances adipose tissue function by increasing adipogenesis and improving cellular energy efficiency by promoting oxidative phosphorylation and thermogenesis. This figure was prepared using the Servier medical art website (www.servier.fr).
Several major challenges stand in the way to understanding how GWAs associations could become therapeutic targets. Most GWAs associations lie within non-coding regions making it difficult to predict their functions and identify targets/genes. Loci can be linked to multiple genes and the likely causal variant requires detailed investigation to elucidate the underlying mechanism. Functional follow up of important GWAs candidate loci now shows that multiple variants of small effect can synergistically drive dysfunction in regulatory networks, for example risk related to FTO (35), ANGPTL4 (17), GUCY1A3 (36), and SHROOM3 (37). To understand the mechanistic basis of increased adiposity associated with FTO, layers of OMICS data connecting epigenetic, gene co-expression and regulator expression followed by validation with genome editing elucidated the risk variant rs1421085 causes a loss of repression in AR1D5B which enhances expression of IRX3 and IRX5 increasing fat storage (35). Mining available OMIC data to gain insights into the complex regulatory circuitry behind these association signals has the potential to speed up functional follow-up by identifying novel links. We consider the three novel signals highlighted by van der Harst and Verweij for their strength of evidence and their importance to these pathways contributing to CAD risk or related traits such as adiposity and how these signals fit with other evidence supporting their contribution to disease risk. These may represent core genes but they may be signals that are context or cell specific to CAD. We also consider what the cell or tissue derived signals could offer therapeutically if they validated in independent studies. To this end, we explore a few examples wherein this paradigm may be relevant.
Trim5, Innate Immune Signaling and Cad Risk
The variant rs11601507 causes a missense mutation in TRIM5 and increases CAD risk (p = 2.1 × 10−12, OR 1.09 (95% C.I. 1.06, 1.11). Chromatin interactions between this variant and eQTLs in the promotors/enhancers of three other genes (TRIM6, OR52S1, OR52B6) suggest these genes enhance the expression of TRIM5. Chromatin interactions reveal relationships of chromatin organization in 3D space that may indicate biological function such as promotor-enhancer interactions. The evidence used to support rs11601507 is from a range of Hi-C experimental cell lines (20) (38). rs11601507 is a cis QTL for HBG2 (Hemoglobin) in whole blood (39) and shows significant tissue specific enrichment in veins and blood vessels [DEPICT analysis, (20)]. Ingenuity® pathway analysis (IPA®) prioritized TRIM5 and TRIM6 along with 14 other genes for association with CVD. IPA® considers upstream and downstream regulators of gene expression based on large scale causal networks (40). Interestingly, this same missense variant rs11601507 and a 5′UTR variant rs3824949 in TRIM5 has previously been associated with mean platelet volume (p = 6 × 10−19 and p = 1 × 10−24, respectively) (41) which is an example of pleiotrophy.
Given the enormous dimensionality of the phenome, it is unlikely that functional variants exist without pleiotrophic effects (42). Pleiotrophy can involve variants having effects on two or more traits via independent pathways (e.g., effects in different tissues) or effect of the variant in one trait causally related to variation in another trait. The risk allele of this variant has the same direction of effect for CAD and mean platelet volume. Using rs11601507 and other variants in a risk score, Astle et al. demonstrated a weak causal relationship between mean platelet volume and CAD risk using Mendelian randomization. Mean platelet volume is associated with increased hemolysis or free hemoglobin in the blood which is linked to increased inflammation. The TRIM5 association may be affecting both traits through inflammatory pathways.
TRIM5 promotes Interferon γ (IFNG) in macrophages, this forms part of the innate immune system (43). It has a capsid specific restriction factor that prevents infection from non-host adapted retroviruses. Interestingly, TRIM5 reciprocally enhances ubiquitination leading to co-operative action of IFNG and NF-κB pathways (44). There is a dynamic relationship between the innate immune system and metabolism, where re-configuration of energy metabolism between oxidative phosphorylation vs. glycolysis can define the immune-phenotype (45, 46). Fatty acids and other metabolites can influence and define immune cell functionality and cause metabolic reprogramming (45). It is hypothesized that this dynamic and reciprocal regulatory relationship between metabolism and inflammation plays a key role in metabolic disease including CAD (7). Macrophages play a key role as innate immune cellular mediators of inflammation. Activated macrophages can recruit other monocytes/macrophages to a developing lesion and increase lipid uptake and instigate metabolic stress and reprogramming in adipose tissue. Macrophages can become “metabolically activated” in the presence of glucose, insulin and palmitate. Metabolically activated macrophages demonstrate similar effects to classically activated macrophages, where both types activate the TLR4 and NF-κB pathways to promote pro-inflammatory cytokine secretion. However, the metabolically active macrophages also activate PPARγ, therefore controlling inflammation by prompting lipid metabolism (47). TRIM5 promotes IFNG and through a mechanism of decreasing tryptophan metabolism (which viruses rely on), IFNG inhibits the central metabolic regulator mTOR and metabolically reprograms macrophages to switch from glycolysis to oxidative phosphorylation and upregulates inflammation. CVD is associated with changes in many immune cell types at multiple sites of critical metabolic function with a cumulative detrimental effect on cholesterol, lipid and glucose homeostasis (7, 22).
Olfactory Signaling Influences Trim5 and is also Linked To Adiposity
The genetic mechanism associated with the TRIM5 variant suggests enhanced olfactory signaling enhances TRIM5 (innate immune signaling) to reduce lipolysis which enhances adiposity and increases risk of CAD. OR52B6, and OR52S1 are G-protein coupled olfactory signaling receptors (ORs) (48). These receptors interact with odorant molecules in the nose, to initiate a neuronal response that triggers the perception of a smell. OR52B6/OR52S1 have not been linked by GWAs signals as important regulatory variants but other variants related to olfaction have been have been significantly linked to obesity development through GWAS (8). Olfactory signaling is highly complex and can play a bidirectional role in controlling energy homeostasis in response to sensory and hormonal signals from the central nervous system (CNS) (49). Essentially the ORs may alter the drive to eat a poor diet, leading to obesity, hence elaborating an environmental insult. Reduced olfactory signaling increases β-adrenergic receptors on white (WAT) and brown adipose tissue (BAT) increasing lipolysis and fatty acid oxidation reducing obesity in mice (49). Olfaction influences the loss of function mutation in ADCY3 (50) gene and its interaction with the major obesity gene MC4R which disrupts ciliary targeting in neuronal cells critical for body weight regulation (35, 51). Heterozygous or homozygous null mice for ADCY3 are unable to smell (35).
In summary, innate immunity is important in the pathogenesis of CVD, here the association between a variant linked to innate immunity is reinforced and mediated through a novel mechanism of olfaction. The immunosuppressant drug cyclosporine is an antagonist for TRIM5 suggesting a potential therapeutic intervention is available to explore for functional relevance (20). More generally, targeting systemic inflammation through interleukin 1β (e.g., Canakinumab) has been shown to reduce CVD risk and by doing so has validated the inflammatory hypothesis of atherothrombosis (52). The variants TRIM5 and PROCR (p = 6.8 × 10−12) reaching GWAs significance are related to inflammation, which are relatively newly identified, show convergence between biological and genetic determinants of CVD and add to this inflammatory hypothesis (18, 20). An alternative therapeutic paradigm to anti-inflammatory modalities may be efforts to mimic the resolution of inflammation using specialized lipid mediators and their targets (53–55).
FNDC3b, Adipogenesis and Cad Risk
rs12897 is a common variant (MAF 0.41) showing a protective association with CAD; OR 0.96 (95% C.I. 0.95, 0.97) (p = 1.9 × 10−10), this SNP is an eQTL for the protein coding gene Fibronectin type III domain containing 3B (FNDC3B) (39) occurring in the 3′ UTR region of the mRNA likely affecting post-transcriptional regulation of gene expression. This SNP was the 3rd top gene prioritized by DEPICT (p = 1 × 10−21) in the overall analysis (20). IPA® prioritized a functional association between the protein of FNDC3B, TRIM5, TRIM6, VEGFA, and 12 other genes for association with CAD supporting a broader connectivity among these.
Adipogenesis is a key regulatory process, which determines adipose functionality, and its dysfunction is associated with metabolic-inflammation, hypoxia and related risks including insulin resistance (6) and deregulated cholesterol homeostasis and lipid metabolism (56) all of which lead to greater T2D and CVD risk (57). FNDC3B (alias FAD104) is a positive regulator of adipogenesis (58); specifically at the early stages of adipogenesis (59, 60). FNDC3B variant rs12897 was previously associated with large scale GWAs on height (p = 3 × 10−39) (61), waist-to-hip ratio (WHR) adjusted BMI (p = 8 × 10−10) and HIP adjusted BMI (p = 3 × 10−12) (62) and heart rate (p = 1 × 10−9) (63). Interestingly, intronic variants near FNDC3B strongly associated with intra-ocular pressure p = 9 × 10−48 (64) and p = 5 × 10−50 (65), however these variants are not in LD with rs12897. Although intraocular pressure may reflect changes in heart rate, this association may operate through a different, peripheral CAD pathway.
GWAs on specific adiposity traits and fat distributions (pericardial fat, visceral fat, WHRadjBMI, body fat percentage) have shown distinct genetic components (66, 67). WHR adjusted BMI and body fat percentage traits identified adipogenesis candidate genes to play key roles in adiposity. These genes included BMP2 (p = 3.3 × 10−14), CEBPA, PPARγ, HOXC-mir196, TBX15, and PEMT but these variants had no apparent regulatory links/eQTLs (62). While CEBPA and PPARγ are essential for white adipose tissue differentiation and are master regulators of adipogenesis, BMP2, like FNDC3B, is involved in early stage adipogenesis. FNDC3B and BMP2 are both involved in the early stage commitment of pre-adipocytes to proliferate and differentiate. FNDC3B (and BMP2) specifically induce and/or regulate the differentiation of committed progenitor cells toward adipogenesis or osteogenesis (68). Adipocytes and in particular pre-adipocytes are now recognized as more than fat-storing organelles having the capability to secrete cytokines and adipokines thus contributing to inflammation (69).
Adipogenesis as a Therapeutic Mechanism to Reduce Metabolic Risk
Defining the effectors which control the fate of adipocytes is of great interest to the therapeutic treatment of obesity. Obese individuals have a smaller proportion of brown adipose tissue (BAT) compared to white adipose tissue (WAT) which expands in response to lipid excess by hypertrophy, hyperplasia and inflammation and upon reaching a certain size become dysfunctional and necrotic, promoting macrophage infiltration. The conversion of WAT to the more functional energy dispersing BAT adipocytes would be a valuable approach to the treatment of obesity and its metabolic complications and is becoming the focus of anti-obesity research (9). Conversion of WAT to BAT can occur by two processes; adipogenesis (i.e., de-novo-adipocyte differentiation of precursor cells which FNDC3B may play a role) or more commonly trans-differentiation (i.e., WAT to beige/brite transition through molecular reprogramming, increasing mitochondrial oxidative phosphorylation/lipolysis requiring increased levels of uncoupling protein 1 (UCP-1) and enervated with b-adrenoreceptors (9). BAT derived from adipogenesis is more sensitive to stimuli from BMP7 (70) and BMP4 (71), irisin/FNDC5B (72), FGF21, and others. Irisin/FNDC5B is a myokine/cytokine that induces thermogenesis except in the obese state where it has a complex adaptive response to counterbalance decreased insulin sensitivity and other metabolic disorders associated with obesity (73) and is a key molecular target to induce browning of WAT (9). FNCD5B expression is highest at early stages of preadipocyte differentiation sharing 53% homology with FNDC3B but their relationship is unclear. While the eQTL affects the expression of FNDC3B, it is not known if this regulation is specific to a CVD relevant tissue or cell type. If regulation of FNDC3B is a key step in increasing adipogenesis, modulation of this process could enhance thermogenesis.
The strongest obesity variant associated to date, FTO, can act through a complex regulatory network also affecting pre-adipocyte differentiation highlighting the importance of this pathway (35). Interestingly, this regulation ensures it is restricted in a cell/tissue specific way to preadipocytes and mesenchymal adipocyte progenitors, not in brain or 120 other cell types (35). The causal variant associated with FTO disrupts AR1D5B binding in the risk haplotype leading to a loss of repression, this derepresses pre-adipocyte enhancer activity and increases IRX3 and IRX5 expression which represses mitochondrial thermogenesis and adipocyte browning making cells more likely to store fat.
Other Variants of Genes Influencing Transdifferentiation of Wat to Bat Are Also Linked To Cad Risk
In addition to FNDC3B, two other variants PRDM16 and TWIST1 recently associated with CAD risk play key roles in adipogenic transdifferentiation of WAT to BAT which highlights this pathway as relevant to disease risk and therapeutic exploration. From a biological perspective PRDM16 is one of the most effective molecular targets to induce white-to-brown adipocyte trans-differentiation (9) and an intronic variant rs2493298 close to PRDM16 was recently identified to increase CAD risk (20). The variant rs2493298 p = 1.9 × 10−9, near PRDM16 occurs in an intronic region which physically interacts (chromatin, Hi-C experiments) with the promotors of three genes that act as enhancers (20) which have roles in metabolism (9). PRDM16 is essential for normal BAT function, it interacts with C/EBPβ and these are considered master regulators of BAT function (74), and functions in a feedback loop with PPARy and SIRT1. No pharmacological targets for PRDM16 are advanced enough to explore in clinical trials (9). PPARy coactivator 1 alpha (PGC1a) is another important control point of the BAT phenotype and it is repressed by TWIST1 which blocks target genes associated with PGC1a activity leading to browning of WAT (75). An eQTL rs21079595 intergenic to TWIST1 increases risk of CAD 1.3 × 10−24 and was prioritized as a core CAD related gene (20). Previously this variant had been linked to HDAC9 gene through proximity, but expression data from the Stockholm-Tartu Atherosclerosis Reverse Network Engineering Task Study (STARNET) in two different tissues prioritized this eQTL variant to TWIST1 (76). Manipulation of PGC1a can also achieve reductions in inflammatory disease risk and enhance adipogenesis through dietary fat modification (6).
In summary, several variants associated with adipogenesis have been associated with CAD risk. Dysregulation of adipocyte browning/thermogenesis, particularly in visceral fat surrounding thoracic and aortic arch, is important in the pathogenesis of CVD. FNDC3B is among several variants that impact adipogenesis, while the regulatory networks among these still need more complete understanding, manipulation of this pathway at the preadipocyte stage could impact CVD risk.
CCM2, Endothelial Function and CVD Risk
The variant rs2107732 causes a missense mutation in the CCM2 gene and is associated with reduced risk of CAD (OR 0.94 (95% C.I. 0.93–0.96), p = 3.6 × 10−8 (20). A variant in the promotor of MYOG1 forms a chromatin interaction with the CCM2 variant suggesting this regulates CCM2 (20). MYOG1 is a muscle specific transcription factor that induces myogenesis (muscle formation). Mutations in an orthologous mouse gene of CCM2 cause a cardiovascular phenotype in mice and mutations in MYOG1 caused abnormalities in inflammation/white blood cells of mice (Mouse Genome Informatics database) (20). Inherited loss-of-function mutations in CCM2 (and also CCM1 and CCM3 genes) are implicated in abnormal vascular morphogenesis and can cause vascular lesions called cerebral cavernous malformations which develop in the human CNS (77). CCM2 is expressed in the brain and heart and CCM genes (including CCM2, CCM1, CCM3) are crucial regulators of heart and vessel formation and integrity by restricting vascular permeability and maintaining vascular homeostasis (78–80). These genes form complexes but also have complex independent roles (81). CCM2 restricts vascular permeability and maintain endothelial barrier function (tight and adherens junctions) by inhibiting Rho A-Rho kinase activity by enhancing Rho A proteasome degradation (79, 82). A lack of CCM2 increases Rho A Rho kinase activity which disrupts endothelial cell-cell contact causing permeability and stress fiber formation„ which is the initial phase in many cardiovascular diseases and characteristic of pathologically activated vascular endothelium. The response of CCM2 may be different in the inflammatory state and the MYOG1 transcription factor may influence this under certain conditions. Endothelial dysfunction reduces the ability of arteries to fully dilate, which stimulates vasodilators from the endothelium like nitric oxide (NO), decreased availability of NO or inactivation due to reactive oxygen species increases dysfunction. An intronic variant in NOS3 is also prioritized as a core causal variant of CAD alongside CCM2 both being important to blood vessel morphology and function (20) with several other rare and common variants in GUCY1A3 PDE5A and PEDE3A (16, 20, 36, 83, 84) highlighting the importance of the NO/cGMP signaling pathway to atherosclerosis and CAD risk.
Increased vascular permeability correlates with neo-angiogenesis (80). CCM proteins also control angiogenesis via Rho-kinase and other signaling pathways (78), CCM2 inhibits angiogenesis, loss of CCM2 causes dramatic angiogenic remodeling abnormalities (85). Adipose tissue is probably the most highly vascularized tissue in the body, as each adipocyte is encircled by capillaries, angiogenesis plays a key role in its function (86). Angiogenesis is driven by a complex interplay of angiogenic factors and inhibitors including vascular endothelial growth factor A (VEGFA). VEGFA is among the top 64 novel CAD loci increasing risk of CAD (OR 1.95 (95% C.I. 1.03–1.06), p = 1.9 × 10−12) (20) and waist to hip ratio adjusted BMI p = 3 × 10−27 (62). CCM proteins and particularly CCM3 can regulate VEGFA expression (80) [typically CCM2 and CCM3 function as a complex, (81)]. Lipid accumulation in adipocytes activates Rho Rho kinase signaling by breaking endothelial cell barriers/stress fiber formation triggering inflammatory changes (87). Vascular remodeling determines the flexibility and metabolic rate of adipose tissue and the communication between adipose and endothelial cells is crucial. Dysfunctional communication in obese individuals contributes to development and progression of T2D including impaired vasodilation, hypoxia and inflammation. CCM2 and VEGFA play roles at the interface of this cellular communication. A more complete understanding of the regulatory networks connecting CCM2 (inhibits angiogenesis) and VEGFA (stimulates angiogenesis, of which there are already targeted drugs), might synergistically increase the resulting therapeutic efficacy to combat obesity and CVD.
GWAs Targets With Functional links to immuno-Metabolism And Coronary Artery Disease
In summary, the three novel GWAs signals implicated in CAD risk play putative roles in immuno-metabolism (Figure 1). TRIM5 has potential to increase innate immunity, inflammation and CAD risk via macrophage infiltration of adipose tissue increasing metabolic stress. Activation of pro-inflammatory immune cells requires a shift to move from energy efficient oxidative phosphorylation to anaerobic glycolysis favoring glucose as substrate. This break or shift occurs when macrophages become polarized (M1) and is associated with nitric oxide production; an M1 effector molecule triggered by increasing oxidative stress. The mutation in CCM2 may reduce oxidative stress to maintain endothelial function, control angiogenesis and vascular remodeling of blood vessels including those surrounding adipose tissue to reduce CAD risk. Inhibiting glycolysis promotes the resolution of inflammation. FNDC3B enhances adipose tissue function by increasing adipogenesis and improving cellular energy efficiency by promoting oxidative phosphorylation and thermogenesis, with PRDM16 and TWIST1 playing similar roles in modifying CAD risk (Figure 1).
Dietary Interventions Connecting Adipogenesis and Metabolic Inflammation as Therapeutic Mechanisms to Reduce Metabolic Risk
Since the recognition that fatty acids can modulate an inflammatory response, e.g., via lipid induced re-programming of macrophage metabolism and inflammation (88) or the NLRP3 inflammasome (89, 90) they have been studied for their immunomodulatory effect on insulin resistance and dysregulated lipid metabolism pathways. Dietary manipulation and certain nutrients have the potential to modulate inflammatory responses. Obesity promotes adipose hypertrophy, with inflammation interacting with the adipogenic process. Pro-inflammatory cytokines IFNG, IL-1β and TNFα, inhibit adipogenesis by downregulating PPARy and C/EBP (91–94) and several dietary components can modulate this effect e.g., reservatrol, flavonoids and polyphenols (95–97). Dietary fat modification to replace saturated fatty acids (SFA) with monounsaturated fatty acids (MUFA) and polyunsaturated fatty acids (PUFA) may provide a potential strategy to lessen inflammation that enhances adipogenesis to attenuate insulin resistance and dysregulated lipid metabolism (6) but the impact of dietary fat modification in humans has varied (98).
Efforts to explain this inter individual variability in response, has focused on the interaction between the genes, metabolites and diet. As diet is the exogenous source of many metabolites, as well as affecting the generation of endogenous metabolites, interactions with the nutritional environment are plausible. However, many putative gene/variant-diet associations have failed to replicate in large studies (99) with various approaches to enhance power (100). Some intriguing examples of specific variant-metabolite interactions modulating disease risk exist from small studies (101, 102). The variant, rs5082 of APOA2 interacts with SFA intake to influence risk of obesity (101). This is modulated through an epigenetic effect on APOA2 regulatory region which promoted an APOA2 expression difference between APOA2 genotypes on a high SFA diet. This selectively dysregulated branched chain and tryptophan metabolic pathways with possible implications for food intake.
Understanding the Regulatory Networks Underlying Metabolic Traits
Attention is shifting to large scale studies integrating transcriptomic and metabolomic data to understand the interplay between genes and metabolites (103). To explore genes playing key roles in immunometabolism more specifically, Nath et al. integrated transcriptomics (focusing on immune networks) and metabolomics using 2,168 individuals from two general population cohorts (104). They identified significant expression quantitative loci in 8 immune gene networks highlighting the genetic foundations of these effects. For example, an eQTL in the ARHGEF3 gene (rs1354034 p = 7 × 10−28) had trans regulatory effects on several genes associated with platelet function and this module had diverse effects on 55 metabolites. Other important core immunometabolic associations related to neutrophil activation and viral response. A subset of the cohort measured repeatedly over 7 years, demonstrated the gene-metabolite effects were temporally stable (104). As long-term OMICs data will be collected on population cohorts over time, these signals are likely to become more reliable.
Identifying the genetic basis to these interactions can be useful therapeutically to modulate the variant itself for individualized treatment or modulate the pathway the variant functions in, which can have much wider implications for population treatment e.g., PCSK9 inhibitors for individual and population level treatment of hypercholesterolemia and CVD risk (34). With better understanding of metabolite-immune interactions, in vivo and interventional studies can be developed to modulate these interactions through existing lipid lowering medications, gut microbe effects or dietary changes. In this way, the immune system itself can be harnessed to reduce the burden of cardiovascular and metabolic disorders (55, 71). With distinct lifestyle strategies now known to differentially affect the way adipose tissue is stored and utilized in the body (105), it is important to understand where and how the drivers of these regulatory networks are acting, which might be under specific situations or locations.
Cellular Specificity of Regulatory Networks
Progress has been made to determine the tissues and cell types underlying disease through the GTex consortium (106). GTex, Roadmap Epigenomics and Functional Annotation of Mammalian Genomes 5 (FANTOM5) provides reference sets for multi-tissue gene expression and epigenomics consistently evaluated on the same individuals with available tissues. Different layers of regulation can exist from post-transcriptional, post-translational, protein-protein interactions and intercellular signaling, mediated through chromatin interactions and expression quantitative trait loci. Assuming that most of the regulation occurs through genes (linked by eQTLs), regulation can occur at the tissue level, broad cell population level or in very specific cell types (106–110). For CVD, multiple cell types or highly specialized cell types may be involved (e.g., vascular, liver, adipose) where cellular networks could have variable expression across cell types (111). The effect of particular variants would then be an average of its effect size in each cell type weighted by cell type importance (11). Mapping GWAs signals to promotors/enhancers measured by cap analysis gene expression (CAGE) found regulation for specific diseases could be turned on/off in similar complex patterns across different cell types. For example, shared cell type specific regulatory networks distinguishing two sub-types of ulcerative colitis could be distinguished based on regulatory signals guided by GWAs signals enriched in either monocytes exposed to inflammatory signals or epithelial cells (108).
To identify cell type specific gene regulation, grouped cell types or deconvolution methods have been used, but the methods tend to be biased to specific cell types or difficult-to-identify less abundant cell types (112). It is possible to calculate the probability a GWAs variant and eQTL tag the same functional effect and infer the tissues where the effect for a trait is likely manifested (113). Single cell RNAseq (scRNAseq) can identify cell-type or context specific eQTLs, but the requirement for fresh tissue and costs limits large scale screening. Mapping monogenic kidney mutations or genome-wide variants associated with chronic kidney disease to gene expression from scRNAseq of 57,979 mouse kidney cells, Park et al. inferred that these variants were expressed in only one particular cell type (114). This suggests, most genetic diseases of the kidney can be traced to single cell types. Using intercellular variation from expression profiles from 25,000 peripheral blood mononuclear cells from 45 donors, scRNAseq identified cell type specific cis-eQTLs. Although gene regulatory networks were highly personal, their approach identified more genes under genetic control or specific cell type in which the effect is most prominent and found examples of SNPs influencing the co-expression of 2 genes (115). scRNAseq has the potential to group and examine the effects of cells along the cell cycle, along a differentiation path (e.g., adipocyte differentiation) or along a response to an environmental stimulus (e.g., inflammatory signaling) (116). With improved understanding of how these genes impact cell types and tissues, more specific targeted interventions can be developed, for instance improved drugs, mobilizing specific fat deposits (105) or nutritional interventions (117).
Conclusions
We have highlighted three novel variants associated with CAD risk which have been prioritized and annotated based on systems genetics approaches including expression quantitative trait analysis and network analysis to infer their functional relevance. These core variants play roles in innate immunity, adipogenesis and endothelial function which drive coronary artery disease and principally in the role that obesity and T2D shape the pathogenesis of CAD through immuno-metabolism. Core variants representing these pathways provide a starting point to potential mechanism that may lead to therapeutic manipulation with further understanding of the regulatory networks connecting these is needed. Given that CAD is a multifactorial disease, it may be possible in the future to develop individual treatment strategies based on these variants or design relevant population level interventions based on the pathways these variants highlight for subsets of people in the population with subtypes of CAD risk related to obesity or T2D.
Author Contributions
MH wrote the paper. YL, CG, and HR discussed and wrote sections, refined and edited the text. All agree with the submission.
Conflict of Interest Statement
The authors declare that the research was conducted in the absence of any commercial or financial relationships that could be construed as a potential conflict of interest.
Funding
HR is the recipient of Science Foundation Ireland (SFI) principal investigator award (11/PI/1119); CG is the recipient of Science Foundation Ireland (SFI) awards (15/IA/3152 and 15/US/B3130). MH and HR were funded by the Joint Programming Healthy Life for a Healthy Diet (JPI HDHL) funded EU Food Biomarkers Alliance FOODBALL (14/JP-HDHL/B3076); MH is also funded through Science Foundation Ireland (SFI) awards (15/IA/3152 and 15/US/B3130). YL and HR were supported by the Irish Department of Agriculture, Food and the Marine, Healthy Beef (13/F/514) programme.
References
1. Leening MJG, Cook NR, Ridker PM. Should we reconsider the role of age in treatment allocation for primary prevention of cardiovascular disease? Eur Heart J. (2017) 38:1542–7. doi: 10.1093/eurheartj/ehw287
2. Tran J, Norton R, Conrad N, Rahimian F, Canoy D, Nazarzadeh M, et al. Patterns and temporal trends of comorbidity among adult patients with incident cardiovascular disease in the UK between 2000 and 2014: A population-based cohort study. PLoS Med. (2018) 15:e1002513. doi: 10.1371/journal.pmed.1002513
3. McMorrow AM, Connaughton RM, Magalhaes T. Personalized cardio-metabolic response to an eight week anti-inflammatory intervention in obese adolescents. Mol Nut Food Res. (2018) 62:e1701008. doi: 10.1002/mnfr.201701008
4. McArdle MA, Finucane OM, Connaughton RM, McMorrow AM, Roche HM. Mechanisms of obesity-induced inflammation and insulin resistance: insights into the emerging role of nutritional strategies. Front Endocrinol (Lausanne). (2013) 4:52. doi: 10.3389/fendo.2013.00052
5. Nazare JA, Smith JD, Borel AL, Haffner SM, Balkau B, Ross R, et al. Ethnic influences on the relations between abdominal sub- cutaneous and visceral adiposity, liver fat, and cardiometabolic risk profile: the International Study of Prediction of Intra- Abdominal Adiposity and Its Relationship With Cardiometabolic Risk/ Intra-Abdominal Adiposity. Am J Clin Nutr. (2012) 96:714–26. doi: 10.3945/ajcn.112.035758
6. Finucane OM, Lyons CL, Murphy AM, Reynolds CM, Klinger R, Healy NP, et al. Monounsaturated fatty acid-enriched high-fat diets impede adipose NLRP3 inflammasome-mediated IL-1β secretion and insulin resistance despite obesity. Diabetes (2015) 64:2116–28. doi: 10.2337/db14-1098
7. Hotamisligil GS. Foundations of immunometabolism and implications for metabolic health and disease. Immunity (2017) 47:406–20. doi: 10.1016/j.immuni.2017.08.009
8. Ghosh S., Bouchard C. Convergence between biological, behavioural and genetic determinants of obesity. Nat Rev Genet. (2017) 18:731–748. doi: 10.1038/nrg.2017.72
9. Giordano A, Frontini A, Cinti S. Convertible visceral fat as a therapeutic target to curb obesity. Nat Rev Drug Discov. (2016) 15:405–24. doi: 10.1038/nrd.2016.31
10. Timpson NJ, Greenwood CMT, Soranzo N, Lawson DJ, Richards JB. Genetic architecture: the shape of the genetic contribution to human traits and disease. Nat Rev Genet. (2018) 19:110–24. doi: 10.1038/nrg.2017.101
11. Boyle EA, Li YI, Pritchard JK. An expanded view of complex traits: from polygenic to omnigenic. Cell (2017) 169:1177–86. doi: 10.1016/j.cell.2017.05.038
12. Fisher R. The correlation between relatives on the supposition of mendelian inheritance. Trans R Soc Edinburgh (1919) 52:399–433. doi: 10.1017/S0080456800012163
13. Illingworth DR, Stein EA, Mitchel YB, Dujovne CA, Frost PH, Knopp RH, et al. Comparative effects of lovastatin and niacin in primary hypercholesterolemia. A prospective trial. Arch Intern Med. (1994) 154:1586–95. doi: 10.1001/archinte.1994.00420140051007
14. chunkert H, König I.R., Kathiresan S. Large-scale association analysis identifies 13 new susceptibility loci for coronary artery disease. Nat Genet. (2011) 43:333–8. doi: 10.1038/ng.784.
15. CARDIoGRAMplusC4D Consortium, Deloukas P, Kanoni S, Willenborg C, Farrall M, Assimes TL, et al. Large-scale association analysis identifies new risk loci for coronary artery disease. Nat Genet. (2013) 45:25–33. doi: 10.1038/ng.2480
16. Nikpay M, Goel A, Won HH, Hall LM, Willenborg C, Kanoni S, et al. A comprehensive 1000 Genomes–based genome-wide association meta-analysis of coronary artery disease. Nat Genet. (2015) 47:1121–30. doi: 10.1038/ng.3396
17. Verweij N, Eppinga RN, Hagemeijer Y, van der Harst P. Identification of 15 novel risk loci for coronary artery disease and genetic risk of recurrent events, atrial fibrillation and heart failure. Sci Rep. (2017) 7:2761. doi: 10.1038/s41598-017-03062-8
18. Howson JMM, Zhao W, Barnes DR, Ho WK, Young R, Paul DS, et al. Fifteen new risk loci for coronary artery disease highlight arterial-wall-specific mechanisms. Nat Genet. (2017) 49:1113–9. doi: 10.1038/ng.3874
19. Nelson CP, Goel A, Butterworth AS, Kanoni S, Webb TR, Marouli E, et al. Association analyses based on false discovery rate implicate new loci for coronary artery disease. Nat Genet. (2017) 49:1385–91. doi: 10.1038/ng.3913
20. van der Harst P, Verweij N. Identification of 64 novel genetic loci provides an expanded view on the genetic architecture of coronary artery disease. Circ Res. (2018) 122:433–43. doi: 10.1161/CIRCRESAHA.117.312086
21. Erdmann J, Kessler T, Munoz Venegas L, Schunkert H. A decade of genome-wide association studies for coronary artery disease: the challenges ahead. Cardiovasc Res. (2018) 114:1241–57. doi: 10.1093/cvr/cvy084
22. Iotchkova V, Huang J, Morris JA, Jain D, Barbieri C, Walter K, et al. Discovery and refinement of genetic loci associated with cardiometabolic risk using dense imputation maps. Nat Genet. (2016) 48:1303–12. doi: 10.1038/ng.3668
23. Kichaev G, Yang WY, Lindstrom S, Hormozdiari F, Eskin E, Price AL, et al. Integrating functional data to prioritize causal variants in statistical fine-mapping studies. PLoS Genet. (2014) 10:e1004722. doi: 10.1371/journal.pgen.1004722
24. Servin B, Stephens M. Imputation-based analysis of association studies: candidate regions and quantitative traits. PLoS Genet. (2007) 3:e114. doi: 10.1371/journal.pgen.0030114
25. Bottolo L, Chadeau-Hyam M, Hastie DI, Zeller T, Liquet B, Newcombe P, et al. GUESSing polygenic associations with multiple phenotypes using a gpu-based evolutionary stochastic search algorithm. PLoS Genet. (2013) 9:e1003657. doi: 10.1371/journal.pgen.1003657
26. Benner C, Spencer CCA, Havulinna AS, Salomaa V, Ripatti S, Pirinen M. FINEMAP: efficient variable selection using summary data from genome-wide association studies. Bioinformatics (2016) 32:1493–501. doi: 10.1093/bioinformatics/btw018
27. Chen W, Larrabee BR, Ovsyannikova IG, Kennedy RB, Haralambieva IH, Poland GA, et al. Fine mapping causal variants with an approximate Bayesian method using marginal test statistics. Genetics (2015). 200:719–36. doi: 10.1534/genetics.115.176107
28. Kichaev G, Roytman M, Johnson R, Eskin E, Lindstrom S, Kraft P, et al. Improved methods for multi-trait fine mapping of pleiotropic risk loci. Bioinformatics (2017) 33:248–55. doi: 10.1093/bioinformatics/btw615
29. Wu C, Pan W. Integration of enhancer-promoter interactions with GWAS summary results identifies novel schizophrenia-associated genes and pathways. Genetics (2018) 209:699–709. doi: 10.1534/genetics.118.300805
30. Schaid DJ, Chen W, Larson NB. From genome-wide associations to candidate causal variants by statistical fine-mapping. Nat Rev Genet. (2018) 19:1–14. doi: 10.1038/s41576-018-0016-z
31. Wu Y, Zeng J, Zhang F, Zhu Z, Qi T, Zheng Z, et al. Integrative analysis of omics summary data reveals putative mechanisms underlying complex traits. Nat Commun. (2018) 9:918. doi: 10.1038/s41467-018-03371-0
32. Myocardial Infarction Genetics and CARDIoGRAM Exome Consortia Investigators, Stitziel NO, Stirrups KE, Masca NG, Erdmann J, Ferrario PG, et al. Coding variation in ANGPTL4, LPL and SVEP1 and the risk of coronary disease. N Eng J Med. (2016) 373:1134–44. doi: 10.1056/NEJMoa1507652
33. Ridker PM, Revkin J, Amarenco P, Brunell R, Curto M, Civeira F, et al. Cardiovascular efficacy and safety of bococizumab in high-risk patients. N Engl J Med. (2017) 376:1527–39. doi: 10.1056/NEJMoa1701488
34. Ference BA, Robinson JG, Brook RD, Catapano AL, Chapman MJ, Neff DR, et al. Variation in PCSK9 and HMGCR and risk of cardiovascular disease and diabetes. N Engl J Med. (2016) 375:2144–53. doi: 10.1056/NEJMoa1604304
35. Claussnitzer M, Dankel SN, Kim K-H, Quon G, Meuleman W, Haugen C, et al. FTO obesity variant circuitry and adipocyte browning in humans. N Engl J Med. (2015) 373:895–907. doi: 10.1056/NEJMoa1502214
36. Kessler T, Wobst J, Wolf B, Eckhold J, Vilne B, Hollstein R, et al. Functional characterization of the GUCY1A3 coronary artery disease risk locus. Circulation (2017) 136:476–89. doi: 10.1161/CIRCULATIONAHA.116.024152
37. Prokop JW, Yeo NC, Ottmann C, Chhetri SB, Florus KL, Ross EJ, et al. Characterization of coding/noncoding variants for SHROOM3 in patients with CKD. J Am Soc Nephrol. (2018) 29:1525–35. doi: 10.1681/ASN.2017080856
38. Nguyen KD, Qiu Y, Cui X, Goh YPS, Mwangi J, Mukundan L, et al. HHS Public Access. (2012) 480:104–8. Available online at: http://yunliweb.its.unc.edu/HUGIn
39. Westra HJ, Peters MJ, Esko T, Yaghootkar H, Schurmann C, Kettunen J, et al. Systematic identification of trans eQTLs as putative drivers of known disease associations. Nat Genet. (2013) 45:1238–43. doi: 10.1038/ng.2756
40. Krämer A, Green J, Pollard J Jr, Tugendreich S. Causal analysis approaches in ingenuity pathway analysis. Bioinformatics (2014) 30:523–30. doi: 10.1093/bioinformatics/btt703
41. Astle WJ, Elding H, Jiang T, Allen D, Ruklisa D, Mann AL, et al. The allelic landscape of human blood cell trait variation and links to common complex disease. Cell (2016) 167:1415–29.e19. doi: 10.1016/j.cell.2016.10.042
42. Verbanck M, Chen CY, Neale B, Do R. Detection of widespread horizontal pleiotropy in causal relationships inferred from Mendelian randomization between complex traits and diseases. Nat Genet. (2018) 50:693–8. doi: 10.1038/s41588-018-0099-7
43. Pertel T, Hausmann S, Morger D, Züger S, Guerra J, Lascano J, et al. TRIM5 is an innate immune sensor for the retrovirus capsid lattice. Nature (2011) 472:361–5. doi: 10.1038/nature09976
44. Chang T-H, Yoshimi R, Ozato K. Tripartite Motif (TRIM) 12c, a mouse homolog of TRIM5, is a ubiquitin ligase that stimulates type I IFN and NF-κB pathways along with TNFR-associated factor 6. J Immunol. (2015) 195:5367–79. doi: 10.4049/jimmunol.1402064
45. Ralston JC, Lyons CL, Kennedy EB, Kirwan AM, Roche HM. Fatty acids and NLRP3 inflammasome–mediated inflammation in metabolic tissues. Annu Rev Nutr. (2017) 37:77–102. doi: 10.1146/annurev-nutr-071816-064836
46. van Diepen JA, Thiem K, Stienstra R, Riksen NP, Tack CJ, Netea MG. Diabetes propels the risk for cardiovascular disease: sweet monocytes becoming aggressive? Cell Mol Life Sci. (2016) 73:4675–84. doi: 10.1007/s00018-016-2316-9
47. Kratz M, Coats BR, Hisert KB, Hagman D, Mutskov V, Peris E, et al. Metabolic dysfunction drives a mechanistically distinct proinflammatory phenotype in adipose tissue macrophages. Cell Metab. (2014) 20:614–25. doi: 10.1016/j.cmet.2014.08.010
48. Genecards. Olfactory Receptor Family. Available online at: https://www.genecards.org/Search/Keyword?queryString=olfactory%20 (accessed April 9, 2018).
49. Riera CE, Tsaousidou E, Halloran J, Follett P, Hahn O, Pereira MMA, et al. The sense of smell impacts metabolic health and obesity. Cell Metab. (2017) 26:198–211.e5. doi: 10.1016/j.cmet.2017.06.015
50. Grarup N, Moltke I, Andersen MK, Dalby M, Vitting-Seerup K, Kern T, et al. Loss-of-function variants in ADCY3 increase risk of obesity and type 2 diabetes. Nat Genet. (2018) 50:172–4. doi: 10.1038/s41588-017-0022-7
51. Siljee JE, Wang Y, Bernard AA, Ersoy BA, Zhang S, Marley A, et al. Subcellular localization of MC4R with ADCY3 at neuronal primary cilia underlies a common pathway for genetic predisposition to obesity. Nat Genet. (2018) 50:180–5. doi: 10.1038/s41588-017-0020-9
52. Ridker PM, Everett BM, Thuren T, MacFadyen JG, Chang WH, Ballantyne C, et al. Antiinflammatory therapy with canakinumab for atherosclerotic disease. N Engl J Med. (2017) 377:1119–31. doi: 10.1056/NEJMoa1707914
53. Crean D, Godson C. Specialised lipid mediators and their targets. Semin Immunol. (2015) 27:169–76. doi: 10.1016/j.smim.2015.05.002
54. Brennan EP, Mohan M, McClelland A, de Gaetano M, Tikellis C, Marai M, et al. Lipoxins protect against inflammation in diabetes-associated atherosclerosis. Diabetes (2018) 67:db171317. doi: 10.2337/db17-1317
55. Börgeson E, Johnson AMF, Lee YS, Till A, Syed GH, Ali-Shah ST, et al. Lipoxin A4 attenuates obesity-induced adipose inflammation and associated liver and kidney disease. Cell Metab. (2015) 22:125–37. doi: 10.1016/j.cmet.2015.05.003
56. O'Reilly M, Dillon E, Guo W, Finucane O, McMorrow A, Murphy A, et al. High-density lipoprotein proteomic composition, and not efflux capacity, reflects differential modulation of reverse cholesterol transport by saturated and monounsaturated fat diets. Circulation (2016) 133:1838–50. doi: 10.1161/CIRCULATIONAHA.115.020278
57. Connaughton RM, McMorrow AM, McGillicuddy FC, Lithander FE, Roche HM. Impact of anti-inflammatory nutrients on obesity-associated metabolic-inflammation from childhood through to adulthood. Proc Nutr Soc. (2016) 75:115–24. doi: 10.1017/S0029665116000070
58. Tominaga K, Kondo C, Johmura Y, Nishizuka M, Imagawa M. The novel gene fad104, containing a fibronectin type III domain, has a significant role in adipogenesis. FEBS Lett. (2004) 577:49–54. doi: 10.1016/j.febslet.2004.09.062
59. Kishimoto K, Kato A, Osada S, Nishizuka M, Imagawa M. Fad104, a positive regulator of adipogenesis, negatively regulates osteoblast differentiation. Biochem Biophys Res Commun. (2010) 397:187–91. doi: 10.1016/j.bbrc.2010.05.077
60. Peng Y, Li H, Li X, Yu S, Xiang H, Peng J, et al. MicroRNA-215 impairs adipocyte differentiation and co-represses FNDC3B and CTNNBIP1. Int J Biochem Cell Biol. (2016) 79:104–12. doi: 10.1016/j.biocel.2016.08.014
61. Wood AR, Esko T, Yang J, Vedantam S, Pers TH, Gustafsson S, et al. Defining the role of common variation in the genomic and biological architecture of adult human height. Nat Genet. (2014) 46:1173–86. doi: 10.1038/ng.3097
62. Shungin D, Winkler T, Croteau-Chonka D, Ferreira T, Locke A, Mägi R, et al. New genetic loci link adipose and insulin biology to body fat distribution. Nature (2015) 518:187–96. doi: 10.1038/nature14132
63. den Hoed M, Eijgelsheim M, Esko T, Brundel BJ, Peal DS, Evans DM, et al. Identification of heart rate associated loci and their effects on cardiac conduction and rhythm disorders. Nat Genet. (2013) 45:621–31. doi: 10.1038/ng.2610
64. Choquet H, Thai KK, Yin J, Hoffmann TJ, Kvale MN, Banda Y, et al. A large multi-ethnic genome-wide association study identifies novel genetic loci for intraocular pressure. Nat Commun. (2017) 8:2108. doi: 10.1038/s41467-017-01913-6
65. Gao XR, Huang H, Nannini DR, Fan F, Kim H. Genome-wide association analyses identify new loci influencing intraocular pressure. Hum Mol Genet. (2018) 27:2205–13. doi: 10.1093/hmg/ddy111
66. Fox CS, Liu Y, White CC, Feitosa M, Smith AV, Heard-Costa N, et al. Genome-wide association for abdominal subcutaneous and visceral adipose reveals a novel locus for visceral fat in women. PLoS Genet. (2012) 8:e1002695. doi: 10.1371/journal.pgen.1002695
67. Lu Y, Day FR, Gustafsson S, Buchkovich ML, Na J, Bataille V, et al. New loci for body fat percentage reveal link between adiposity and cardiometabolic disease risk. Nat Commun. (2016) 7:10495. doi: 10.1038/ncomms10495
68. Kishimoto K, Nishizuka M, Katoh D, Kato A, Osada S, Imagawa M. FAD104, a regulatory factor of adipogenesis, acts as a novel regulator of calvarial bone formation. J Biol Chem. (2013) 288:31772–83. doi: 10.1074/jbc.M113.452961
69. Wang B, Wood IS, Trayhurn P. Hypoxia induces leptin gene expression and secretion in human preadipocytes: differential effects of hypoxia on adipokine expression by preadipocytes. J Endocrinol. (2008) 198:127–34. doi: 10.1677/JOE-08-0156
70. Schulz TJ, Huang TL, Tran TT, Zhang H, Townsend KL, Shadrach JL, et al. Identification of inducible brown adipocyte progenitors residing in skeletal muscle and white fat. Proc Natl Acad Sci USA. (2011) 108:143–8. doi: 10.1073/pnas.1010929108
71. Gustafson B, Hammarstedt A, Hedjazifar S, Hoffmann JM, Svensson PA, Grimsby J, et al. BMP4 and BMP antagonists regulate human white and beige adipogenesis. Diabetes (2015) 64:1670–81. doi: 10.2337/db14-1127
72. Boström P, Wu J, Jedrychowski MP, Korde A, Ye L, Lo JC, et al. A PGC1a dependent myokine that derives browning of white fat and thermogenesis. Nature (2012) 481:463–8. doi: 10.1038/nature10777
73. Pérez-Sotelo D, Roca-Rivada A, Baamonde I, Baltar J, Castro AI, Domínguez E, et al. Lack of adipocyte-Fndc5/irisin expression and secretion reduces thermogenesis and enhances adipogenesis. Sci Rep. (2017) 7:16289. doi: 10.1038/s41598-017-16602-z
74. van Marken Lichtenbelt WD. Cold-activated brown adipose tissue in healthy men. Yearb Endocrinol. (2010) 2010:115–7. doi: 10.1056/NEJMoa0808718
75. Pan D, Fujimoto M, Lopes A, Wang YX. Twist-1 is a PPARδ-inducible, negative-feedback regulator of PGC-1α in brown fat metabolism. Cell (2009) 137:73–86. doi: 10.1016/j.cell.2009.01.051
76. Franzen O, Ermel R, Cohain A, Akers N, Di Narzo A, Talukdar HA, et al. Cardiometabolic risk loci share downstream cis- and trans-gene regulation across tissue and diseases. The Stockholm-Tartu Atherosclerosis Reverse Network Engineering Task (STARNET) study. Science (2016) 353:827–31. doi: 10.1126/science.aad6970
77. Liquori CL, Berg MJ, Siegel AM, Huang E, Zawistowski JS, Stoffer T, et al. Mutations in a gene encoding a novel protein containing a phosphotyrosine-binding domain cause type 2 cerebral cavernous malformations. Am J Hum Genet. (2003) 73:1459–64. doi: 10.1086/380314
78. Fischer A, Zalvide J, Faurobert E, Albiges-Rizo C, Tournier-Lasserve E. Cerebral cavernous malformations: from CCM genes to endothelial cell homeostasis. Trends Mol Med. (2013) 19:302–8. doi: 10.1016/j.molmed.2013.02.004
79. Crose LES, Hilder TL, Sciaky N, Johnson GL. Cerebral cavernous malformation 2 protein promotes smad ubiquitin regulatory factor 1-mediated RhoA degradation inendothelial cells. J Biol Chem. (2009) 284:13301–5. doi: 10.1074/jbc.C900009200
80. DiStefano PV, Kuebel JM, Sarelius IH, Glading AJ. KRIT1 protein depletion modifies endothelial cell behavior via increased vascular endothelial growth factor (VEGF) Signaling. J Biol Chem. (2014) 289:33054–65. doi: 10.1074/jbc.M114.582304
81. Draheim KM, Li X, Zhang R, Fisher OS, Villari G, Boggon TJ, et al. CCM2-CCM3 interaction stabilizes their protein expression and permits endothelial network formation. J Cell Biol. (2015) 208:987. doi: 10.1083/jcb.201407129
82. Whitehead KJ, Chan AC, Navankasattusas S, Koh W, London NR, Ling J, et al. The cerebral cavernous malformation signaling pathway promotes vascular integrity via Rho GTPases. Nat Med. (2009) 15:177–84. doi: 10.1038/nm.1911
83. Erdmann J, Stark K, Esslinger UB, Rumpf PM, Koesling D, DeWit C, et al. Dysfunctional nitric oxide signalling increases risk of myocardial infarction. Nature (2013) 504:432–6. doi: 10.1038/nature12722
84. Klarin D, Zhu QM, Emdin CA, Chaffin M, Horner S, McMillan BJ, et al. Genetic analysis in UK Biobank links insulin resistance and transendothelial migration pathways to coronary artery disease. Nat Genet. (2017) 49:1392–7. doi: 10.1038/ng.3914
85. Boulday G, Blecon A, Petit N, Chareyre F, Garcia LA, Niwa-Kawakita M, et al. Tissue-specific conditional CCM2 knockout mice establish the essential role of endothelial CCM2 in angiogenesis: implications for human cerebral cavernous malformations. Dis Model Mech. (2009) 2:168–77. doi: 10.1242/dmm.001263
86. Cao Y. (2010). Adipose tissue angiogenesis as a therapeutic target for obesity and metabolic diseases. Nat Rev Drug Discov. (2010) 9:107–15. doi: 10.1038/nrd3055
87. Hara Y, Wakino S, Tanabe Y, Saito M, Tokuyama H, Washida N, et al. Rho and Rho-kinase activity in adipocytes contributes to a vicious cycle in obesity that may involve mechanical stretch. Sci Signal. (2011) 4:ra3. doi: 10.1126/scisignal.2001227
88. Lancaster GI, Langley KG, Berglund NA, Kammoun HL, Reibe S, Estevez E, et al. Evidence that TLR4 is not a receptor for saturated fatty acids but mediates lipid-induced inflammation by reprogramming macrophage metabolism. Cell Metab. (2018) 27:1096–110.e5. doi: 10.1016/j.cmet.2018.03.014
89. Reynolds CM, Mcgillicuddy FC, Harford KA, Finucane OM, Mills KHG, Roche HM. Dietary saturated fatty acids prime the NLRP3 inflammasome via TLR4 in dendritic cells-implications for diet-induced insulin resistance. Mol Nutr Food Res. (2012) 56:1212–22. doi: 10.1002/mnfr.201200058
90. Wen H, Gris D, Lei Y, Jha S, Zhang L, Huang MTH, et al. Fatty acid-induced NLRP3-ASC inflammasome activation interferes with insulin signaling. Nat Immunol. (2011) 12:408–15. doi: 10.1038/ni.2022
91. McGillicuddy FC, Harford KA, Reynolds CM, Oliver E, Claessens M, Mills KHG, et al. Lack of interleukin-1 receptor I (IL-1RI) protects mice from high-fat diet-induced adipose tissue inflammation coincident with improved glucose homeostasis. Diabetes (2011) 60:1688–98. doi: 10.2337/db10-1278
92. Isakson P, Hammarstedt A, Gustafson B, Smith U. Role of Wnt, Tumor Necrosis Factor-α, and Inflammation. Diabetes (2009) 58:1550–7. doi: 10.2337/db08-1770
93. Vidal C, Bermeo S, Li W, Huang D, Kremer R, Duque G. Interferon gamma inhibits adipogenesis in vitro and prevents marrow fat infiltration in oophorectomized mice. Stem Cells (2012) 30:1042–8. doi: 10.1002/stem.1063
94. Zuniga LA, Shen W-J, Joyce-Shaikh B, Pyatnova EA, Richards AG, Thom C, et al. IL-17 regulates adipogenesis, glucose homeostasis, and obesity. J Immunol. (2010) 185:6947–59. doi: 10.4049/jimmunol.1001269
95. Rayalam S, Yang JY, Ambati S, Della-Fera MA, Baile CA. Resveratrol induces apoptosis and inhibits adipogenesis in 3T3-L1 adipocytes. Pytother Res. (2008) 22:1367–71. doi: 10.1002/ptr.2503
96. Kim GS, Park HJ, Woo JH, Kim MK, Koh PO, Min W, et al. Citrus aurantium flavonoids inhibit adipogenesis through the Akt signaling pathway in 3T3-L1 cells. BMC Complement Altern Med. (2012) 12:31. doi: 10.1186/1472-6882-12-31
97. Ejaz A, Wu D, Kwan P, Meydani M. Curcumin inhibits adipogenesis in 3T3-L1 adipocytes and angiogenesis and obesity in C57/BL mice. J Nutr. (2009) 139:919–25. doi: 10.3945/jn.108.100966
98. Lyons CL, Kennedy EB, Roche HM. Metabolic inflammation-differential modulation by dietary constituents. Nutrients (2016) 8:e247. doi: 10.3390/nu8050247
99. Li SX, Imamura F, Ye Z, Schulze MB, Zheng J, Ardanaz E, et al. Interaction between genes and macronutrient intake on the risk of developing type 2 diabetes; systematic review and findings from European Prospective Investigation into Cancer (EPIC)-InterAct. Am J Clin Nutr (2017) 5:263–75. doi: 10.3945/ajcn.116.150094
100. Shungin D, Deng WQ, Varga TV, Luan J, Mihailov E, Metspalu A, et al. Ranking and characterization of established BMI and lipid associated loci as candidates for gene-environment interactions. PLoS Genet. (2017) 13:e1006812. doi: 10.1371/journal.pgen.1006812
101. Lai C-Q, Smith CE, Parnell LD, Lee Y-C, Corella D, Hopkins P, et al. Epigenomics and metabolomics reveal the mechanism of the APOA2-saturated fat intake interaction affecting obesity. Am J Clin Nutr. (2018) 108:188–200. doi: 10.1093/ajcn/nqy081
102. Weng LC, Guan W, Steffen LM, Pankow JS, Pankratz N, Chen MH, et al. Pleiotropic effects of n-6 and n-3 fatty acid-related genetic variants on circulating hemostatic variables. Thromb Res. (2018) 168:53–9. doi: 10.1016/j.thromres.2018.05.032
103. Bartel J, Krumsiek J, Schramm K, Adamski J, Gieger C, Herder C, et al. The human blood metabolome-transcriptome interface. PLoS Genet. (2015) 11:e1005274. doi: 10.1371/journal.pgen.1005274
104. Nath AP, Ritchie SC, Byars SG, Fearnley LG, Havulinna AS, Joensuu A, et al. An interaction map of circulating metabolites, immune gene networks, and their genetic regulation. Genome Biol. (2017) 18:146. doi: 10.1186/s13059-017-1279-y
105. Gepner Y, Shelef I, Schwarzfuchs D, Zelicha H, Tene L, Meir AY, et al. Effect of distinct lifestyle interventions on mobilization of fat storage pools: CENTRAL magnetic resonance imaging randomized controlled trial. Circulation (2018) 137:1143–57. doi: 10.1161/CIRCULATIONAHA.117.030501
106. Ongen H, Brown AA, Delaneau O, Panousis NI, Nica AC, Dermitzakis ET. Estimating the causal tissues for complex traits and diseases. Nat Genet. (2017) 49:1676–83. doi: 10.1038/ng.3981
107. Shu L, Chan KHK, Zhang G, Huan T, Kurt Z, Zhao Y, et al. Shared genetic regulatory networks for cardiovascular disease and type 2 diabetes in multiple populations of diverse ethnicities in the United States. PLoS Genet. (2017) 13:1–25. doi: 10.1371/journal.pgen.1007040
108. Baillie JK, Bretherick A, Haley CS, Clohisey S, Gray A, Neyton LPA, et al. Shared activity patterns arising at genetic susceptibility loci reveal underlying genomic and cellular architecture of human disease. PLoS Comput Biol. (2018) 14:1–24. doi: 10.1371/journal.pcbi.1005934
109. Fagny M, Paulson JN, Kuijjer ML, Sonawane AR, Chen C-Y, Lopes-Ramos CM, et al. Exploring regulation in tissues with eQTL networks. Proc Natl Acad Sci USA. (2017) 114:E7841–50. doi: 10.1073/pnas.1707375114
110. Saha A, Kim Y, Gewirtz ADH, Jo B, Gao C, McDowell IC, et al. Co-expression networks reveal the tissue-specific regulation of transcription and splicing. Genome Res. (2017) 1843–58. doi: 10.1101/gr.216721.116
111. Sonawane AR, Platig J, Fagny M, Chen CY, Paulson JN, Lopes-Ramos CM, et al. Understanding tissue-specific gene regulation. Cell Rep. (2017) 21:1077–88. doi: 10.1016/j.celrep.2017.10.001
112. Newman AM, Liu CL, Green MR, Gentles AJ, Feng W, Xu Y, et al. Robust enumeration of cell subsets from tissue expression profiles. Nat Methods (2015) 15:453–7. doi: 10.1038/nmeth.3337
113. Brown AA, Viñuela A, Delaneau O, Spector TD, Small KS, Dermitzakis ET. Predicting causal variants affecting expression by using whole-genome sequencing and RNA-seq from multiple human tissues. Nat Genet. (2017) 49:1747–51. doi: 10.1038/ng.3979
114. Park J, Shrestha R, Qiu C, Kondo A, Huang S, Werth M, et al. Single-cell transcriptomics of the mouse kidney reveals potential cellular targets of kidney disease. Science (2018) 360:758–63. doi: 10.1126/science.aar2131
115. van der Wijst MG, Brugge H, de Vries DH, Deelen P, Swertz MA, Franke L. Single-cell RNA sequencing identifies celltype-specific cis-eQTLs and co-expression QTLs. Nat Genet. (2018) 50:493–7. doi: 10.1038/s41588-018-0089-9
116. Macaulay IC, Ponting CP, Voet T. Single-cell multiomics: multiple measurements from single cells. Trends Genet. (2017) 33:155–68. doi: 10.1016/j.tig.2016.12.003
Keywords: GWAS, immuno-metabolism, coronary artery disease, nutrition, omnigenic
Citation: Hughes MF, Lenighan YM, Godson C and Roche HM (2018) Exploring Coronary Artery Disease GWAs Targets With Functional Links to Immunometabolism. Front. Cardiovasc. Med. 5:148. doi: 10.3389/fcvm.2018.00148
Received: 07 May 2018; Accepted: 01 October 2018;
Published: 06 November 2018.
Edited by:
Jeanette Erdmann, Universität zu Lübeck, GermanyReviewed by:
Wolfgang Lieb, Christian-Albrechts-Universität zu Kiel, GermanyClint L. Miller, University of Virginia, United States; Loreto Munoz Venegas, Universität zu Lübeck, Germany
Copyright © 2018 Hughes, Lenighan, Godson and Roche. This is an open-access article distributed under the terms of the Creative Commons Attribution License (CC BY). The use, distribution or reproduction in other forums is permitted, provided the original author(s) and the copyright owner(s) are credited and that the original publication in this journal is cited, in accordance with accepted academic practice. No use, distribution or reproduction is permitted which does not comply with these terms.
*Correspondence: Maria F. Hughes, maria.hughes@ucd.ie